Merlin Video's hybrid recommendation model for determining the audience of films. The logistic regression layer combines a collective filtering model with information about the frequency and duration of the cinema visit to calculate the likelihood of wanting to watch this movie. The model is trained from beginning to end (end-to-end), and the loss function is propagated back to all learning components.The output of the trailer is the most important element in the preparation of film premieres. A spectacular trailer raises the rating of audience expectations, introduces the audience to the plot, presents the main characters, conveys the overall mood of the picture. At the same time, according to reviews on the trailer, filmmakers have the opportunity to understand which aspects of the movie the audience likes or dislikes - this information usually becomes the basis for a further marketing campaign. The trailer directly correlates with the charges in the first days of the show. Then the figure of high fees in the early days attracted the attention of mass audiences and the media, which largely ensures the overall commercial success of the picture.
Since we are talking about hundreds of millions of dollars, the best scientists are working on creating more efficient trailers. Machine learning specialists from 20th Century Fox have published a
scientific paper describing a system called Merlin Video. This machine vision system generates a diagram of views from the trailer (in the illustration above). Representation data is used to
predict the reaction of the viewers . According to the authors of the scientific work, this is the first time that a film studio uses a computer vision system to calculate audience interest in a film.
The tool is based on the innovative hybrid model of “collective filtering” (Collaborative Filtering, CF), which isolates the characteristic features from the trailer video sequence: color, lighting, faces, objects, landscapes.
')
This information is combined with demographic data, information about the attendance of the movie theater (frequency, last visit date). As a result of the training system allows you to make accurate predictions and make recommendations based on the trailer.
The neural network was trained on Nvidia Tesla P100 GPU GPUs in the Google Cloud, in the TensorFlow depth learning framework and in the
cuDNN primitive
library . As data for training, hundreds of movie trailers used in recent years, as well as millions of records about the behavior of viewers, were used.
“Having found a suitable presentation of these features and uploading them to a model that has access to historical records of movie attendance, you can find nontrivial associations between trailer signs and future audience choices after the movie is released in movie theaters or streaming services,” the authors write.
The results of the Merlin Text (for text) and Merlin Video (for video) systems to predict the audience of the film The Greatest Showman are shown in the table. In the right column - the actual audience after the fact.
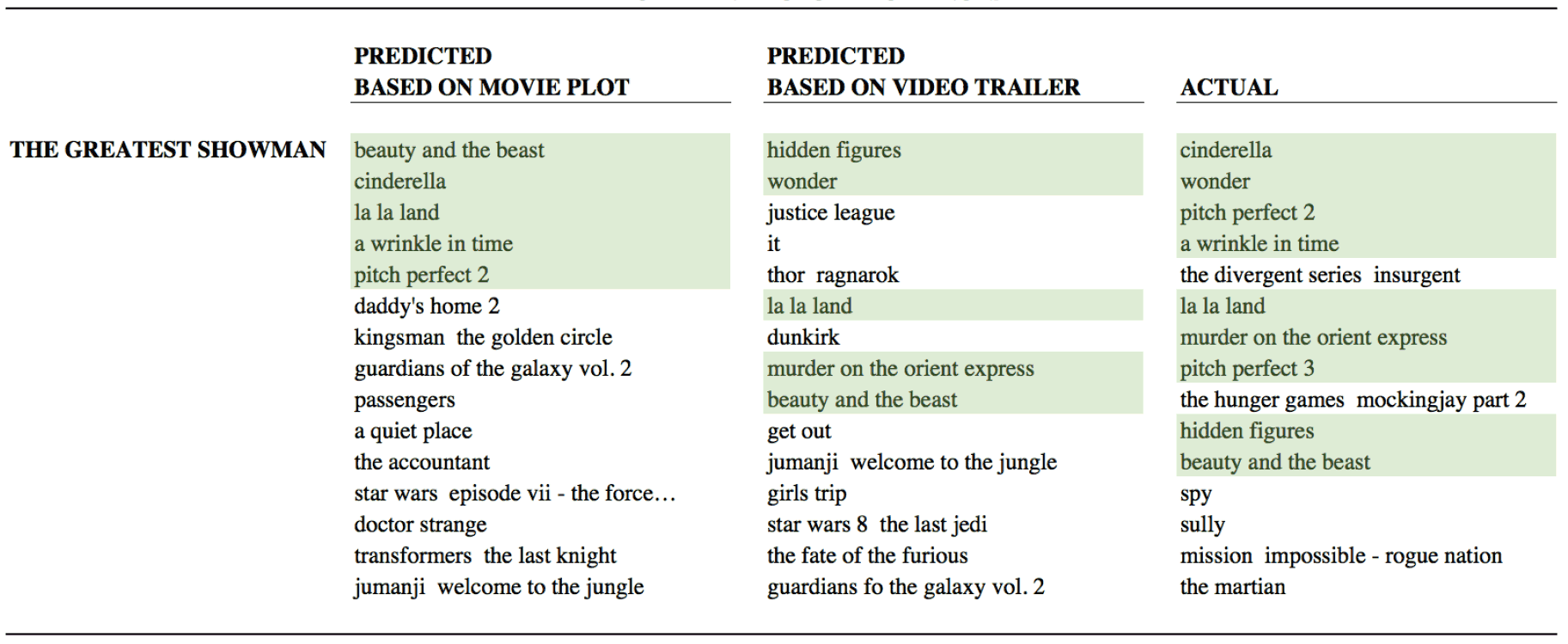
As you can see, the text analysis accurately predicted the audience of the movie, but the analysis of the video sequence added several missing pieces. Experiments have shown that a small computer system with a trailer analysis shows a 6.5% better result for AUC (area under the ROC curve) than a text analysis system, that is, a script.
With the help of such a weak Artificial Intelligence, the marketing departments of film studios will be able to more accurately understand the interests of the audience. They will be able to better understand which people will be interested in the new film. Most importantly, with which past films this audience overlaps. In this way, more effective marketing campaigns can be targeted to specific audiences.
Now researchers are working to combine in a single system the system of predicting the audience for analyzing the scenario and video sequence of the trailer. In this case, the forecast will be as accurate as possible.
The scientific article was
published on July 12, 2018 on the site of preprints arXiv.org (arXiv: 1807.04465v1).