The material, the translation of which we publish today, is dedicated to the story of how Airbnb optimizes server parts of web applications with an eye to the increasing use of server-side rendering technologies. Over the course of several years, the company gradually transferred its entire frontend to a
uniform architecture, according to which web pages are hierarchical structures of React components filled with data from their API. In particular, during this process there was a systematic rejection of Ruby on Rails. In fact, Airbnb is planning to switch to a new service based solely on Node.js, thanks to which fully prepared pages rendered on the server will be sent to users' browsers. This service will generate most of the HTML code for all Airbnb products. The rendering engine in question is different from most of the backend services used by the company due to the fact that it is not written in Ruby or Java. However, it differs from traditional high-loaded Node.js-services, around which mental models and auxiliary tools used in Airbnb are built.
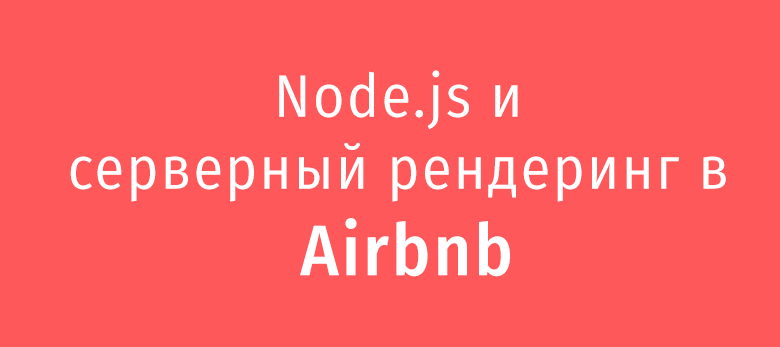
Node.js platform
Reflecting on the Node.js platform, you can draw in your imagination how an application built with the asynchronous data processing capabilities of this platform quickly and efficiently serves hundreds or thousands of parallel connections. The service pulls out the data it needs from everywhere and processes it a little to match the needs of a huge number of customers. The owner of such an application has no reason to complain, he is confident in the lightweight model of simultaneous data processing used by him (in this material we use the word "simultaneous" to convey the term "concurrent", for the term "parallel" - "parallel"). She perfectly solves her task.
Server-side rendering (SSR, Server Side Rendering) changes the basic ideas leading to a similar vision of the issue. So, server rendering requires large computational resources. The code in the Node.js environment is executed in one thread, as a result, to solve computational problems (as opposed to input / output tasks), the code can be executed simultaneously, but not in parallel. Node.js is capable of handling a large number of parallel I / O operations; however, when it comes to computing, the situation changes.
')
Since, when using server rendering, the computational part of the request processing task is increased in comparison with the part related to I / O, simultaneously incoming requests will affect the speed of the server response because they are competing for processor resources. It should be noted that when using asynchronous rendering, the competition for resources is still present. Asynchronous rendering solves the problems of responsiveness of a process or browser, but does not improve the situation with delays or concurrency. In this material we will focus on a simple model that includes only computational loads. If we talk about a mixed load, which includes both input and output operations and calculations, then simultaneously incoming requests will increase delays, but taking into account the advantage of a higher system capacity.
Consider a command like
Promise.all([fn1, fn2])
. If
fn1
or
fn2
are promises resolved by means of the I / O subsystem, then during the execution of this command, parallel execution of operations can be achieved. It looks like this:
Parallel execution of operations using I / O subsystemIf
fn1
and
fn2
are computing tasks, they will be executed like this:
Performing computational tasksOne of the operations will have to wait for the completion of the second operation, since there is only one stream in Node.js.
In the case of server rendering, this problem occurs when the server process has to handle several simultaneous requests. Processing of such requests will be delayed until the requests received earlier are processed. Here's what it looks like.
Processing simultaneous requestsIn practice, request processing often consists of many asynchronous phases, even if they imply a serious computational load on the system. This can lead to an even more difficult situation with the alternation of tasks for processing such requests.
