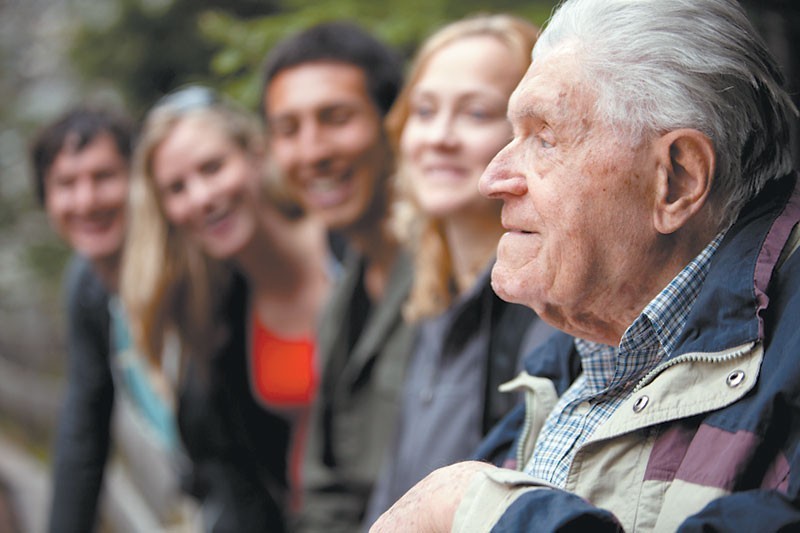
Today Alzheimer's disease is one of the most insidious diseases, it is very difficult (and expensive) to predict its occurrence. And although an already developed disease cannot be stopped, there is evidence that early detection helps to slow or stop Alzheimer's disease and brain degradation. Therefore, the search for a reliable way to determine the risk of developing the disease is occupied by the minds of researchers.
As the human body ages, cognitive impairment is inevitable. With age, people become more forgetful, more often lose their train of thought, and find it difficult to make decisions or perform tasks that previously did not cause difficulties. Doctors call this a mild cognitive impairment. It affects most people when they get older.
')
Many people with mild cognitive impairment develop a more severe form - Alzheimer's disease. A person loses vocabulary, often uses wrong word replacements, ceases to recognize close relatives, loses basic self-care skills, and ultimately becomes completely dependent on other people who help him. Most people with this diagnosis die within a few years after the discovery of Alzheimer's disease.
Interestingly, such a scenario is not waiting for all people with mild cognitive impairment. Over time, the patient's condition may not deteriorate, and in some cases even improve. Therefore, doctors want to find ways to identify those who are more likely to develop Alzheimer's disease.
South Korean scientists have proposed to use for this purpose in-depth training. The technology they have developed can accurately identify people who may develop Alzheimer's in the next three years.
The deep neural network learns to recognize unique traces of the disease in images of
positron emission tomography of the brain (PET). Alzheimer's disease is known to be characterized by undesirable growth of protein lumps, called amyloid plaques, and slow brain metabolism, which is measured by the rate at which the brain uses glucose.
Certain types of PET scans can detect signs of both of these conditions, and therefore they can be used to identify people with mild cognitive impairment, which ultimately lead to the development of Alzheimer's disease.
In theory, it sounds very encouraging, but in practice it is rather difficult to interpret the resulting images. The researchers found one or two bright markers that can be found by specially trained people, but this method is time consuming and is not immune from errors. Therefore, Korean scientists decided to replace people with a deep neural network.
In recent years, Alzheimer's researchers around the world have created a database of brain images of patients with and without Alzheimer's disease. And South Korean colleagues used this dataset to train a convolutional neural network to recognize the difference between them.

(A) - The convolutional neural network architecture is applied to two PET images made using a biological glucose analogue,
fluorodeoxyglucose and
floorbetapyra , substances administered to a patient to diagnose Alzheimer's disease. Each layer, function can be extracted using the three-dimensional function of convolution and activation (ReLU). Multilayer convolutions provide a one-dimensional output, and the last layer has two nodes that correspond to Alzheimer's disease (AD) and the normal state of the brain (NC).
(B) - Depth neural network was trained according to PET data from healthy and sick patients. Researchers used tenfold cross-validation. After training, the AI was directly used for classification between transforming into a disease or not transforming mild cognitive impairment (MCI). The researchers assessed the accuracy of the prediction for patients with a disorder that could lead to illness. In addition, scientists also performed an analysis of the receiver’s performance characteristics (ROC).
The data set consisted of images of the brain of 182 people aged 70 years with a healthy brain and 139 brain images of people of about the same age who were diagnosed with Alzheimer. As a result, the AI was able to recognize the difference between a healthy and diseased brain with an accuracy of 90%.
In addition, the researchers used their machine to analyze a different set of data, which consisted of brain scans of 181 people aged 70 with mild cognitive impairment, 79 of whom underwent Alzheimer's disease for three years. The task assigned to scientists was to identify these people most susceptible to deterioration.
According to the results of the experiment, AI identified those who are at risk for developing Alzheimer's disease with an accuracy of 81%. This result is significantly higher than that given by doctors, visually analyzing images.
More generally, the technique of Korean scientists is just one example of the increasing use of in-depth training and machine vision in medical diagnostics. Available evidence suggests that machines can detect complex conditions earlier and more accurately than humans.
doi:
arXiv: 1704.06033