Growth Hacking is fanned by many myths. Some consider it a panacea for all ills, others - almost as charlatanry. Distrust is fueled by cases with incredible figures of tens and hundreds of times that are trying to copy mindlessly and without receiving the same growth declare the approach inoperative.
But in order to “crack the growth” of a company, it is not enough just to single out the team and assign them the task of looking for growth points. Growth Hacking is a very complex process that requires high expertise and a clear methodology.
Since the founding of Retail Rocket, growth has become an integral part of the company's work. For over six years, we have developed a unique system for testing and selecting algorithms. Including thanks to this methodology, we can provide the highest ROI on the market. And today we want to share the experience of using Growth Hacking in ecommerce.
')
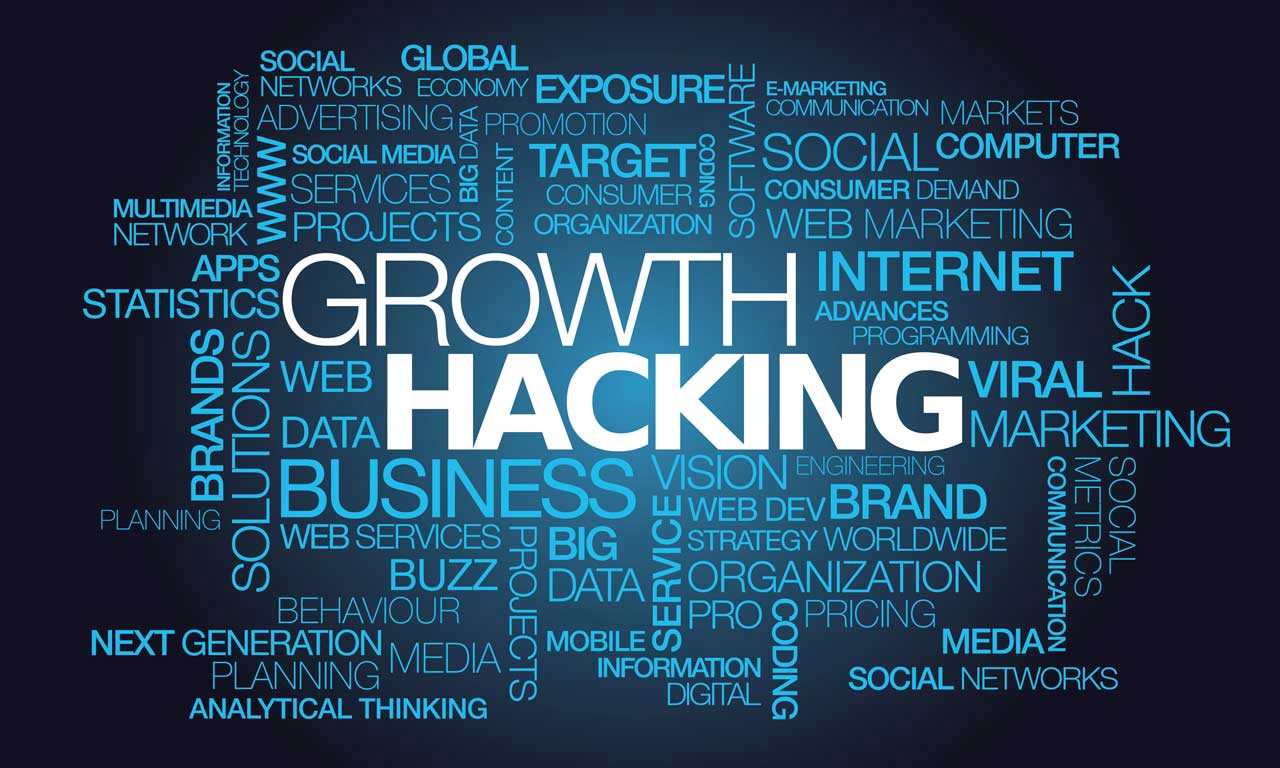
What is Growth Hacking and why any business needs it
Growth Hacking is the continuous work of an individual team on the formulation, organization and analysis of experiments that allow for a high rate of growth in business performance. This means that a business creates a separate department that generates and tests hypotheses that should affect conversion, revenue, profit, and other metrics.
The emergence and active use of Growth Hacking is associated with a large-scale rise in the culture of start-ups, when there is a need for an understandable and accessible tool for testing hypotheses that provide companies with multiple growth.
At the heart of Growth Hacking'a there are three principles:
- Rapid Metric Rise
- Permanent optimization of results
- Open process
If you depict the process schematically, then everything looks quite simple: you need to generate a hypothesis that presumably increases one or more metrics, test it, for example, using A / B testing, and analyze the result. Successful hypotheses can be scaled and optimized, and in the event of failure, the cycle repeats.

