Toloka is the largest source of human-tagged data for machine learning tasks. Every day in Toloka tens of thousands of performers produce more than 5 million estimates. For any research and experiments related to machine learning, large amounts of qualitative data are needed. Therefore, we begin to publish open datasets for academic research in various subject areas.
Today we will share links to the first public datasets and talk about how they were going. And we'll show you where it’s right to put emphasis on the name of our platform.
An interesting fact: the more complex the technology of artificial intelligence, the more it needs human help. People lay out images into categories to train computer vision; People evaluate the relevance of pages to search queries. people convert speech to text so that the voice assistant learns to understand and speak. Human evaluations are necessary for the machine to continue to work without people and better people.
Previously, many companies collected such assessments solely with the help of specially trained staff assessors. But over time, tasks in the field of machine learning became too many, and the tasks themselves for the most part ceased to require special knowledge and experience. So there was a demand for help from the “crowd”. But to find a large number of random performers on their own and not everyone can work with them. Crowdsourcing platforms solve this problem.
')
Yandex.Toloka (correctly pronounced this way, with an emphasis on the last syllable) is one of the world's largest crowdsourcing platforms. We have over 4 million registered users. More than 500 projects collect estimates every day with our help. A pleasant fact: this year, at the Data Labeling section at the Data Fest conference, all six speakers from different companies mentioned Toloka as a source of markup for their projects.
A lot has already been said about Toloki’s use in business. Today we will talk about our other direction, which we consider no less useful.
Studies in Toloka
Crowdsourcing and, in general, the task of mass collection of human markup exists about as much as the industrial application of machine learning. This is an area in which huge amounts of money are spent on all technology companies. But at the same time, for some reason, it was she who was greatly under-invested from the point of view of research: there are relatively few serious studies and articles about working with crowd, unlike other areas of ML.
We would like to change that. Our team sees Toloka not only as a tool for solving applied problems, but also as a platform for scientific research in various subject areas.
Public datasets Toloki
We want to support the scientific community and attract researchers to Toloka, so we are starting to publish data sets for non-commercial, academic purposes. They may be of interest to researchers of different directions: here are chat bots, and data for testing models of aggregation of the verdicts of pushers, for linguistic research, for computer vision problems. Tell about them:
Toloka Persona Chat RusDataset from 10 thousand dialogs will help researchers of dialogue systems to work out approaches to learning chat bots. We prepared it together with
iPavlov , a project of the laboratory of neural systems and deep learning at MIPT, which conducts research in the field of conversational artificial intelligence and develops
DeepPavlov , an open library for creating interactive assistants. Dataset Persona Chat Rus contains profiles describing a person’s personality and dialogues between study participants.
How the data was collectedAt the first stage, with the help of Toloka users, we collected profiles containing information about a person, his hobbies, profession, family and life events, and selected those that are suitable for dialogues.
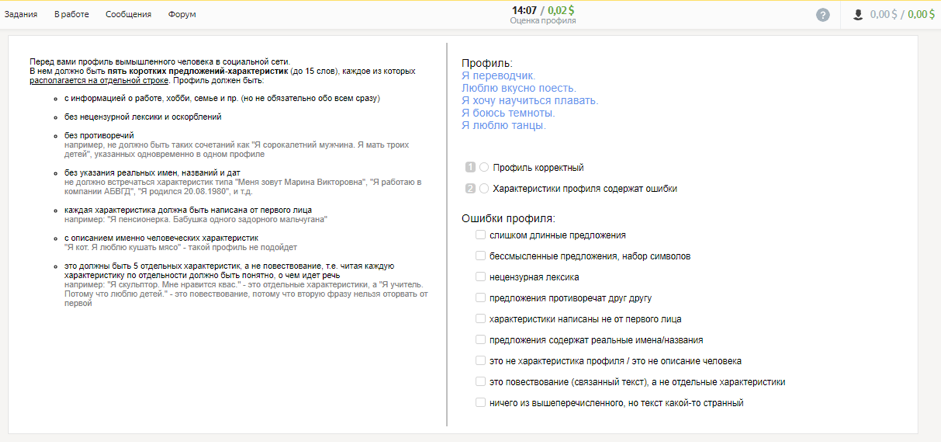
At the second stage, we invited the participants to play the role of a person described by one of these profiles, and communicate with each other in the messenger. The purpose of the dialogue is to learn more about the interlocutor and tell about yourself. The received dialogues were checked by other performers.

