The history of AI is often told as the history of cars, gradually becoming smarter. But in the story the human factor is lost, the question of designing and training machines, and how they appear, thanks to the efforts of man, mental and physical.
Let's explore this, the human history of AI, with how innovators, thinkers, workers, and sometimes speculators created algorithms that can reproduce human thought and behavior (or pretend to reproduce them). The idea of ​​supramental computers that do not require human participation can be exciting - but the true history of smart machines shows that our AI is as good as we are good.
When Charles Babbage played chess with the first mechanical Turk
The famous engineer of the XIX century, perhaps inspired by the first example of the hype around AI
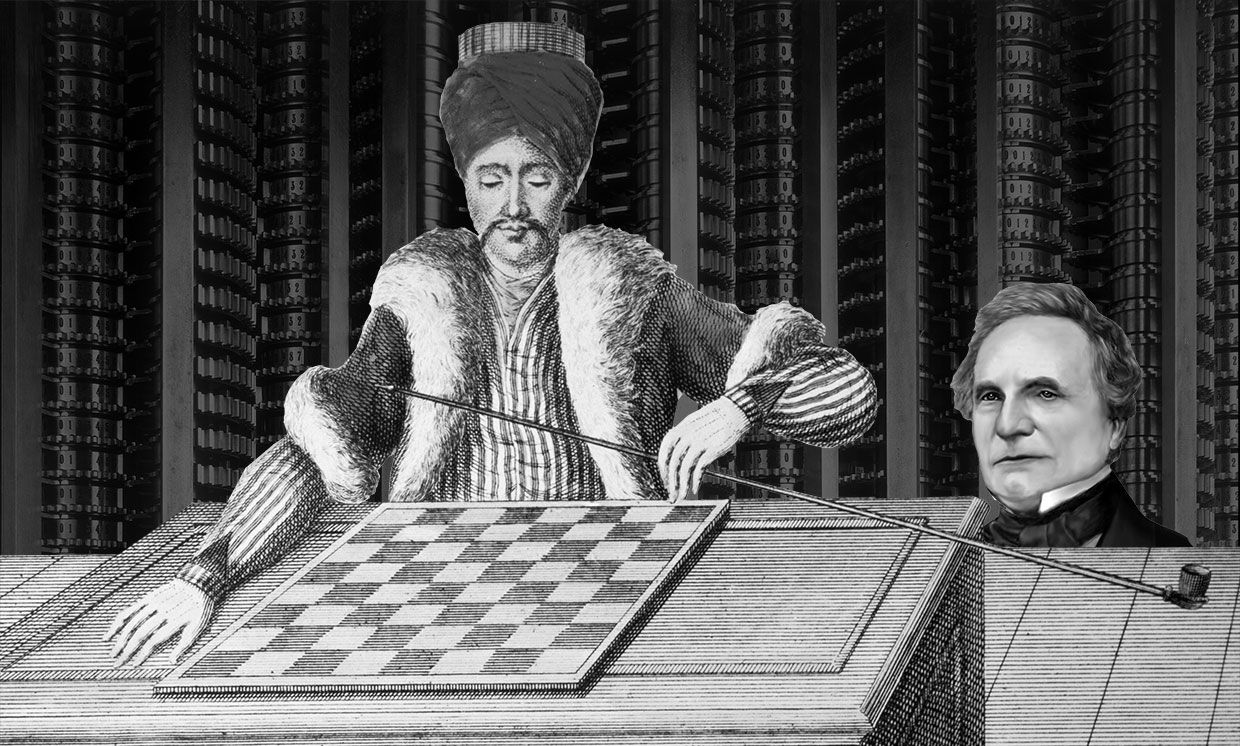
In 1770, at the court of the Austrian Empress
Maria Theresa , the inventor
Wolfgang von Kempelen demonstrated a
machine that plays chess . “The Turk”, as Kempelen called his invention, was a life-size human figure cut from cedar [according to other sources - wax / approx. transl.], dressed as a representative of the Ottoman Empire, sitting at a wooden cabinet, on the table top of which was a chessboard.
