Language-understanding machines would be very helpful. But we do not know how to build them.
About the illustrations to the article: one of the difficulties in computer understanding of the language is the fact that often the meaning of words depends on the context and even on the appearance of letters and words. In the images presented in the article, several artists demonstrate the use of various visual hints that convey a meaning that goes beyond the limits of the letters themselves.In the midst of a tense go game that ran in Seoul in South Korea between Lee Sedol, one of the best players of all time, and AlphaGo, AI created by Google, the program made a mysterious move that demonstrated its defiant superiority over a human rival.
')
On the 37th move, AlphaGo decided to put the black stone in a strange at first glance position. Everything went to the fact that she had to lose a significant piece of territory - the beginner's mistake in the game, built on the control of the space on the board. Two commentators argued about whether they understood the computer correctly and whether it had broken. It turned out that, despite the contradiction to common sense, the 37th move allowed AlphaGo to build a formidable structure in the center of the board. The program from Google essentially won the game with a move that none of the people would have thought of.
The victory of AlphaGo is also impressive because the ancient game of go was often viewed as a test for intuitive intelligence. Its rules are simple. Two players take turns placing black or white stones at the intersection of the horizontal and vertical lines of the board, trying to surround the opponent’s stones and remove them from the board. But to play it well is incredibly difficult.
If chess players are able to calculate a game a few steps forward, in Go it quickly becomes an incredibly difficult task, moreover, there are no classic gambits in the game. There is also no easy way to measure the benefits, and even for an experienced player it can be difficult to explain why he made such a move. Because of this, it is impossible to write a simple set of rules that a program playing at expert level would follow.
AlphaGo was not taught to play go. The program analyzed hundreds of thousands of games and played millions of games with itself. Among the various AI techniques, she used the increasingly popular method known as deep learning. It is based on mathematical calculations, the method of which is inspired by the way the interconnected layers of neurons in the brain are activated when processing new information. The program taught itself over many hours of practice, gradually honing its intuitive sense of strategy. And the fact that she was then able to win from one of the best go players in the world is a new milestone in machine intelligence and AI.
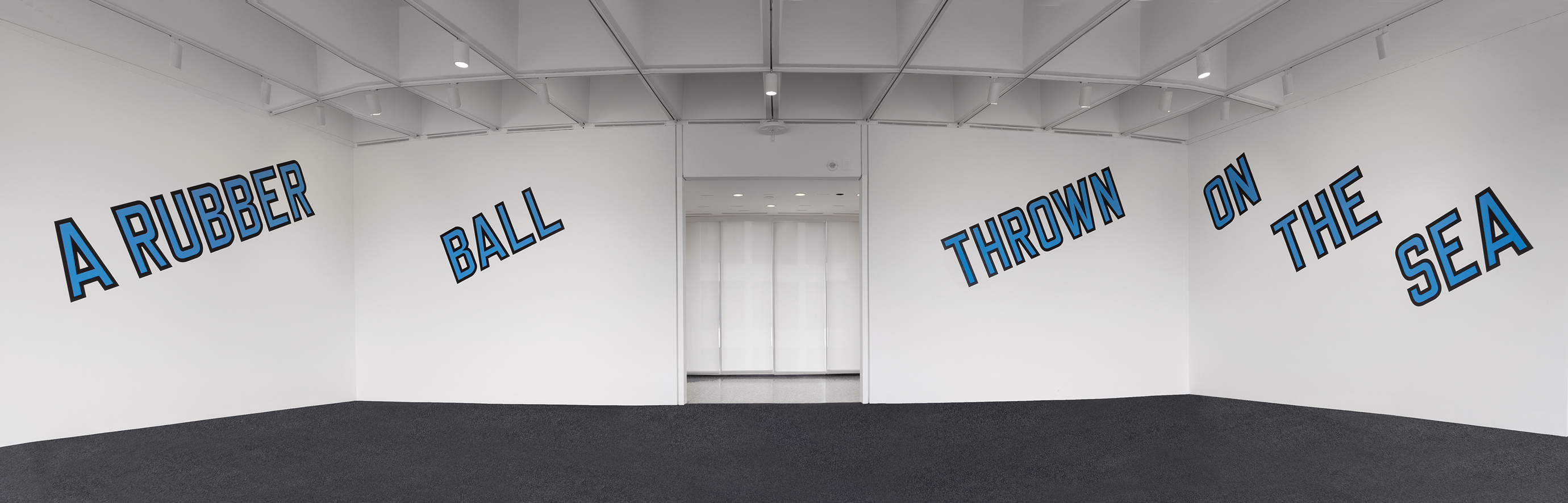
A few hours after the 37th move, AlphaGo won the game and began to lead 2-0 in a five-game match. After that, Sedol stood in front of a crowd of journalists and photographers and politely apologized for letting down mankind. “I was speechless,” he said, blinking under the lines of flashlights.
