The 42Ark Taiwanese company and the American manufacturer of smart cat feeders CatFi Box use surveillance cameras to detect the cat's faceIn 1941, the German electrical engineer Walter Bruch installed a CCTV system (Closed Circuit Television - closed-circuit television system) at the test site where Fau-2 missiles were tested. This is the first known case of video surveillance in practice. The operator had to constantly sit in front of the monitor. This continued until 1951, until the first VTR (VideoTape Recorder) devices appeared, recording an image on a magnetic tape.
Writing to the carrier did not save the operator from the need to participate in the process. Identification of persons, determining the location of objects, even motion detection — all these functions were performed by a person sitting in front of the monitor in real time or after an after-fact study of the video archive.
')
The wheel of progress is rolling further. Video surveillance received a video clip that completely changed the process of working with the system. Remember the
story about the cat and the neural network of deep learning? Yes, this is also part of the video analytics, but tiny. Today we will talk about technologies that are fundamentally changing the world of CCTV systems.
Queue detection and beta test
The first IP camera in the world Neteye 200, created in 1996 by AxisVideo surveillance was conceived as a security closed system, designed only to address security issues. The limitations of analog video surveillance did not allow the equipment to be used in any other way. The integration of video surveillance with digital systems has opened up the possibility of automatically obtaining various data by analyzing the sequence of images.
The importance is difficult to overestimate: in the usual case, after 12 minutes of continuous observation, the operator begins to miss up to 45% of the events. And up to 95% of potentially alarming events will be missed after 22 minutes of continuous observation (according to IMS Research, 2002).
Complicated video analysis algorithms have appeared: counting visitors, counting conversions, statistics of cash transactions and much more. In this system, the observation operator disappears - we leave the computer the opportunity to "look" and draw conclusions.
The simplest example of smart video surveillance is motion detection. It is not so important whether there is a built-in detector in the camera itself - if you install, for example, the
Ivideon Server software on a computer, then the motion detector will use software. One detector is able to replace several video surveillance operators at once. And already in the 2000s, the first video analytics systems began to appear, capable of recognizing objects and events in the frame.
Ivideon is now in the development of several video analytics modules - since we
released OpenAPI , things have gone faster due to integration with partners. Some of the projects are still in closed testing, but something is already done. First of all, this is
integration with cash registers to control cash transactions (for now, based on iiko and Shtrikh-M). Secondly, a queue detector has been developed.
We had an
Ivideon Counter , which determined the number of customers in the room. Analytics allowed to move away from special equipment towards cloud computing. Now we do not need a specific camera - any surveillance camera with 1080p + resolution will do. Now we want not just to count people, but to determine the queues. Therefore, they are ready to any store, shopping center or office, where people walk and stand forming queues, to provide a free camera for the queue detection test.
Write to us to take part in the project.
In addition, Ivideon works with face recognition technology.
Who recognizes and how
DeepFace technology is tested by Facebook on the example of recognizing the emotional face of Sylvester StalloneApple, Facebook, Google, Intel, Microsoft and other technological giants are working on solutions in this area. Video surveillance complexes with automatic recognition of passengers' faces are installed in 22 US airports. In Australia, they are developing a biometric system for recognizing faces and fingerprints as part of a program designed to automate passport and customs control.

Baidu, the largest Chinese Internet company, conducted a successful experiment to refuse tickets using face recognition technology with an accuracy of 99.77%, with a shooting and recognition duration of 0.6 seconds. At the entrances to the park there are stands with tablets and special frames that shoot. When a tourist comes to the park for the first time, the system photographs it in order to use the facial recognition feature of the photo in the future. New images are compared with photos from the database - this is how the system determines whether a person has the right to visit.
