"Pizza Future" appear around the world. In the photo - Zume Pizza from CaliforniaIn the beautiful Russia of the future, you go to the pizzeria, visit the post office or the bank, take the necessary thing - and quietly leave. There will be no queues. Just automatically debiting money from your account. At the same time, the quality of goods will increase significantly, because machines, not people, will check them.
Cloud solutions in the coming years will change the world of retail. VR, AR, video analytics, neural networks - all these technologies are connected with clouds and fall under the direct responsibility of VSaaS.
“Dodo Pizza” is an international network of pizzerias with an interesting transparency structure - data on profits and losses are open, and investors can follow vital pizzeria indicators online: revenue, average check, performance, expenses.
')
"Dodo Pizza" installs video surveillance in every pizzeria. Not only Fedor Ovchinnikov can watch the broadcast from the camera, but any person on the Dodo site. Video surveillance is part of Dodo’s openness and transparency strategy. And it is a tool to increase sales.
The basis for the success of Dodo - the desire to automate business processes. To this end, the company created a cloud information system "Dodo IS" and began to globally change the approach to video surveillance.
For Dodo, it all started with a simple desire to ensure order and show customers the process of making pizza. In each pizzeria in the kitchen there is a camera. Broadcast is conducted via the Internet and is available for viewing to all.
Over the past year, cameras in pizzerias from the “live presence” tool have evolved into a data analysis tool for video analytics.
