Translator's commentary: A detailed interview with the legendary linguist, published 6 years ago, but in no way lost its relevance. Noam Chomsky - “modern Einstein”, as it is called, shares his thoughts on the structure of human thinking and language, artificial intelligence, the state of modern sciences. The other day he turned 90 years old, and this seems to be a sufficient reason to publish an article. The interview is conducted by a young cognitive scientist Yarden Kats, he himself is well versed in the subject, so the conversation is very informative, and the questions are as interesting as the answers.
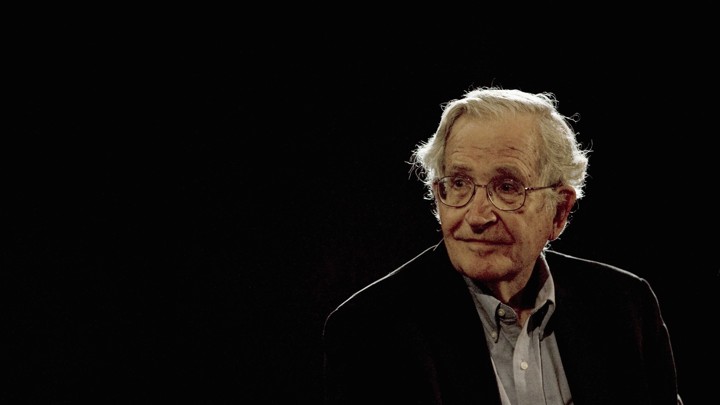
If we aim to make a list of the greatest and most unattainable intellectual tasks, then the task of “decoding” ourselves - understanding the internal structure of our minds and brains, and how the architecture of these elements is encoded in our genome - would definitely be at the top. However, the various areas of knowledge that were taken on this task, from philosophy and psychology to computer science and neuroscience, are overwhelmed by disagreement about which approach is correct.
In 1956, computer scientist John McCarthy introduced the phrase "artificial intelligence" (AI) to describe the science of studying the mind by recreating its key features on a computer. Creating a smart system using hand-made equipment, instead of our own “equipment” in the form of cells and tissues, should have become an illustration of complete understanding, and entail practical applications in the form of smart devices or even robots.
')
However, some of McCarthy’s colleagues from related disciplines were more interested in how the mind works in humans and other animals. Noam Chomsky and his colleagues worked on what later became known as cognitive science - the discovery of mental concepts and rules that underlie our cognitive and mental abilities. Chomsky and his colleagues overturned the dominant at that moment paradigm of behaviorism, led by Harvard psychologist B.F. Skinner, in which the behavior of animals was reduced to a simple set of associations between action and its effect in the form of encouragement or punishment. The shortcomings of Skinner's work in psychology became known from Chomsky’s 1959 criticism of his book Verbal Behavior, in which Skinner tried to explain language skills using behavioral principles.
Skinner's approach focused on the association between stimulus and animal response — an approach that is easily representable as an empirical statistical analysis that predicts the future as a consequence of the past. The concept of the Chomsky language, on the other hand, focused on the complexity of the internal representations encoded in the genome and their development in the course of obtaining data into a complex computer system that cannot be simply decomposed into a set of associations. The behavioral principle of associations could not explain the richness of language knowledge, our infinitely creative use of it, or why children quickly learn it from the minimal and noisy data that the environment provides them. “Language competence,” as Chomsky called it, was part of the body’s genetic makeup, as was the visual system, the immune system, the cardiovascular system, and we should study it just as we study other, more mundane biological systems.
David Marr, a specialist in neuroscience — Chomsky’s MIT colleague — identified a common approach to studying complex biological systems (such as the brain) in his sensational book, "Vision," and Chomsky’s language competence analysis more or less fits this approach. According to Marr, a complex biological system can be understood at three different levels. The first level (“computational level”) describes the system input and output, which define the task performed by the system. In the case of the visual system, the input can be an image projected on our retina, and the output can be the identification of objects in the image by our brain. The second level (“algorithmic level”) describes the procedure by which input turns into output, that is: how the image on our retina can be processed to achieve the task described at the computational level. Finally, the third level (“implementation level”) describes how our biological equipment from cells performs the procedure described at the algorithmic level.
Chomsky and Marr’s approach to understanding how our mind works is as far from behaviorism as possible. Here, the emphasis is on the internal structure of the system, which allows it to perform a task, rather than on an external association between the past behavior of the system and the environment. The goal is to penetrate the “black box” that controls the system and describe its internal structure, something like a programmer can explain to you the principle of how a well-developed software product works, and also instruct how to run it on a home computer.
