Welcome to our readers on the pages of the blog
iCover ! At the International Solid State Circuits Conference (ISSCC-2016) International Conference in San Francisco (ISSCC-2016), which took place in early February, the development team from MIT (Massachusetts Institute of Technology) demonstrated the current prototype of the Eyeriss chip, which was created as a conceptual solution to recreate the capabilities of neural network algorithms. in a wide range of low power devices.
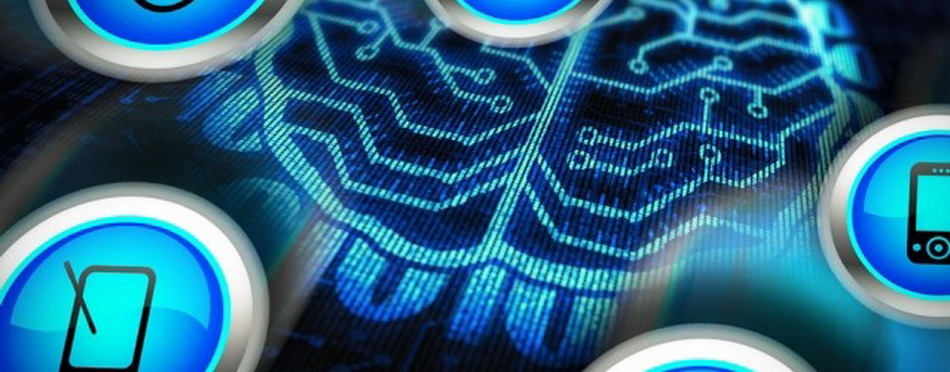
One of the objective reasons why artificial neural networks have not been properly developed in our smartphones or tablets is the lack of a compact power source of sufficient power. After all, the so-called brain-like, “brain-like” artificial intelligence systems, at least in the form in which they are represented by modern technologies, depend in their work on powerful multi-core processors, which consume an incredible amount of energy in comparison with our brain. Imagine such solutions at the user-class device level, in any case, until recently, it was not possible. At the same time, the idea of ​​“miniaturization” of artificial intelligence stirs the minds of developers quite a long time ago and, as it turns out, already brings its own tangible results.
Neural networks were in the center of attention of scientists from the first days of research on artificial intelligence, but in the 1970s, they were somewhat forgotten. In the last decade, technologies associated with the use of the capabilities of neural networks have been studied at the level of “deep learning” programs.
')
“Deep learning has many uses, such as object recognition, speech, or face recognition,” note Vivienne Sze and Emanuel E. Landsman, associate professor at the Massachusetts Institute of Electrical Engineering and Computer Science, whose group developed new chip. “Now, neural networks are quite complex and, they work mainly on powerful chips. Imagine that you can transfer this functionality to your mobile phone or embedded device and then process huge amounts of information without using a Wi-Fi connection. Processing large amounts of data on your smartphone will avoid delays due to the exchange of data with the network, which, in turn, will allow many applications to work much more efficiently. And besides, the proposed solution will provide new quality protection of confidential information. ”
Neural networks, as a rule, are implemented on the basis of multi-core graphics processors (GPU). At the International Conference in San Francisco, MIT researchers presented a new 168-core chip designed to implement artificial intelligence algorithms based on neural networks. In comparison with a mobile GPU (it is not indicated in comparison with which one), the processor demonstrated 10 times greater efficiency, which allows the use of a custom mobile device for local launch of powerful algorithms of artificial intelligence without the need to send data for cloud processing. Highlights of the development are reflected in the MIT
press release of February 3, 2016.
The new chip, named by the developers of Eyeriss, can be widely used on the Internet of Things, wearable electronics, self-driving vehicles, production equipment, and even in agriculture, helping with the solution and coordination of current tasks. With artificial intelligence algorithms on board, mobile devices will be able to make decisions at the local level, providing the user with a ready result, rather than a set of raw data from the Internet, as a guide to action. And, of course, one of the applications of local neural networks is their use in creating autonomous robots of various purposes.
Divide and rule
Neural networks, as a rule, have a multilayer structure and each layer contains a large number of processing nodes. At the initial stage of processing, data comes and is distributed between the nodes of the lower layer. After processing the obtained data by each of the nodes, the result is transmitted for processing to the nodes of the next layer. At the output of the last layer, the result of solving the problem is formed. Accordingly, to solve large-scale problems according to the described algorithm, considerable computational resources will be required.