AlphaGo's amazing success shows how much progress has been made in AI over the past few years, after decades of despair and problems described as “the winter of AI”. In-depth training allows machines to independently learn how to perform complex tasks, the solution of which several years ago could not be imagined without the participation of human intelligence. Robomobili already looming on the horizon. In the near future, systems based on in-depth training will help with the diagnosis of diseases and the issuance of treatment recommendations.
But despite these impressive shifts, one of the main possibilities is not given in any way. AI: language. Systems like Siri and IBM Watson can recognize simple verbal and written commands and answer simple questions, but they are not able to speak or actually understand the words used. For AI to change our world, this must change.
Although AlphaGo does not speak, there is technology in it that can give a better understanding of the language. At Google, Facebook, Amazon, and research laboratories, researchers are trying to solve this stubborn problem using the same AI tools — including deep learning — that are responsible for AlphaGo's success and the revival of AI. Their success will determine the scope and properties of what is already beginning to turn into an AI revolution. This will determine our future - will there be machines with which it will be easy to communicate, or will AI systems remain mysterious black boxes, even if they are more autonomous? “It’s not possible to create a humanoid system with AI if it is not based on language,” said Josh Tenenbaum [Josh Tenenbaum], a professor of cognitive sciences and computation from MIT. “This is one of the most obvious things that determines human intelligence.”
Perhaps the same technologies that allowed AlphaGo to conquer will allow computers to master the language, or something else is needed. But without understanding the language, the influence of AI will be different. Of course, we will still have unrealistically powerful and intelligent programs like AlphaGo. But our relationship with the AI ​​will not be so close, and probably not so friendly. “The most important question from the beginning of the research was“ What if you got devices that are intelligent in terms of efficiency, but not similar to us in terms of lack of sympathy for who we are? ”Says Terry Winograd, honored Professor at Stanford University. "You can imagine machines that are not based on human intelligence, work with big data and control the world."
Talking with cars
A couple of months after the triumph of AlphaGo, I went to Silicon Valley, the heart of the AI ​​boom. I wanted to meet with researchers who have made significant progress in practical applications of AI and are trying to give machines an understanding of the language.
I started with Vinohrad, who lives in the suburbs on the southern edge of the Stanford campus in Palo Alto, not far from the headquarters of Google, Facebook and Apple. His curly gray hair and thick mustache give him the appearance of a respectable scientist, and he infects with his enthusiasm.
In 1968, Grape made one of the earliest attempts to teach cars to talk. Being a mathematical prodigy, keen on language, he came to the new MIT AI laboratory to get a degree. He decided to create a program that communicates with people through text input in everyday language. At the time, it did not seem such a daring goal. In the development of AI, very big steps were taken and other teams at MIT built complex computer vision systems and robotic manipulators. “There was a sense of unknown and unlimited possibilities,” he recalls.

But not everyone believed that the language is so easy to conquer. Some critics, including the influential linguist and MIT professor Noam Chomsky, thought that it would be very difficult for AI researchers to teach machines understanding, since the mechanics of language were very poorly studied in humans. Grapes recalls a party at which a student Chomsky left him after he heard that he was working in an AI laboratory.
But there are reasons for optimism. Joseph Weizenbaum, a MIT professor of German descent, made the first chatbot program a couple of years ago. Her name was ELIZA and she was programmed to respond as a psychologist from cartoons, repeating key parts of statements or asking questions inspiring to continue the conversation. If you informed her that you are angry with your mother, the program could answer “What else comes to your mind when you think about your mother?”. A cheap stunt that worked surprisingly well. Weisenbaum was shocked when some test subjects began to check their dark secrets to his car.
