See through the illusions of the fabricated world
"On the Internet, no one knows that you are a dog"So says the dog sitting at the computer in a caricature of Peter Steiner in the weekly New Yorker of 1993. The caricature captured the radical changes in the character of human interactions, which had just begun in 1993, changes that not only delight with their capabilities, but also frighten for the same reason.
Over the past quarter century, we all learned the "dog lesson." Anyone, anywhere can be a random stranger on the Internet. An experienced manager at a music forum can be a kid in his mom's basement. A fourteen-year-old girl in chat can be a police officer under cover. The African oil heiress in your inbox is undoubtedly a crook.
')
But while we learned not to trust names and texts, the images are different. We assume that the image must have a real source and we tend to trust the images. A business profile with a photo clearly belongs to someone. The person in the photo, on a dating site, may be 10 pounds heavier or 10 years older, but if there is a photo, the person obviously exists.
But this is no longer the case. New machine learning algorithms allow you to quickly create synthetic "photos" of people who have never been.
Computers are good, but the visual processing ability of your brain is even better. If you know what to look for, you may notice these fakes at a glance (at least for the moment). The hardware and software used to generate them will continue to be improved and it may take only a few years until people fall behind in this race of fakes and detection.
Our goal is to let you know how easily you can fake a digital identity, and help you discover these fakes with just one glance.
Technique
Let's begin by emphasizing that we are not the authors of the phenomenal algorithm used to create these faces. The StyleGAN algorithm (
the Github repository ) used to create these images was developed by Tero Karras, Samuli Laine and Timo Aila from NVIDIA based on the earlier work of Ian Goodfellow and his Generative Adversarial Neworks colleagues (GAN).
In February 2019, NVIDIA graphics hardware maker discovered the code for its photo-realistic software for face generation - StyleGAN. The software uses a generative-adversarial network approach, in which two neural networks play cat and mouse, one trying to create artificial images that are indistinguishable from real photographs, and the other trying to determine the difference. Two networks teach each other. After a few weeks, the network for creating images can create images similar to fakes on this site.
The StyleGAN algorithm synthesizes photorealistic faces.In addition to the code, NVIDIA published weights for a fully trained neural network so that users can skip the lengthy learning process and immediately start generating faces. The network was trained on images from a combination of two large photo collections of CELEBA-HQ and
FFHQ . The first includes thousands of photographs of celebrity faces. And the second is 70,000 photos of people posted on Flickr under a Creative Commons license.
On our website, in the form of a game, we present pairs of images: the real one from the FFHQ collection and the synthetic one, generated by StyleGAN and posted on this
personondoesnotexist.com (web demonstration of the StyleGan system,
to generate a new image ).
Learn to identify fake faces at first sight.
No matter how remarkable the StyleGAN algorithm is, it leaves a few “hints” in each image it creates. They vary from image to image, not everyone has all or even many of these “glitches”, but after a little practice you can learn to identify them at a glance. We learned many of these tricks from an excellent
textbook published by Kyle McDonald in 2018.
- Water spots
We cannot expect future algorithms to have this problem, but one of the distinguishing features of the current StyleGAN algorithm is that it usually creates brilliant spots that are somewhat like water spots on old photographic prints. Such spots may appear anywhere on the image, but often appear on the border between the hair and the background.
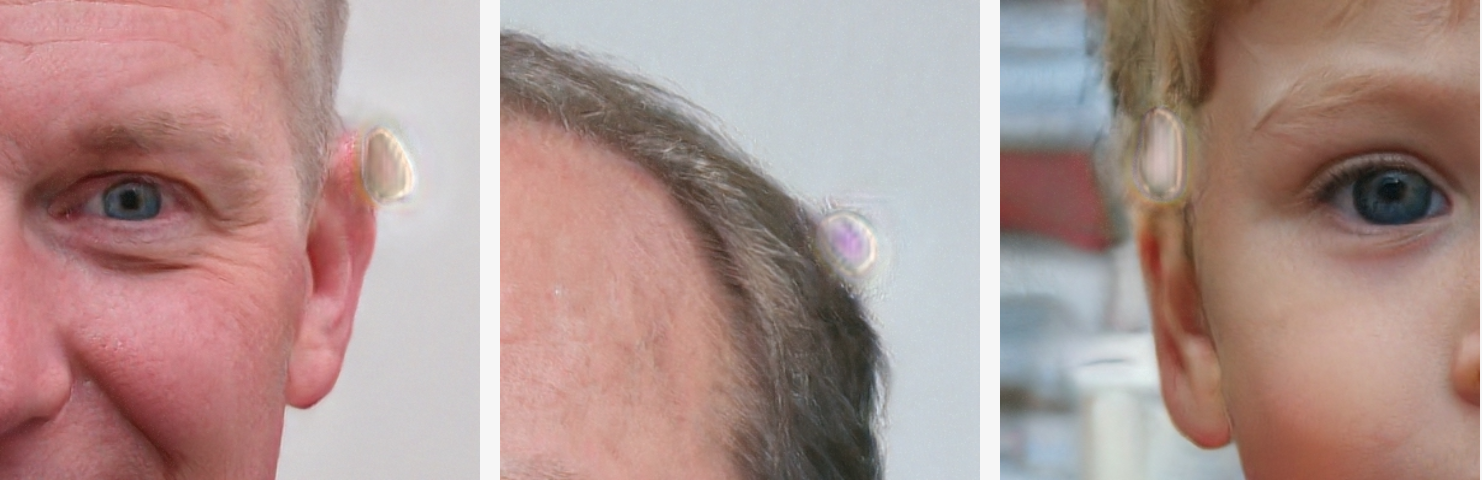
- Background problems
Generating background images may go wrong. The neural network trains on the face, and does not pay so much attention to what is happening on the sides. In the worst case, you will get some very strange "companions", as in the first figure below. Sometimes you just get chaotic cubic shapes. And sometimes the background looks almost like a torn picture.