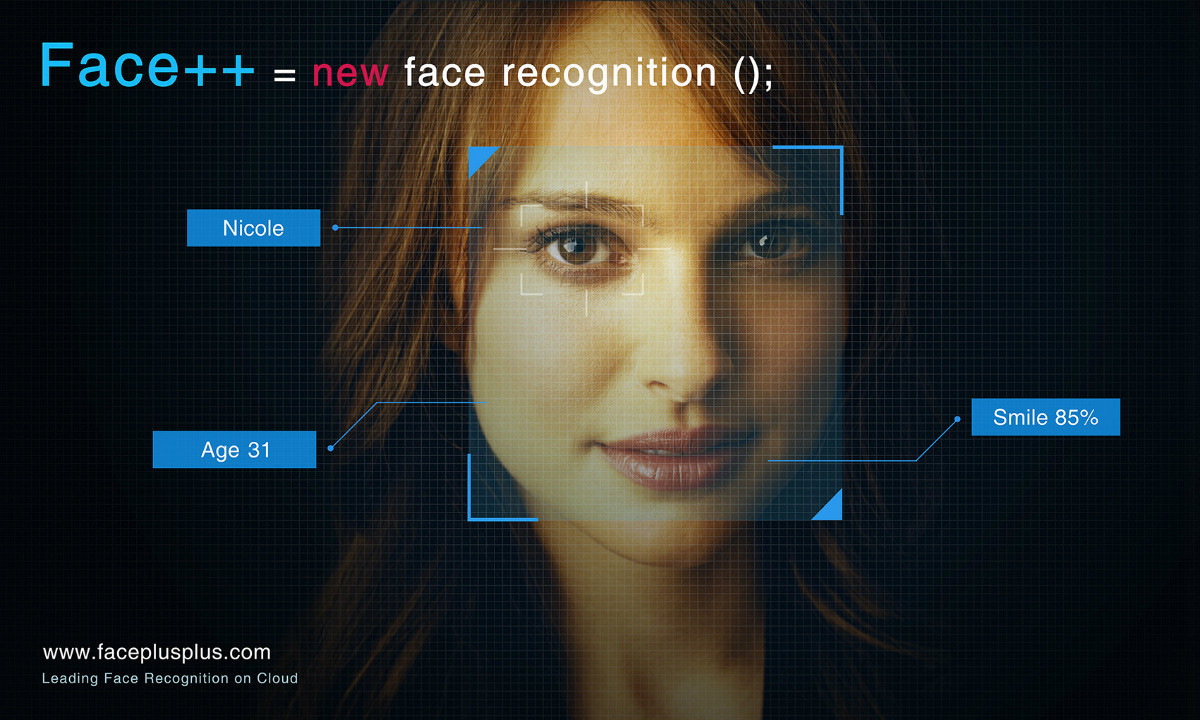
In China, technology is generally very good. In 2015, Alipay, an online payment platform operator that is part of the Alibaba holding, launched a payment verification system based on Face ++, a cloud-based face recognition platform created by Chinese startup Megvii. The system is called Smile to Pay - it allows Alipay users to pay for online purchases by shooting selfies (Alipay identifies the owner by a smile). UBER in China has begun to
use Face ++-based face recognition for drivers to counter fraud, identity theft and additional safety for passengers.
But it is more interesting to look not at foreign solutions, but at services created in Russia. These technologies are much closer to the end user (if he is from our country), you can get acquainted with them, in the future to unite for use in your own product. There are a lot of face recognition companies around. Recall a few that remain on hearing.

Vocord company, founded back in 1999, works with FaceControl 3D with synchronous images from stereo cameras, builds a 3D model of a face in a frame, and automatically searches for a match between the resulting model and models in an existing database. In 2016, Vocord
began to use its own mathematical algorithm for face recognition, which is based on convolutional neural networks, thanks to which their algorithms now work with any surveillance camera. The company claims that they can recognize the faces (in the size of 128x128 pixels) of people following in the stream. At the end of 2016, the Vocord DeepVo1 algorithm showed the best results in global identification testing, correctly
recognizing 75.127% of individuals.
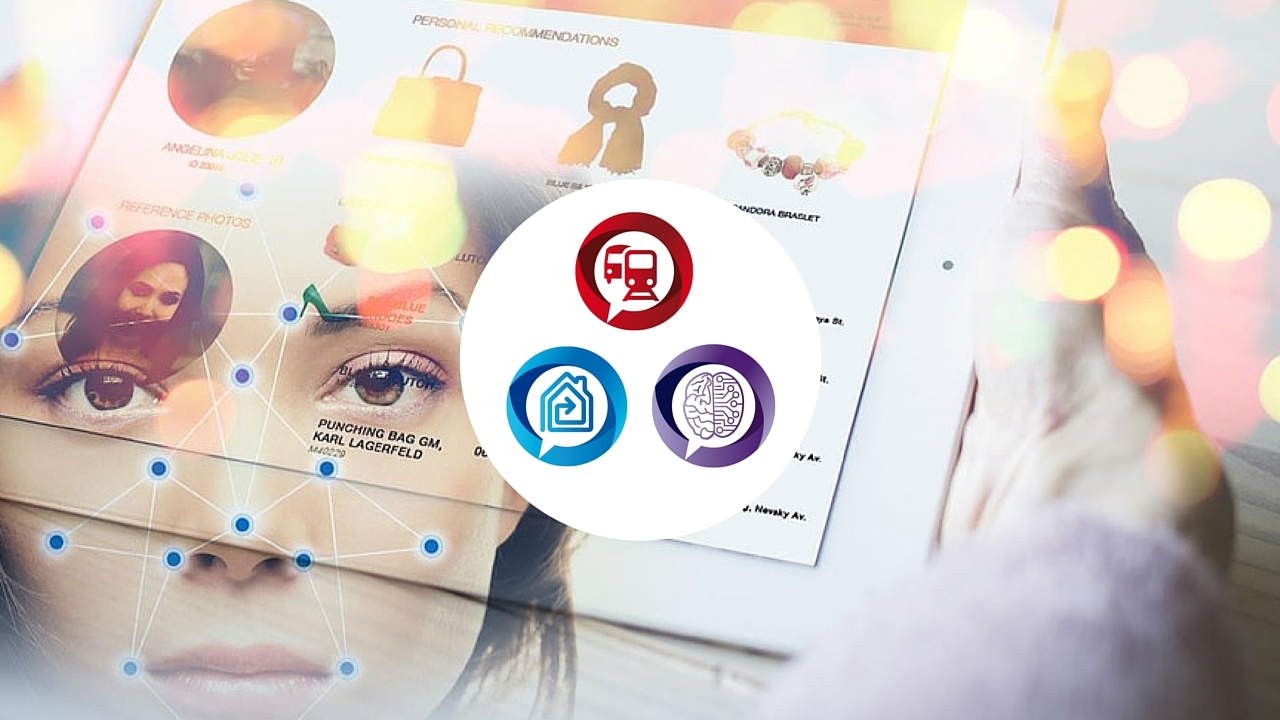
Founded in 2012, VisionLabs won the largest technological competition in Russia and Eastern Europe,
GoTech , and was included in the list of finalists for the European program
Challenge UP! », Designed to accelerate the market launch of solutions and services based on the Internet of Things concept, has attracted multimillion investments and is already introducing its products into the commercial sector. Recently, Otkrytie Bank launched VisionLabs face recognition system to optimize customer service and waiting time for customers in the queue. Well, it is worth reading a wonderful story, as the experts from CROC
caught the cat with the help of VisionLabs.
VisionLabs, which showed one of the best results in recognition and error level, also works with neural networks that reveal specific features of each face, such as eye section, nose shape, auricle relief, etc. Their system Luna allows you to find all these features of the person on the photo in the archives. Another solution of the company, Face Is, recognizing the customer’s face in the store, finds his profile in the CRM system, learns the purchase history and interests of the buyer from it, and sends a notification to the phone with a personal offer of a discount on his favorite product category.
