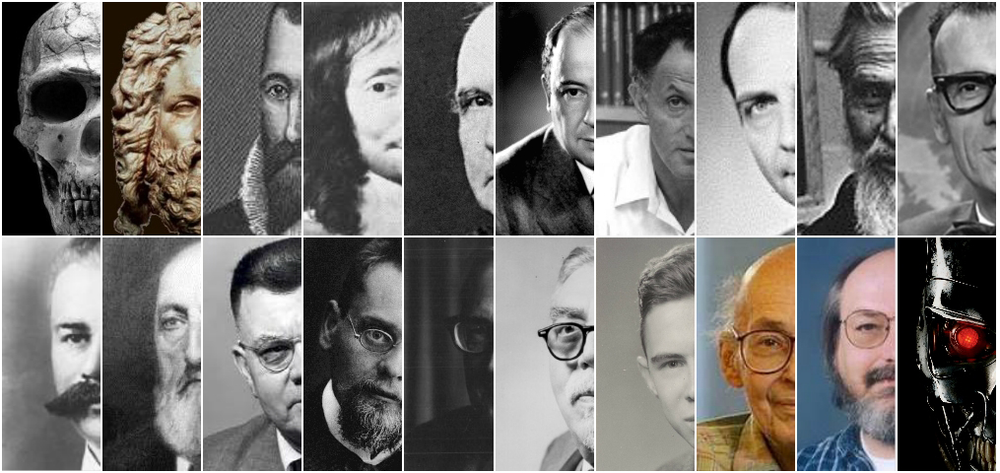
In 16 games, cars overpowered man (at 17, if we take into account Lee Sedol’s defeat in go), but in the future they will have even more impressive achievements: solving the most stunning mathematical, physiological and biological problems, defeating diseases and old age, eliminating road accidents , triumph in military conflicts and much more.
The world has changed right before our eyes, but not everyone noticed it. When and how did programs learn to play flawlessly? Does the loss of one person always indicate the defeat of all mankind? Will artificial intelligence gain consciousness?
')
About the author. The article is based on the lecture “Artificial Intelligence. History and Prospects ”, held in the Moscow office of the Mail.Ru Group by Sergey oulenspiegel Markov. Sergey Markov is involved in machine learning at Sberbank. In the banking sector, predictive models are built for managing a business process on the basis of fairly large training samples, which can include several hundred million cases. Among his hobbies, Sergey specifies chess programming, AI for games, minimax problems. The SmarThink program, created by Sergey Markov, became the champion of Russia (2004) and the CIS (2005) among chess programs (2004), and today is among the top 30 strongest programs in the world. Sergey is also the founder of the non-profit scientific and educational portal of the 22nd century .How it all began
TechnoCore is the dwelling place of a multitude of artificial intelligence in the tetralogy of Hyperion's Songs of Dan Simmons. One example of visualization of strong AI in science fiction.There was an unpleasant story with the very concept of AI. Appearing initially in the scientific field among specialists working in the field of computer science, it very quickly got into the public environment and underwent various changes. If today you ask the average person what AI is, most likely, you will hear from him the very definition that AI specialists have in mind.
Before you begin a story, you need to decide what we mean by artificial intelligence, and where is the boundary where computer science ends and adjacent areas begin.
AI in general is the methods used to automate tasks that are traditionally considered to be intellectual tasks, and for solving which a person uses his natural intelligence. By automating the problem being solved by a person with the help of the brain, we thereby create an AI. Another thing is that such an AI is usually called applied or, with some contempt, weak. The unpleasant nuance is that this is the only type of AI available to humanity today.
As for the strong AI, artificial general intelligence (
AGI ), there are certain developments here, but so far we are at the very beginning of the path. Strong AI is a system capable of solving any intellectual tasks. In fiction, an image of just such a person-like AI was formed, capable of solving any problem.
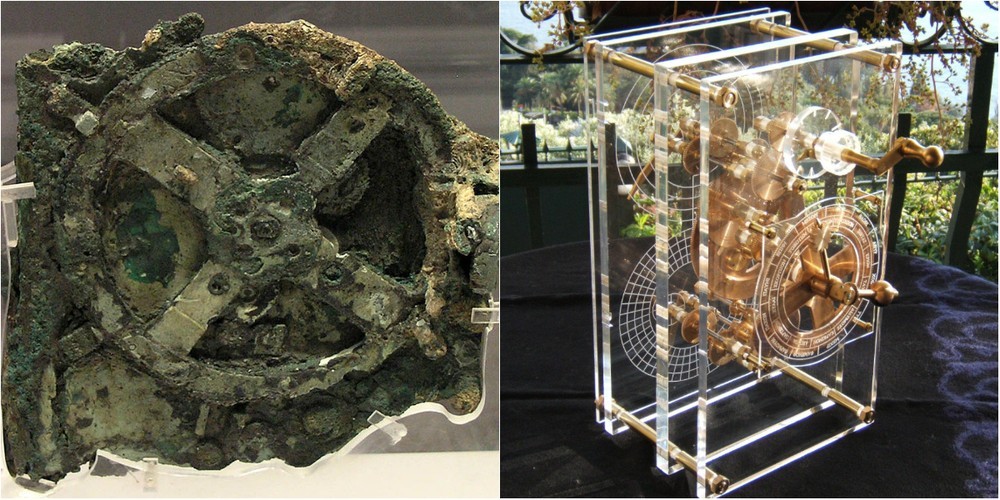
Since we define artificial intelligence in computer science so widely, then from a formal point of view we will find the elements of the existence of AI even among the ancient Greeks. We are talking about the famous
Antikythera mechanism , which is one of the
inappropriate artifacts . This device is intended for astrophysical calculations. The photo above shows one of the surviving fragments and a modern reconstruction of the artifact. The mechanism contained 37 bronze gears in a wooden case, on which dials with arrows were placed. It was possible to restore the position of the gears inside the mineral-coated fragments using X-ray computed tomography.
By itself, the task of counting was once considered intellectual — if a person could count well, then he could be called an intellectual.
John Napier counting device , designed for quick multiplication.Account tasks were automated one of the first. Even before the first mechanical devices, crafts such as neperovy sticks appeared. Scottish mathematician, one of the inventors of logarithms,
John Napier , created the counting device in 1617. Against the background of modern technology, it seems ridiculous: paper rolls are, in fact, the first logarithmic tables for performing some operations. But for contemporaries, it was about the same miracle as for us some AlphaGo. At one time there were even poets who devoted poems to non-peppered sticks (Napier's bones). They say why you are worn with the bones of your ancestors, these are the bones that you really should be proud of.
Pascal summing machine - an arithmetic machine, invented by the French scientist Blaise Pascal in 1642Pascal, along with the German mathematician Wilhelm Schickard, who proposed his version of the computer in 1623, laid the foundations for the advent of most computing devices. To this day have survived several cars created during the life of Pascal. Such a machine was able to add, divide, subtract and multiply.