Suppose our requests consist of a chain of tasks that resembles the following:
renderPromise().then(out => formatResponsePromise(out)).then(body => res.send(body))
. When a couple of such requests arrive in the system, with a small interval between them, we can observe the following picture.
Processing requests that came with a small interval, the problem of the struggle for processor resourcesIn this case, it takes about twice as long to process each request than it takes to process an individual request. As the number of requests processed simultaneously increases, the situation becomes even worse.
In addition, one of the typical goals of an SSR implementation is the ability to use the same or very similar code on both the client and the server. The major difference between these environments is that the client environment is essentially the environment in which one client works, and the server environment, by its nature, is a multi-client environment. What works well on the client, such as singletons or other approaches to storing the global state of the application, leads to errors, data leaks, and, in general, to confusion, while simultaneously processing multiple requests to the server.
These features become problems in a situation where you need to simultaneously handle multiple requests. Everything usually works quite normally under lower loads in a cozy environment of the development environment, which is used by one client represented by a programmer.
This leads to a situation that is very different from the classic examples of Node.js applications. It should be noted that we use the JavaScript runtime for the rich set of libraries available in it, and because it is supported by browsers, and not for its model of simultaneous data processing. In this application, the asynchronous model of simultaneous data processing demonstrates all its shortcomings, which are not compensated by advantages, which are either very few or not at all.
Lessons from the Hypernova project
Our new rendering service, Hyperloop, will be the main service with which users of the Airbnb website will interact. As a result, its reliability and performance play a crucial role in ensuring the convenience of working with the resource. By implementing Hyperloop in production, we take into account the experience that we gained while working with our earlier server rendering system -
Hypernova .
Hypernova does not work like our new service. This is a pure rendering system. It is called from our monolithic Rail service, called Monorail, and returns only HTML fragments for specific rendered components. In many cases, such a “fragment” represents the lion’s share of a page, and Rails provides only a page layout. With legacy technology, parts of the page can be linked together using ERB. In any case, however, Hypernova does not load any data needed to form a page. This is a Rails task.
Thus, Hyperloop and Hypernova have similar performance characteristics related to computing. At the same time, Hypernova, as a production service that processes significant amounts of traffic, provides a good field for testing, leading to an understanding of how the Hypernova replacement will behave in combat conditions.
Hypernova work patternThis is how Hypernova works. User requests come to our main Rails application, Monorail, which collects the properties of React components that need to be displayed on a page and makes a request to Hypernova, passing these properties and component names. Hypernova renders the components with properties in order to generate the HTML code that needs to be returned to the Monorail application, which then inserts this code into the page template and sends it all back to the client.
Sending the finished page to the clientIn the event of an abnormal situation (this may be an error or a response time out) in Hypernova, there is a fallback option, using which components and their properties are embedded in the page without HTML generated on the server, after which it is sent to the client and rendered there hopefully successful. This led us to not considering Hypernova as a critical part of the system. As a result, we could allow the occurrence of a certain number of failures and situations in which timeout is triggered. Adjusting the request timeouts, we, based on observations, set them at about the level of P95. As a result, it is not surprising that the system worked with a basic timeout rate of less than 5%.
In situations of traffic reaching peak values, we could see that up to 40% of requests to Hypernova are closed by timeouts in Monorail. On the Hypernova side, we have seen
BadRequestError: Request aborted
lesser height. These errors, in addition, existed in normal conditions, while in normal operation, due to the architecture of the solution, the other errors were not particularly noticeable.
Peak values ​​of timeouts (red lines)Since our system could work without Hypernova, we didn’t pay much attention to these features, they were perceived rather as annoying little things, and not as serious problems. We explained these problems by the features of the platform, by the fact that the launch of the application is slow due to the rather heavy initial garbage collection operation, due to the peculiarities of compiling the code and caching data, and for other reasons. We hoped that the new React or Node releases would include performance improvements that would mitigate the disadvantages of slow service startup.