The requirements initially presented to the chip by the developers themselves put them in a fairly rigid framework: on the one hand, the solution should be energy efficient, on the other hand, they should operate with simple blocks of information. Finally, the chip must have the ability to simulate various types of neural networks with regard to the current tasks assigned to it. All these requirements were successfully implemented in the processor Eyeriss.
The chip, developed in the MIT laboratory, is an already formed neural network localized at the level of a 168-core processor, which in the future can be integrated into mobile devices.
The key to Eyeriss efficiency is minimizing the frequency of data exchange between the cores and external memory banks, the operation associated with high power consumption and time costs. While the cores of traditional GPUs are tied to one common memory bank, each Eyeriss core has its own memory. In addition, the data before being sent to the neighboring nuclei undergo a compression procedure.
Another advantage of the implemented algorithm is the ability of the cores to “communicate” with each other directly, bypassing the “middleman” in the form of a system memory bus. This is a critical feature to simulate the work of the "convolutional neural network" (CNN). All computational work necessary for pattern recognition and speech is performed locally in Eyeriss, without the need to access network resources, which allows the device to function effectively even in the absence of an external network.
Finally, another advantage of Eyeriss is the principle of the “smart” distribution of separate computational problems between cores within the framework of a single solvable problem. In its local memory, the kernel must store not only the data processed by the nodes, but also the data describing the nodes themselves. In order to maximize the performance of the data processing process, as well as to load Eyeriss the maximum amount of data from the main memory, the algorithm for distributing data of both types is optimized with a specially designed chip for this purpose in real time taking into account the features of the current neural network.
At the International Solid State Circuits Conference event in San Francisco, the development team, using the capabilities of the “user-level” Eyeriss chip, demonstrated the implementation of the pattern recognition algorithm within a local neural network. A similar task - noted in the press release - was implemented earlier, but at the level of state projects of the most modern of the created neural networks.
“This work is very important because it demonstrates how effectively embedded processors for in-depth study are able to provide the required power and performance optimization and bring complex computing processes from the cloud to mobile devices,” said Mike Polley, senior vice president of the Mobile Processors Laboratory at Innovations Lab Samsung, complementing: “In addition to the innovative hardware solution complex, MIT’s research clearly demonstrates how the embedded core can be made useful for handler applications using AlexNet and Caffe standard network architecture. "
Eyeriss project funding, starting with the creation of a unit based on the MIT laboratory, was partially funded by the American defense department DARPA. Not surprisingly, the first who responded to the processor's announcement with impressive editorial material was the well-known military analyst Patrick Tucker. The new Eyeriss processors installed on mobile devices of US soldiers, in his opinion, will be able to solve the most complicated computational problems associated with processing huge amounts of information without connecting to a common network.
Thus, at present, the US Air Force receives daily, from drones hovering over Afghanistan, up to 1,500 hours of HD video and up to 1,500 photos of super high resolution. Moreover, all this endless stream of information has to be visually analyzed by operators in the old manner, since the existing computer software is not able to distinguish a peasant wandering a stick along a mountain path from a terrorist with a launcher for guided missiles. To solve such problems, methods of machine learning based on representational training were originally developed.
Eyeriss processors are ideal for installation on unmanned military drones, as they allow for intelligent processing of an array of images and videos using “deep learning” technologies directly on board the aircraft. In this case, the separated useful data could be sent directly to the combat units operating in the specified region, bypassing the center for analyzing operational information.
Brief summaryDuring the experiments, the Eyeriss chip demonstrated an energy efficiency level that is ten times higher than the performance of modern mobile graphics chips. In this case, with its help, it is technologically possible to ensure the operation of algorithms of artificial intelligence on devices that have compact dimensions - from smartphones and tablets - to wearable electronics. The delays created by the networks when exchanging data for such a processor are minimized, since most of the computations the chip can perform locally. On the basis of Eyeriss, it will be possible to create not only all sorts of "smart devices", but also robots with a certain degree of independence in making decisions.
Specific time intervals during which Eyeriss will be able to transform into a commercial product and in all power to reveal their capabilities at the consumer level, the developers from MIT have not yet called. A certain optimism inspires involvement in the development of NVIDIA's leading specialists and the lively interest of senior Samsung researchers.
A source
Dear readers, we are always happy to meet and wait for you on the pages of our blog. We are ready to continue to share with you the latest news, review materials and other publications, and we will try to do everything possible so that the time spent with us will be useful for you. And, of course, do not forget to subscribe to
our headings .

Our other articles and events