Grapes wanted to do something that would convincingly pretend that they understand the language. He began by reducing the scope of the problem. He created a simple virtual environment, the "block world", consisting of a set of fictional objects on a fictional table. He then created a program, calling it SHRDLU, able to parse all the nouns, verbs, and simple grammar rules necessary for communication in this simplified virtual world. SHRDLU (a meaningless word made up of the linotype's keyboard letters in a row) could describe objects, answer questions about their relationships, and change the blocky world in response to commands entered. She even had some memory and if you asked her to move the red cone, and then wrote about a certain cone, she assumed that you meant this red cone, and not any other.
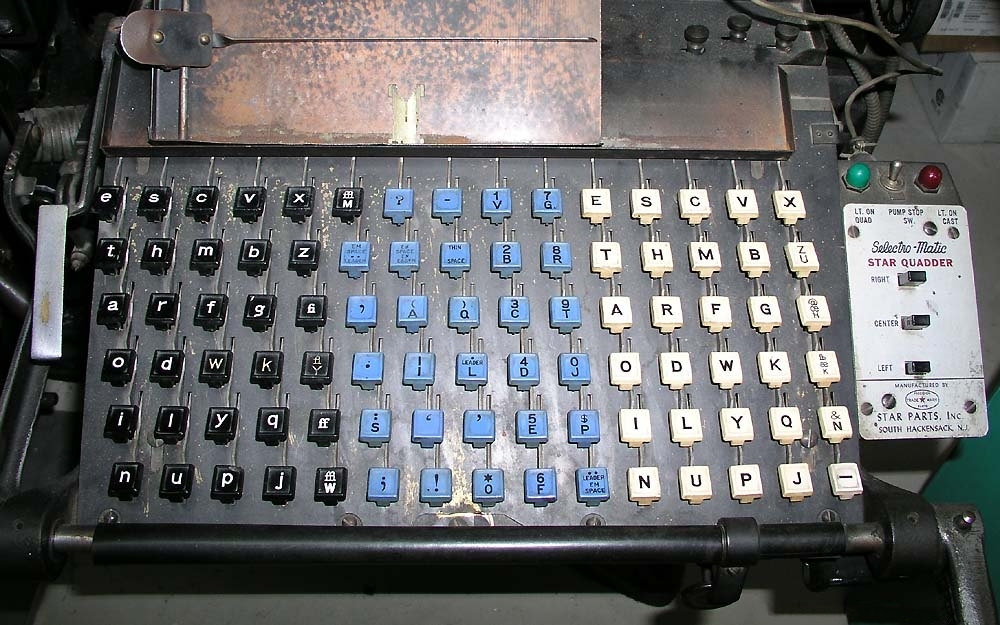
SHRDLU has become the banner of a huge progress in the field of AI. But it was just an illusion. When Grapes tried to expand the block world of the program, the rules necessary to account for additional words and the complexity of the grammar became unmanageable. Only a few years later he gave up and left the AI ​​field, concentrating on other studies. “The restrictions turned out to be much stronger than it seemed then,” he says.
Grapes decided that with the tools available at the time, it was impossible to teach the machine to truly understand the language. The problem, according to Hubert Dreyfus, a professor of philosophy at the University of California at Berkeley, expressed in the 1972 book What Computers Can't Do [What Computers Can't Do], requires a lot of instinct which cannot be set by a set of simple rules. That is why, before the start of the match between Sedol and AlphaGo, many experts doubted that the machines could master the game of go.