As is now commonly believed, the history of cognitive science is the history of Chomsky’s apparent victory over Skinner’s behavioral paradigm — an event often referred to as a “cognitive revolution”, although Chomsky himself denies such a name. This accurately reflects the situation in cognitive science and psychology, but in other related sciences, behavioral thinking is not going to die. Behaviorist experimental paradigms and associationist explanations of animal behavior are used by neuroscience specialists, whose goal is to study the neurobiology of laboratory animal behavior, such as rodents, where the three-level system approach proposed by Marr is not applicable.
In May 2011, in honor of the 150th anniversary of the Massachusetts Institute of Technology, a symposium “Brains, Minds and Machines” (Brains, Minds and Machines) was held, at which leading informatics scientists, psychologists and neuroscience specialists gathered to discuss past and future artificial intelligence and its connection to neuroscience.
The implication was that the meeting would inspire everyone with interdisciplinary enthusiasm for reviving the scientific question from which the whole field of artificial intelligence grew: How does the mind work? How did our brain create our cognitive abilities, and can it ever be embodied in a machine?
Noam Chomsky, speaking at the symposium, was not enthusiastic. Chomsky criticized the AI sphere for adopting an approach similar to behaviorism, only in a more modern, computationally complex form. Chomsky said that reliance on statistical techniques for finding patterns in large volumes of data is unlikely to give us the explanatory guesses that we expect from science. For Chomsky, a new AI — focused on using statistical training techniques for better data processing and making predictions based on them — is unlikely to give us general conclusions about the nature of rational beings or how thinking is arranged.
This criticism evoked Chomsky’s detailed response from Google’s research director and renowned AI researcher, Peter Norvig, who defended the use of statistical models and argued that the new AI methods and the definition of progress were not far from what was happening. and in other sciences.
Chomsky replied that the statistical approach may be of practical value, for example, for a useful search engine, and it is possible with fast computers that can process large amounts of data. But from a scientific point of view, Chomsky believes, this approach is inadequate, or, speaking more strictly, superficial. We have not taught a computer to understand what the phrase "physicist Sir Isaac Newton" means, even if we can build a search engine that returns plausible results to users who enter this phrase there.
It turns out that there are similar disputes among biologists who are trying to understand more traditional biological systems. As the computer revolution opened the way to the analysis of large amounts of data, on which the whole “new AI” holds, so the sequencing revolution in modern biology gave rise to flowering fields of genomics and system biology. High-throughput sequencing, a technique by which millions of DNA molecules can be quickly and cheaply read, has transformed the genome sequencing from an expensive 10-year-long enterprise into a laboratory procedure available to ordinary people. Instead of the painful study of separate isolated genes, we can now observe the behavior of a system of genes acting in cells as a whole, in hundreds, thousands of different conditions.
The sequencing revolution has just begun, and a huge amount of data has already been obtained, bringing with it excitement and new promising prospects for new therapies and diagnostics of human diseases. For example, when the usual medicine does not help a certain group of people, the answer may be in the patients' genome, and there may be some feature that does not allow the medicine to work. When enough data has been gathered to compare the relevant features of the genome in such patients, and the control groups are correctly selected, new customized medicines may appear leading to something like “personalized medicine”. It is understood that if there are sufficiently developed statistical tools and a sufficiently large data set, interesting signals can be drawn from the noise created by large and poorly studied biological systems.
The success of such phenomena as personalized medicine and other consequences of the sequencing revolution and the system-biological approach is based on our ability to work with what Chomsky calls the “mass of raw data” —and this puts biology at the center of the discussion, like the one in psychology and artificial intelligence since the 1960s.
Systems biology also met with skepticism. The great geneticist and Nobel laureate Sydney Brenner once defined it: “low input, high throughput, no output science” (loosely translated: “much ado about nothing, and no science at the end”). Brenner, the same age as Chomsky, who also participated in that symposium on AI, was just as skeptical about the new systemic approaches to understanding the brain. Describing a popular systems approach to mapping brain circuits, called Connectomics, which attempts to describe the connections of all neurons in the brain (that is, makes a diagram of how some nerve cells are connected to others), Brenner called it a "form of madness."
The ingenious attacks of Brenner on systems biology and related approaches in neuroscience are not far from Chomsky’s criticism in the direction of AI. Unlike externally, systems biology and artificial intelligence encounter the same fundamental task of reverse engineering of a highly complex system, whose internal structure is mostly a mystery. Yes, emerging technologies provide a large array of data related to the system, of which only a fraction can be relevant. Should we rely on powerful computational capabilities and statistical approaches to isolate the signal from noise, or should we look for more basic principles underlying the system and explaining its essence? The desire to collect more data is unstoppable, although it is not always clear what theory this data can fit into. These discussions raise the eternal question of the philosophy of science: What makes a scientific theory or explanation satisfactory? How is success determined in science?