"Dodo Pizza" used two services that extend the possibilities of video surveillance: the Dbrain platform and the Ivideon queue detector.
DBrain neural network
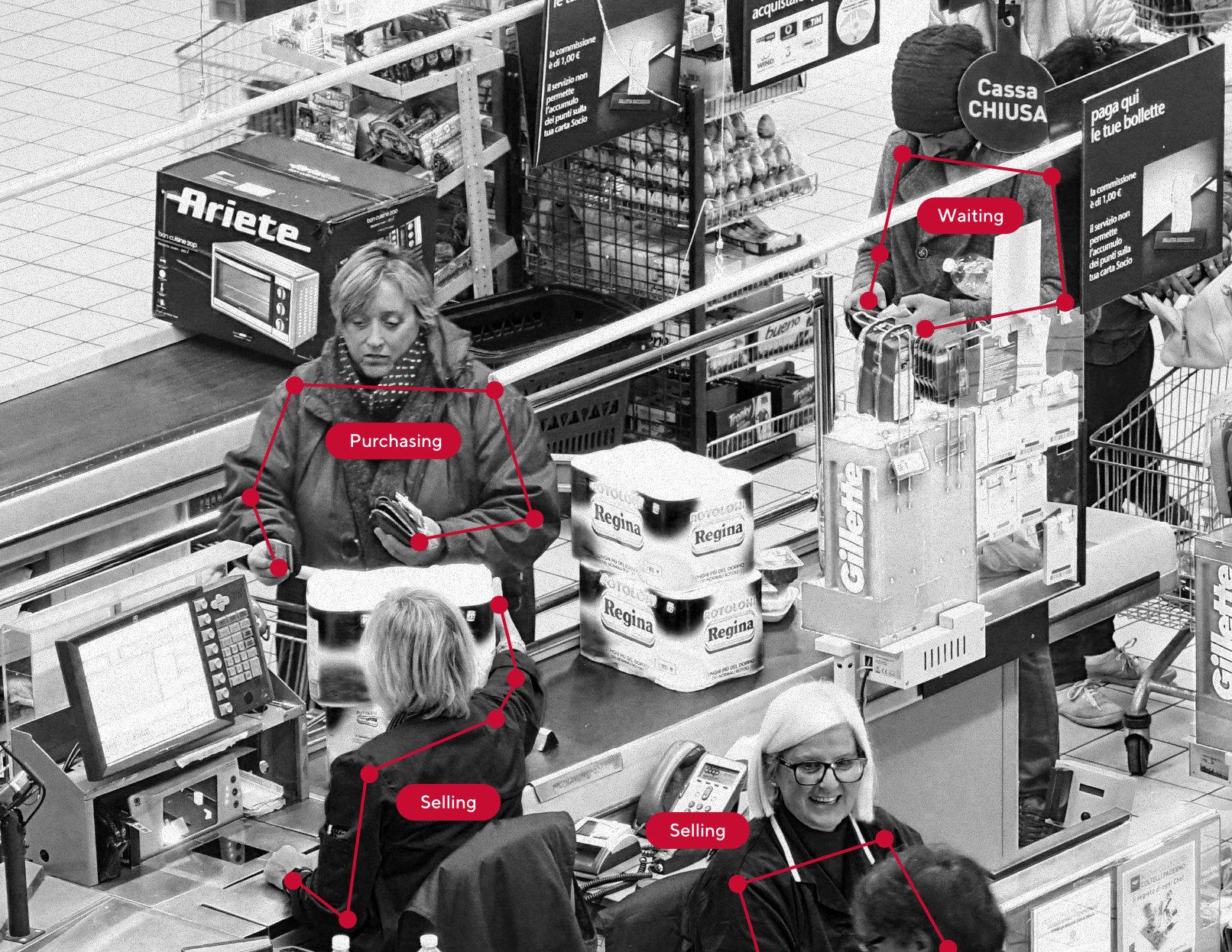
CEO and co-founder of
Dbrain - Dmitry Matskevich. A few years ago, he participated in the creation of the Icon8 chat bot, instantly processing photos using neural networks. The user chooses one of the six styles available for use in the bot - at the start of the project in 2016, this was surprising.
Icon8 gathered 7.5 million users - the most popular project at the time in Telegram, awarded with a grant from Pavel Durov.
Dmitry used the experience gained in DBrain. The company relies on neural networks and adds distributed registry technology to them.
DBrain is a blockchain platform for working together to create a "weak" AI. Training a neural network involves a large amount of manual labor when marking data - the process is expensive and time consuming. The team has figured out how to reduce the cost of creation and improve the accuracy of neural networks: any person can, for money, become a “teacher” for a neural network.
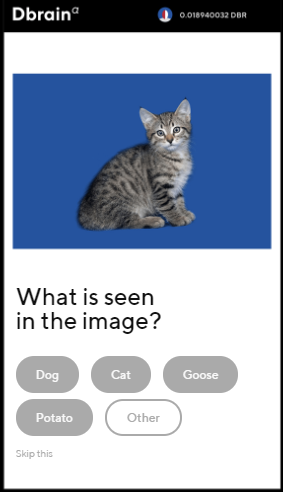
It is very simple to train a grid - we look at the photo in the application and we answer, who is depicted on it: chanterelle, cat, dog or ball of wool. Perhaps this is the most incredible interpretation of the game "The Cow MUUU".
At the request of Dodo Pizza, DBrain developed an application based on machine learning, which controls the quality of pizza cooking. The application is implemented as a Telegram-bot, evaluating pizza from a photo. The bot will independently evaluate the product from 0 to 10 by the key criterion - the quality of the test.
