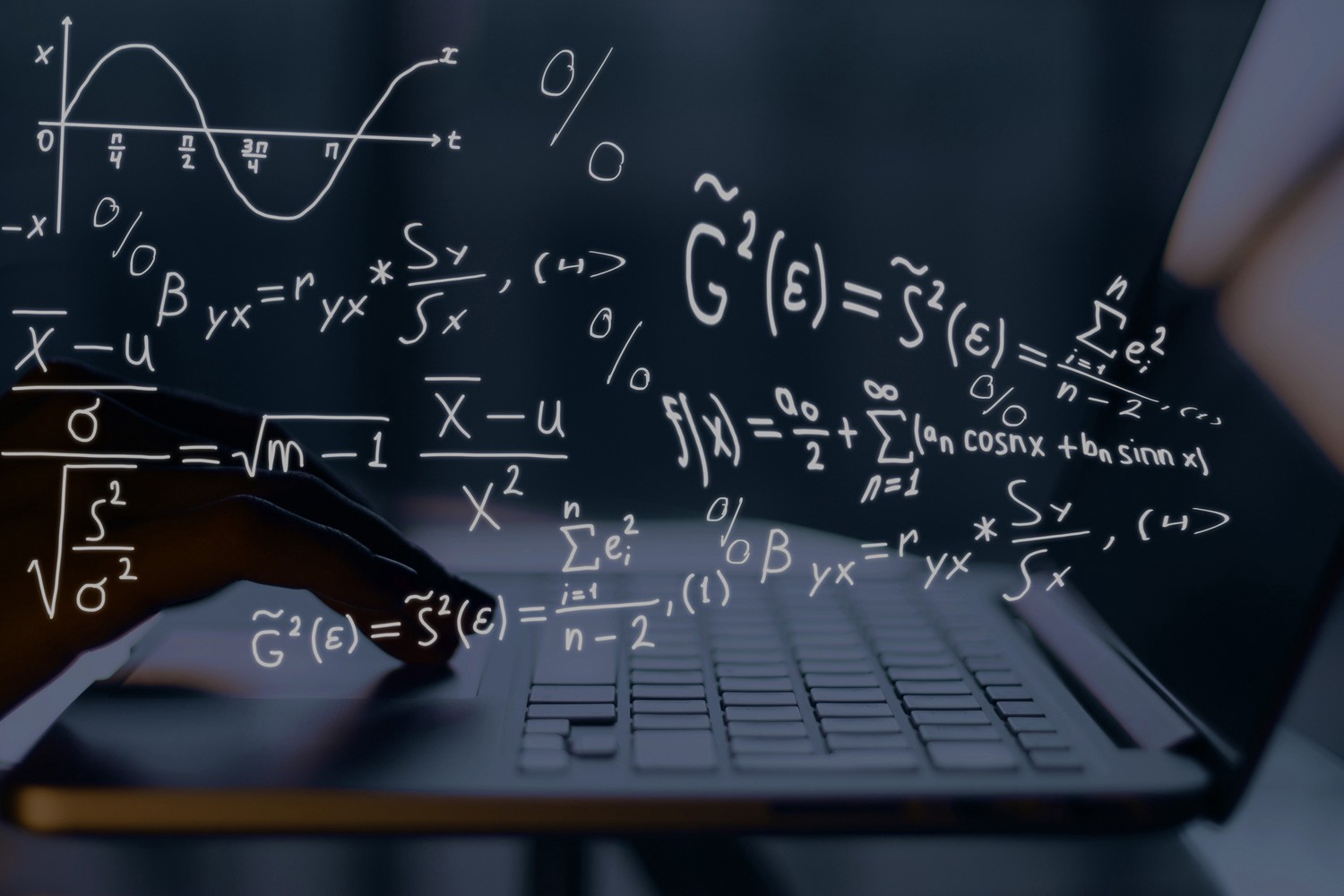
Popular science and even entertainment media in our time are filled with news about the success of AI projects. That artificial intelligence wins a man in go, then learns to play StarCraft and comes out the winner from the fight with recognized champions. And this is only a small fraction of the achievements, in fact there are many more. An ordinary person (in a sense not connected with the IT sphere) may think that real, “big” artificial intelligence is about to appear, about which science fiction writers write and make films.
But all is not so bright. For example, the other day there was information that the AI ​​tried to pass the test in higher mathematics (school test, standard for the UK) and could not do it.
In principle, the causes of failure can be explained without much difficulty. So, a person in solving mathematical problems involves the following abilities and capabilities.
')
Modifies for itself the characters in essence, such as numbers, arithmetic operators, variables (which together form functions) and words (defining the question, the meaning of the problem, etc.).
- Planning (for example, ranking functions in the order required to solve a mathematical problem).
- The use of auxiliary algorithms for the compilation of functions (addition, multiplication).