A complete working copy of a machine invented by the English mathematician Charles Babbage in 1822. The so-called difference machine is designed to automate calculations by approximating functions by polynomials and calculating finite differences.Almost all computer specialists have heard about
Charles Babbage . Alas, Babbage could not create a working computer. For a long time, disputes have not subsided whether the differential machine proposed by him works in principle. In the period from 1989 to 1991, to the 200th anniversary of the birth of Charles Babbage, a working copy of the analytical machine was assembled from preserved drawings and with minor modifications. Modifications are needed because of the "bugs" found in the drawings. Perhaps Babbage deliberately made a few distortions, struggling with unlicensed pirated copying.
The analytical machine is a prototype of the modern
Von Neumann machines . When IBM engineers built the first electronic machine, they used Babbage's drawings. We are used to talking about the von Neumann architecture, that is, a machine with a distributed computing device, an input / output device, memory, etc. However, the basis is the ideas of Babbage.
In addition, in the machine code of the Babbage machine, a conditional branching operator and a cycle operator were used. This car really can be considered the great-grandfather of what later managed to do on the basis of electronics.
Jacquard machine - shedding mechanism loom for the production krupnouzorchatyh fabrics. Created in 1804. Allows you to separately manage each thread of the base or a small group of them.Input-output devices, punch cards appeared before Babbage - they were used in weaving Jacquard looms to set the order of the threads in the manufacture of fabric.
New time
Tabulator is an electromechanical machine designed for automatic processing of numeric and alphabetic information recorded on punch cards.The first statistical tabulator was built by American
Herman Hollerith in 1890 to solve problems related to statistical calculations. A well-known inventor and creator of punch card equipment successfully took part in a competition organized by the US government to find means of automating the processing of population census results. For manual calculation and analysis, it would take about 100 people working for 4-5 years to simply summarize and calculate the total sociological indicators of the census.
Punch card Hollerith.A tabulator is a very primitive summary table. Each card corresponds to the questionnaire, holes are made in it, corresponding to the answer. The cards were loaded into the tabulator, he quickly chased them through himself and gave the result in the form of the same card with the calculated amount. These amounts were invested in another tabulator, etc.
Winning the competition, Hollerith founded his own company, the Tabulating machines company, and after a series of mergers and acquisitions, it became known as IBM.
Games played by cars
As in any experimental science, the computer sciencé section dealing with AI needed its own fruit fly, a kind of model object, on which it was possible to test AI methods. The game is the most famous fruit fly Drosophila in the field of AI.
The game is a pure model space. It sets the conditions, states, uniquely defined and described as a set of parameters. Creating an AI for the game, we thereby abstract away from the mass of engineering problems that lie between the AI and the practical solution of the problem. We abstract from data coding on input and output, signal conversion, perception tasks, etc. For us, the intellectual challenge exists in the game in its pure form. This is convenient because AI specialists are lazy and do not want to do anything other than AI.
Chess machine " Turk ".The game is a spectacle. If you show a car that can play well, it will impress even an unknowing person. The game machine has attracted attention for hundreds of years. In the picture above, you see an illustration of the famous hoax - the first chess machine, designed by the inventor
Wolfgang von Kempelen in 1769. Inside the “machine”, the real chess player was actually hiding. The leading chess players of their time did not disdain into this box. Johann Baptist Algayer, the strongest Austrian chess player of the end of the 18th - beginning of the 19th centuries, played a number of games for the chess machine.
This complex mechanical device is not artificial intelligence, but it is curious that it was used to fool contemporaries with it. People really believed that some skillful master could create a system capable of playing chess with the help of gears, levers, mechanisms and counterweights. A record of the game played by a mechanical Turk against Napoleon has been preserved (however, some historians question its authenticity). So, it is possible that even higher persons were fooled.
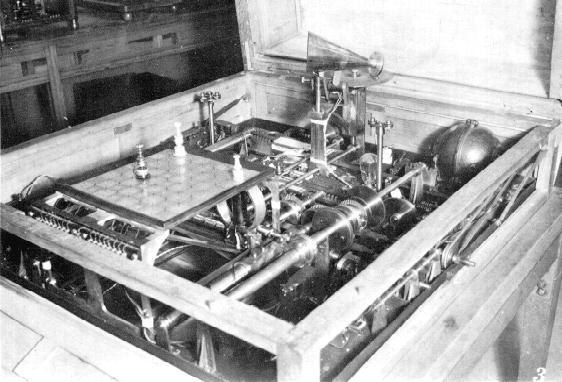
And this is the first device that actually skillfully play chess. El Ajedrecista (translated into Russian - "chess player"). It was created in 1912 by the famous Spanish mathematician and engineer
Leonardo Torres Quevedo .
El Ajedrecista was a chessboard on which the machine moved the king and the rook using electromagnets. The machine guaranteed to checkmate the king and the rook to the lonely king from one position: the king stood on White on H8, the rook on G7, and the black king could stand on any field. Two surviving copies of the machine are exhibited in the Polytechnic Museum of Madrid.
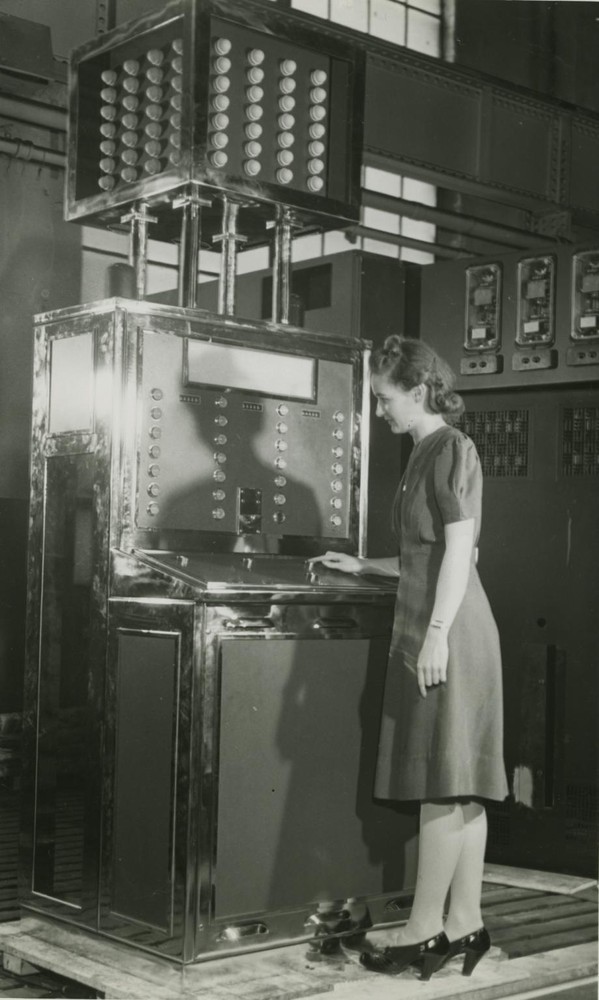
This is another famous AI of the past - the Nimatron mechanism, created in 1940 to play in It. Nim is a math game in which two players take turns taking items laid out in several piles (usually three). In one move, you can take any number of items (more than zero) from one pile. Wins the player who took the last item.