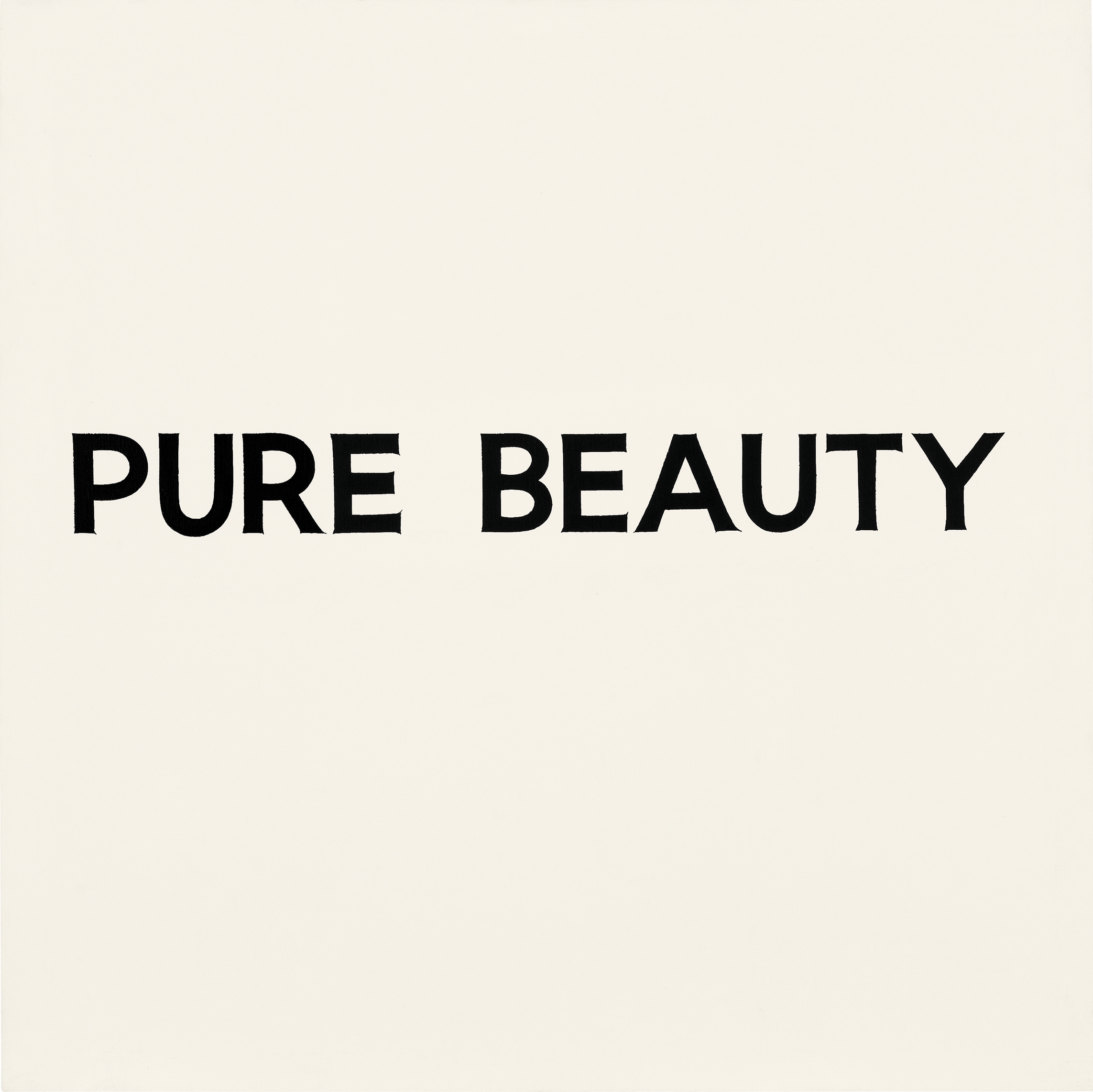
But while Dreyfus was proving his point, several researchers developed an approach that ultimately gives the machines the intelligence of the right kind. Inspired by neuroscience, they experimented with artificial neural networks — layers of mathematical simulations of neurons that can be trained to activate in response to certain inputs. At the beginning, these systems worked impossibly slowly and the approach was rejected as impractical for logic and reasoning. However, a key opportunity for neural networks was the ability to learn what was not manually programmed, and later it turned out to be useful for simple tasks like handwriting recognition. This skill found commercial use in the 1990s for reading numbers from checks. Proponents of the method were confident that over time, the neural networks will allow the machines to do much more. They argued that someday this technology will help and recognize the language.
Over the past few years, neural networks have become more complex and powerful. The approach flourished with key mathematical improvements, and, more importantly, faster computer hardware and the emergence of a huge amount of data. By 2009, researchers at the University of Toronto showed that multilayer deep learning networks can recognize speech with record accuracy. And in 2012, the same group won the machine vision competition using a deep learning algorithm that showed amazing accuracy.
A deep learning neural network recognizes objects in pictures with a simple trick. The layer of simulated neurons receives input as a picture and some of the neurons are activated in response to the intensity of individual pixels. The resulting signal passes through multiple layers of interconnected neurons before reaching the output layer, signaling the observation of the object. The mathematical technique called back propagation is used to adjust the sensitivity of the neurons of the network to create the correct answer. It is this step that gives the system the opportunity to learn. Different layers in the network respond to properties such as edges, colors, or texture. Such systems today are able to recognize objects, animals or faces with rival accuracy with human.