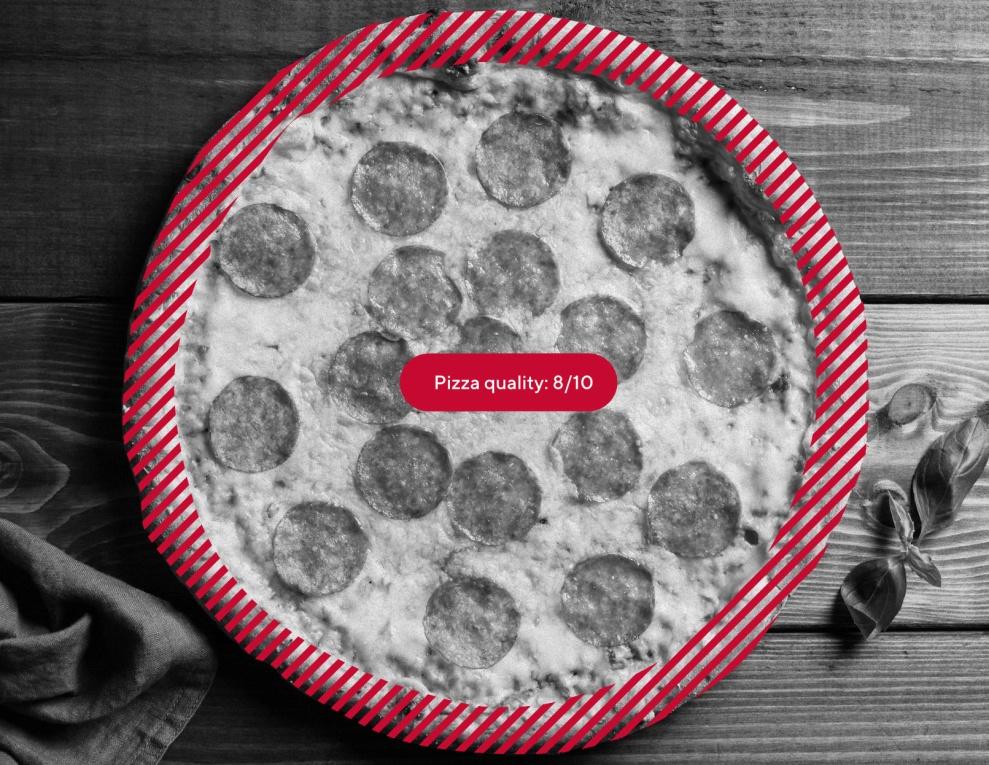
The bot works with an army of mystery shoppers - this is the inner community of “Dodo Pizza”, numbering more than 50,000 people. The quality rating of pizzerias is formed on the basis of reports of customers, who weekly evaluate almost 700 pizzas for compliance with the standards. Volunteers receive for the work of Dodo-rubles, which can pay for the pizza with the next order.
But where is the video surveillance?
Video surveillance reduces costs and fully automates the work on the ranking of pizzerias. To do this, it is enough to provide the application from Dbrain with video data instead of photos. Using the camera, the program will have time to check not 2 pizzas a week, like an ordinary person, but 300 per day - exactly everything that comes out of the oven.

Dbrain's neural network segmented pizza on 50,000 photos and learned to look for flaws in each segment. For example, if at the edges of the pizza, the net detects small swellings that are hardly visible to the human eye, it will immediately “understand” about the poor quality of the dough. At the same time, the network compares each pizza with an “ideal” model created on the basis of data provided by secret buyers and experts who professionally deal with the quality assessment of pizza.
Queue Detector Ivideon
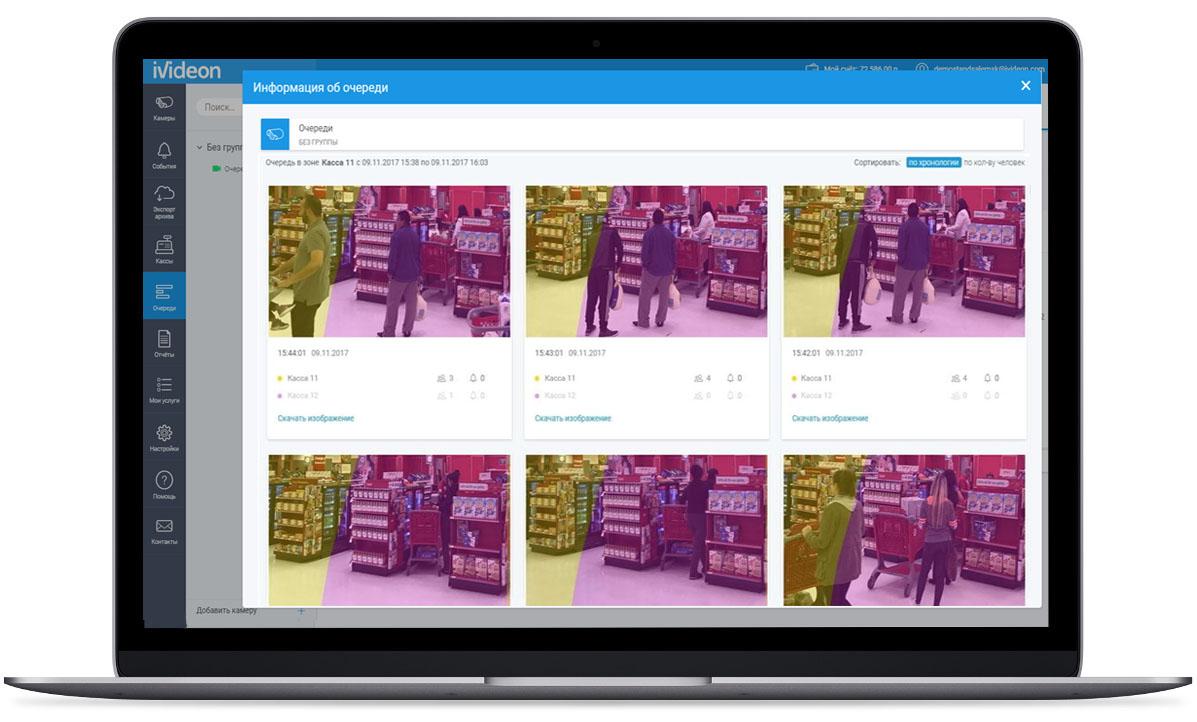
Ivideon used machine learning to create
a queue detector . Detectors have come up with a great many, but all of them are not without flaws. People in the queue do not behave like static geometric shapes. People move from place to place, disrupting the operation of external sensors, become so that they merge with the background or with each other, and prevent the camera from distinguishing objects.
Machine learning made it possible to single out the “main thing” from a person — the head. Considering the heads, it was possible to achieve almost 100% accuracy in determining the presence of a person in the frame. And then it’s enough just to count the number of people and send the manager a notification about the occurrence of a queue for an instant reaction.
