The heads-up (one-on-one) no-limit hold'em pre-flop and flop decision treeThe pioneer of modern game theory, John von Neumann, said: “Real life is all about bluffing, little tricks of deception, thinking about what the other person expects from you. That's what the game is in my theory "(quote from the 13th series of the documentary series"
The Rise of Humanity ").
In other words, John von Neumann foresaw that in order to create a strong AI, a computer must learn to play games with incomplete information that most closely match human behavior in real life. Games like poker.
Board games are a traditional area of experimentation in the field of artificial intelligence. Every year, the AI plays people in different games. At first, checkers surrendered, then chess, then Atari video games, the last of which was the game of go. But all these are games with complete information, in which all players have complete information about the state of the game. Poker is a completely different matter.
')
Scientists have long been trying to develop a program that could beat a person in no-limit Texas Holdem. Unlike other applications of weak AI, here successful development will pay off instantly, because every day in online poker rooms they play banks for billions of dollars.
John von Neumann said that poker delights him, and this is not surprising, given the unique features of this game with incomplete information. Each player has only a part of the information about the state of the game - and he acts on the basis of this partial information, as well as evaluating the actions of other players.
Previously, the AI achieved some success only when playing
limit hold'em, the most primitive version of the game with a limited step of raising stakes. In the limit option, the player has only 10
14 development options. For comparison, in the no-limit hold'em of such options already 10
160 . By the way, in the game of development options
10,170 , but there is a game with complete information, that is, a fundamentally simpler task.
Games with incomplete information require a completely more complex level of recursive thinking than games with complete information. Here, the correct action of the AI depends, among other things, on the information that the AI received from the actions of the opponent. But the information that the opponent issued, in turn, is a derivative of the previous AI actions and the information that the AI gave to the opponent by their actions. This is the recursive thinking that the DeepStack program deals with. And she copes very well, judging by the results of games with professionals (see table).
Heads-up program results with professional playersThe architecture of the DeepStack program is shown in the illustration. The program overestimates its actions at every stage when decision-making is required of it. To calculate the value of each bet, the foresight tree (lookahead tree) is used, the values for the colors of which are calculated using a neural network previously trained in random game situations.
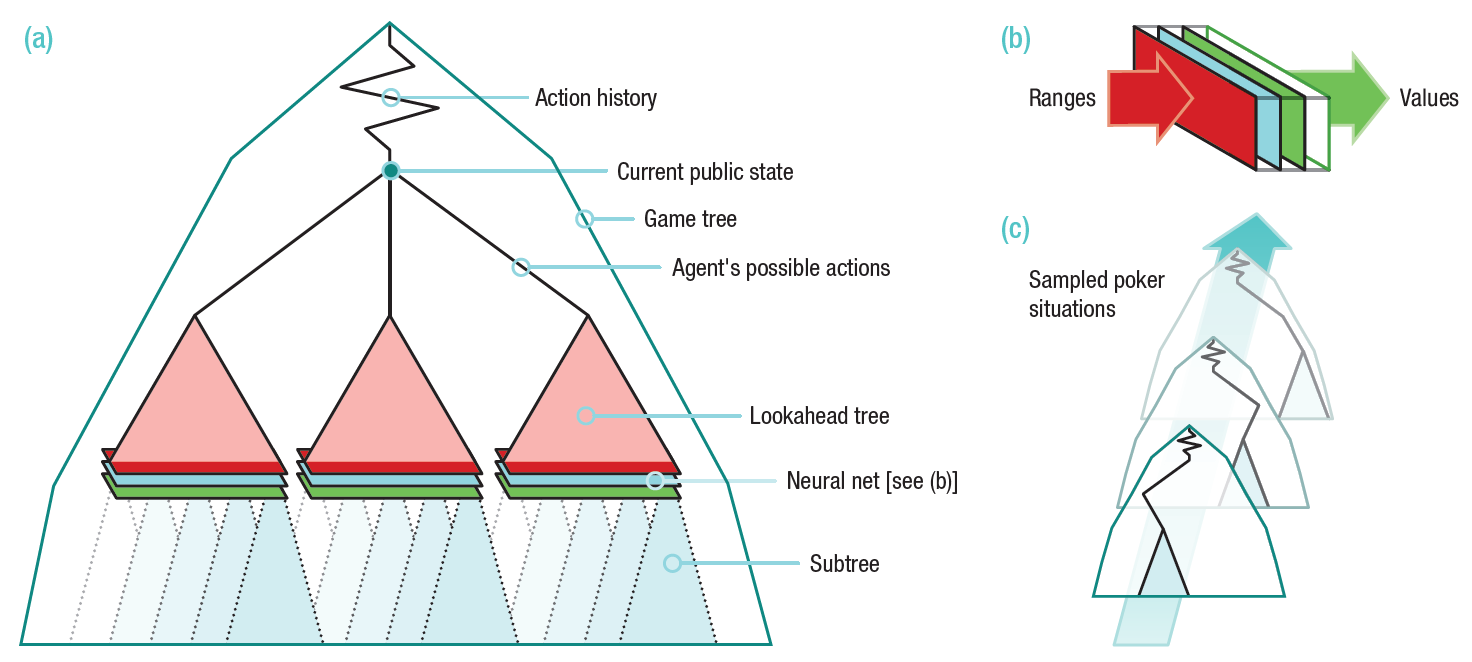
The structure of the neural network demonstrates that the bank size, open cards and player ranges (possible combinations with which a player could enter the game in the way he entered it (call, raise, 3-bet, etc.) served at the entrance, probability of each combination). The neural network consists of seven fully connected hidden layers. The output values are then processed by another neural network, which checks that the actions satisfy the
zero-sum limit.
