The developers at Google Brain have proven that "conflicting" images can hold both a person and a computer; and the possible consequences are frightening.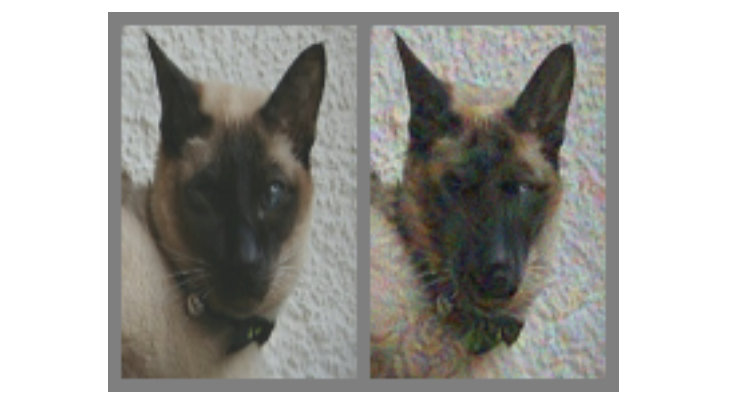
In the picture above - on the left, without a doubt, the cat. But can you say unequivocally whether the cat is on the right, or just a dog that looks like him? The difference between them is that the right one is made using a special algorithm that does not give to computer models called “convolutional neural networks” (CNN, convolutional neural network, hereafter SNS) to conclude unambiguously that in the picture. In this case, the SNS believe that this is more a dog than a cat, but most interestingly, most people think the same way.
This is an example of what is called a “picture-contradiction” (hereinafter referred to as the Carp): it has been specifically modified to deceive the SNA and prevent the contents from being correctly determined. Researchers at Google Brain wanted to understand whether it is possible to force biological neural networks in our heads to fail in the same way, and eventually created options that affect both machines and people equally, causing them to think that they are looking at something not really.
')
What are "conflicting images"?
Almost everywhere, recognition algorithms are used for recognition in the SNA. By “showing” the program a large number of different illustrations with pandas, you can train her to recognize pandas, as she learns by comparison in order to identify a common feature for the entire set. As soon as the SNS (also called
“classifiers” ) gathers a sufficient array of “panda signs” on the training data, it will be able to recognize the panda in any new pictures that will be provided to it.
We recognize pandas by their abstract characteristics: small black ears, large white heads, black eyes, fur, and all that jazz. SNA acts differently, which is not surprising, since the amount of information about the environment that people interpret every minute is much more. Therefore, taking into account the specifics of the models, it is possible to influence the images in such a way as to make them “contradictory” by mixing with carefully calculated data, after which the result will look almost like the original, but completely different for the
classifier , which will start to make mistakes when trying to determine content.
Here is an example with a panda:
An image of a panda combined with indignation can convince the classifier that this is actually a gibbon.Source: OpenAIThe classifier based on the SNC is sure that the panda is on the left, by about 60%. But if you slightly add (“create indignation”) source by adding what looks like a chaotic noise, the same classifier will be 99.3 percent sure that now he is looking at a gibbon. Small changes, which even cannot be clearly seen, give rise to a very successful attack, but it will only work on a specific computer model and will not conduct those that could be “taught” on something else.
To create content that causes an inappropriate response from a large and heterogeneous number of artificial analysts, you must act rougher — tiny corrections will not affect. What worked reliably could not be done with “small means”. In other words, if you want to get content that works from any angles and distances, you will have to intervene substantially, or as a person would say, more obvious.
In the sight - a man
Here are two examples of coarse carp where a person can easily find a hindrance.
Source: Open AI on the left, Google Brain on the rightThe picture of the cat on the left, which is defined as a computer SNS, made with the help of "broken geometry." If you take a closer look (or even not too close), it will be seen that several angular and box-shaped structures are planned, which may resemble the outlines of a system unit. And the image of the banana on the right, which is recognized as a toaster, consistently gives a false positive from any point of view. People will find a banana here, but the strange thing next to it has some signs of a toaster - and this fools technique.