This is one of the first games for which they built a complete mathematical theory as early as 1901-1902. From the point of view of the Nimatron methods, he did not represent anything new, relying on the already known optimal strategy for this game, but was one of the first solutions to the theory of games in hardware.
The Nimatron project was led by
Edward Ulear Condon , one of the fathers of modern quantum mechanics. He considered this project the biggest failure in his life, but not from a technical, but from the financial side. Working on Nimatron, Condon and his team invented many technologies (among which, for example, a method of manufacturing printed circuit boards), which were later in demand in computer technology. But the team of specialists did not patent anything, and as a result did not earn anything.
Optimal strategy
Ernst Friedrich Ferdinand Zermelo is a German mathematician who made a significant contribution to the theory of sets and the creation of axiomatic foundations of mathematics.Talking about artificial intelligence for games is not complete without mentioning Ernst Zermelo and his theorem. Russian-speaking fans of this topic are lucky. Zermelo's work was originally written in German. And if it was only translated into English in 1999, then into Russian - back in the late 1960s - early 1970s. Because of this, in the Russian-language literature it is written more or less correctly about what Zermelo did, and in the English-language literature they are still confused. For example, in one of the works it is said that Zermelo proved that if whites play perfect moves in chess, then they will necessarily achieve at least a draw. In fact, no one has provided such evidence to date, but Zermelo made another important discovery.
He did not play chess very well, and he didn’t know the rules of the game very well. Maybe because of this, he proved in 1913 the first formal theorem of game theory. According to the book “Managed Processes and Game Theory”, published in the USSR in 1955, the correct description of Zermelo's contribution is: “Zermelo proved the determinism of games like chess, and that rational players can, using complete information, develop an optimal game strategy ".
The usual rules of the game of chess know almost everything, but there are several other very important and not quite obvious rules. For example, the rule of 50 moves says that if no pawn moved forward for 50 moves and not a single piece was taken, a draw is awarded. Or if a position repeated three times - a draw is also awarded. There can be no endless game of chess in terms of the number of moves - at some point the game will somehow stop. Ernst Zermelo did not know this, and he thought that endless games were possible in chess: you went as a figure - you returned it, and you play until the universe crumbles into dust.
How to win in tic-tac-toe
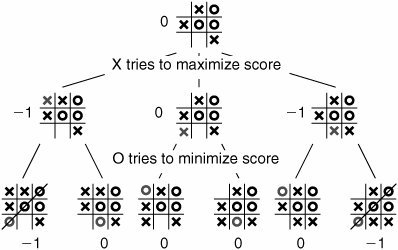
So, let's see how the optimal strategy of the game is calculated. Let's a little distract from chess, let's take a simpler example - tic-tac-toe, one of the oldest games in the world, which appeared in ancient Egypt. For this game, Zermelo used
reverse induction - the method by which a search is conducted in the opposite direction: starting with the positions where one of the parties has already received a pat or mate, and ending with a specific position on the board.
Zermelo did not give a detailed description of reverse induction, but used its principle, according to which there is a position advantageous for one of the parties. We can assign a score for such positions: –1 - won the toes, 1 - won the crosses, 0 - a draw.
Now we can go up the tree from these positions and see if there is a move from a position leading to a winning position. If there is a move, then the position is considered to be winning.
On the other hand, if there is no move leading to a winning position, but there is at least one move leading to a drawn position, then this position is considered a draw. And if there is not a single move leading either to a draw or to a winning position, then the position is considered lost.
Using this rule, we in the tree, starting from the leaves, move up, and assign estimates to nodes until we assign estimates to all positions. Having received all the estimates for all positions, we see the ideal algorithm for the game. Having appeared in some position of the game, we will always see a move (if there is one) leading to a winning position. If there is no winning move, then we will make a draw move, and only in the worst case will we go to a losing position. Theoretically, such a system capable of storing the evaluation tree for each position in memory will play at the level of God.
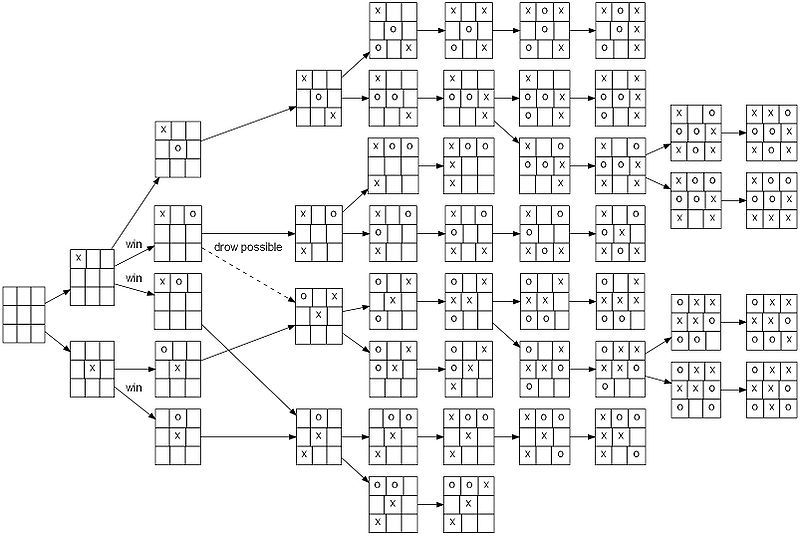
Zermelo understood this, but believed that this tree has infinite branches, where there are no terminal positions. He asked a question: is it possible for a game that has an infinite tree by the size of positions in it to calculate the optimal strategy for a finite time, that is, it is guaranteed to make an ideal move in each position? And he proved that despite the fact that the tree itself can be infinite, we can find the optimal strategy in a finite number of iterations. And this finite number of iterations does not exactly exceed the number of different positions in the game.
EDSAC (Electronic Delay Storage Automatic Calculator) is the world's first active and practically used computer with a stored program, created in 1949 at the University of Cambridge (United Kingdom). In 1952, the implementation of the tic-tac-toe game for the EDSAC computer was one of the first video games. The computer has learned to play ideal games against a person, operating with the optimal strategy known to him.
Reverse induction: the history of the method
Economist Oscar Morgenstern , one of the authors of game theory, and mathematician John von Neumann , who made an important contribution to quantum physics, functional analysis, set theory, and other branches of science.The work on the interpretation of reverse induction is in the field of set theory. Another area of knowledge, without which it is impossible to imagine the development of game theory, lies in the field of economics. In 1944, John von Neumann and Oscar Morgenstern published a monograph “Game Theory and Economic Behavior”, in which the authors summarized and developed the results of game theory and proposed a new method for evaluating the utility of benefits. In this work, the definition of the reverse induction method was formally given for the first time.