Kempelen declared that his car was capable of defeating any court, and this challenge was accepted by one of Maria Theresa’s advisers. Kempelen opened the cabinet doors, showing a mechanism similar to a clockwork — a complex network of levers and gears, and then he inserted a key into the car and started it. The machine gun came to life, and raised a wooden hand to move the first figure. Within 30 minutes he defeated his rival.
')
Turk made a splash. In the next ten years, Kempelen performed with his chess machine in Europe, defeating many of the smartest people of the time, including Benjamin Franklin and Frederick II. After the death of Kempelen in 1804, Turk was acquired by
Johann Nepomuk Melzel , a student at a German university and designer of musical instruments, who continued to perform throughout the world.
One of those who were allowed to see the car in more detail was
Charles Babbage , the famous British engineer and mathematician. In 1819, Babbage played with Turk twice and lost both times. According to historian Tom Standedzh, who wrote a detailed history of the Turk, Babbage suspected that the machine was not a smart machine, but merely a cunning hoax, and that there was a person in it who controlled the movements of the Turk from the inside.

Babbage was right. The following was hidden behind the Turk's screen: Kempelen and Melzel hired grandmasters to sit covertly inside a large box. The grandmaster could see what was happening on the board thanks to the magnets, which gave a mirror image of the spaced pieces.
To control Turk's hand, the hidden player used a pantograph - a system of blocks that synchronized the movements of his hand with a wooden Turk. The player moved the lever on the magnetic board, rotated it to unlock and close Turk's fingers, and then move the piece to the right place. The room where the grandmaster was sitting contained several sliding panels and a chair on wheels, moving on blurred rails, which allowed him to move forward and backward when Melzel opened the box for everyone to see.
And although Babbage suspected of such tricks, he did not waste time exposing, as many of his contemporaries. However, his meeting with Turk seems to have determined his thinking for many years.
Charles Babbage designed differential machine number 2 from 1847 to 1849, but during his lifetime it was not built.Shortly thereafter, he began work on an automatic mechanical calculator called "
differential machine ", which he intended to use to create error-free logarithmic tables. The first project of the machine, which could weigh 4 tons, contained 25,000 metal components. In the 1830s, he abandoned it, starting work on an even more complex mechanism, the "analytical machine." She had a “repository” and a “mill” that worked like a memory and a processor, as well as the ability to interpret the software instructions contained on punch cards.
Initially, Babbage hoped that the analytical machine would work simply as an improved differential version. But his colleague,
Ada Lovelace , realized that the programmability of the machine allows it to operate in a more generalized mode. She said that such a machine would generate a new type of “poetic science,” and mathematicians would train the machine to perform tasks by programming it. She even predicted that the machine would be able to compose "complex scientific musical works."
Ada Lovelace and Charles BabbageBabbage finally agreed with Lovelace, and presented how the potential of a general-purpose machine capable of not only grinding numbers can change the world. Naturally, his thoughts returned to the meeting with Turk. In 1864, he wrote in his diary about his desire to use "mechanical recording" to solve completely new problems. “After serious reflection, for my test, I chose a clever machine capable of successfully playing an intellectual game such as chess.”
Although technically Turk and the Babbage machine are in no way connected, the possibility of machine intelligence, embodied in the mystification of Kempelen's background, seems to have inspired Babbage to think about cars in a completely new light. As his colleague, David Brewster, later wrote: “These automatic toys, once entertaining commoners, are now involved in increasing the capabilities and development of the civilization of our species.”
The meeting of Babbage with the Turk at the very beginning of the computational history serves as a reminder that hype and innovation sometimes go hand in hand. But she teaches us one more thing: the intellect attributed to machines is almost always based on hidden human accomplishments.
Invisible female computer programmers ENIAC
The computing people who managed ENIAC received almost no recognition
Marilyn Veskov (left) and Ruth Lichterman were two ENIAC female programmersOn February 14, 1946, journalists gathered at Moore’s School of Engineering at the University of Pennsylvania to watch an open demonstration of one of the first general-purpose electronic digital computers in the world: ENIAC (electronic numerical integrator and computer).