We sat with Noam Chomsky on a April afternoon in a rather messy conversation, hiding in a secret corner of Frank Gehry’s dizzying Static Center MIT building. I wanted to better understand Chomsky’s criticism of artificial intelligence, and why, he believes, he is moving in the wrong direction. I also wanted to explore the application of this critique to other scientific areas, such as neuroscience and systems biology, which all work with the task of reverse engineering complex systems — and where scientists often find themselves in the midst of an infinitely expanding sea of data. Part of the motivation for the interview was that Chomsky is now rarely asked about science. Journalists are too interested in his opinion on US foreign policy, the Middle East, the Obama administration, and other common topics. Another reason was that Chomsky belongs to that rare and special type of intellectuals who is rapidly dying out. Since the publication of the famous essay by Isaiah of Berlin, various thinkers and scholars began to put Fox-Hedgehog as a favorite entertainment in the academic environment: Hedgehog - meticulous and specialized, aimed at consistent progress in clearly defined framework, against Fox, more rapid, driven by ideas thinker who jumps from question to question, ignoring the scope of the subject area and applying his skills where they apply. Chomsky is special because he makes this distinction into an old and unnecessary cliché. Chomsky has no depth in return for flexibility or breadth, although, for the most part, he devoted his entire early scientific career to the study of certain topics in linguistics and cognitive sciences. Chomsky’s work has had a tremendous impact on several areas besides his own, including computer science and philosophy, and he doesn’t move away from discussing and criticizing the influence of these ideas, which makes him especially interesting for interviewing people.
I want to start with a very simple question. At the dawn of artificial intelligence, people were optimistic about progress in this area, but everything turned out differently. Why is the task so difficult? If you ask specialists in the field of neuroscience why it is so difficult to understand the brain, they will give you absolutely unsatisfying answers: there are billions of cells in the brain, and we cannot read them all, and so on.
Chomsky: There's something in that. If you look at the development of science, all sciences are like a continuum, but they are divided into separate areas. The greatest progress is achieved by science, which studies the simplest systems. Take, for example, physics — there is tremendous progress in it. But one of the reasons is that physicists have an advantage that no other science has. If something gets too complicated, they pass it on to someone else.
For example, chemists?
Chomsky: If the molecule is too big, you give it to the chemists. Chemists, if for them the molecule is too large or the system becomes too large, give it to biologists. And if it’s too big for them, they give it to psychologists, and in the end it ends up in the hands of literary critics, and so on. So not everything that is said in neuroscience is completely wrong.
But maybe - and from my point of view it is very likely, although neuroscience doesn’t like it - that neuroscience has been on the wrong path for the last couple of hundred years. There is a fairly good book by very good neuroscientist, cognitive scientist Randy Gallistel, together with Adam King, which he says — in my opinion, plausibly — that neuroscience developed, being fascinated by associationism and related ideas about how people and animals work. As a result, they were looking for phenomena with associative psychology properties.
How with hebbov plasticity? [Theory attributed to Donald Hebb: associations between environmental stimuli and stimulus responses can be encoded by enhancing synaptic connections between neurons - approx. Ed.]Chomsky: Yes, as the strengthening of synaptic connections. He spent years trying to explain: if you want to study the brain properly, you need, like Marr, to first ask what tasks he performs. Therefore, he is mainly interested in insects. So, if you want to study, say, the ant's neurology, you ask, what does an ant do? It turns out that ants do quite complex things, such as building a path. Look at the bees: their navigation requires quite complex calculations, including the position of the sun, and so on. But in general, with which he argues: if you take the cognitive abilities of an animal or person, these are computing systems. So you need to look at computational atomic units. Take the Turing machine, this is the simplest form of calculation, you need to find atoms that have the properties "read", "write" and "address". These are minimal computational units, so you need to look for them in the brain. You will never find them if you look for the enhancement of synaptic connections or the properties of fields, and so on. You need to start with the following: see what is already there and what works, and you can see it from the highest level in the Marr hierarchy.
True, but most neuroscientists do not sit and do not describe the inputs and conclusions of the phenomenon they are studying. Instead, they put the mouse in a laboratory learning task, and record as many neurons as possible, or find out if the X gene is necessary for learning the task, and so on. Such assertions stem from their experiments.
Chomsky: It is so ...
Is there a conceptual error in this?Chomsky: Well, you can get useful information. But if there really is some kind of calculation that involves atomic units, you will not find them in this way. It's about how to look for lost keys under a different lantern, just because it's lighter there (
referring to a well-known joke - note transl. ). This is a debatable question ... I do not think that the position of Gallistel was widely accepted by neurobiologists, but this is a plausible position, and it was made in the spirit of Marr’s analysis. , , , . , , , . , . , , . , , — , . , , — , , , . , , . , -, , -, .