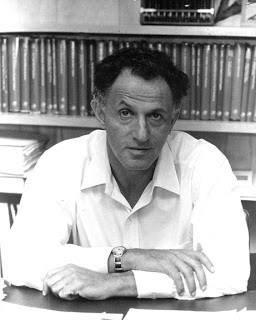
In 1965, the mathematician
Richard Belman proposed to use the method of retrospective analysis to create databases of chess and checkers endgame decisions (the final part of the game). He gave an algorithmic description of the application of the method of reverse induction for constructing an optimal strategy in games.
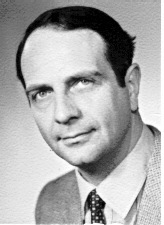
In 1970, the mathematician
Thomas Strohlein defended his doctoral dissertation on chess completion. The fact is that in chess there are special cases when there are few pieces on the board. In such cases, for a small number of figures, we can solve the problem by inverse induction.
So, we are closer to understanding how the AI wins a man in chess. The computer does not need to make endgame calculations every time - just look at the already calculated result in the database and make the perfect move. Endgame databases, including all possible arrangements of figures, search in the opposite direction, starting with the positions where one side has already received a pat or checkmate, and ending with a specific position on the board.
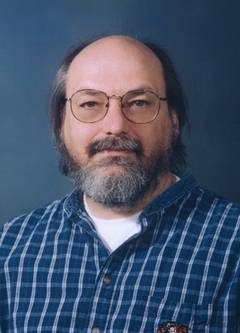
In 1977,
Ken Thompson , known for his contribution to the creation of the C language and UNIX, introduced one of the world's first endgame tables for all five-figure endings.
In 1998, our compatriot, programmer
Yevgeny Nalimov created an extremely efficient generator of chess endings, thanks to which, given the increase in computer productivity, by the early 2000s all six-figure endings were counted.
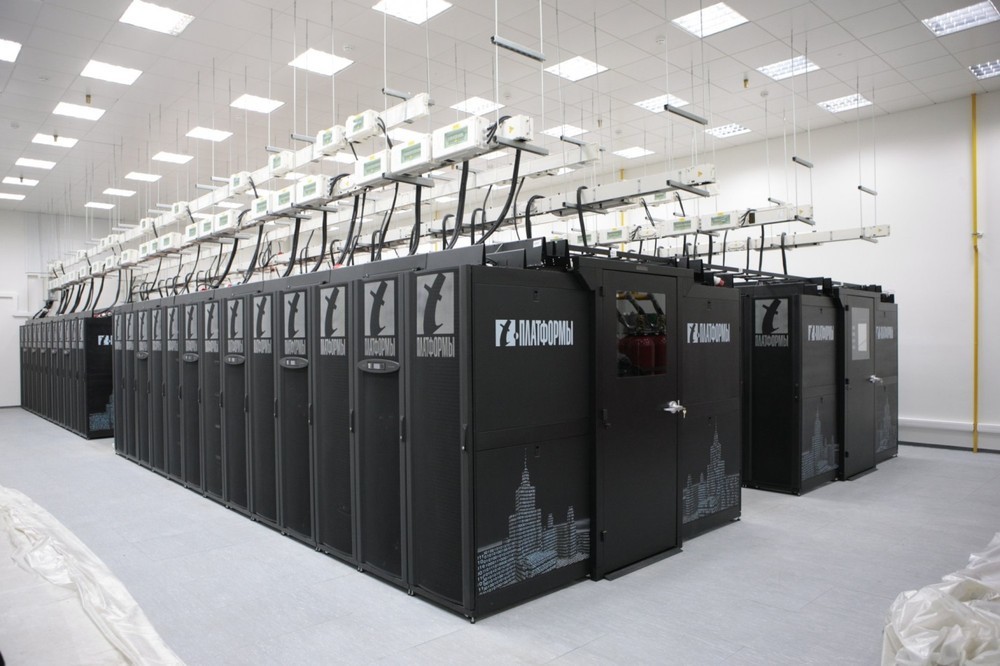
This chapter will be closed by a photograph not of a person, but of a computer. 2012 . . «», «» IBM BlueGene/P.
, . , 50 . , , 50 . , , .
(Federation Internationale des Echecs — ) 50 75 , .
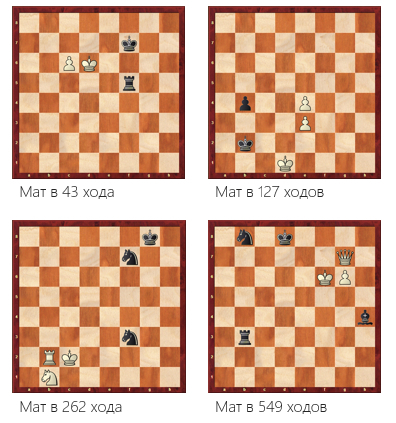
, 75 , . — 549 .
50 . , ? , .
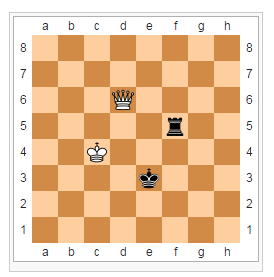
— « ». , . — , , . .
, , — . . — , . , , , .
-20.1967 .
. ,
.
, «
». 1974 . . , , .
, — . ,
.
, - .. —
, - , , , . - , , . , , .
—
. , , , . , - , , , , . , .
—
. . , - — 900 , .
29 2007 . () . ( «») «» , , .
— , . The size of its search space is 5 × 10
20 . , 18 ( 50 200) 10
14 .
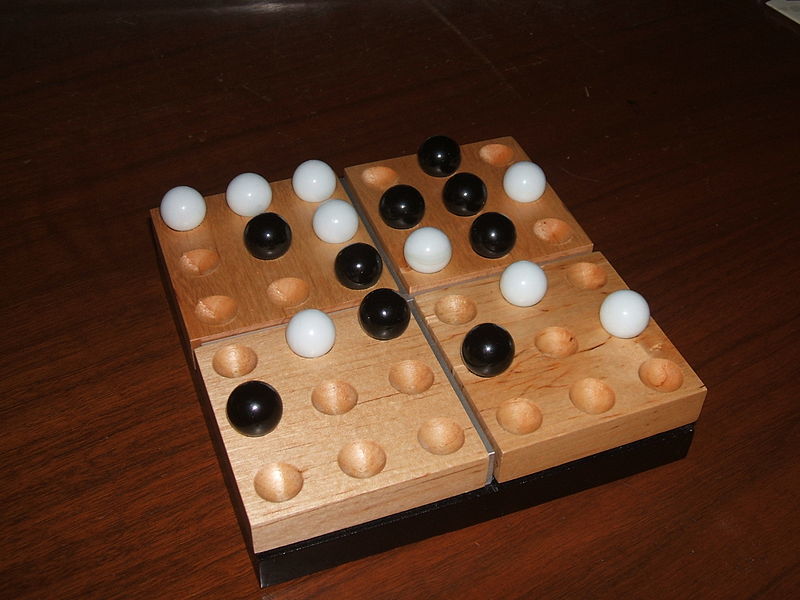
2016 . 16 .