Arthur Burks, a mathematician and chief engineer of the ENIAC team, was in charge of demonstrating the capabilities of the machine. At first he instructed the computer to add 5000 numbers, which was done in 1 second. Then he demonstrated how the machine can calculate the trajectory of the projectile faster than the projectile itself would need to fly from gun to target.
Reporters were amazed. It seemed to them that the Burks needed only to press a button, and the car would come to life in order to calculate in a few moments what people had previously spent on days.
What they did not know, or what was hidden during the demonstration, is that behind the seeming intelligence of the machine there was a hard and advanced work of a team of programmers consisting of six women who used to work as “computers”.
Betty Jennings (left) and Francis Bilas working with the main control panel ENIACThe plan for building a machine capable of counting the trajectory of projectiles was born in the early years of World War II. Moore’s School of Engineering worked with a ballistic research laboratory (BRL), where a team of 100 trained “calculators-people” manually counted artillery firing tables.
The task required a good level of knowledge in mathematics, including the ability to solve nonlinear differential equations,
differential analyzers and
slide rules . But at the same time, calculations were considered to be clerical work, a task too tedious for male engineers to do. Therefore, BRL hired women - mostly university graduates and maths - for this job.
Over the course of the war, the ability to predict the projectile flight path was increasingly associated with military strategy, and the BRL increasingly demanded results.
In 1942, physicist
John Mouchli wrote a
service note with a proposal to create a general-purpose programmable electronic calculator capable of automating calculations. By June 1943, Mouchley, together with engineer J. Presper Eckert, received funding for the construction of ENIAC.
J. Presper Eckert, John Mouchley, Betty Jean Jennings and Herman Goldstein to ENIACThe purpose of the electronic computer was the replacement of hundreds of people-calculators from BRL, as well as increasing the speed and efficiency of calculations. However, Mouchley and Eckert realized that their new machine would need to be programmed to calculate trajectories using punched cards, using technology that IBM had been using for their machines for several decades.
Adele and Hermann Goldstein, a couple who led the work of the calculator people at BRL, suggested that the strongest mathematicians from their team should do this work. They chose six - Kathleen McNulty, Francis Bilas, Betty Jean Jennings, Ruth Lichterman, Elizabeth Schneider and Marilyn Veskov - and promoted them from human calculators to operators.
Elizabeth "Betty" Schneider works for ENIACTheir first task was to thoroughly get acquainted with ENIAC. They studied the machine drawings to understand its electronic circuits, logic, and physical structure. There was something to learn: the 30-ton monster took about 140 square meters. m., used more than 17,000 vacuum tubes, 70,000 resistors, 10,000 capacitors, 1,500 relays and 6,000 manual switches. A team of six operators was responsible for setting up and installing the machine to perform certain calculations, work with equipment serving punch cards, and search for errors in the work. To do this, operators sometimes had to climb inside the car and replace a failed electronic lamp or wiring.
ENIAC did not have time to finish in time to calculate the flight of projectiles during the war. But soon her power involved
John von Neumann to calculate nuclear fusion. This required the use of more than a million punch cards. Los Alamos physicists relied entirely on operator programming skills, since only they knew how to handle such a large number of operations.
ENIAC Programmer Kathleen McNultyHowever, the contribution of female programmers received very little recognition or appreciation. In particular, because the programming of the machine is still closely associated with manual calculations, and therefore was considered not entirely professional work, suitable only for women. Leading engineers and physicists focused on the development and creation of iron, which they considered more important for the future of computers.
Therefore, when ENIAC was finally presented to the press in 1946, six female operators remained hidden from the public eye. The dawn of the Cold War came, and the US military eagerly demonstrated their technological superiority. Representing ENIAC as an autonomous smart machine, engineers painted an image of technological superiority, hiding the human labor used.
The tactic worked, and influenced the media coverage of computers in the following decades. On the ENIAC news spread all over the world, the car took the main attention and received such epithets as “electronic brain”, “wizard” and “human-made robot brain”.
The heavy and painstaking work of six female operators crawling inside the car, replacing the wiring and lamps so that the car could perform its “reasonable” actions, was covered very little.