Toloka Aggregation Relevance 2Dataset allows you to explore the methods of quality control in crowdsourcing. It contains almost half a million anonymized evaluations of performers collected on the project “Relevance (2 grades)” in 2016. Here you will find both impersonal toloker grades and benchmark grades that will help measure the quality of the responses. The study of these data will allow you to track how the opinion of the performers affects the quality of the final assessment, what methods of aggregation of results are better to use and how many opinions need to be collected in order to get a reliable answer.
How the data was collectedThe contractor was offered a request and the region of the user who set it, a screenshot of the document and a link to it, the ability to use search engines and answer options: “Relevant”, “Irrelevant”, “Not displayed”.
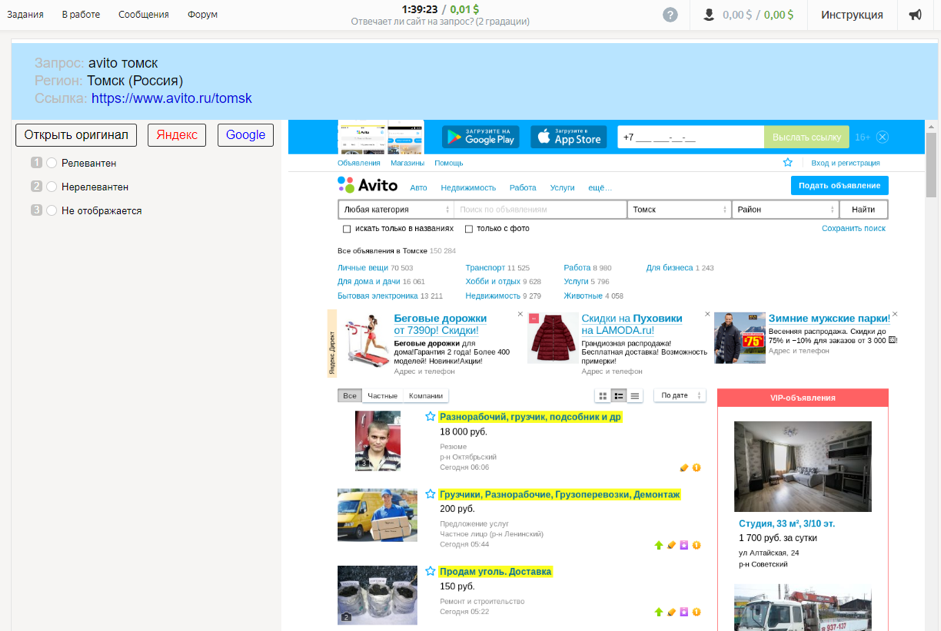
Toloka Aggregation Relevance 5This dataset is the same as the previous one, only estimates were collected here not on a binary, but on a five-point scale in the project “Relevance (5 gradations)”. Dataset contains over a million ratings.
How the data was collectedEvaluation of documents in five grades is more complicated and requires more qualifications. The contractor was offered a request and the region of the user who asked it, a screenshot of the document and a link to it, buttons for using search engines and five response options: “Vital”, “Useful”, “Relevant +”, “Relevant -”, “Irrelevant”.
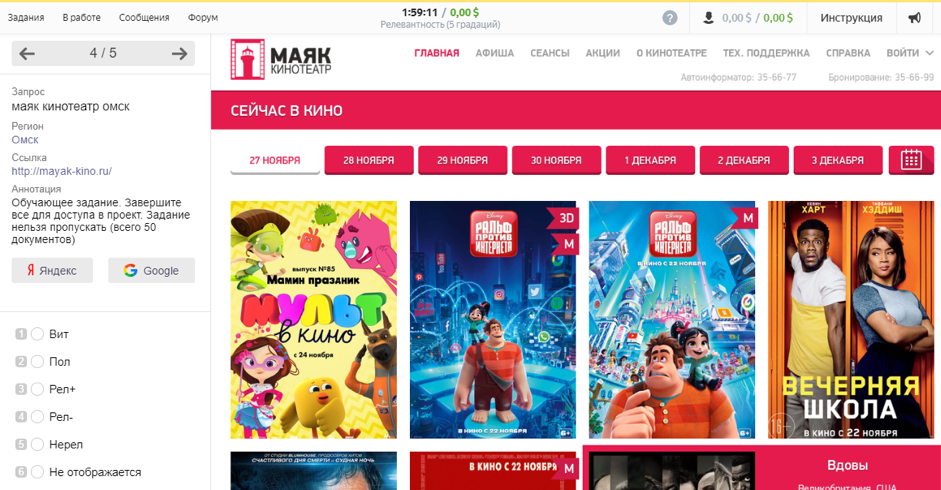
The main quality indicator is the accuracy of aggregated responses, assessed on the basis of control tasks (golden seals). Some tasks in dataset have not one, but several correct answers. Any of these answers is considered correct. Accuracy by main aggregation methods:
â—Ź The majority opinion is 89.92%.