Startup Skillaz, engaged in automating the process of hiring employees, and VisionLabs are going to
present at the end of 2017 a computer recognition system that will evaluate the behavior of job seekers when hiring. After analyzing the data, the system will draw conclusions about the professional qualities of a person and suitability for the position. The full characteristics of the machine hire system are not disclosed by the company. It is only known that the candidate’s interpersonal skills will be evaluated on the basis of his answers to a specific set of questions asked by the online-interview system. The neural network will look for the relationship of the candidate's behavior in the picture from the video surveillance camera and the degree of expression of one or another competence.
The grid, which is a doctor Lightman and Sherlock Holmes in one person, will take into account the candidate's mimicry, his gestures, as well as physiognomy. It is worth noting that the method of determining the type of a person’s personality, his spiritual qualities, based on the analysis of external facial features and his expression, in modern psychological science is considered a classic example of pseudoscience. How to cope with this contradiction in the new product is still unclear.
Slide from the presentation NTechLab, depressing Salman RadaevNTechLab started with an application that determined the breed of dogs from a photo. Later, they wrote a FaceN algorithm, with which they participated in the international contest
The MegaFace Benchmark in the fall of 2015. NTechLab won in two nominations out of four, bypassing the Google team (after a year, Vocord will win in the same competition, and NTechLab will shift to the 4th position). Success allowed them to quickly implement the
FindFace service, searching for people from photos on VKontakte. But this is not the only way to apply technology. At the Alfa Future People festival organized by Alfa-Bank, with the help of FindFace, visitors could find their photos among hundreds of others by sending a selfie to a chat bot.
In addition, NTechLab
showed a system capable of real-time recognition of gender, age and emotions, using an image from a video camera. The system is able to assess the audience's response in real time, so that you can determine the emotions that visitors experience during presentations or broadcasts of advertising messages. All NTechLab projects are built on self-learning neural networks.