Why Alan Turing wanted AI to make mistakes
Infallibility and intelligence are not the same thing.

In 1950, at the dawn of the digital age,
Alan Turing published an article, which was later destined to become the most famous of his works, “
Computers and Mind ”, in which he
asked : “Can machines think?”
Instead of trying to define the concept of "machine" and "thinking", Turing describes a different method of finding the answer to this question, inspired by the salon game of the Victorian era - the game of imitation. According to the rules of the game, a man and a woman who are in different rooms talk to each other, passing the notes through an intermediary. The mediator, who also plays the role of judge, needs to guess which of them is a man and who is a woman, and his task is complicated by the fact that a man is trying to imitate a woman.
Inspired by this game, Turing developed a thought experiment in which one of the participants was replaced by a computer. If the computer can be programmed to play imitation so well that the judge could not tell the difference, says about the machine or the person, then it would be reasonable to conclude, Turing asserted that the machine has intelligence.
This thought experiment became known as the
Turing test , and to this day remains one of the most famous and controversial ideas in AI. It does not lose its attractiveness, since it gives an unequivocal answer to the very philosophical question: “Can the machines think?” If the computer passes the Turing test, then the answer is “yes”. As the philosopher
Daniel Dennett wrote , the Turing test should have stopped the philosophical debate. “Instead of arguing endlessly about the nature and essence of thinking,” Dennett writes, “why don’t we agree that whatever this nature is, everything that this test can pass does have it”.
However, a more careful reading of Turing's work reveals a small detail that introduces a slight ambiguity in the test, suggesting that perhaps Turing had in mind not a practical test of the machine for mind, but a philosophical provocation.
In one of the pieces of work, Turing gives a simulation of how a test could look using an imaginary intelligent computer of the future. The person asks questions, and the computer answers.
Q: Please write a sonnet about the bridge over the Fort.
A: Then I have to refuse. I never did poems.
Q: Fold 34957 and 70764.
A: (answer after a 30-second pause): 105621.
Q: Do you play chess?
Oh yeah.
Q: My king is on e1; I have no other figures. Your king is at e3, and the rook is at a8. Your turn. How will you go?
A: (After thinking for about fifteen seconds): La1, mat.
In this conversation, the computer made an arithmetic error. The real sum of the numbers will be 105721, not 105621. It is unlikely that Turing, a brilliant mathematician, accidentally allowed it. Rather, it is Easter eggs for the attentive reader.
Elsewhere in the Turing article, it seems to hint that this error is a programmer’s trick designed to deceive the judge. Turing understood that if attentive readers of computer responses see an error, they will decide that they are talking to a person, assuming that the machine will not allow such an error. Turing wrote that the machine can be programmed to "deliberately include errors in the answers, designed to confuse the interrogator."
And if the idea of ​​using errors to hint at the human mind was difficult to comprehend in the 1950s, today it has become a design practice for programmers working with the processing of natural languages. For example, in June 2014, the chatbot
Zhenya Gustman became the first computer to pass the Turing test. However, critics pointed out that his wife managed to do this only thanks to a built-in stunt: he simulated a 13-year-old boy, whose English was not his first language. This meant that his mistakes in syntax and grammar, as well as the incompleteness of knowledge, were mistakenly attributed to naivety and immaturity, instead of the inability to process natural languages.
Similarly, after Google’s
Duplex voice assistant
hit the public with its pauses in the conversation and using the sounds that fill them, many indicated that this behavior was not the result of the system’s thinking, but a specially programmed action designed to simulate the human thinking process.
Both cases implement the idea of ​​Turing that computers can be specifically made to make mistakes in order to make an impression of a person. Like Turing, programmers Eugene Gustman and Duplex understood that a superficial imitation of human imperfection could deceive us.
Perhaps the Turing test evaluates not the presence of a machine’s mind, but our readiness to consider it reasonable. As Turing himself
said : “The idea of ​​the mind itself is more emotional than the mathematical one. How reasonable we consider the behavior of something is determined no less by our own state of mind and skills than by the properties of the object in question. ”
And, perhaps, the mind - this is not some kind of substance that can be programmed by the machine, - which, apparently, meant Turing, - but a feature that manifests itself through social interaction.