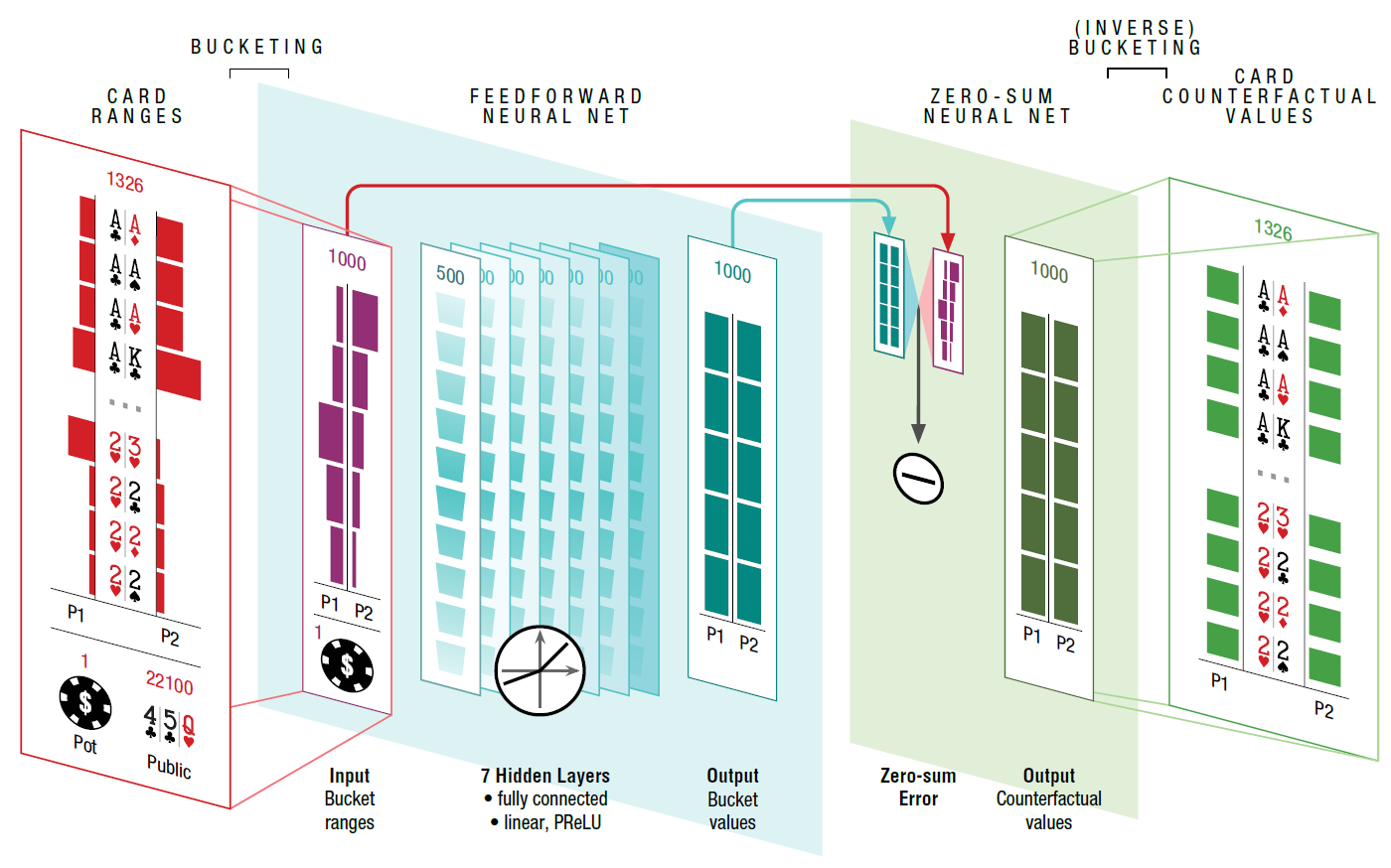
A feature of the program is that it actively resists the analysis of its strategy by the opponent. In other words, the program uses the
Nash equilibrium - a key concept of game theory. By Nash equilibrium is meant a set of strategies that no participant can increase the gain by changing their strategy if other participants do not change their strategies. From the point of view of the antagonistic poker game, the main task of DeepStack is to find the Nash equilibrium, that is, to minimize the possibility of another player exploiting his strategy for profit. Absolutely all poker programs developed until today were easily exploited after testing their strategy using the LBR (local best-response) technique - see a recent
review of the most modern poker bots .
