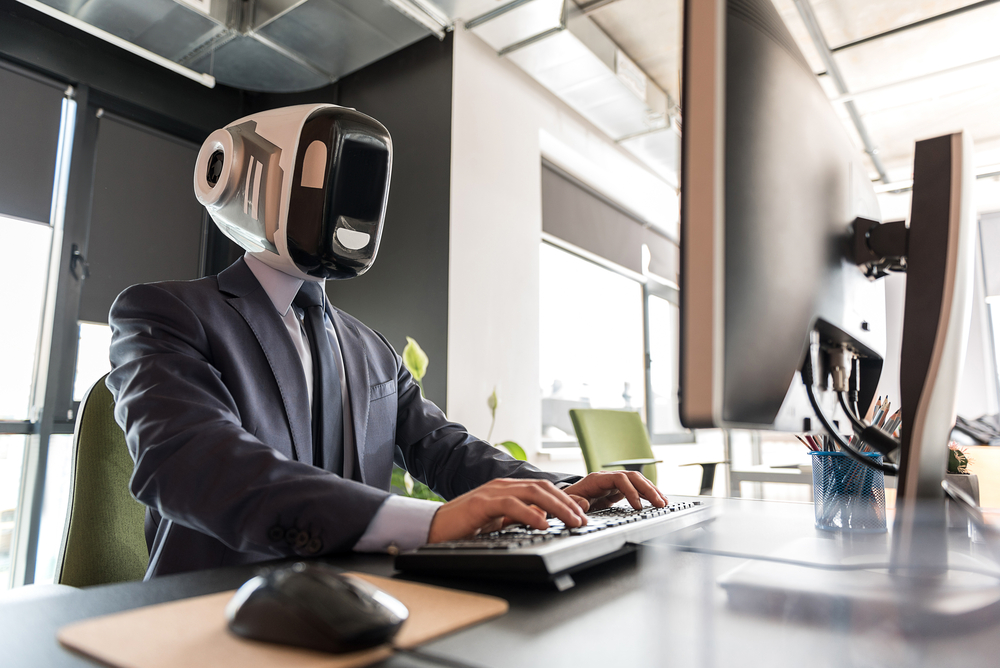
Some time ago we found our old materials, which we used to teach the first streams in our machine learning courses at the
School of Data and compared them with the current ones. We were surprised how much we added and changed in 5 years of study. Realizing why we did it and how, in fact, the approach to solving the problems of Data Science has changed, we decided to write this publication.
We started training with the basic methods and algorithms of machine learning, told how to apply them in practice, how to select parameters, how to clean and prepare data, how to measure quality. We believed (and still believe) that the preparation of a full-fledged data scientist should include not only methods of classical machine learning, but also methods for analyzing graphs (social networks, SNA), analyzing texts, working with neural networks and big data (Big Data).
')
Thus, at the end we had an expert in a wide field of Data Science, who was able to apply an extensive arsenal of methods in practice. We took the same specialists into our business. First, in the company where we worked and supervised the relevant areas, and then into our product development business based on machine learning,
Data Studio .
But later we realized that this is not only not enough for the successful implementation of Data Science projects, but that this is not even the main thing.
The approach at the beginning of the practice of Data Science and, to be honest, many analysts still have the following: give me the data, I clear it, make a feature vector, divide it into a training and test sample, run several ML algorithms, and here is the result.
Does this approach have the right to life?
Yes, it does, but where the subject area is already well studied and there is already a good accumulated experience of applying analytics. Examples? Bank scoring, outflow from operators, cross-sales (Next Best Offer) in retail, banks, telecoms, stock performance forecast in retail, stock forecast. This list can be continued.
And now let's imagine other areas: forecast of arrival time in multimodal transportation (ship, train, truck): what signs will you use? Type of cargo, weight of cargo, the presence of certain sorting nodes? And if you think? Maybe some simpler and more obvious signs (even without machine learning models) will give you significant accuracy?
Or you need to predict the sensitivity of large customers to price changes for certain products. How to determine the elasticity? What exactly will you predict?
But is it necessary to build a model, if the production process is then changed anyway?
It turns out that you need to be able to work in new subject areas of application of analytics, as in well-studied areas, there are already many developments and this is already the “red ocean”.
What do you need to go into new areas with analytics?
To do this, you need to be able to deeply understand the subject area of ​​a specific process, which is often not described. Understand what data is generally needed in principle, understand exactly what business is doing here. Do you need analytics here, do you need any predictive algorithms, do you need to change the business process, are there operational levers (what’s the point of predicting equipment shutdown if there’s no way to avoid it?).
If you add up, you need the following things:
- Analytical approach, the ability to formulate and test hypotheses
- Understanding of the principles and features of the business and individual processes
- Understanding the economics of processes
- Understanding of technology
- Ability to bind data to business processes
And, if to tear away from machine learning, what sphere can do it best? That's right - management consulting. And where this is taught using the so-called case method (many examples from different business situations) - this is true, in the MBA (master of business administration) courses.
Thus, it turns out that the ideal Data Scientist is an MBA graduate with consulting experience who has completed machine learning courses.
This is, of course, a brute force, but it’s true that among the contractors are those who have a culture of analytical thinking developed inside at the level of processes and standards, at the level of selection and training of employees. We also follow the same approach in our
Data Studio . And, which is logical, we laid down the same approach in our studies at the
School of Data .
You can argue. After all, the above written is more applicable in consulting, where every time you don’t know in advance which project domain will come from. And what about large companies, where is the area basically delineated?
In companies, we see all the same specifics described above, and the need for the analyst and the whole team to understand the business, the need for responsibility for the final result.
It is for this reason that in large companies we now see a trend in the specialization of Data Science divisions and the transfer of analytics functions from a centralized division, one for the whole company, to a business function, that is, closer to the business. With such specialization, the analyst's ability to quickly understand a new business and offer really applicable solutions, rather than models, is a competitive advantage.
What exactly has changed in our curriculum? We have previously taught on the basis of practical cases. Changed the structure and nature of cases. Previously, our cases looked like tasks on Kaggle: this is the task, this is the target variable, this is the quality metric, this is the data.
Now the task sounds different: here is the task in terms of the client, here is a description of the client process. Formulate the task of analytics, suggest a quality metric, evaluate the expediency of using analytics, calculate the economic effect, suggest methods, formulate a request for the data you need. But then everything is as usual: clean the data, build a model and so on. And we give such examples from completely different areas, the benefit, the presence of our own consulting in this area greatly expands the set of available tasks that we solved on our own experience.
But the discipline of an analytical approach is not only the practice of case studies. We also teach standard frameworks (basic analysis patterns) used in consulting. We also added to the training the process of developing an analytical product that we follow in the classroom, from business analysis to presenting the results to the customer and planning the deployment of a productive solution including stages, roles, key decision points and points of interaction with the customer.
We give a separate role to the presentations - too often we have seen a gap between the thoughts of analysts and the perception of these thoughts by the customer’s employees.
In general, we believe that the task of preparing a data scientist now is not how to prepare a specialist for existing areas (there are already quite a few courses for this and this has largely become the commodity), but to prepare an expert researcher to work in new areas Digitalization is just coming.
Well, and, as usual - the beginning of a new course in our
School of Data on September 16. We take orders for new projects to
Data Studio all the time, just as we recruit employees (see the open vacancies section).
PS We have updated our site a bit to make it more convenient. Therefore, do not be surprised at the new look.