DARPA Dreamer Targeting Cybernetic Intelligence
Joseph Carl Robnett Liklider made proposals to create a "symbiosis of man and machine," which led to the invention of the Internet

At 10:30 am on October 29, 1969, a graduate student at the University of California at Los Angeles sent a two-letter
message from the SDS Sigma 7 computer to another machine, located a few hundred kilometers away, at the Stanford Research Institute in Menlo Park.
The message read: “LO.”
The graduate student wanted to write a “LOGIN”, but the packet exchange network that supported the transfer of the message, ARPANET, fell down before it was possible to write the entire message.
In the history of the Internet, this moment is marked as the beginning of a new era of online communications. But it is often forgotten that the ARPANET technical infrastructure was based on a radical idea about the future symbiosis of man and computer, developed by a man named
Joseph Karl Robnett Liklider .
Liklider, who had a psychological education,
became interested in computers in the late 1950s , working in a small consulting company. He was interested in how these machines could enhance the joint intelligence of mankind, and he began to conduct research in the actively developing field of AI. After examining the literature that existed then, he found that programmers intend to “teach” machines to perform certain actions for a person, such as playing chess or translating texts, and with greater efficiency and quality than people.
This concept of machine intelligence did not suit Liclayder. From his point of view, the problem was that the existing paradigm considered people and machines to be intellectually equivalent creatures. Liklider also believed that in fact people and machines are fundamentally different in their cognitive abilities and strengths. People do well with certain sensible tasks — such as creativity or judgment — and computers with others, such as memorizing data and processing it quickly.
Instead of forcing computers to imitate people's intellectual activity, Liklider suggested that people and machines work together in which each side uses its strengths. He suggested that such a strategy would move from competitions (such as the game of people playing chess with computers) to previously unthinkable forms of intellectual activity.
In the work of 1960, "The
symbiosis of the machine and man, " Liklider described this his own idea. “I hope that soon enough, the human brain and computers will be closely connected with each other, and that the resulting partnership will be able to reflect in ways that no brain could, and process data in the way their modern machines did not.” For Liclider, a promising example of such a symbiosis was a system of computers, network equipment, and human operators, known as the “semi-automatic ground environment,” or SAGE, which opened two years earlier to track US airspace.
In 1963, Liklider got a job as a director of advanced research projects (then called ARPA, and now DARPA), where he had the opportunity to implement some of his ideas. In particular, he was interested in developing and implementing what he first called the "
intergalactic computer network ."
The idea came to him when he realized that ARPA needed to invent an effective method of updating data related to programming languages ​​and technical protocols, available to teams scattered far from each other, consisting of people and machines. The solution to this problem was a communications network uniting these teams over long distances. The problems of networking them were similar to the problems that the science fiction writers pondered, as he pointed out in the
memorandum describing this concept: “How to begin communication between intelligent beings completely unrelated to each other?”
Liclider, MIT professor, and his student, Jeff HarrisLiclider left ARPA before the development program for this network was launched. But over the next five years, his lofty ideas became an integral part of the development of ARPANET. And as ARPANET evolved into what we know today as the Internet, some began to see that this new method of communication is a joint work of human and technological entities, a symbiote, which sometimes behaves, as Belgian cybernetics
Francis Hailien said like the global brain.
Today, many significant breakthroughs in machine learning are based on the collaboration of people and machines. For example, the trucking industry is increasingly looking for ways to enable human drivers and computing systems to use their strengths to improve delivery efficiency. Also in the field of transportation, Uber has developed a system in which people are given tasks that require good driving skills, such as entry and exit from the highway, and cars remain hours of routine driving on the highway.
Although there are many other examples of the symbiosis of man and machine, the cultural tendency to imagine machine intelligence in the form of a certain separate supercomputer with the human-level mind is still quite strong. But in fact, the future of cyborgs, which Liklider imagined, has already arrived: we live in a world of symbiosis of machines and people, which he described as “living together in close connection, or even in the union of two different organisms”. Instead of focusing on the fear of people replacing cars, Liclayder’s legacy reminds us of the possibilities of working with them.