. , : 3 009 081 623 421 558. Edison Cray, - (NERSC), «» . 98 304 .
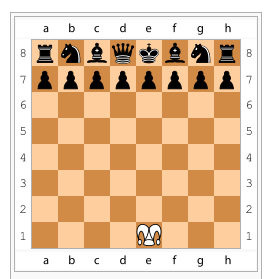
, «
», , — . , . , .
DRAMA:
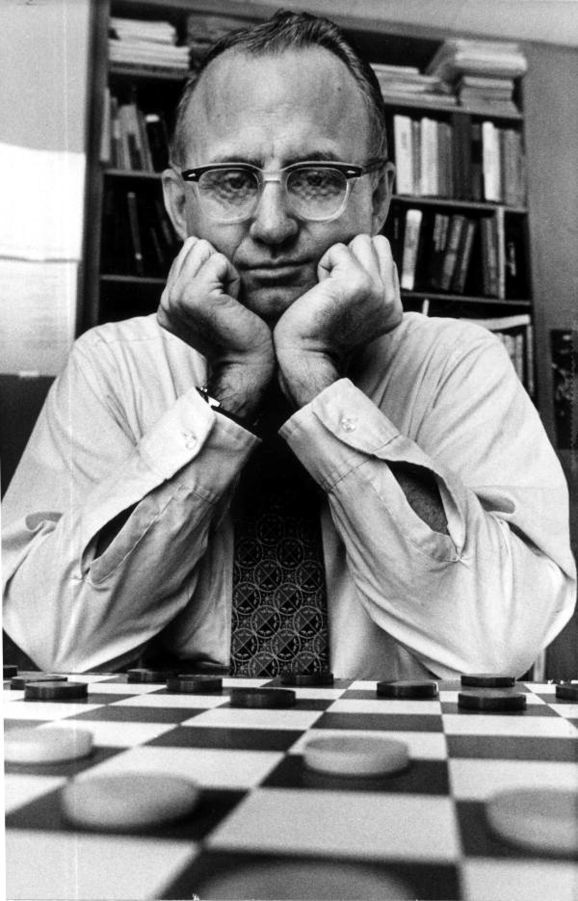
30 . 45- .
1992 . «». 4:2 33 . , , , 64 .
— . . 1995 . «» , . 32 . .
., , 1997 .
Deep Blue . : Deep Blue 200 , 1—3 . 20 .
IBM , , , . — .
Deep Blue , : , — . , — .
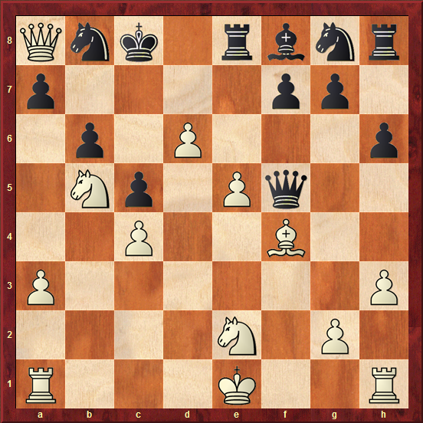
In fact, it is not. — . Deep Blue, , 356 . 35 , — 35 , — ..
. , 2008 . 2015 . 27 , 26 — . , . , . . -, . , —
Stockfish — . , — Deep Blue — .
. , . , , , 3—5 . , , . , , .. — .
, , : ? 86 150 . 1 . . , , 150 (1,510
17 ) . , «» , .
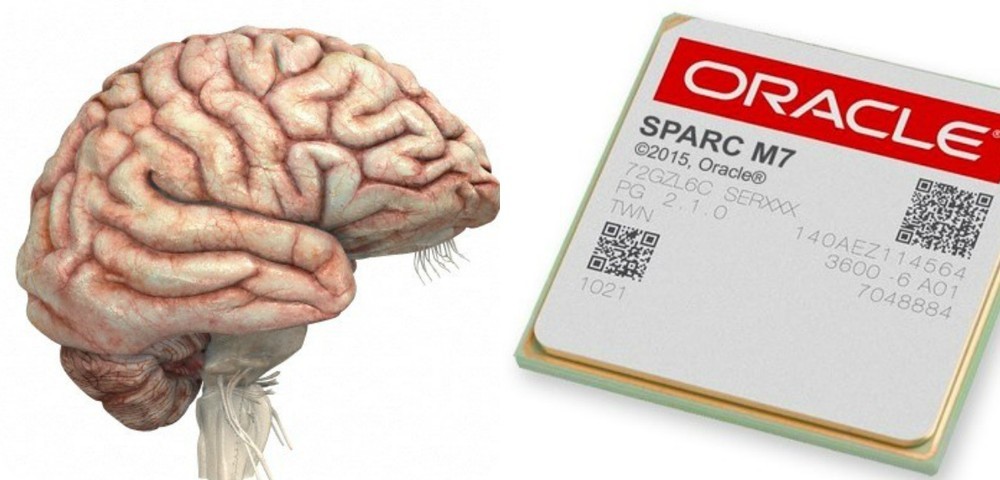
(
Sparc-M7 ) 10 . (
FPGA ) 20 . 210
9 , 1,510
17 . , — . Sparc 4,3 . : — 0,1 , — 1 , «» » — 10—100 ( 1000 ).
, -, , . , , , . , , , , , .
Neural networks
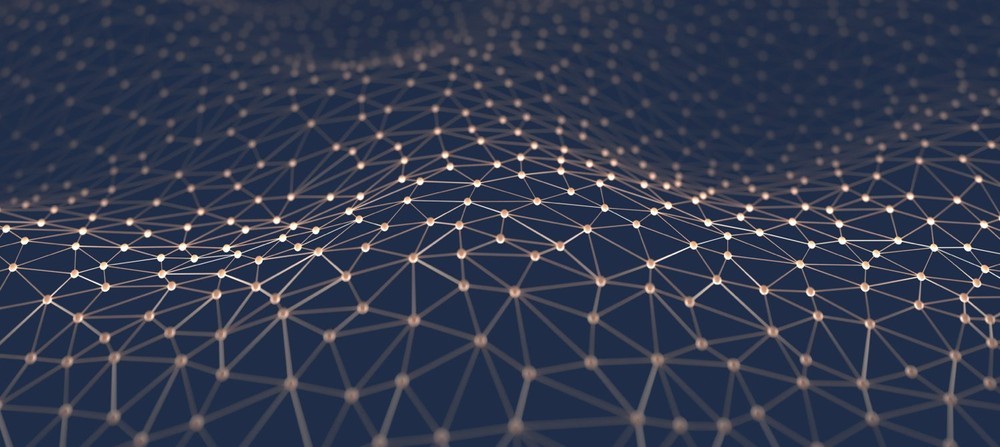
— «» «». «» — , , , , .