When you make a guaranteed suitable “controversial” image that needs to beat a whole company of discriminating models, very often this leads to the appearance of a “human factor”. In other words, what will confuse a single neural network may not be perceived as a problem by a human, and when you try to get a rebus that is definitely suitable for cheating five or ten at once, it turns out that it works on the basis of the mechanisms that people are completely useless.
As a result, there’s absolutely no need to try to make a person think that an angular cat is a computer case, and the sum of a banana and a strange daub looks like a toaster. Much better when creating a carp designed for us with you, immediately focus on the use of models that perceive the world in the same way as people.
Cheating on the eye (and the brain)
SNS with deep learning and human vision are somewhat similar, but in essence, the neural network "looks" at things "computer-like." For example, when she is fed a picture, she “sees” a static grid of rectangular pixels at the same time. The eye works differently, a person perceives high detail in the sector about five degrees to each side of the line of sight, but outside of this zone attention to detail is linearly reduced.
Thus, unlike a car, for example, blurring the edges of an image will not work with a person and will simply go unnoticed. The researchers were able to model this feature by adding a “retinal layer” that changed the data supplied by the SNA, as they would look to the eye, in order to limit the neural network to the same framework in which ordinary vision works.
It should be noted that a person copes with his own lack of perception by the fact that the glance is not directed at one point, but is constantly moving, viewing the image as a whole, but it was also possible to compensate for the conditions of the experiment, leveling the differences between the SNA and people.
Note from the work itself:
Each experience began with an installation crosshair, which appeared in the center of the screen for 500-1000 milliseconds, and each subject was instructed to fix a glance at the crosshairs.The use of the “retinal layer” was the last step that had to be taken as part of the “fine adjustment” of machine learning to “human features”. When generating samples, they were driven through ten different models, each of which had to unambiguously name, say, a cat, for example, a dog. If the result was “10 out of 10 were wrong”, then the material was transmitted for human testing.
Does it work?
The experiment involved three groups of pictures: "pets" (cats and dogs), "vegetables" (zucchini and broccoli) and "threats" (spiders and snakes, although as a snake host I would suggest another term for evaluation). For each group, success was counted, if the test was chosen incorrectly - he called the dog a cat, and vice versa. Participants sat in front of the monitor, which displayed the image for about 60 or 70 milliseconds, and they had to press one of the two buttons denoting the object. Since the image was shown for a very short time, this smoothed out the difference between how people perceive the world and neural networks; the illustration in the title, by the way, is striking in its persistence of error.
What was shown to the subjects could be an unmodified image (image), a “normal” carp (adv), a “flipped” (flip) carp on which the noise was turned upside down before overlaying, or a “spurious” carp on which the layer noise was applied to a picture that does not belong to any of the types in the group (false). The last two options were used to control the nature of the perturbation (will the noise structure affect otherwise upside down, or just “is-not”?), Plus it was given an opportunity to understand whether the interference really deceive people or just slightly reduce accuracy.
Note from the work itself:
“False”: a condition was added to force the subject to make a mistake. We added it, because if the initial changes reduce the accuracy of the observer, this can occur due to a decrease in the direct image quality. In order to show that the carp really works in every class, we introduced the options where no choice could be correct and their accuracy was 0, and watched what kind of “correct” answer was in that case. We demonstrated arbitrary images from ImageNet, to which the impact was applied from one or another class in the group, but not suitable for any of them. The participant in the experiment was to determine what was in front of him. For example, we could show the picture of the aircraft, distorted by the imposition of "dog" noise, although during the experience the subject had to learn only a cat or a dog.Here is an example showing, as a percentage, the number of people who were able to clearly define the picture as a dog, depending on how the noise was used. Let me remind you, it was only 60-70 milliseconds to take a look and make a decision.
Source: Google Brain
Original picture with a dog; Carp with a dog, adopted by both a man and a computer for a cat; control picture with a layer of noise turned upside down.But the final results:
Source: Google Brain
The results of the study, how true people identify these pictures with comparison with distorted ones.The diagram shows the accuracy of the match. If you choose a cat and it really is a cat, accuracy increases. If you choose a cat, but this is actually a dog, transformed by noise into a kind of cat, the accuracy decreases.