So, DeepStack is not completely exploited using LBR. Coupled with the real results that the bot showed in the game with the professionals, there is only one question: why did the developers publish information about this architecture in the public domain?
The scientific work was
published on January 6, 2017 on the site arXiv.org, where articles are laid out before being published in the official journal.
The development team is led by computer science professor
Michael Bowling from the University of Alberta (USA).
DeepStack Development TeamThe Department of Poker Bots at the University of Alberta (Computer Poker Research Group) was created in the 90s, the first bot created here was
Loki in 1997. Then there were
Poki (1999),
PsOpti / Sparbot (2002),
Vexbot (2003),
Hyperborean (2006),
Polaris (2007),
Hyperborean No-Limit (2007),
Hyperborean Ring (2009),
Cepheus (2015) and, finally , the crown of creation - DeepStack.
In the near future, the DeepStack program will be tested in games with more experienced professionals, who are much higher than the guys in the table at the beginning of the article. Starting this weekend, the program
will play in a tournament at the Pittsburgh casino , where the arrival of several world-class professionals is expected. For 20 days DeepStack should play about 120,000 hands. This is quite a lot to accurately assess the quality of the program.
At the moment, DeepStack has played 44,852 hands against professional volunteers selected by the International Poker Federation. Players received cash prizes for a good game (first prize of $ 5,000 CAD), so people played at full strength. Nevertheless, the program is in a good plus.
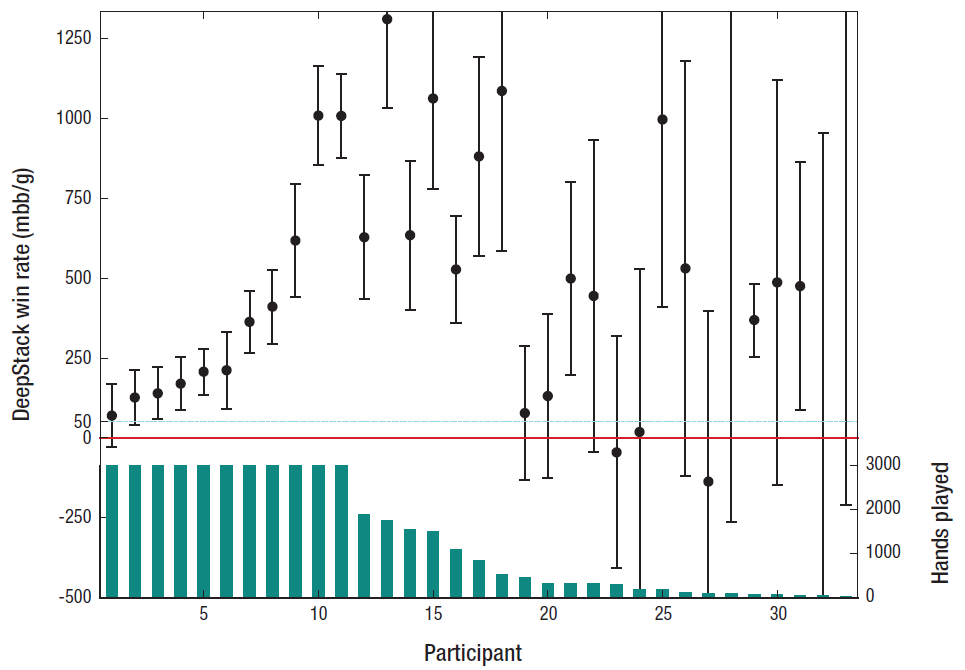