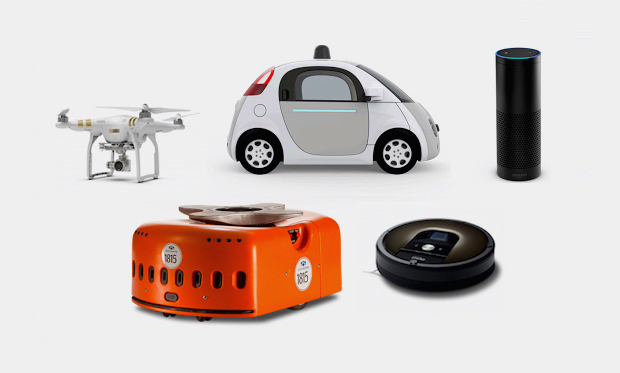
In the field of new technologies and products, we are accustomed to the fact that the “figure” is synonymous with all the advanced, modern and high-tech, and the “counterpart” - the whole of retrograde, obsolete and low-tech.
But if you think that the analogue has died, you are mistaken. Analog processing is not only a key component of the set of vital systems on which we rely, but also makes way for a new generation of computing and intelligent systems that underlie the very interesting technologies of the future: artificial intelligence and robotics.
')
Before we discuss the revival of the analog — and why engineers and innovators working on AI and robots should pay attention to this — you need to understand the importance and legacy of the old analog century.
Love for analog
During World War II, analog circuits played a key role in the operation of the first
automatic air defense systems , and in the following decades analog computers were needed to calculate the flight paths of rockets and spacecraft.
Analogue prevailed in control systems and communications in aircraft, ships and power plants. Some of these systems work to this day. Not so long ago, analog circuits controlled large parts of the telecommunications infrastructure (remember phones with dial pads?), And even copy machines in the office, when early photocopying units reproduced images without a single digital bit.
The love of analog has existed for so long, since this technology has constantly proven its accuracy, simplicity and speed. She corrected the course of missiles, led the court, recorded and reproduced music and video, united us for many decades. And then in the 1960s a figure appeared and quickly conquered the world.
Carburetors with feedback made a more efficient combustible mixture based on exhaust. But because of their complexity and unreliability, they were quickly replaced by digital fuel injection systems.Kingdom figures
Why has the figure replaced the analog? The greatest weakness of the analog is the lack of flexibility. When you try to give it flexibility, the complexity of the system grows exponentially. Complexity leads to a decrease in reliability, and engineers have begun to notice that
Moore's law makes calculations reliable and inexpensive.
At this time, MEMS and microforming technologies distributed sensors that receive physical signals and convert them to digital. Pretty quickly, the opamps were replaced by logic gates, falling exponentially. Instead of mechanical connections, information was
transmitted by wire , and the designers brought digitization of everything to the limit.
In the modern world of consumer electronics, analog is used only for interacting with people, capturing and producing sounds, images and other feelings. In large systems, the analog is used to physically rotate the wheels and steering of the machines that move us in our analog world. But in most other systems, engineers are trying to maximize the use of digital signals. The advantages of digital logic - low cost, speed, reliability, flexibility - have instilled in engineers an allergy to analog processing.
However, now, after a long break, the
prediction of Carver Mead about the return of the analogue begins to come true.
“Large-scale adaptive analog systems are more resistant to component degradation and errors than conventional systems and use less energy,”
Mead , Professor Caltech and a pioneer in microelectronics, wrote in 1990 for Proceedings of the IEEE. "Therefore, adaptive analog technology is likely to be able to realize the full potential of silicon chip production."

Everyone loves analog
Electronics developers perceive analogue as a necessary evil for interaction with the outside world. But it turns out that AI and
deep learning algorithms work better on analog and neuromorphic computer platforms.
In my company Lux Capital, we
sponsored Nervana , which built specialized integrated circuits that used convolutional neural networks to speed up the training of deep learning algorithms. And although the mathematical operations were carried out in digital form, the architecture of the system imitated the human brain at a high level.
Inspired by nature
Ask anyone (even a child) to sketch a robot, and you will most likely get an image resembling Rosie, the robot maid from The Jetsons, or C-3PO from Star Wars. And this is not surprising - for decades such a type of robots has been described in science fiction books, television and films. Recently, the idea of robots and their appearance evolves. Ask the
millennial to give an example of a robot, and he may call Roomba, Amazon's Echo, or even Siri.
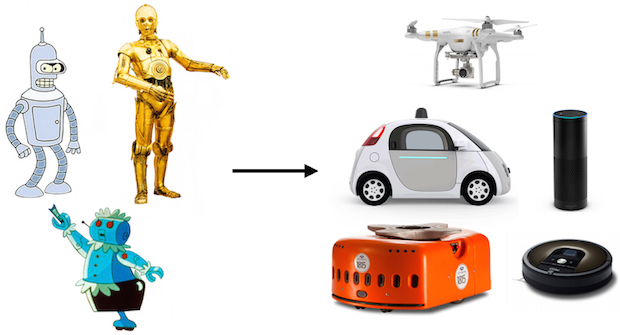
There is a steady trend towards intellectualization and robotization of an increasing number of gadgets and other systems present in our life. These systems will require small, portable and low-power computers; they will have to be able to respond at any time. This is a complex set of tasks for modern systems that typically consume a decent amount of energy (unless they are in standby mode) and must be connected to cloud services to perform useful functions. Here analog can help.
Taking inspiration in nature, scientists experiment with vision and hearing using analog circuits that consume a small amount of energy. The Stanford
Brains in Silicon project and the University of Michigan
IC Lab , with the support of
DARPA SyNAPSE and
the US Naval Research Laboratory , create tools that facilitate the creation of analog neuromorphic systems. There are also little-known startups. Instead of launching deep networks on conventional digital circuits, they develop analog systems capable of doing similar computations with much less energy, inspired by our analog brains.
Noise is not a problem
Why do we need to switch to analog? It's simple: we are at a unique stage of progress, where the neural networks we are trying to develop are more suitable for analog systems, despite the fact that explosive demand for such AI systems is expected.
Traditional hard algorithms work only when the calculations are accurate. If the contours on which traditional algorithms operate are not accurate, errors will get out of control and spread throughout the system. In neural networks, the internal state should not be accurate and clear, and the system adapts to display the desired result based on the specified input parameters. Our brains are very noisy systems that work great. Engineers learn that they can also build deep networks on silicon chips using similar “noisy” approaches — achieving energy savings hundreds of times.
The implications of this are massive. Imagine that in the future, wearable devices or assistants like Amazon Echo almost do not use energy, and can even extract it from the environment, and do not require power wires and batteries. Or imagine a gadget that doesn't need to be connected to the cloud to be “smart.” His "intelligence" is enough to work even without Wi-Fi and cellular. And this is only the beginning of what, I think, will become the new category of AI and robots, which will appear in the near future - and all thanks to the good old analogue.