The manager receives a notification and decides to open another cashier. Or close the cashier, which misses the unloaded cashier.
Another important part of the service is statistics. The detector counts the number of people in the queue, builds graphs and diagrams characterizing the distribution of customers in time. With such data it is easier to optimize the work schedule of the staff, assess the quality of the marketing campaign, or identify the drawbacks of the window dressing.
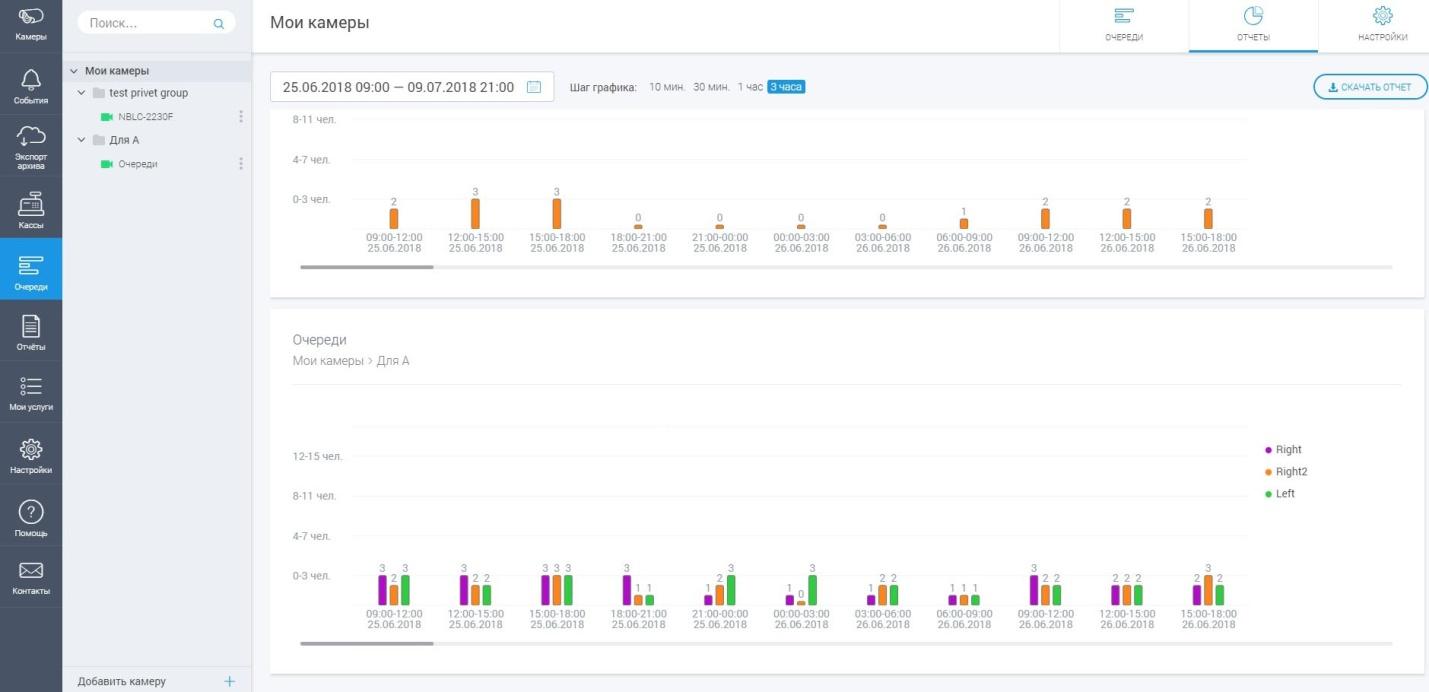
Reports are generated in the CSV format, data is also available in the Ivideon personal account interface. From the report you can find out:
â—Ź problem areas (with the possibility of comparison within one or several institutions);
â—Ź peak loads, the length and dynamics of the formation of queues;
â—Ź video data for each cluster of people.
With the report data it is easy to go further and make predictions for the days and weeks ahead. Now it is easy to find out which cash registers, in which hours receive the maximum and minimum load.

Ivideon also processes data from cash desks by check. To do this, the queue management system must be supplemented with a free
integration module with 1C. From the interface 1C, the user receives the video for any system events, such as printing a receipt, warehouse operations (acceptance, shipment), the return of the goods.
Investment valuation
The cost of the project with Dbrain "Dodo" in open sources has not yet published. Even if we take it at the maximum cost, the solution still looks profitable - the neural network does not have to ask and does not claim free pizza.
The costs of the queue detector can be seen by any user of the Ivideon service in a personal account - 800 rubles per camera per month. The same amount includes a motion detector, a cloud archive (which itself is paid), the ability to embed a broadcast from the camera to your website or share video on social networks.