Algorithmic bias appeared in the 1980s
In medical school, they thought that a computer program would make the process of making students more honest, but it turned out to be the opposite.

In the 1970s, Dr. Joffrey Franglen of the
Medical School of St.. George in London began to write an algorithm for the elimination of applications for applicants for admission.
At that time, three-quarters of the 2,500 people who submitted their application annually were eliminated by special people who evaluated their written application, as a result of which applicants did not reach the interview stage. Approximately 70% of people who went through primary screenings enrolled in medical school. Therefore, the initial dropout was an extremely important stage.
Franglen was deputy dean and also dealt with screening applications.
Reading the applications required a break of time, and it seemed to him that this process could be automated. He studied the dropout technique of students, which he and other certifiers used, and then he wrote a program that, as he said, “imitated the behavior of certification people”.Franglen's main motivation was to increase the efficiency of the adoption process, and he also hoped that his algorithm would eliminate the inconstant quality of the staff’s work. He hoped that by transferring this process to the technical system, it would be possible to achieve an absolutely identical assessment of all applicants, and create an honest screening process.In fact, everything turned out the opposite.Franglen completed his algorithm in 1979. That year, applicants' applications were checked in parallel by a computer and people. Franglen discovered that his system agreed with the assessors in 90-95% of cases. The administration decided that these figures allow replacing officials with an algorithm. By 1982, all primary enrollment applications began to be evaluated by the program.After a few years, some staff members became concerned about the lack of diversity among enrolled students. They conducted an internal investigation of the Franglen program and found certain rules that evaluated applicants for seemingly irrelevant factors, such as place of birth or name. However, Franglen convinced the committee that these rules were collected on the basis of data collected on the work of the assessors and did not greatly influence the sample.In December 1986, two school lecturers learned about this internal investigation and went to the British Commission for Racial Equality. They told the commission that they had reason to believe that the computer program was used for covert discrimination against women and color ones.The commission launched an investigation. It was found that the algorithm separated the candidates for Caucasians and non-Europoids, based on their names and places of birth. If their names were not European, it went to them in the negative. The mere presence of a non-European name subtracted 15 points from the total amount of the applicant. The commission also found that women on average underestimated by 3 points. Based on this system, up to 60 applications were rejected daily.At that time, racial and sexual discrimination was very strong at British universities — and the St. Georg was caught on this only because she entrusted this bias to a computer program. Since it was provable that the algorithm evaluated women and people with non-European names lower, the commission received solid evidence of discrimination.The medical school was accused of discrimination, but it got off quite easily. Trying to make amends, the college contacted people who could have been wrongfully discriminated, and three of the rejected applicants were offered schooling. The Commission noted that the problem in the medical school was not only technical, but also cultural. Many employees treated the sorting algorithm as the ultimate truth, and didn’t take the time to figure out how it sorts applicants.At a deeper level, it is clear that the algorithm supported the prejudices that already existed in the applicants admission system. Franglen after all checked the car with the help of people and found a match of 90-95%. However, having encoded the discrimination that the certifiers had in the car, he ensured an endless repetition of this bias.The case of discrimination in the school of sv. Georg attracted a lot of attention. As a result, the commission banned the inclusion of information about race and ethnicity in applicants' statements. However, this modest step did not stop the spread of algorithmic bias.Algorithmic decision-making systems are increasingly deploying in areas with a high degree of responsibility, for example, in health care and criminal justice, and repetition, as well as strengthening the existing social bias resulting from historical data, is a serious concern. In 2016, journalists from ProPublica showed that software used in the United States to predict future crimes was biased against African Americans. Later, researcher Joy Bulamwini showed that Amazon's facial recognition software is more mistaken in the case of black women.And although machine bias is rapidly becoming the most discussed topic in AI, algorithms are still considered mysterious and undeniable mathematical objects that produce reasonable and unbiased results. As AI critic Kate Crawford says, it’s time to recognize that algorithms are “creatures of people” and inherit our bias. The cultural myth of the undeniable algorithm often hides this fact: our AI is only as good as we are.How Amazon shoved mechanical turks into a car
