History of heart rhythm of a patient with atrial fibrillation. Illustration: CardiogramStill, from the "smart watch" is some practical use. If you use them with in-depth training applications and analyze the data, the medical information is really helpful.
Remember how you went to your therapist - he applied a stethoscope to his chest and listened to the work of the heart, paying attention to noises and other anomalies. In which case you could be sent to a cardiogram or ultrasound of the heart. But the problem is that in most cases he will not hear any arrhythmia, even if it is. Very often, arrhythmia appears only periodically. In reality, approximately 25% of people develop an arrhythmia throughout their lives, but most of us
will never know about it . According to
statistics , 10% of strokes occur in patients with undiagnosed atrial fibrillation.
')
Fitness bracelets and other wearable pulse-measuring gadgets are a whole new world. Imagine that your heart rate is measured constantly, every day, and in case of a problem, the doctor receives a notification. But how accurate are such measurements from an ordinary wristwatch?
To test this,
Cardiogram , the developer of the relevant mobile application, in collaboration with the University of California at San Francisco Cardiology Department,
conducted a large-scale study of mRhythm .
First, a neural network was developed that was taught to recognize signs of atrial fibrillation in the history of the collected data - the most common type of cardiac arrhythmia. The neural network architecture is shown in the illustration.
The data from the sensors arrive in four layers of residual and convolutional neurons with a choice of maximum elements (max-pooling) after each layer. The output is sent to the four residual bidirectional LSTM layers. In the end, a single convolutional layer with a filter length of 1 generates a predictive estimate at each time step.For learning, the neural networks used data from users of the Cardiogram mobile application. They were sent 200 mobile cardiographs AliveCor. Users recorded 6338 cardiograms with a positive or negative result for atrial fibrillation. After learning, the neural network was able to calculate the forecast based on the usual statistics from Apple Watch. As a heuristic preliminary learning, the neural network also processed 139 million cardiac rhythm measurements from Cardiogram users.
After such training, the accuracy of diagnosis was higher than that of other types of diagnosis. The University of California at San Francisco presented the results of the study at the
Heart Rhythm Society Cardiology Conference.
Accuracy of recognition of atrial fibrillation was tested on 51 patients before and after cardioversion — procedures for restoring an even heart rate in patients with arrhythmia (current defibrillation or chemical methods).
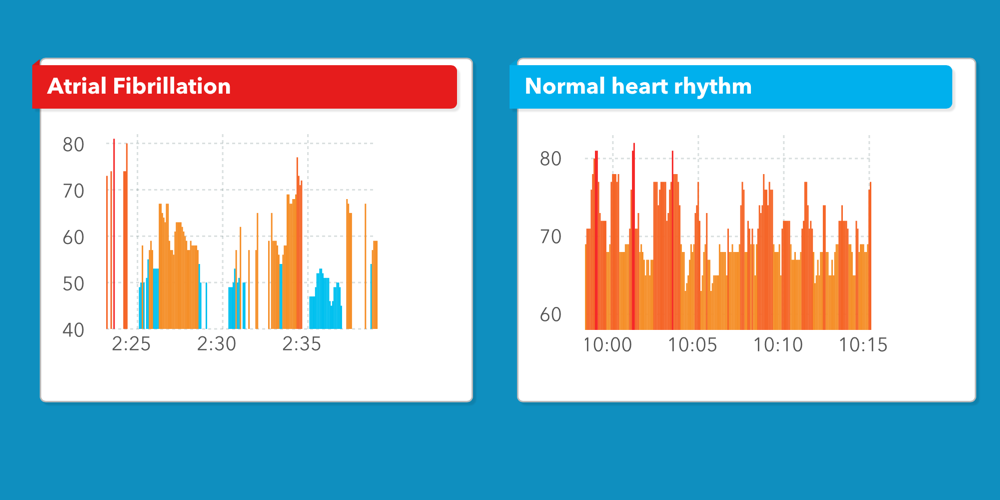
After learning the neural network on heart rate data from Apple Watch watches from the Apple Watch application, the AUC (area under the curve) was 0.97, which allowed
determining atrial fibrillation with a sensitivity of 98.04% and a specificity of 90.2% ! These are excellent performance, given the fact that Apple Watch is a simple electronic device, assembled in China, which gives out very inaccurate information, and it wasn’t close to these medical instruments. Nevertheless, even this data is sufficient for diagnosis.
"Smart watches" with the measurement of the pulse has not yet gained wide popularity, but almost everyone can afford this gadget. It is interesting in itself to collect heart rate statistics. Just watch how the indicators change, how often you enter the fourth and fifth zones relative to the maximum pulse - and how quickly the normal heart rate is restored after that.
Driving at rush hour, being late for an important meeting at 16:00. Illustration: Cardiogram
Crazy training. Illustration: Cardiogram
The game of tennis. Illustration: CardiogramData mining of heart rate statistics is not the only application of neural networks in medicine. In December 2016, a team of Google researchers trained the neural network to identify diabetic retinopathy (one of the most severe complications of diabetes when the retinal vessels are affected)
with greater accuracy than ophthalmologists . A month later, researchers at Stanford University published a scientific article in
Nature about how a neural network detects cases of skin cancer from photographs of lesions.
Detection of atrial fibrillation is another important achievement of in-depth training as applied to medicine. Specialists from Cardiogram are confident that in addition to atrial fibrillation, the neural network can detect other heart diseases according to data collected from smart watches. The only question is learning. A self-study option with reinforcements is also considered, when users press a button, signaling a panic attack, for example. Over time, the neural network will highlight the signs of a panic attack and will be able to detect it on its own.