- Using short-term memory to store intermediate values ​​(for example, h (f (x))).
- Application in practice of previously obtained knowledge of rules, transformations, processes and axioms.
DeepMind was trained and tested on a selection of different types of math problems and tasks. The developers did not use crowdsourcing, instead they synthesized a data set to generate a large number of test problems, control their level of complexity, etc. The development team used the “free form” text data format.
The initial data were based on tasks from assignments for students in UK schools (age under 16). Tasks were taken from areas such as arithmetic, algebra, probability theory, etc.
The DeepMind team, choosing the neural network architecture for solving mathematical problems, stopped at LSTM (
long short-term memory ) and
Transformer (neural network architecture for working with sequences).
DeepMind tested two LSTM models for working with mathematical problems: a simple LSTM and Attentional LSTM, the scheme of which is shown in the figure below.
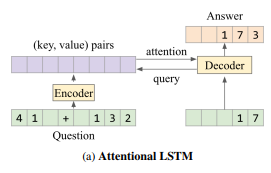
Below is a diagram of the Transformer model
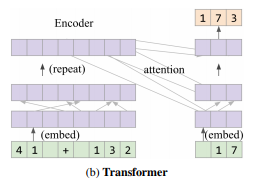
The result was not too good. Only 35% of AI answers were correct, this is an unsatisfactory assessment by the standards of any school.
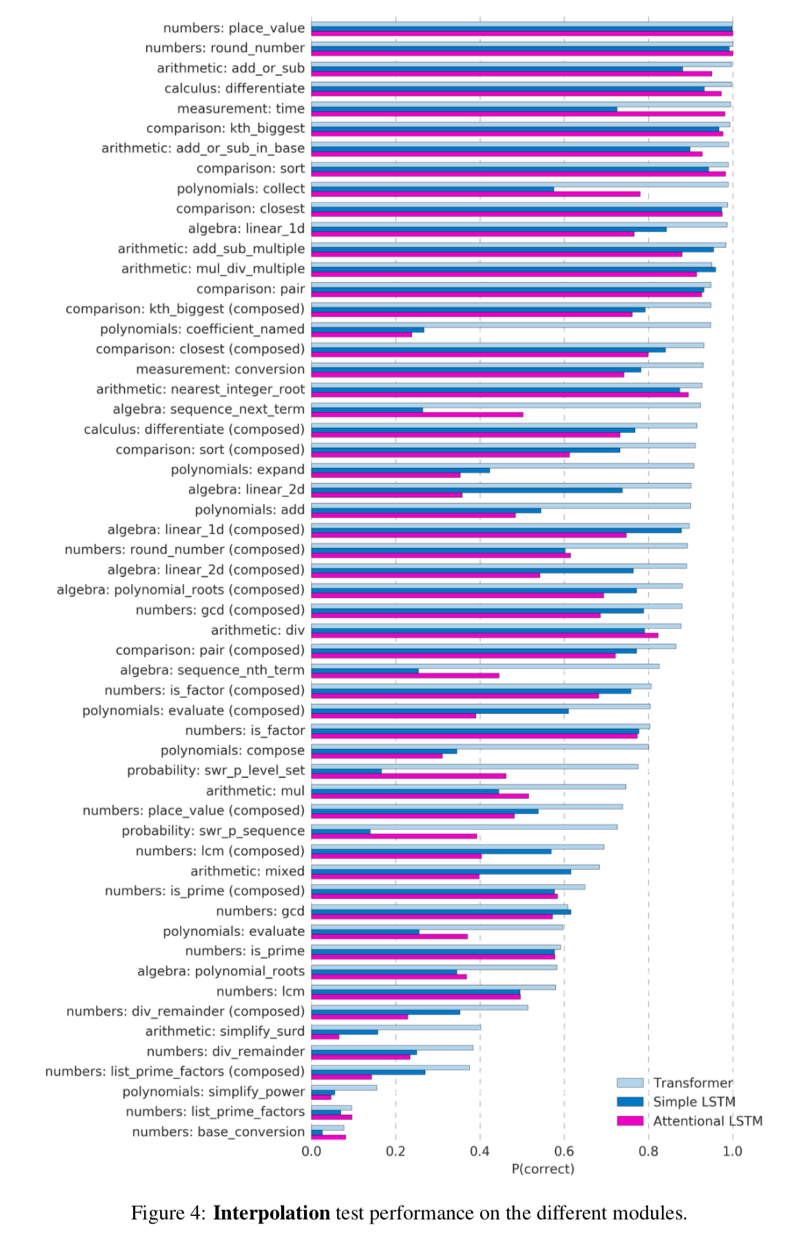
Of course, researchers from DeepMind have just started working with mathematics and AI. In the future we can expect more success, as it was with the same AlphaGo.
The full research data can be found at
this link .