Today's invisible digital workers resemble the man who ruled the 18th century Mechanical Turk
At the turn of the millennium, Amazon began to expand its services beyond the sale of books. With the growing number of different product categories on the website, the company had to invent new ways to organize and categorize them. Part of this task was the removal of tens of thousands of duplicate products appearing on the site.Programmers tried to make a program that can automatically eliminate duplicates. Identifying and deleting objects seemed like a simple task accessible to the machine. However, programmers soon surrendered, calling the data processing task " impossible ". For a task involving the ability to notice minor inconsistencies or similarities in images and texts, human intelligence was required.Amazon has a problem. Removing duplicate products was trivial for people, but a huge amount of items would require a lot of manpower. Managing employees engaged in one such task would be non-trivial.Wenky Harinarayan, the company's manager, came up with a solution. His patent describes “hybrid computational cooperation between a person and a machine”, which breaks down a task into small sections, subtasks, and distributes them across a network of human employees.If duplicates are removed, the main computer can split the Amazon site into small sections — say, 100 pages of openers — and send sections to people over the Internet. They then needed to identify duplicates inside these sections and send puzzle pieces back.The distributed system offered a critical advantage: workers did not need to accumulate in one place, they could perform their subtasks on their computers, wherever they were and when they wanted. In fact, Harinarayan designed an effective way of distributing low-skilled, but difficult to automate, work across a wide network of people who can work in parallel.This method proved to be so effective for the company's internal operations that Jeff Bezos decided to sell the system as a service to third-party organizations. Bezos turned Harinaraiyan technology into a market for workers. There, enterprises that had tasks that were easy for people (but difficult for robots) could find themselves a network of freelance workers who perform these tasks for a small fee.So appearedAmazon Mechanical Turk , or mTurk for short . The service started in 2005 , and the user base began to grow rapidly. Enterprises and researchers around the world began to download the so-called. “Tasks for human intelligence” on the platform, such as decoding audio or tagging images. The tasks were performed by an inter-ethnic and anonymous group of workers for a small fee (one upset employee complained that the average remuneration was 20 cents).The name of the new service was sent to the 18th century chess machine, Mechanical Turku, invented by small entrepreneur Wolfgang von Kempelen. And just like in the fake automation in which the person who was playing chess was sitting, the mTurk platform was designed to conceal human labor. Instead of names, platform workers have numbers, and communication between the employer and the worker is personalized. Bezos himself called these dehumanized workers " artificial artificial intelligence ."Today mTurk is a thriving market with hundreds of thousands of workers from all over the world. And although the platform provides a source of income for people who may not have access to other work, working conditions there are very dubious. Some critics arguethat hiding and dividing workers, Amazon eases the task of operating them. In research work from December 2017, it was found that the median employee’s salary is about $ 2 per hour, and only 4% of workers earn more than $ 7.25 per hour.Interestingly, mTurk has become a critical service for developing machine learning. The MO program issues a large data set, where it learns to look for patterns and draw conclusions. MTurk workers are often used to create and mark up these sets, while their role in the development of MOs often remains in the shadows.The dynamic between the AI ​​community and mTurk corresponds to the one that has been present throughout the history of computer intelligence. We eagerly admire the appearance of autonomous "intelligent machines", ignoring or deliberately hiding the human labor that creates them.Perhaps we can learn a lesson from Edgar Allan Poe ’s comments . When he studied the Mechanical Turk von Kempelen, he did not succumb to this illusion. Instead, he wondered what the hidden chess player was to sit inside this box, “wedged” among the gears and levers in a “painful and unnatural posture.”At the moment, when headlines about AI breakthroughs dot the news field, it's important to remember Poe’s sober approach. It can be quite fascinating - even if it is dangerous - to succumb to the hype around AI and overly get involved in the ideas of machines that do not require mere mortals. However, with careful consideration, you can see traces of human labor.