Ivideon's path to video analytics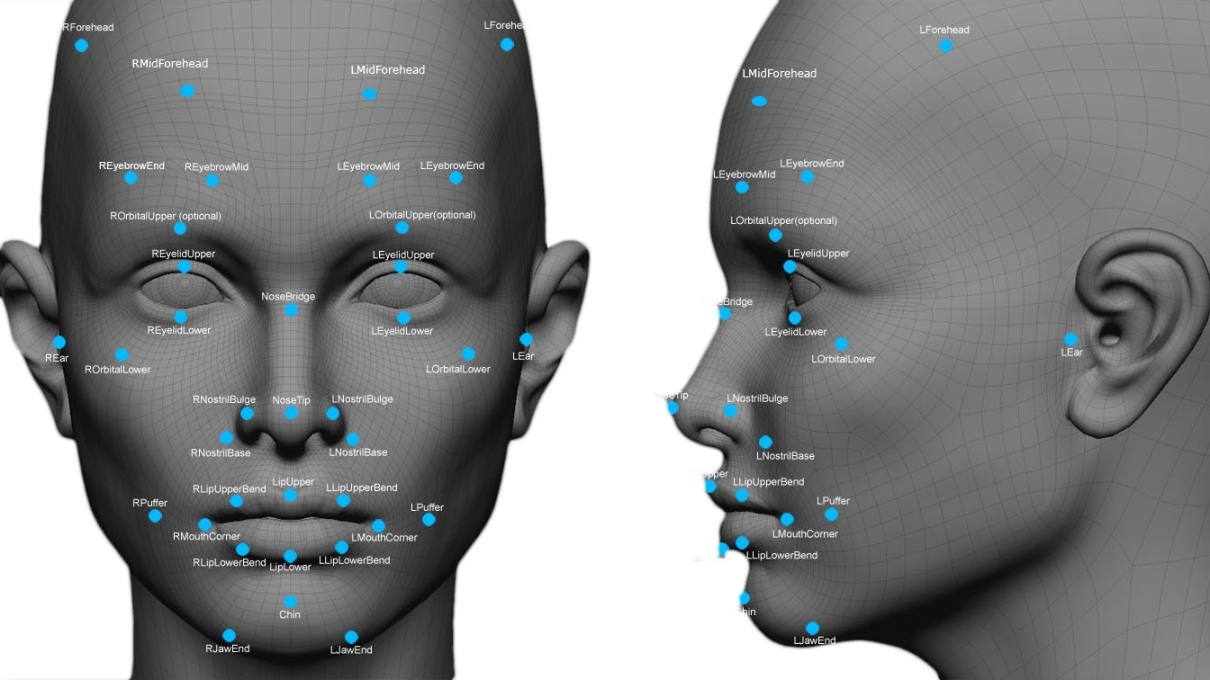
Face recognition is one of the most difficult tasks in the field of video analytics. On the one hand, everything seems to be clear and has been used for a long time. On the other hand, identification solutions in a crowd of people are still very expensive and do not provide absolute accuracy.
In 2012, Ivideon began working with video analysis algorithms. That year we launched applications for iOS and Android, entered foreign markets, launched decentralized CDN networks with servers in the USA, the Netherlands, Germany, Korea, Russia, Ukraine, Kazakhstan and became the only international video surveillance service that works equally well all over the world. In general, it seemed that making analytics with blackjack and recognition would be simple and fast ... we were young, the grass seemed greener, and the air was sweet and weary.
[
At that time, we considered the classical algorithms. First you need to detect and localize the faces in the image: use the Haar cascades , search for regions with a skin-like texture, etc. Suppose we need to find the first person and accompany only him in the video stream. Here you can use the algorithm of Lucas-Canada . We find the face by the algorithm and then define the characteristic points in it. We accompany the points with the help of the algorithm of Lucas-Canada; after their disappearance we believe that the face has disappeared from sight. Having received the characteristic features of the face, we will be able to compare it with the features embedded in the database.
To smooth the trajectory of the object (person), as well as to predict its position in the next frame, we use the Kalman filter . Here it should be noted that the Kalman filter is designed for linear motion models. For nonlinear, the Particle Filter algorithm is used (as an option Particle Filter + Mean Shift algorithm).
You can also use the background subtraction algorithms: a library with examples of the implementation of the algorithms for subtracting the background + an article on the implementation of the light background subtraction algorithm ViBe. In addition, do not forget one of the most common methods of Viola-Jones , implemented in the library of computer vision OpenCV. ]
Simple face recognition is good, but not enough. It is also necessary to ensure stable tracking of several objects in the frame, even if they jointly intersect or temporarily “disappear” behind the obstacle. Consider any number of objects crossing a certain zone and take into account the directions of intersection. To know when an object / object appears and disappears in the frame - hover the mouse on the dirty cup on the table and find the moment in the video archive, when it appeared there and who left it. In the process of tracking an object can change quite strongly (in terms of transformations). But from frame to frame, these changes will be such that it will be possible to identify the object.
In addition, we wanted to make a universal cloud solution accessible to all - from the most demanding users. The solution had to be flexible and scalable, because we ourselves could not know what the user wants to follow and what the user wants to consider. It is possible that someone would have been supposed to make a cockroach run with automatic determination of the winner on the basis of Ivideon.
Only five years later we started testing individual components of video analytics - we will tell you more about these projects in new articles.
PS So, we are looking for volunteers for queuing detector tests. As well as users of the SHTRIH-M system for the test of a new system of control of cash transactions. Write to the
mail or in the comments.