Yesterday I sketched in the article a couple of thoughts about neural networks (hereinafter referred to as NS) and had the imprudence to call it not quite neatly, which did not escape the shrewd look of my talent's admirers, who accused me that I tricked them to read my own cunning and loud headline. masterpiece. Therefore, in order to compensate for the bitterness from the unfulfilled expectations in this article, I present to the court a strict but fair public reflection on some areas of the application of the National Assembly.
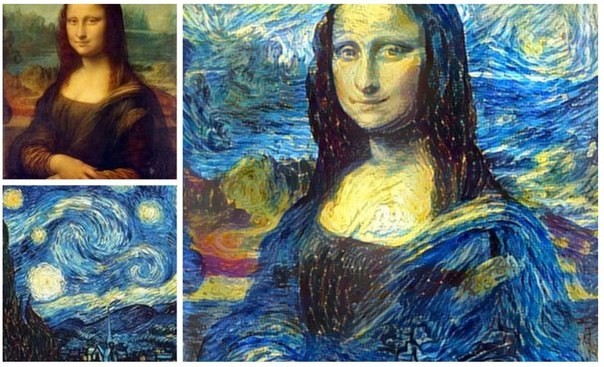
So, the scope of the NA. I think that after some time this title will also look like “areas of application of mathematics” in the sense that, firstly, the area of ​​application of mathematics is extremely wide, and secondly, it is not specified which particular subsection of mathematics is being discussed. Neural networks will be used in countless spheres, there will be many variations of their architectures and they will be able to solve all the same tasks as our brain, since they are built on a similar principle.
In the last paragraph, I did not say anything about how long they would be able to accomplish these tasks and with what characteristics of computers. Even if the performance of the National Assembly will be equal to the performance of the brain (which, according to Ray Kurzweil, a matter of decades), we still do not know how the brain works and whether its parts are not pre-trained in advance. Here I mean the “genetic memory” formed by millions of years of evolution. That is, the structure of connections and their strength (read, weights in NA) in neurons in the human brain can be set immediately at birth, and during life they are adapted to a specific environment. For example, Noam Chomsky (a famous linguist) believes that some grammar of the language (the base class of the language in terms of OOP, if you will) is already in the head at birth. But let's consider the less distant future and even the present.
')
Neural networks are related to machine learning technologies, apparently due to the fact that learning (setting parameters) on data is an integral part. Accordingly, neural networks can solve problems that relate to machine learning, namely: classification, regression, clustering. NA can also be used in learning tasks with reinforcement. Such techniques are used in decision making systems. For example, the company DeepBrain, which Google bought, taught the National Assembly to play video games. Rather, their NA learned on their own, just looking at the screen. Similar NA may drive a car. But a lot has been written about all this, but I see no point in retelling other people's thoughts.
I want to describe the fields of application of the NA, depending on what kind of data this NA is working with: numbers, images, text, sounds, speech, video.
Different network architectures work well with different data. For example, convolutional networks are used for images, recurrent networks are used for speech recognition. They are also used for natural language processing: in machine translation problems, interactive systems. For the data type “number” in problems of forecasting, approximation, regression (all this, informally speaking, it boils down to the fact that there is a certain function that is modeled using the NN), you can use the standard multilayer perceptron.
Let us limit ourselves today to the fields of application of the NA for image processing, many analogies can be drawn for other types of data.

So,
- You can use the NA to define something in the image (classification task). It can be a handwritten number, a cat, a dog, a face, or a tumor — the NA is not the same. Accordingly, it can be applied in any areas where you have images (photos) and an object of interest. Recognition of emotions, recognition of road signs, car numbers, secret objects on maps, whatever.
- NA can stylize the image. An example can be seen on KDPV. To train the National Assembly in the paintings of famous artists, and then change your photo to the chosen style. For example, Prizma does it (interestingly, will I be paid for advertising?). Something similar for the video - MSQRD, which bought Facebook. For speech, you can imagine its distortion. Well, for the sounds - the stylization of music.
- NS can search among other images of objects similar to the one that is on yours. For example, FindFace.ru. As I understand it, Natalya Yefremova works in her, who made a report on the National Assembly and whose article is on Habré.
- NA can generate new images. Write new lyrics, create music. The idea is simple and elegant: you train the NS, and then invert the output to the input.
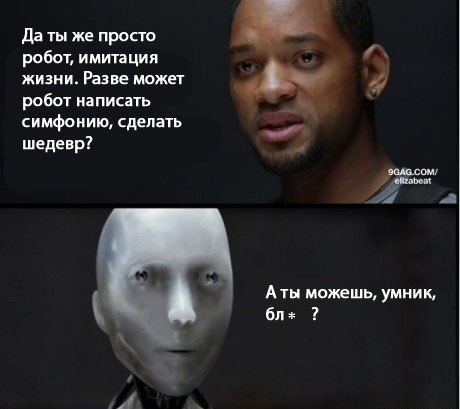
The picture is no longer so relevant.
- NA can understand what is depicted in the photo, that is, to define sematics - the meaning. So the National Assembly can not just say that the person is in the photo, but also describe the whole scene with words: the girl in the red blouse strokes the cat.
Neural networks can work as memory, they memorize signs, parts of images. This can be used for data compression or as hash functions. And speaking of hash functions, one can not but mention the use of NA in cryptography. NA can be used to encrypt data. I do not know if there are existing implementations or ideas, but I somehow thought that the National Assembly could even be used for cryptanalysis: teach it on a set of encrypted text - a plaintext, and then give it a new encrypted text and hopefully get a decryption because That it internally approximates the operation of the encryption algorithm with the key parameters.
These were some (not all) fields of application of NA.
Thanks for attention!