I suspected that what was happening was very likely the result of poor load balancing or a consequence of problems in the deployment of solutions when increasing delays were manifested due to excessive computational load on the processes. I added an auxiliary layer to the system to log information about the number of requests processed simultaneously by separate processes, as well as to record cases in which the process received more than one request.
Research resultsWe considered the delay of the service to be the culprit for the delays, and in fact the problem was caused by parallel requests competing for CPU time. According to the measurement results, it turned out that the time spent by the request while waiting for the completion of processing other requests corresponds to the time spent processing the request. In addition, this meant that the increase in delays due to simultaneous processing of requests looks the same as an increase in delays due to an increase in the computational complexity of the code, which leads to an increase in the load on the system when processing each request.
This, moreover, made it more obvious that the
BadRequestError: Request aborted
could not be confidently explained by the slow launch of the system. The error was based on the request body parsing code, and occurred when the client canceled the request before the server was able to read the request body completely. The client stopped working, closed the connection, depriving us of the data that is needed in order to continue processing the request. It’s much more likely that this was due to the fact that we started processing the request, after this the event loop turned out to be blocked by rendering for another request, and then we returned to the interrupted task in order to complete it, but the result was that the client , who sent us this request, has already disconnected, interrupting the request. In addition, the data transmitted in requests to Hypernova were quite voluminous, on average, in the region of several hundred kilobytes, and this, of course, did not contribute to the improvement of the situation.

Error caused by disconnecting the client that did not wait for an answerWe decided to deal with this problem, using a couple of standard tools, in which we had a lot of experience. This is a reverse proxy server (
nginx ) and a load balancer (
HAProxy ).
Reverse proxying and load balancing
In order to take advantage of the multi-core processor architecture, we run several Hypernova processes using the built-in module Node.js
cluster . Since these processes are independent, we can simultaneously handle simultaneously incoming requests.
Parallel processing of requests coming at the same timeThe problem here is that each Node process is fully occupied all the time that lasts for processing a single request, including reading the request body sent from the client (in this case Monorail plays its role). Although we can read multiple requests in parallel in a single process, this, when it comes to rendering, leads to alternation of computational operations.
Node process resource utilization is tied to client and network speed.
As a solution to this problem, consider a buffering reverse proxy server that will allow you to maintain communication sessions with clients. The inspiration for this idea was the unicorn web server that we use for our Rails applications.
The principles declared by unicorn perfectly explain why this is so. For this purpose we used nginx. Nginx reads the request coming from the client to the buffer, and sends the request to the Node server only after it has been completely read. This data transfer session runs on a local machine, through a loopback interface, or using Unix domain sockets, and this is much faster and more reliable than transferring data between individual computers.
Nginx buffers requests and then sends them to the Node serverDue to the fact that nginx is now engaged in reading requests, we were able to achieve a more uniform load of Node processes.
Uniform loading of processes through the use of nginxIn addition, we used nginx to process some requests that do not require access to Node processes. The discovery and routing layer of our service uses low-load
/ping
requests to verify communication between hosts. Processing all of this in nginx eliminates a significant source of additional (albeit small) load on Node.js processes.
The following improvement concerns load balancing. We need to make thoughtful decisions about the distribution of requests between Node-processes. The
cluster
module distributes requests in accordance with the round-robin algorithm, in most cases with attempts to bypass processes that do not respond to requests. With this approach, each process receives a request in turn.
The
cluster
module distributes connections, not requests, so all this does not work as we need. The situation gets worse when using permanent connections. Any persistent connection from a client is tied to a single specific workflow, which complicates the efficient distribution of tasks.
The round-robin algorithm is good when there is a low variability in request delays. For example, in the situation illustrated below.
Algorithm round-robin and connections for which requests are stably receivedThis algorithm is no longer so good when it is necessary to process requests of different types, for the processing of which you may need completely different time costs. The most recent request sent to a certain process is forced to wait for the processing of all requests sent earlier, even if there is another process that has the ability to process such a request.