, , , , , , , « », . , — , . , [] . , — , . ?: . , , MIT, (
— . . ) C. elegans , , , , , 800 …
, 300..: … , , [ C. elegans] . , .
. , « » (GOFAI), , , , , , , . , , — , — . : , ?: . : , , , , , .
….: , … , , , . , (
— . . ). , , , , , , . , , , , .
, , , - , . «» — , , , , — , , , -.
( , — . ) — - , . , , . , , , , , , — , , . , — , .
. , . : , , : , , , , , , , , , , , . , . , — - , . , , . , , . , , . , , - , , Wall Street Journal — .
, — , , , , , — , — , . . — , , . — , -, . , , , , . , , , …
… ?: … . , , . , , , . MIT 1950-, . , , . , . , . 1960- , -. , — . , , , . . , , , , . , , , 10 . , , , . . , , , , , , , . , - , — , - , - — , , , .
Clear. . , , …: … , , , .
. , , , , , , , . - , — , — . .
: , .
?: , . , ? , , - ? , , ?
. : , ...: . , : , . . : , . . , , « ». , , , , , . - , , , , . This is the first step. ? , . . , , , - . , , , , — . , , — . , , : «, », — — , . , , . , , «» «», . , , . -, , . , , , , — — , , . , , . : , , , , , - . , -, — , , . , …
, ...: … , , . , , — , . : « », «» «», «», . . «», , , . . , . — , . , , . , .
, , , , : . . . , - , . , , , , . — , . , , 17 , , — , — , . : , , . , ? , , , , .
, - , . , - . 1640 , - , , . — , — , , . , , , . , — , . , , - . , , , . , , — , , , . , . , - , , . , : , , , , .
, , — , — …: … , .
… , , .: , . . , , - , — . , , , , , . , . . , , , . . . . , , , , , . , . . — , . , , — . , . , , .
....: . , . . , , , . , , , , .
, ?: — , , . . , , , -, , , , , — . , . : , , , , , , , .
, [, ], , , , .: , . , - . , . , , . , …
…: , . — — - . . …
, ...: []. , , — - , , , … , , , , , . , , . : , , [
. ed. : ( ), , ] - , — . , , , , . , . , : , . , , , - .
, , ?: . , . . , , . , , , , : , , . , , , , -, — .
, — , - .: . . , . , , . . , . , — . , , . , 7 6, : « , , 10» — 3, 3, 10 3, 13. — , . .
— . . . , . . . — . , . , . , , . — , . .
, , 3 ...: …
… ...: . AND…
… , .: , . , , , - . — , , — , , , , . , . , , . . , . , , — , . , . , , - . — , , . , - , , .
. , , — , - ...: , , . .
, , .: . . , - . , , , , -. , . — , , , — - . , . , — , , — , , .
, : , , .: . , . , , , .
, . , , .: . , , , . []. , , , , , . , , , - , .
, . , , , . , , — .: , , — . , . , , , //.
. , , , . — .: , , . , . — , , . . — . , , .
, , , .: , . , — .
, , , … , — ?: . , , , , , . , . , , , . , , . . — , . , .
, , , , .: . , , , .
« X » « », , . , .: , , , .
— , .: . , . , , , . , . , . , . , .
. , « ». . : , , , . . , , — .: , , . , . , , .
( — . . ).: , . — [].
.: , , . — , — , , . , , , , . , , , , . . , : , ? : . , , .
, , .: , - . : , , , , .
, « »? [ MIT/] , , , . -, , ?: , , , . .
, - ? , Foxp2? [ . : , , , . , . , .]: Foxp2 , . . , , — , , . , , , , , — . , , , . , . , , , . , .
, , . , , , . , , , . , , . , .
, , ?: , . , — — , . , : « ?» «» , . , , — , — - , , . , , . , , : . , , , . , . , , .
, . : « » — . ? ? , , , . , , .
, . : «The horse raced past the barn fell.» (
, , — . . ). , , , , . «The horse raced past the barn» , : «fell» ? , , . , , -, . , , - .
. , - , . : . : « , ». : « , , ?» . , . « , , ?» - , . , . , . , , , — .
, , . , , -, . , , , , .
- . : , , . , , . - , , , — , , — - , , …
?: . , , . , — . . , , , , , , .
.: , . ? , . , , , . , . , . , . , . : , — — , , . , , - .
. , , , . , MIT, , , , , , , . , -, , ? , ? , — , .
: , , — . , , , , . . , , . , , - . , -, , . , , , — , . , , , , , . , ? : .
?: . , . . , , . , . — , . , . , , « ». , . , , . , , «?», . , : , — , , . .
: . , , .
: , , — 2,5- , « » , .
— .