«» , , . , . hacking — , .
, , . , , .
, ,
. , , - . , . , , , , , . , .
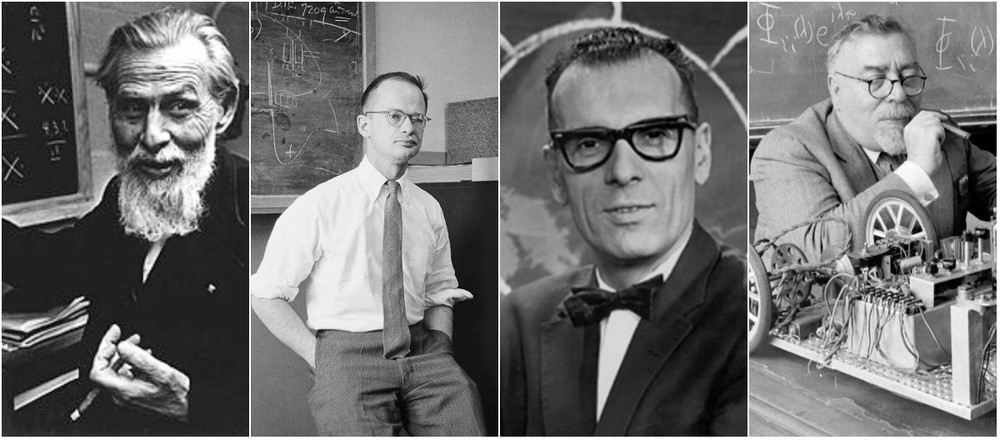
, ,
,
,
1940- . — .
:- , ;
- ;
- ;
- , ;
- W i — .
In many ways, the relationship between these people laid some basic things in science. For example, we do not call neural networks cybernetic systems, although McCallack and Pitts began to work under the authority of
Norbert Winner , the founder of cybernetics. The bohemian lifestyle of McCallack and Pitts and Winner’s parties didn’t like it very much. It is believed that she strongly influenced the discord between scientists. Pitsu was worth his career, and, quite possibly, his life - he later drank himself. But they did not make an ideological argument from a quarrel. Their paths diverged for personal, not scientific reasons.
What do we know about neurons? The first surface basis of a
connectoma is the neurons connected by axons and dendrites, the outgoing and incoming processes. The place of contact between the axon and the dendrite is the synapse. Like much in nature, sigmoid is present here as an adder. The signals from the neuron propagate along the outgoing processes, enter the next neuron, summed up and a certain amount is transmitted further along the process, converting synapses through certain threshold functions.
MARK 1 - the world's first neurocomputer, created in 1958 by Frank Rosenblatt .Perceptron is the first artificial neural network embodied in practice by Frank Rosenblatt. The scientist decided that there is no time to wait until the electronic machines are fast enough to simulate the work of the neural network on the Von-Neumann architecture. He began to make neural networks of "sticks and scotch" - a sort of array of electronic neurons and heaps of wires. These were small neural networks (up to several dozen neurons), but they were already used in solving some practical problems. Rosenblat was even able to sell the devices used in the analysis of some arrays to several banks.
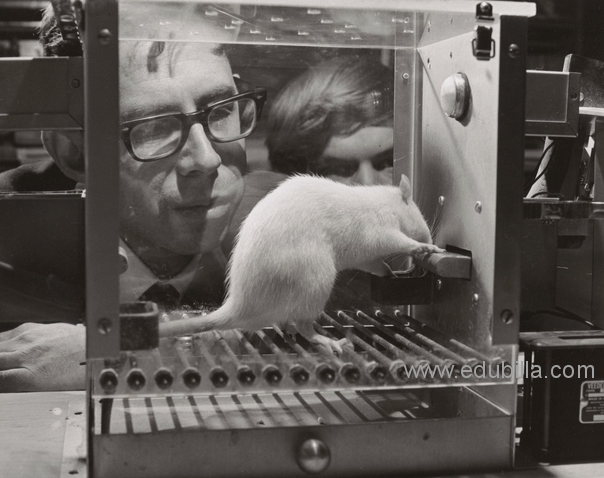
Since 1966, he became engaged in unusual things. For example, he taught rats to go through a labyrinth, trained them, then killed them. Then he extracted their brains, ground them into a gruel, and fed this gruel to the next generation of rats. Thus, he checked whether this would improve the performance of the next generation of rats during the passage of the mazes.
It turned out that the brain eaten by rats does not help, although they love this kind of food. This was one of the strong arguments in favor of the fact that information is stored in the connection of the brain, and not in its individual cells and components. Oddly enough, in those years there were specialists who believed that knowledge could exist inside cells.
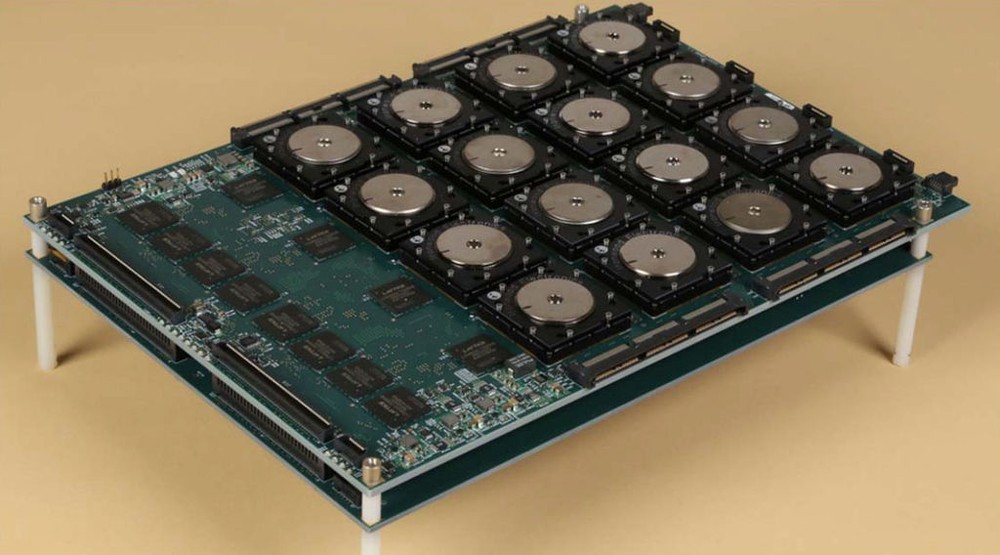
Half a century after Rosenblatt’s inventions, we now have a
TrueNorth neuromorphic processor, created by IBM for DARPA. The chip contains more than 5 billion transistors and simulates the work of up to 1 million model "neurons" and up to 250 million connections between them ("synapses"). IBM says that a real emulator of the human brain will assemble from processors of this kind.