As you can see, people are much more correct in a sample of unadjusted images or with inverted noise layers than in a sample of "contradictory". This proves that the principle of attack on perception can be transferred from computers to us.
Not only are the effects indisputably effective, they are also thinner than expected - no box or pseudo-testers, or anything like that. Since we have seen both the layers with noise, and the images before and after processing, it is necessary to figure out exactly what confuses us in this. Although the researchers are cautious, stating that "our examples are specially made to fool the head, so you should be careful using people as experimental subjects to study the effect."
The team will try in the future to derive some general rules for certain categories of modification, including "
breaking the edges of an object , especially by moderate effects, perpendicular to the edge line;
correcting the bordering areas by increasing the contrast while texturing the border;
changing the texture ;
using dark parts images in which the level of impact on perception is high even in spite of tiny disturbances. " Below are examples of red circled areas where the described methods are best seen.
Source: Google Brain
Examples of pictures with different principles of distortionWhat is the result?
The bottom line is that this is more, much more than just a clever trick. The guys from Google Brain have confirmed that they can create an effective technique of deceiving perception, but they do not fully understand why it works, taking into account the level of abstraction, and it is possible that this is literally the basic level of reality:
Our project raises fundamental questions about how carp affects, how neural networks and the brain work. Did you manage to transfer attacks from the SNA to the brain because the semantic representations of information in them are similar? Or because both of these ideas correspond to some general semantic model that naturally exists in the outside world?
In conclusion, if you really want to screw up a little, the researchers will be happy to do you a favor, pointing out that “visual recognition of objects ... is difficult to give an objective assessment. Is the "Fig.1" true objective dog, or is it objectively a cat that can make people think that it is a dog? "In other words, does the picture really turn into a subject, or does it just make you think differently?
Eerie here is that (and I seriously say “eerie”) that in the end you can get ways to influence any facts, because the distance between manipulating SNA and manipulating a person is obviously not too large. Accordingly, machine learning technologies can potentially be used to distort pictures or videos in the right key, which will replace our perception (and the corresponding reaction), and we will not even understand what happened. From the report:
For example, a cluster of models with deep learning can be trained on people's assessments of the level of trust in certain types of people, features, and expressions. It will be possible to generate “contradictory” perturbations that will increase or decrease the feeling of “authenticity”, and such “corrected” materials can be used in news clips or political advertising.
In the long term, theoretical risks include the possibility of creating sensory stimulations that crack the brain in a huge variety of ways and with very high efficiency. As you know, many animals are seen in vulnerability to over-threshold stimulations. For example, cuckoos can at the same time pretend to be helpless and issue a plaintive cry, which, in combination, causes other breeds to feed cuckoo chicks before their own offspring. “Contradictory” patterns can be considered as such a peculiar form of superthreshold stimulation for neural networks. And it is a matter of great concern that the excessive incentives, which in theory will affect the person much more than simply force them to hang the tag “this is a cat” on the dog's picture, can be created using a machine, and then transferred to people.
Of course, such methods can also be used “for good”, and a number of options have already been proposed, such as “to sharpen the characteristic features of images in order to increase the level of concentration, for example, when monitoring the air situation or analyzing x-ray images, since this work is monotonous, and the consequences inattention can be terrible. " Also, “user interface designers can use perturbations to develop more intuitive interfaces.” Hmmm This is definitely great, but I’m somehow more worried about breaking into my brain and putting up a level of trust in people, do you understand?
Some of the questions posed will be the subject of future research - perhaps it will become clear what exactly makes specific pictures more suitable to pass the error to the person, and this may provide new clues for understanding the principles of the brain. And this, in turn, will help create more advanced neural networks that will learn faster and better. But we should be careful and remember that, like computers, we are sometimes not so difficult to deceive.
The project
" Adversarial Examples of Human and Computer Vision" , by Gamaleldin F. Elsayed, Shreya Shankar, Brian Cheung, Nicolas Papernot, Alex Kurakin, Ian Goodfellow, and Jascha Sohl-Dickstein, from Google Brain , can be downloaded from
arXiv . And if you need more controversial pictures that work on people, then the supporting material is
here .