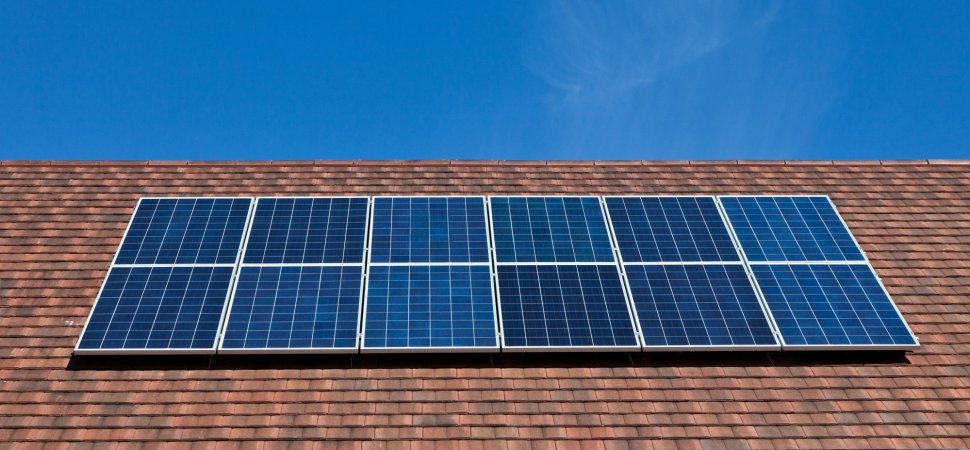
Scientists from the United States
created a neural network to detect solar panels on satellite images. In addition, it is able to predict the dynamics of the distribution of panels in a particular region, depending on the various characteristics of the region, including socio-economic. According to the results of the system, the developers created a map with information about the popularity of solar energy in the United States.
A team of scientists led by Professor Ram Rajagopal is working on his project, called DeepSolar, at Stanford University. The basis of the project is the quite popular Inception-v3
convolutional neural network , which was trained using dataset from 1.28 million photographs of various objects.
The network has been modified in accordance with the tasks. To continue the work, the specialists cleared the parameters of the last layer of the neural network, creating a specialized dataset.
')

The neural network was partially taught on Google Maps satellite imagery, and partially on images from other sources. Google map service was used because the maps have information about the presence of solar panels. The convolutional neural network was “punished” for incorrect recognition of objects on the map.
As a result, she was able to learn how to determine the presence of solar panels with an accuracy of over 93%. Next was added a layer that can select panels on the image - both small areas and large regions of "solar farms". The final test of the neural network was carried out on the basis of scanning the database containing more than 1 billion images of various US regions from satellites.
As a result, scientists have obtained a fairly large database, which includes the coordinates of the location of solar panels in almost the entire United States. As it turned out, there are more than 1.47 million such objects in the country, which is more than the number in the databases of various photocell accounting systems. The project of Stanford scientists is even more ambitious than that of Google - the company keeps records of solar panels as part of the Google Sunroof project. At the same time, the DeepSolar neural network is able to distinguish between “home” panels and industrial panels that cover a large area.
It is clear that in the second case the panels are easier to distinguish, and the accuracy of recognition by their neural network is over 96%.

At the next stage of the project, the experts compared the actual data with various characteristics of the regions, as mentioned above. As it turned out (and this can hardly be called a surprise), there are more panels in economically developed locations than in not very secured ones. Next comes the "plateau", which is formed after exceeding the income limit of $ 150 thousand per household. We also managed to find out that solar panels are starting to appear in the region in the event that energy production is 4.5-5 kWh per day per square meter.

Based on the data collected, researchers have developed a method for predicting the degree of penetration of solar panels in certain regions. The technique uses 94 different parameters, including the level of insolation, electricity tariffs, the income level of residents and others. The result of the calculations is the forecast of the number of solar panels per household.
According to the developers, their neural network can be used to monitor the penetration rate of solar panels in various regions, not only in the United States, but also in other countries. Gradually, scientists are going to analyze the distribution of solar energy worldwide, using high-quality images from Google and other sources as a basis. The database will be updated annually, despite the fact that this is a non-commercial project.
Neural network and its work can be used to achieve various goals. For example, analyzing the situation with the spread of photovoltaic cells to assess the effectiveness of programs for the development of alternative energy. Analysts may use the data in their own interests, although it is not yet clear whether the data will be provided free of charge or have to be paid for their use. Be that as it may, it is clear that the AI ​​and neural networks are increasingly penetrating into our life and work.