- Glasses
Currently, algorithms are very difficult to create realistic glasses. A common problem is asymmetry. Often the frame will have different styles on the left and on the right. In other cases, the frame will simply be curved or uneven.
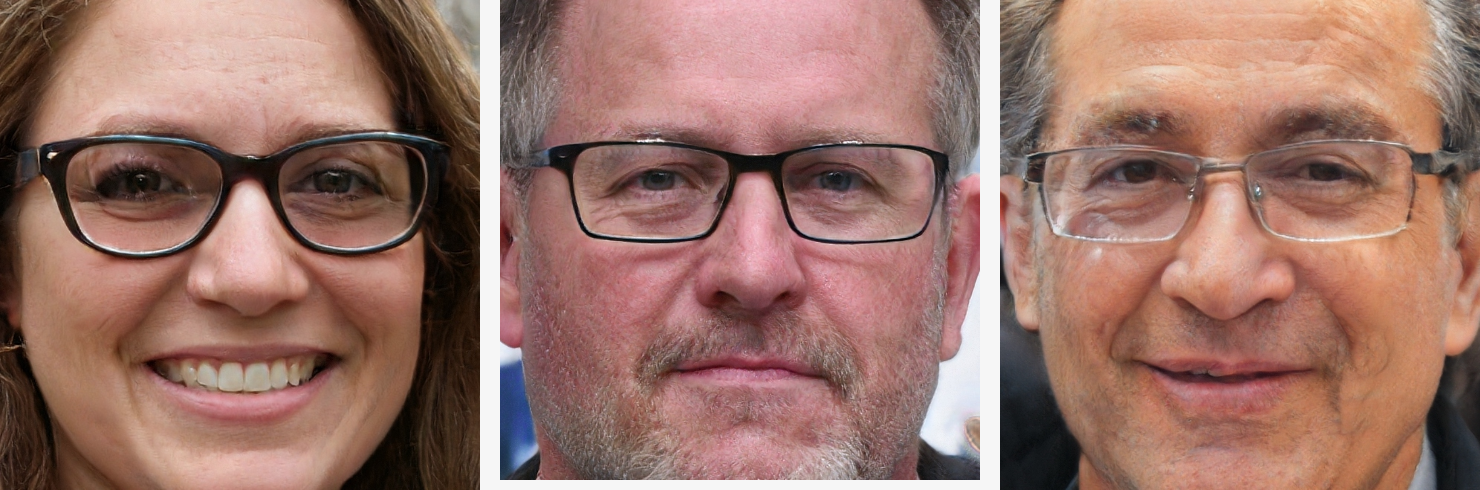
- Asymmetry
In general, symmetry is a problem for face generation algorithms. In addition to asymmetrical glasses, pay attention to the asymmetry in the hair on the face, the different earrings in the left and right ear, as well as the various forms of the collar or fabric on the left and right side.