â—Ź Dawid-Skene - 90.72%.
â—Ź GLAD - 90.16%.
Lexical Relations from the Crowd (LRWC)Dataset contains the views of the speakers of the Russian language on the generic-specific relations between the words: the connection between the general (hyperonym) and the particular (hyponym) Collected by the researcher Dmitry Ustalov in 2017.
How the data was collected300 nouns used in modern Russian are taken for research. With the help of thesauruses (RuTez, RuWordNet) and automated methods for the formation of hyperonyms (Watset, Hyperstar) 10,600 species-specific pairs (such as “kitten” - “mammal”) were obtained. The participants in the study needed to answer the question: “Is it true that the kitten is a type of mammal?” To correctly formulate the question, the hyperonyms were put into the genitive case using the morphological analyzer and the pymorphy2 generator.
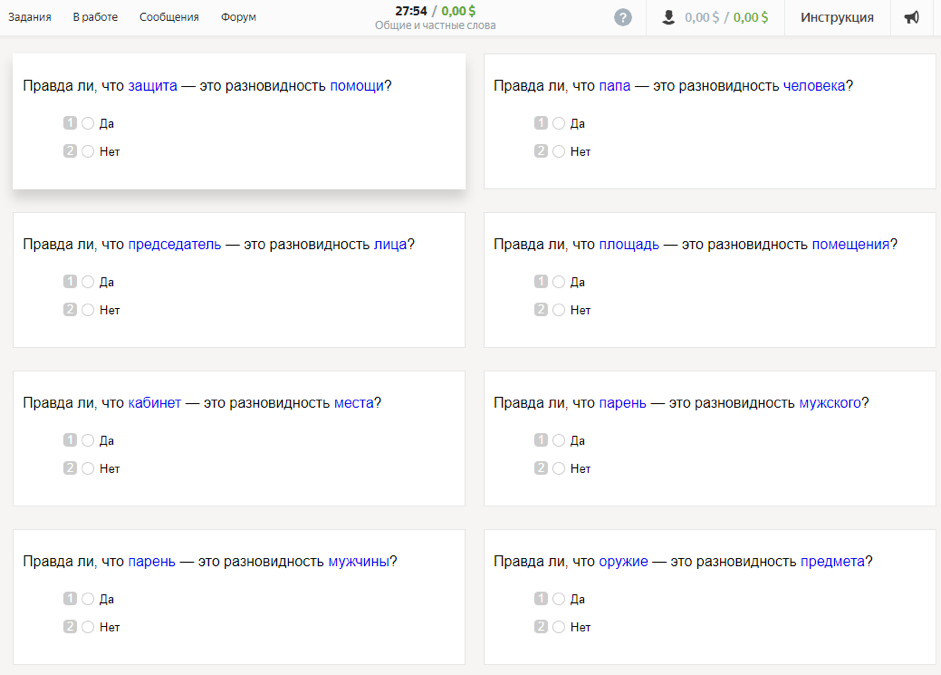
Seven Russian-speaking performers over 20 years old were marked out by each pair. According to the results obtained after the aggregation of all assessments, 4576 pairs of words received positive answers, and 6024 - negative. Interestingly, the research participants were more unanimous in choosing a negative response than a positive one.
Human-Annotated Sense-Disambiguated Word Contexts for RussianIn dataset, 2562 contextual meanings of 20 words are collected, representing the greatest variety of semantic values. The study was conducted by Dmitry Ustalov in 2017.
How the data was collectedThe study participants were shown the word and an example of its use in speech. It was necessary to determine the meaning of the word in the context of the utterance and choose one of the answers.

Toloka Business ID RecognitionFor this dataset, we prepared 10 thousand photographs of information plates of organizations and a text file with numbers (TIN and OGRN), which were indicated on the tablet. Having trained on this data, the computer vision model will be able to recognize a sequence of numbers in an image. Dataset is provided by the Yandex.Spravochnik service.