For those who already use the cloud archive to store data from the camera, connecting the detector looks like an obvious step. Given the periodic updates, we should expect changes that will expand the functions of the service. After the detector of people as objects, obviously, the detector of faces will follow.
Business in this story is interested in profit. It’s early to draw conclusions from Dodo, but another company (Interlogika)
publishes the following figures: the total cost of implementing the queue detection system, taking into account the purchase of cameras (they were not at the facility), was about 500,000 rubles. Maintenance of the system costs 30,000 rubles per month. Due to the growth of quality of work and savings on staff (an additional seller appears only when needed), the average revenue of the store increased by 7%. The system will pay off in less than 1 year, and then begin to make a profit.
findings
The camera is a small-functional piece of plastic. It helps to monitor employees, customers, goods - thereby changing the quality of service. But nothing more than a flash of light and show a picture, the camera can not do.
Even 10-15 years ago, the camera was similar to the version of a security dog ​​with limited health abilities - it watches the perimeter, signals the guard about the violators, helps to solve crimes and that's it. With the advent of video analytics, the camera itself has not changed, but its capabilities have expanded many times.
It seems that all the major players in Russia are launching separate test projects with video analytics. Safmar Retail, which owns 30% of the consumer electronics market, relies on the blockchain and augmented reality. X5 Retail Group is testing Skolkovo Intelligence Retail resident technologies for monitoring the availability of goods on the store shelves using machine vision and Ivideon cameras. IIDF separately invests in the platform for shopping without queuing.
Small and medium business froze in anticipation of their fate.