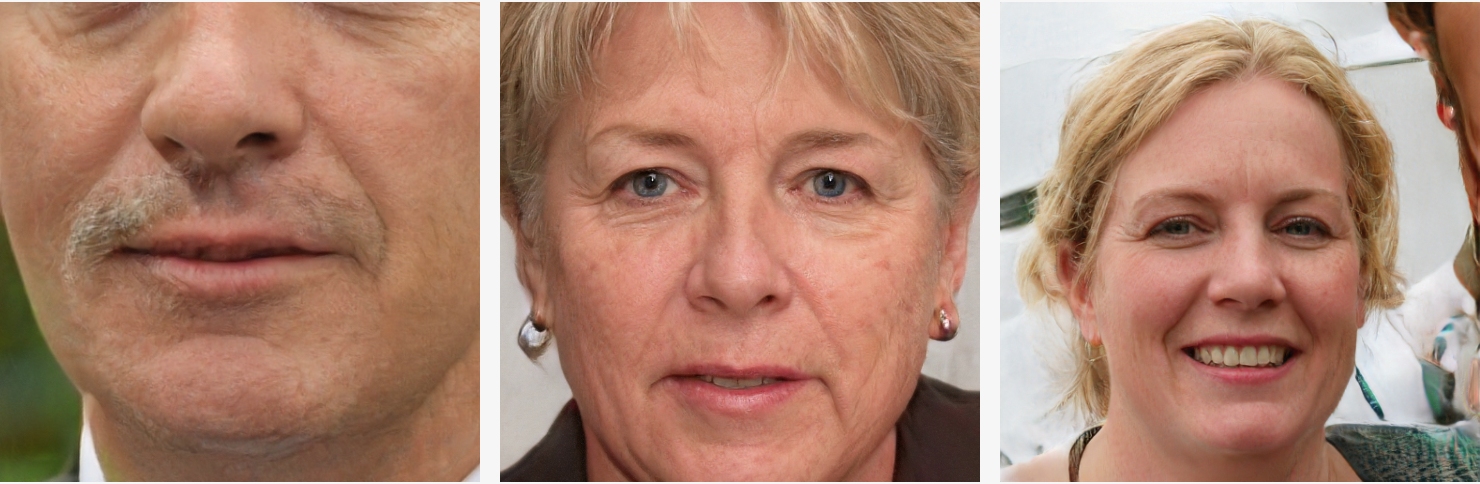
- Hair
Hair is very difficult to make realistic. Sometimes on the face or anywhere else there will be detached strands of hair, as in the first image below. In other cases, the hair will be too straight, with “highlighting,” as Kyle McDonald put it, “it’s as if someone smeared a bunch of acrylic with a spatula or a huge brush.” We see it in the middle image. Sometimes a strange glow or halo appears around the hair, as in the last image.

- Oozing fluorescence
There is one interesting feature: fluorescent colors sometimes flow from the background onto the hair or face.

- Teeth
Teeth are not easy to portray. Often the teeth are strange or asymmetrical. In some cases, you may even see three cutters, as in the last photo below.
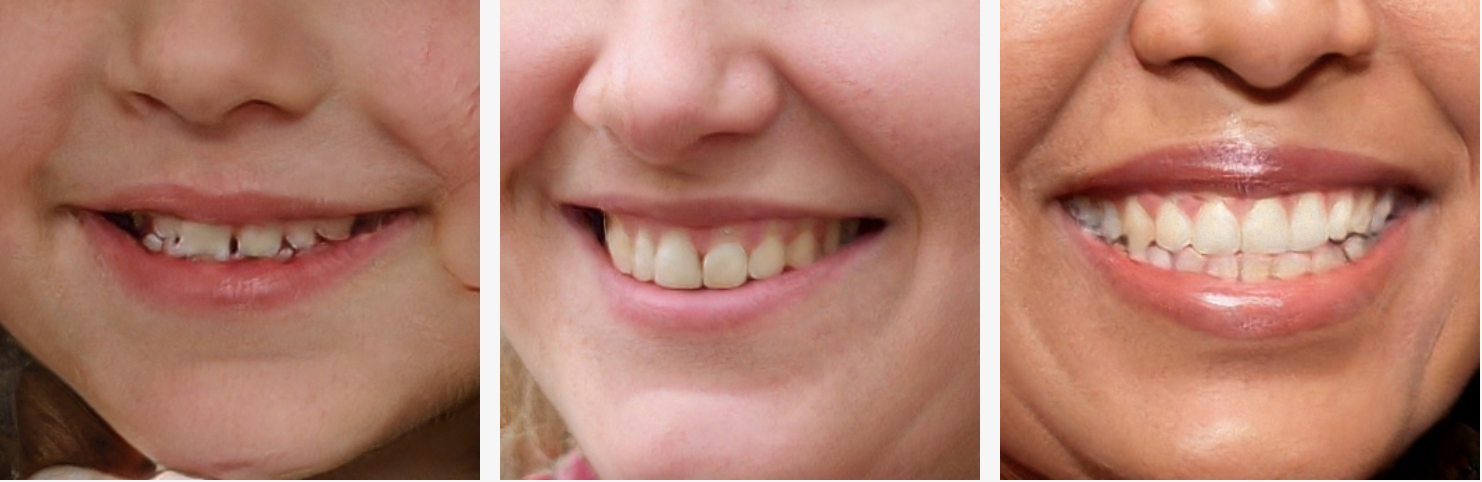
Signs that the picture is real :
Finally, now that you know which things are difficult for the neural network, you can find the signs of a real image and be confident enough that the image is real. These include symmetrical glasses and earrings, real satellites in the photo and detailed backgrounds, especially with readable text.
Given all this, you can play our
game .
You will find that by practicing a little bit, you can detect fake images very quickly.