IBM created an entire institute for the development of this technology, and developed a special programming language for neural networks. The neural network is very inconvenient to emulate on von Neumann architecture. In a neural network, each neuron is simultaneously a computing device and a memory cell.
If we start spinning the neuron matrix inside the von Neumann machine, then we are faced with a shortage of computational cores compared to the number of memory cells. The cycle of calculations of all synapses turns out to be long. Although the task is very well parallelized within a single cycle, you will still need the input values you received in the previous calculation step in the next step. Maximum parallelization efficiency can be achieved when you have as many processing cores as synapses.
The neuromorphic processor is an attempt to make a special piece of iron for the fast calculation of neural networks. And since neural networks are now quite actively used both in pattern recognition, and in data analysis, and in many other places, the appearance of such an element base will allow increasing the efficiency by several orders of magnitude at once.
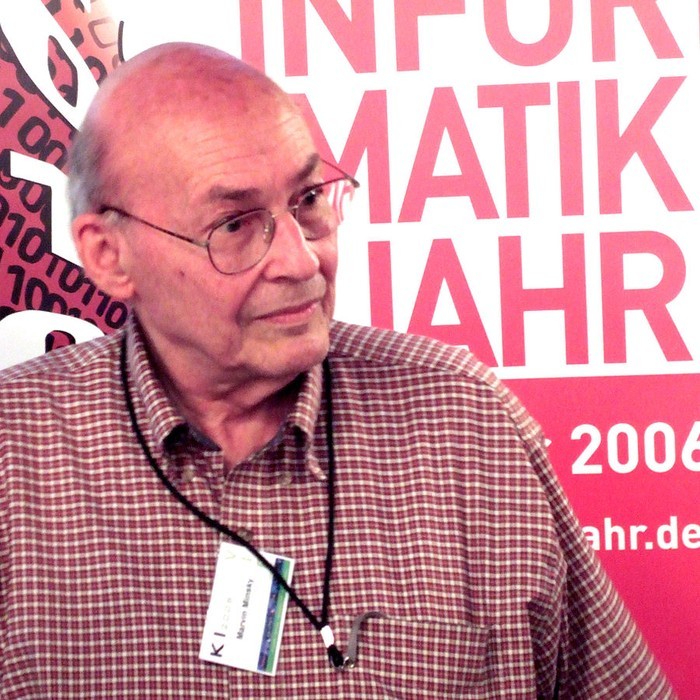
Let's go back to the 1970s. After the success of Rosenblatt,
Marvin Minsky became a prominent specialist in the field of AI development. Together with his colleague Papert, he wrote the book Perceptrons, which showed the fundamental limitations of perceptrons. Indirectly, this work contributed to the onset of the “winter” of AI (the 1970s and the 1980s), when there was a lot of doubt about the possibilities of creating a full-fledged AI based on neural networks.
Interest in neural networks returned in the early 1990s, when it became clear that the relatively small neural networks available at that time were already capable of solving some problems no worse than the classical methods of
nonlinear regression .
Leon A. Gatys et al./arXiv.orgIn 2016, news about the achievements of neural networks began to appear almost every week. A team of scientists from Germany
developed an artificial neural network that allows you to "synthesize" an image from two independent sources: one of them gives only content, the other - only style. Neural network has
learned how to realistically color black and white images. The neural network was
taught to put geotag on pictures of food and cats. The neural network
began to calculate funny pictures. Well, and so on.
New breakthrough: AlphaGo
Go game has always been a tough nut to AI. Skeptics argued that go programs would never win, or they would win very soon. Go is more complicated than chess by
10,100 times — namely, it is so many times more possible positions of stones on a standard 19 × 19 board than in chess.
The breakthrough was achieved in the AlphaGo program thanks to the
Monte-Carlo-search algorithm for a game tree, controlled by two neural networks trained on the basis of a large array of games of professional go players. First, AlphaGo won the European champion, then defeated Lee Sedol, one of the strongest (possibly the strongest) players in the world.
However, the real revolution that led to the victory of the program over a man in go, occurred much earlier, when
convolutional neural networks began to be used.
In go it is very difficult to come up with the structure of the evaluation function, which, without going over, will be able to assess with good quality how good the position is. It is necessary to somehow evaluate the patterns, the structures formed by the chips on the board. It was not possible to find a sufficiently good solution until convolutional neural networks appeared that were well coping with this task.
Image: The Atlantic .In AlphaGo, one of the neural networks (value network) is used to evaluate positions at the terminal nodes of the search tree, the other (policy network) is designed for smart selection of candidate moves. A small Monte-Carlo brute force tree is also used. Each node is considered 1-2 moves, the average branching ratio - less than two. Due to this, it became possible to count a sensible tree with a very limited number of options, and due to this it is possible to find a really strong move in a position.
At first, AlphaGo trained on batches of the strongest go players. 160 thousand games from 29.4 million positions were used as input for training. From the moment when it began to play as well as ordinary people, the body for training the neural network or for building parameters of the evaluation function was automatically generated. The program began to play with itself, adding new games to the training set.
Threats and prospects: a bad, good and very good scenario
Image: TechRadar .Different fears associated with artificial intelligence are caused by several problems. People are afraid of themselves, are afraid to make a mistake when creating an AI - suddenly it will not behave exactly as we want. Imagine such a hypothetical situation: the car is explained for a long time that there are edible and inedible mushrooms, and poisonous mushrooms are deadly for humans. Next, the car is asked: Does any person can eat any mushrooms? “Of course, any,” replies the car. “But some only once in a lifetime.”
If the people making up the algorithms of AI have allowed bugs or missed erroneous data into the training sample, the behavior of such AI can be quite unexpected. And to trust such a decision making system is dangerous. Someone will be irresponsible, at the same time someone will be stupid, and we will get a script in the spirit of "Terminator".
Familiar fears? However, this story is not directly related to AI, we are only talking about the fact that people do not trust themselves. We are not only afraid that the developed mind will be evil. He may turn out to be kind, he will want to bring people good, but he will see this “good” in his own way.
Humanity with a developed AI has two good scenarios for the development of events and one global bad. The global bad is that our technological power is growing too fast. In the XIX century. with a strong desire to arrange an apocalypse, mankind would not have had enough strength to annihilate all life on the planet, even with total insanity.
In the middle of the twentieth century. distraught leaders of leading countries and several military commanders in key posts would embody a scenario of self-destruction. To do this, just take a few dozen 200-ton thermonuclear bombs and drop them at the equator - the globe will split in half.