With the use of technology of deep learning to the language there is an obvious problem. Words are arbitrary characters and in this way they are essentially different from images. Two words can have a similar meaning and contain completely different letters. And the same word can mean different things depending on the context.
In the 1980s, researchers gave a cunning idea of ​​turning a language into a type of problem that a neural network can handle. They showed that words can be represented as mathematical vectors, which allows us to calculate the similarity of related words. For example, the “boat” and “water” are close in the vector space, although they look different. Researchers at the University of Montreal, under the leadership of Yoshua Bengio and another group from Google, used this idea to build networks in which each word in the sentence is used to build a more complex representation. Geoffrey Hinton, a professor at the University of Toronto, and a prominent in-depth study researcher who also works at Google, calls this the “mental vector.”
Using two such networks, you can make translations from one language to another with excellent accuracy. And by combining these types of networks with the one that recognizes the objects in the pictures, you can get amazingly accurate subtitles.
Meaning of life
Sitting in a conference room in the heart of the bustling Google headquarters in Mountain View (California), one of the company's researchers who developed this approach, Quoc Le [Quoc Le], talks about the idea of ​​a machine capable of supporting a real conversation. Lee's ambitions explain how talking machines can be helpful. “I need a way to simulate thoughts in a car,” he says. “And if you want to simulate thoughts, then you can ask the car what it is thinking about.”

Google is already teaching its computers the basics of the language. In May, the company unveiled the Parsey McParseface system that can recognize syntax, nouns, verbs, and other text elements. It's easy to see how understanding a language can help a company. The Google search algorithm once simply tracked keywords and links between web pages. Now RankBrain system reads the text of pages to understand its meaning and improve search results. Lee wants to push this idea even further. Adapting a system that turned out to be useful for translations and captions of pictures, they and their colleagues created Smart Reply, which reads the contents of letters to Gmail and offers possible answers. They also created a program that has been trained on the basis of the Google support chat to answer simple technical questions.
Recently, Lee has created a program that can generate tolerable answers to difficult questions. She trained on dialogues from 18,900 films. Some answers are frighteningly accurate. For example, Lee asked, “What is the meaning of life?” And the program responded, “In service to the highest good.” “A good answer,” he recalls with a smirk. “Perhaps better than I would answer myself.”
There is only one problem that becomes apparent when looking at a greater number of system responses. When Lee asked, “How many feet does a cat have?”, The system responded, “I think four.” Then he asked, “How many legs does a centipede have?” And received a strange answer, “Eight.” In essence, Lee’s program does not understand what he is talking about. She understands that some combinations of characters fit together, but she does not understand the real world. She doesn't know what a centipede looks like, or how it moves. It is still an illusion of intelligence, without common sense, which people take for granted. Systems of deep learning in this sense are rather fragile. A Google system that creates image captions sometimes makes weird mistakes, for example, describes a road sign as a refrigerator with food.
By a strange coincidence, a man who can help computers better understand the real meaning of words turned out to be Terry Winograd's neighbor in Palo Alto. Fei-Fei Li, director of the Stanford Artificial Intelligence Laboratory, was on maternity leave during my visit, but she invited me home and proudly introduced me to her three-month-old baby, Phoenix. “Please note that she looks at you more than me,” Li said when Phoenix stared at me. - This is because you are new; This is an early face recognition. "
Lee spent most of her career researching machine learning and computer vision. Several years ago, under her leadership, an attempt was made to create a database of millions of images of objects, each of which was signed by the corresponding keywords. But Lee believes that the machines need a more complex understanding of what is happening in the world and this year her team released another database with images, the annotations for which were much richer. For each picture, people made dozens of signatures: “Dog on a skateboard,” “The dog has a thick waving fur,” “A road with cracks,” and so on. They hope that machine learning systems will learn to understand the physical world. “The lingual part of the brain receives a lot of information, including from the visual system,” says Lee. “An important part of AI will be the integration of these systems.”
This process is closer to teaching children to associate words with objects, relationships, and actions. But the analogy with teaching people does not go too far. Kids do not need to see a dog on a skateboard to imagine it or describe it in words. Lee believes that today's tools for AI and machine learning will not be enough to create a real AI. “It’s not just going to be a deep learning with a big data set,” she says. “We, the people, are very poor at calculating big data, but very well with abstractions and creativity.”
