Trained multilayer phase mask (handwriting classifier). The right shows the physical model of the D²NN optical neural network printed on a 3D printer: layers 8 × 8 cm with a distance of 3 cm between each otherA group of researchers from the University of California at Los Angeles has developed a new type of neural network that uses light instead of electricity. The journal Science published
an article describing the idea, the working device, its performance, and the types of applications that, according to the authors, are well calculated in a new type of neural network.
Fully optical deep learning framework Diffractive Deep Neural Network (D²NN), which is physically formed from a variety of reflective or transparent surfaces. These surfaces work together, performing an arbitrary function, learned as a result of training. While obtaining the result and forecasting in the physical network is completely optically organized, the training part with the design of the structure of the reflecting surfaces is calculated on a computer.
So, in a physical model, D²NN consists of several reflective or transparent layers. On these layers, each point either passes or reflects the incoming wave. Thus, this point is an artificial neuron that is connected to the neurons of the next layers through optical diffraction. The structure of D²NN is shown in the illustration.
')
Diffraction deep neural network (Diffractive Deep Neural Network, D²NN).In Figure A, a diagram of several transparent / reflective layers, where each point is a neuron with a complex coefficient of transparency or reflection. These coefficients are derived through deep learning. After the learning phase, the D²NN design is fixed - and the corresponding plates are printed on the 3D printer, which carry out calculations based on the function obtained as a result of the preliminary training. Unlike electronic computer networks, here calculations are performed
at the speed of light .
During the experiments, scientists trained and experimentally tested several types of D²NN. Figure B shows the classifier of handwritten symbols; Figure C shows the imaging lens.
In the lower part of the illustration, the work of the diffraction optical neural network (left) and the electronic neural network (right) is compared. Based on coherent waves, D²NN works with complex input values and multiplicative offset. The weights in D²NN are based on free-space diffraction and determine the coherent interference of secondary waves, which are the phase and / or amplitude modulated by the previous layers. The symbol "ο" means the operation of Hadamard’s work, that is, the logical multiplication of the corresponding terms of two sequences of equal length.
The researchers explain that the structure of the optical neural network is organized according
to Huygens principle , according to which each wavefront element can be considered as a center of a secondary perturbation generating secondary spherical waves, and the resulting light field at each point in space will be determined by the interference of these waves. Thus, an artificial neuron in D²NN is connected to other neurons of the next layer through a secondary wave, which is modulated in amplitude and phase by both the input interference pattern created by the earlier layers and the local transmit / reflectance at this point.
By analogy with standard deep neural networks, the transmission / reflection coefficient of each point / neuron can be considered as a multiplicative term “bias” (bias), which is iteratively corrected in the process of learning the diffraction network using the back propagation error method. After numerical training, the D2NN construction is fixed and the transmission / reflection coefficients of the neurons of all layers are determined. Then you can make the calculated layers by any method: 3D printing, lithography, etc.
Scientists emphasize that the optical neural network performs the function at the speed of light and does not need energy. Thus, it is an efficient and fast way to implement machine learning tasks.
To test the idea, the researchers made a neural network able to recognize numbers from zero to nine - and report the result. After training on 55,000 number images, a printed seven-layer neural network showed an accuracy of 93.39%.
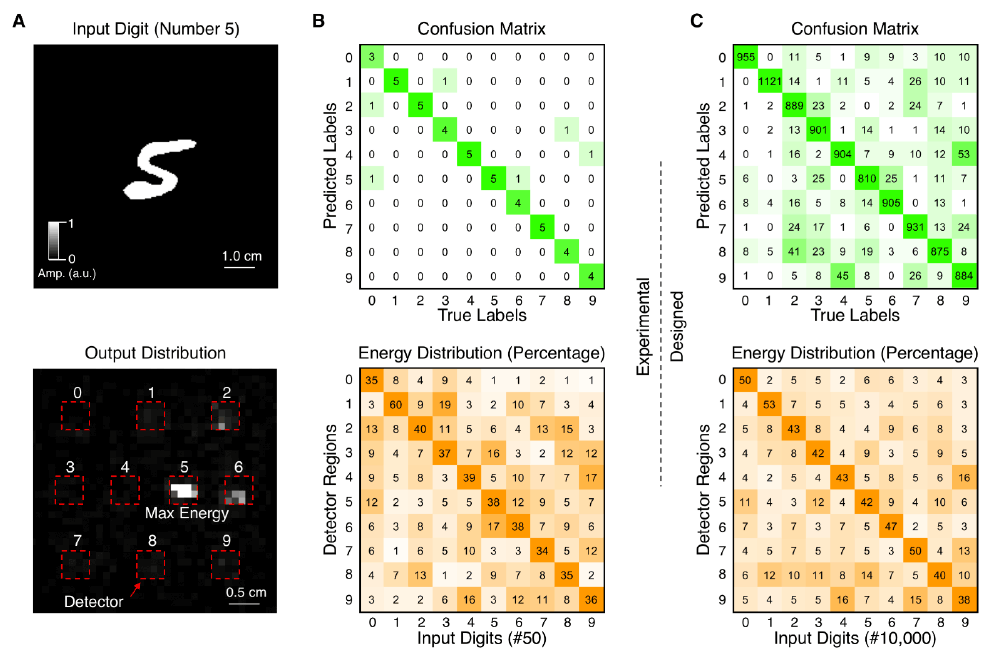
In recognition of fashionable clothes and shoes, the five-layer neural network showed an accuracy of 81.13%, a ten-layer one - 86.60%.

According to researchers, an optical type neural network can be used in specialized devices that require high speed, such as identifying a specific person in a crowd of moving people.
The scientific article was
published on July 26, 2018 in the journal
Science (doi: 10.1126 / science.aat8084,
pdf ).