Image: Plague Inc.In the XXI century. technological strength is such that a large number of defendants are not required to implement the apocalyptic scenarios. Suppose a biochemical laboratory creates a killer virus. We do not know how to quickly defend against viruses. It is possible that it is not so difficult to make an infection that will be at the same time very virulent, having the appropriate fragments from the influenza virus, and at the same time 100% lethal, having received a part, for example, from Ebola. To implement such a project, a few dozen educated madmen will suffice.
The story is a little far-fetched, but this kind of scenario becomes all the more likely the more we master the technology. Despite the fact that our technologies are becoming more powerful, biologically and socially, we are not far from the Upper Paleolithic - our brain remained about the same.
Assessing the facts of global threats, you quickly come to the conclusion that before the advent of a universal AI, humankind is able to commit global suicide using simple and effective technologies. It is impossible to criminalize scientific and technical progress, but progress itself provides us with the tools to control existing threats.
If our civilization slips through this bottleneck, two good scenarios will open. Scenario one: we are a dead-end branch of evolution. The mind, surpassing us, will develop further, and we will remain funny monkeys living in their own world with their passions and interests. In this world, we will have nothing to share with intelligent machines, as there is nothing to share with chimpanzees and gorillas. On the contrary, we like to play with primates, to make funny films about them. Perhaps the AI will shoot documentary series about people and show off its budding.
The second scenario: from a certain moment the level of technology development will exceed the complexity of the organization of our body. And from this point on, the difference between our machines, our tools, and ourselves will inevitably wear out. We will interfere with our own organism, expand our neural networks with artificial additions, we will add postneocortex to the brain.
Imagine that we glue an artificial neural network with our brain. An artificial neural network, for example, is 10 thousand times larger than our neural network. It is known that the brain is a plastic thing, and even injuries of the brain, knocking pieces out of it, often do not lead to loss of personality, self-identification. Therefore, it is possible that the meat brain can be cut and thrown away from the system of glued brain with an artificial network without harming the personality.
Maybe it will be different: we will begin to repair the brain with the help of nanorobots, and we will communicate with the artificial neural network through remote channels. In any case, the person will become part of the metasystem. And to determine where artificial intelligence ends and begins natural, at some level of technology development, it will become simply impossible.
Last words: AI as a person
Image: Machine OverlordsIs self-awareness possible in artificial intelligence?
Mind as a child of evolution appeared not just like that, but as a mechanism for adapting to the environment, allowing it to survive and produce healthy offspring. Scientists express the opinion that consciousness is a kind of reflection of our psyche. Our psyche discovers itself and begins to consider it as an independent object. In this regard, it is possible that self-awareness is only a side effect of adaptation.
Mirror neurons can be involved in the development of new skills through imitation.In the course of evolution, human
mirror neurons appeared, which are used by social organisms to predict the behavior of other individuals. That is, we need a model somewhere in our head, which we can apply to another person in order to predict his behavior. At some point, the mirror neurons began to “examine” themselves as well.
If this theory is correct, then similarly, through side effects, the development of artificial intelligence, which inevitably develops self-awareness, is possible. If we need the AI to predict the behavior of other AI or people, he will have a model for predicting actions, and sooner or later he will apply this model to himself. Awareness is an inevitable consequence of the development of a complexly organized intellectual system.
The opposite point of view says that the AI will remain to some extent instinctive, that is, it will act without self-awareness and self-reflection, and nevertheless will begin to solve a very wide range of intellectual tasks. To some extent this is happening right now: a modern chess program plays better than people, but it does not have self-awareness.
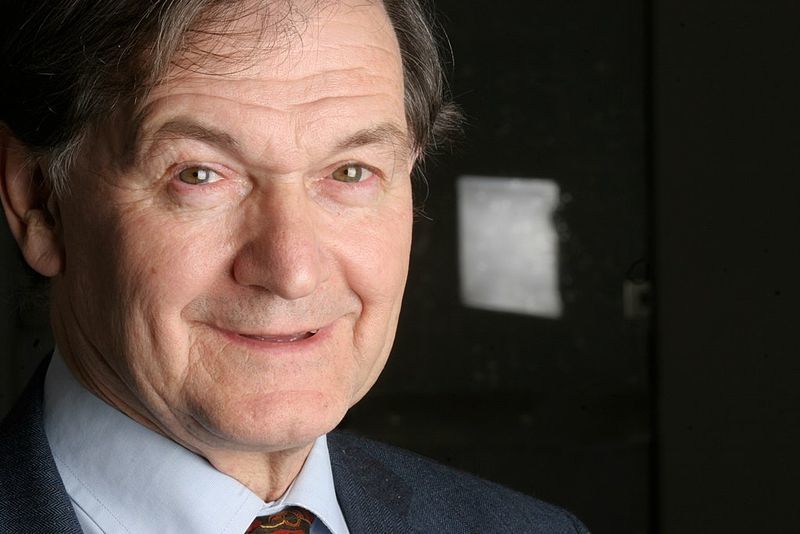
As for copying consciousness to an electronic carrier, this story is also not all clear. English scientist
Roger Penrose argues that human consciousness is not algorithmic, and therefore cannot be modeled using a conventional computer such as a
Turing machine . According to Penrose, consciousness is associated with quantum effects, which means that
the Heisenberg uncertainty principle will not allow an accurate snapshot of the electronic activity of the brain. Therefore, our consciousness, copied to the machine, will not be an exact copy. Most researchers disagree with this opinion, considering that the thresholds of signals in the brain are very far from the quantum level.
The support vector machine learning method was proposed by Vladimir Vapnik in 1995.In conclusion, recall the neural networks. In fact, neural networks in practice in machine learning are models for the lazy. In the absence of the opportunity to correctly set up the support vector (
SVM ) method or build a
random forest , we take a neural network, drive the training sample into it, get some characteristics of the model, etc.
Neural networks are not universal and they have a lot of problems - they are prone to noise, to overclassing in certain situations. For a number of tasks, we know better methods. When solving chess problems, we do not use neural networks, but machines still play better than humans. This is a good example of creating a system that solves some problems better than the brain on a slower primitive element base using algorithms that are not used by the brain.
Is it possible to make a universal AI on non-neural networks? Of course! We just start with a
bionic approach . First we try to improve what we have now, and then we will look for more advanced methods.
Whichever way we choose, you should not perceive it as a human drama on the opposition of man and computer. Under each post about the victory of AI in the next game, someone will write a comment about SkyNet and Judgment Day. Why is losing a car perceived as losing a person? This is the winning person, not only the one who played at the board, but the one who created the AI. And this is a much more important achievement: one of them learned to move figures, and the other was one step ahead and wrote a program that moves figures to victory.
PS If you liked the article, then the next time we will talk about in-depth training with reinforcements, about the program’s decision to kill or save a person, about self-driving cars and whole cities controlled by AI.