In practice, everything is much more complicated. From the choice of hypothesis to the process of testing and evaluating the results - a great deal of expertise and experience is needed at each stage. In part, we talked about this in the article
“Pitfalls of A / B testing or why 99% of your split tests are conducted incorrectly?” .
We have two Growth Hacker teams in Retail Rocket. One deals with the growth of metrics on the site, while the other is responsible for improving the effectiveness of trigger letters. The approaches of the teams to the processes of growth hacking in some moments are similar, and somewhere they differ, about which we will describe in more detail in the course of the article.
Let's start with the hypothesis selection process.
How to find and select a hypothesis to test
Globally, there are 2 approaches to Growth Hacking:
- Random generation of ideas and their verification
- System testing of hypotheses collected in advance
The first method is more expensive and resource intensive, there are more chances of failure, but sometimes strong and breakthrough ideas are born.
The second method works fine if there are repeatability of experiments and you can assemble a whole pool of hypotheses. It gives more predictable results, since there are already collected statistics on the results of previous tests.
For Retail Rocket clients, we use a combination of these methods. First we test the solutions that are most likely to work on this store.
We conducted more than 2000 tests on sites and over 5000 tests in trigger letters, stepped on all possible rakes during these tests, took into account the experience of not only Russian but also foreign stores, so we can with great confidence recommend each online store that option. which will be effective for him. Using system testing of hypotheses, we increase expectations and reduce the likelihood of error.
Many of our hypotheses, we not only describe in cases and show the results of their implementation, but also explain why we are testing just such a solution. Some hypotheses are based on psychological postulates and research, others - destroy common stereotypes.
After a system check of hypotheses recommended by the store, the stage of more experimental and risky work begins. Growth Hacking specialists are looking for new solutions that will bring the store an increase in conversion and an average bill. For trigger letters, new hypotheses, as a rule, are created during the brainstorm process, and each hypothesis must be justified from the point of view of psychology. The team working with sites prefers a more practical approach based on the study of research.
Some hypotheses are offered by our clients. We evaluate the feasibility of testing them, build approximate calculations, how much it can work, and if we understand that the result is worth the effort, we carry out a test. Certainly, clients know their audience better, therefore, in combination with our experience, some hypotheses give excellent results. The most successful ideas even become part of our hypothesis pool. For example, the triggering scenario “Notice of a decrease in the price of goods in a basket” after a series of successful tests took a worthy place in our map of triggers.
Thus, thanks to the combination of the expertise of the marketers of the store with the experience of Retail Rocket specialists, a WIN-WIN system is obtained, in which both parties benefit.
How to test a hypothesis, or why we chose A / B tests
The AB-testing process is excellent for testing hypotheses in online space. He has a clear methodology and he gives transparent results, of course, if you carry out a split test correctly.
We have a clear methodology for the test, which includes several stages of testing and eliminates possible errors. There is a tool for dividing traffic. There is expertise and experience, thanks to which, when questions arise, we know where to look and where to look for problems.
A / B test can be divided into several stages:
- Run the test. There may be a lot of potential errors. Included in the list of typical ones are incorrect layout and segmentation errors. Our methodology is based on the cross-checking of tests and careful coordination of all the details with the retailer, which minimizes possible errors.
- Stop the test. How long should the test go so that the results can be interpreted unambiguously? How to understand where the novelty effect turns into a working method to increase conversion?
- Evaluation of the results. Starting from data collection and cleaning techniques to conclusions that any analyst can test, in the test reports we give the most complete and transparent information about what effect a particular solution gives.
Thus, following the results of testing, the store clearly understands which hypothesis will bring the store an increase in conversion and sales, and which to introduce does not make sense.
How it looks in practice
Let us tell by the example of a pair of case studies how Growth Hacking works in Retail Rocket.
Case 1. Using the principle of social proof in trigger letters
As we mentioned above, in the trigger scenarios hypotheses are often based on various psychology postulates. For example, a good result in most cases shows the application of the principle of social proof. Its essence is that people trust the opinions of other people when choosing a product. Social proof can be used in various ways, for example, reviews of products, ratings, information about how many users view a product at the same time, etc.
We decided to test the hypothesis of increasing demand for the product through the introduction of the “Purchased Today” block, which demonstrated the amount of goods purchased by other users.
The work was carried out by means of A / B testing, in which all the recipients of letters are randomly divided into two segments. Segment A is sent the original version of the letter, segment B - a letter with a change-hypothesis, which should increase the effectiveness of mailings.
Hypothesis testing was carried out in the trigger script “Letter with related goods after ordering” of the Mamsy sales club:

According to the results of testing, the conversion into orders of a letter with an embedded hypothesis
was 60.5% higher than that of the standard version (the statistical reliability of the result was 96.8%).
Case 2. Choosing the most effective option to display recommendations on the search page
Now let's move to the site and look at the hypothesis test on the example of the online pharmacy Zdravsiti.
The main focus of testing on the websites of online stores we do is to check the effectiveness of various algorithms in order to understand which mechanics of recommendations will bring the retailer maximum results. But we also check how the various design elements will affect the conversion, average check and revenue figures. This may be the introduction of a slider in the recommendations, the addition of labels with discounts or other design solutions.
In this case, the hypothesis was that if you add the CTA element to the goods in the recommendation blocks, it will be easier for the user to add the product to the basket and due to this the conversion and the average bill will increase.
Efficiency studies were conducted using A / B testing mechanics. All site visitors were randomly divided into 3 segments:
- The first segment showed a block of recommendations without a CTA-element (basic appearance)
- The second segment showed a block of recommendations with the addition of the CTA element “To cart” (adds goods to the basket)
- Recommendations were not shown to the third segment (control group)

According to the test results, the
introduction of the hypothesis “Recommendation block with the addition of the CTA-button“ To the basket ”on the search page of the online store Zdravcity.ru increases conversion by 1.05% (statistical significance 99.5%). In combination with an average check increase of 7.3%, which gives a projected increase in revenue of 8.4%.
These are just a couple of examples of how you can generate and test various hypotheses for the growth of metrics. We regularly talk about it in the cases, so if you want to learn more interesting hypotheses and the results of their verification, go to our
blog .
And remember, the more experiments you do, the higher your result will be in the long run. Therefore, conduct A / B tests, test hypotheses, and find those solutions that will bring growth to you.