How the data was collectedFirst, we launched the task in the Toloki mobile app: the performers were asked to come to the address marked on the map, find an organization and take a picture of its information board. This and other field assignments help to maintain up-to-date information in the Yandex.References.
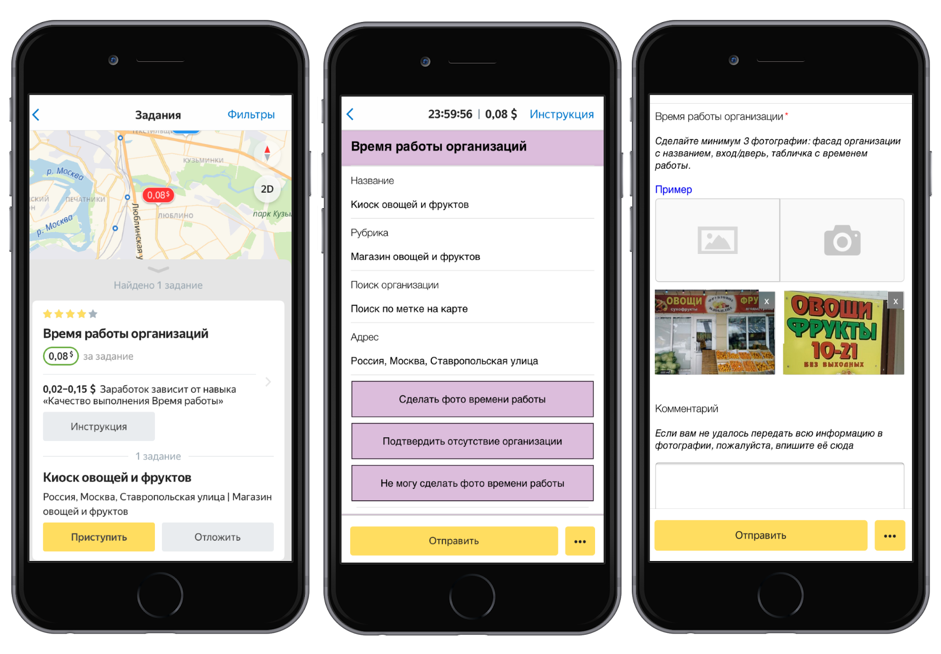
Then the quality of the assignments checked by other performers. The photos, which indicate the INN and OGRN, we sent to decrypt. The tolokers reprinted these numbers from photographs, after which we processed the results and formed a dataset.
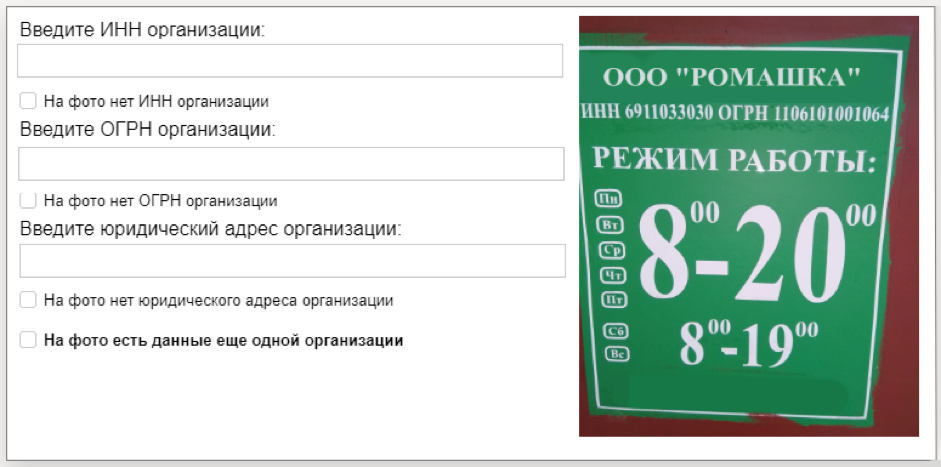
Toloka Aggregation FeaturesDataset contains about 60 thousand estimates in 1 thousand tasks with the correct answers for almost all tasks. Artists classified sites in five categories according to the availability of content for adults. In addition to each task, 52 valid-valued indicators are attached that can be used to predict the category.
You can select and download datasets via the link:
https://toloka.yandex.ru/datasets/ . We do not plan to dwell on this and encourage researchers to pay attention to crowdsourcing and talk about their projects.