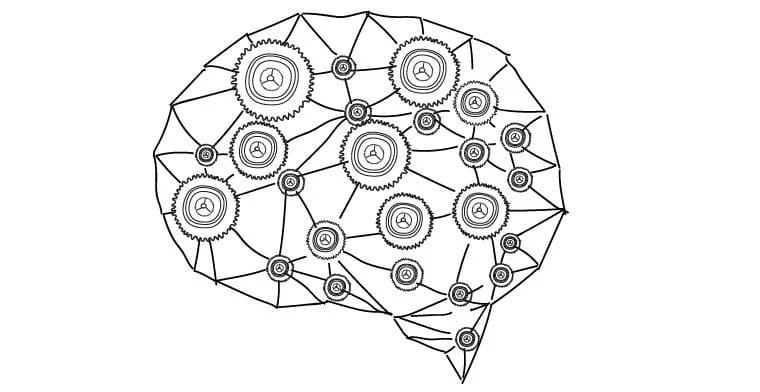
It is known that artificial intelligence needs thousands of examples to learn to recognize new objects. In this, he is significantly inferior to a person with his ability to recognize a class of objects by one representative. In addition, the process of learning a neural network takes a lot of time.
DeepMind , a Google Artificial Intelligence unit, has
found a way around this.
DeepMind researchers made several corrections for the deep learning algorithm, which allows it to recognize objects in images or other things from a single example using the links between a neural network and external memory. This technology is known as “one-time” training. The development team demonstrated the operation of this method on a large database of images with tags, as well as in writing and speech.
')
The best algorithms are able to reliably recognize objects, but for this they need a lot of data and time. The algorithm, trained to recognize cars on the road, has to study several thousand examples in order to work confidently in a car with an autopilot. Collecting such a large amount of data is often impractical: a robot that should help you navigate in unfamiliar terrain should not spend a lot of time learning.
To fix this, a researcher from DeepMind Oriol Vinyals added a memory component to the in-depth learning system — a neural network type trained to recognize things by adjusting a number of interconnected layers. His work is similar to neurons in the human brain. In order for such a network to work efficiently, it needs to see a multitude of images to fine-tune the communication between virtual neurons.
The DeepMind team demonstrated the capabilities of the updated system based on the
ImageNet database, which was developed by scientists at Stanford and Princeton University. It is organized in accordance with the hierarchy of the lexical database of the English language
WordNet , however, while it works only with nouns. With new software, artificial intelligence still needs to analyze several hundred categories of images, but after that it can learn to recognize new objects from a single image. The algorithm effectively determines the characteristics of the object that make it unique. ImageNet recognition accuracy has improved from 87.6% to 93.2% compared with competing approaches.
Neural network architecture
Vinyals says their development will be especially useful in recognizing the meanings of new words. He believes that this will be important for Google, since the algorithm allows the system to quickly learn the values of new search criteria.
“I think this is a very interesting approach that allows you to train the network from such large amounts of data once,” said Sang Van Lee, head of the Laboratory for Brain and Machine Intelligence at the Korean Institute of Advanced Technologies (
KAIST ). However, other scientists are in no hurry to praise the achievement of the DeepMind team. They are skeptical of its usefulness, since the method presented is still far from the speed of human learning. As rightly pointed out by Sam Gershman, an associate professor at the Harvard Brain Research Department, when people learn to “collect” an image from its component parts, they do it on the basis of knowledge about the world or common sense. That is, a sigway may look very different from a bicycle or a motorcycle, but it can be assembled from the same parts. Both scientists agree that machine intelligence will take a long time before it can achieve human abilities.
Science is still far enough from the disclosure of the mystery of "one-time" training of the human brain. However, the achievement of researchers from Google sets new goals for scientists, which deserve further study.
The technology of "one-time" training was known before, but it was not adapted to work with depth learning algorithms. In one educational project last year
, probabilistic programming methods were
used , which included this technology. In fact, the program generated a unique algorithm for each character using the strokes of an imaginary pen. The software did not imitate the algorithm for teaching the child to read and write, but it was similar to the way adults go when they learn a new language.
In-depth learning systems become much more capable if memory mechanisms are added. Another group in Google DeepMind has recently
developed a working memory network — a differentiated neural computer. He can not only perform complex tasks, but also decide what information to keep in his memory. So AI learned to navigate the system of the London Underground after studying a few more simple network diagrams. Like a regular computer, such a network uses its memory to manage complex data structures. However, the system can be trained on the basis of data, like a neural network. Scientists have high hopes for a differentiated neural computer. An improved version of such a computer will be able to scan Wikipedia and remember all the significant names, places and dates, and use this knowledge in a completely new way.