No one knows how to endow machines with these human qualities and whether it is possible at all. Is there something exclusively human in such qualities that does not allow the AI ​​to possess them?
Specialists in cognitive sciences, for example, Tenenbaum from MIT, believe that today's neural networks lack critical components of the mind - regardless of the size of these networks. People are able to learn relatively quickly on relatively small amounts of data, and they have a built-in ability to effectively simulate a three-dimensional world. “Language is built on other possibilities, probably lying more deeply and present in babies even before they begin to speak the language: visual perception of the world, working with our motor apparatus, understanding the physics of the world and the intentions of other creatures,” says Tenenbaum.
If he is right, then without attempts to simulate the human learning process, create mental models and psychology, it will be very difficult to recreate an understanding of the language with AI.
Explain yourself
Noah Goodman's office at Stanford Psychology is almost empty, with the exception of a couple of asbestos paintings on one of the walls and a few overgrown plants. At the time of my arrival, Goodman scribbled something on the laptop, putting his bare feet on the table. We walked around the sun-filled campus to buy iced coffee. “The peculiarity of the language is that it relies not only on a large amount of information about the language, but also on the universal understanding of the world and these two areas of knowledge are implicitly related to each other,” he explains.
Goodman and his students developed the Webppl programming language, which can be used to endow computers with probabilistic common sense, which during conversations is quite important. One experimental version is able to recognize a pun, and the other is hyperbole. If she is told that some people have to spend an “eternity” waiting for a table in a restaurant, she will automatically decide that the literal meaning of this word is unlikely to be used in this case and that people are most likely waiting for a long time and getting annoyed. The system cannot yet be called true intelligence, but it shows how new approaches can help AI programs speak a little more vitally.
Also, the example of Goodman shows how difficult it will be to teach machines language. Understanding the meaning of the concept of "eternity" in a certain context is an example of what the AI ​​systems must learn, while this is actually a fairly simple and rudimentary thing.
Nevertheless, despite the complexity and complexity of the task, the initial successes of researchers using deep learning for pattern recognition or go games give hope that we are on the verge of a breakthrough in the language field as well. In this case, this breakthrough arrived just in time. If an AI should become a universal tool, help people supplement and strengthen their own intelligence and perform tasks in a seamless symbiosis mode, then language is the key to achieving this state. Especially if the AI ​​systems will increasingly use deep learning and other technologies for self-programming.
“In general, deep learning systems are awe-inspiring,” says John Leonard, a professor who studies ro-mobils at MIT. “On the other hand, their work is quite difficult to understand."
Toyota, a student of autonomous driving technology, launched a research project at MIT led by Gerald Sussman, an expert on AI and programming languages, to develop an autonomous driving system that could explain why she did something other action. The obvious way to give such an explanation would be verbal. “Creating systems that are conscious of their knowledge is a very difficult task,” says Leonard, who runs another Toyota project at MIT. “But, yes, ideally, they should give not just an answer, but an explanation.”
A few weeks after returning from California, I met David Silver, a Google DeepMind researcher and AlphaGo developer. He spoke about the match against Sedol at a scientific conference in New York. Silver explained that when the program in the second game made its decisive move, his team was as surprised as the others. They could only see that AlphaGo predicted the odds of winning, and this prediction did not change much after the 37th move. Only a few days later, after carefully analyzing the game, the team made a discovery: after digesting previous games, the program calculated that a human player could make such a move with a probability of 1 in 10,000. And her training games showed that such a maneuver provides an unusually strong positional advantage .
So, in a sense, the car knew that this move would hit the weak point of Sedol.
Silver said that Google is considering several opportunities to commercialize this technology, including intelligent assistants and tools for medical care. After the lecture, I asked him about the importance of being able to communicate with the AI ​​managing such systems.
“An interesting question,” he said after a pause. - For some applications this may be useful. For example, in health care, it may be important to know why a particular decision was made. ”In fact, AIs are becoming more and more complex and confusing, and it’s very hard to imagine how we will work with them without language — without being able to ask them, “Why?”. Moreover, the ability to easily communicate with computers would make them more useful and look like magic. After all, language is the best of our ways to understand and interact with the world. It is time for cars to catch up with us.