Uneven process loadIf you distribute the requests shown above more rationally, you get something like the one shown in the figure below.
Rational distribution of requests by threadsWith this approach, waiting is minimized and it becomes possible to send responses to requests faster.
You can achieve this by placing requests in a queue, and assigning them to a process only when it is not busy processing another request. For this purpose we use HAProxy.
HAProxy and process load balancingWhen we used HAProxy for load balancing on Hypernova, we completely eliminated timeout peaks, as well as
BadRequestErrors
errors.
Simultaneous requests were also the main cause of delays during normal operation; this approach reduced such delays. One of the consequences of this is that now only 2% of requests were closed by timeout, not 5%, with the same timeout settings. The fact that we managed to move from a situation with 40% of errors to a situation with timeout in 2% of cases showed that we are moving in the right direction. As a result, today our users see the website's loading screen much less often. It should be noted that the stability of the system will be of particular importance for us with the expected transition to a new system that does not have the same backup mechanism as Hypernova has.
Details about the system and its settings
In order for all this to work, you need to configure nginx, HAProxy and Node-application. Here is
an example of a similar application using nginx and HAProxy, by analyzing which, you can understand the device of the system in question. This example is based on the system that we use in production, but it is simplified and modified so that it can be performed in the foreground on behalf of an unprivileged user. In production, everything should be configured using some kind of supervisor (we use runit, or, increasingly, kubernetes).
The nginx configuration is fairly standard, using the server listening on port 9000, configured to proxy requests to the HAProxy server, which listens on port 9001 (in our configuration, we use Unix domain sockets).
In addition, this server intercepts requests to the endpoint
/ping
to directly service requests directed at verifying network connectivity. nginx ,
worker_processes
1, nginx — HAProxy Node-. , , , Hypernova, ( ). .
Node.js
cluster
. HAProxy,
cluster
, .
pool-hall . — , , ,
cluster
, .
pool-hall
, .
HAProxy , 9001 , 9002 9005. —
maxconn 1
, . . HAProxy ( 8999).
HAProxyHAProxy . ,
maxconn
.
static-rr
(static round-robin), , , . , round-robin, , , , , . , , . .
, , . ( ). , , , , . , , .
HAProxy
HAProxy. , , , . , , ( ) . , ,
cluster
. , .
ab
(Apache Benchmark) 10000 . - . :
ab -l -c <CONCURRENCY> -n 10000 http:
15 4- -,
ab
, . (
concurrency=5
), (
concurrency=13
), , (
concurrency=20
). , .
, -, . , . , , , , . , , , .
, — .
maxconn 1
, , .
HTTP TCP , , , . ,
maxconn
, . , , (, , ).
, , , , , , .
— , .
option redispatch
retries 3
, , , , , , . .
, - , . , . , , . 100 , 10 , , . , . ,
accept
.
, (
backlog ) , . SYN-ACK (
, , , ACK ). , , , , .
, , , , . , , 1.
maxconn
. 0 , , , , , . , . - , , .
abortonclose
, . ,
abortonclose
. nginx.
, , . ( ) , , , , , . HAProxy , , ( ). , , , HTML. , , . , , ( , , ). , , . , , , . HAProxy, MAINT HAProxy.
, , ,
server.close
Node.js , HAProxy , , , . , , , , , .
, ,
balance first
, (
worker1
) 15% , , ,
balance static-rr
. , «» . . (12 ), , , - . , , , «» «». .
, , Node
server.maxconnections
, ( , ), , , , . ,
maxconnection
, , , . JavaScript, ( ). , , , . , , , HAProxy Node , . , , .
, , , ,
.
Results
Node.js . , , , -. Node.js . , , , , , , , nginx HAProxy.
, Airbnb , Node.js .
Dear readers! Do you use server rendering in your projects?