In some tests, in-depth training already works better than human experts.
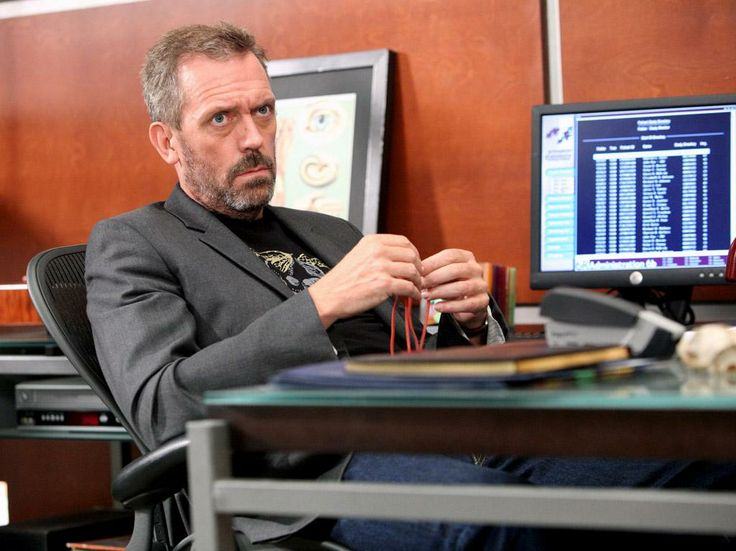
One evening last November, a 54-year-old woman from the Bronx arrived at the emergency room at Columbia University Medical Center complaining of a severe headache. She was blurring everything in front of her eyes, and her left hand was numb and weak. The doctors examined it and recommended to do a CT scan of the head.
A few months later, on the morning of one of the January days, four future radiologists gathered in front of a computer screen on the third floor of the hospital. There were no windows in the room, and it was only illuminated by the on screen. Angela Lignelli-Dipple, head of the neuro x-ray department of the university, stood behind the interns with a pencil and tablet. She taught them to understand CT scans.
“When the brain is dead, it's easy to diagnose a stroke,” she says. “The trick is to diagnose a stroke even before too many nerve cells die.” Strokes usually occur due to blockage of blood flow or bleeding, and the neurogenetic examiner has only 45 minutes to diagnose so that doctors can intervene in the process - for example, to eliminate an emerging clot. “Imagine that you are in the emergency room,” Linel-Dipl continued, increasing the intensity of an imaginary situation. - Every minute another part of the brain dies. Lost time is a lost brain. ”
')
She glanced at the clock on the wall, with a second hand running across the dial. “So what’s the problem?” She asked.
Usually strokes are asymmetric. The supply of blood to the brain forks left and right, and then is divided into streams and tributaries on each side. A blood clot or bleeding usually affects one of the branches, leading to a deficiency on one side of the brain. When the nerve cells are cut off from the blood flow and begin to die, they swell a little. In the picture, clear boundaries between anatomical structures may be blurred. In the end, the fabric shrinks, leaving a dried shadow on the image. But this shadow is still visible in the pictures a few hours or even days after a stroke, when the possibility of intervention has already been missed. “Up to this point,” Linel-Dipl told me, “there is a hint in the picture,” a warning about an impending stroke.
Images of the brain of a woman from the Bronx cut the skull from the base to the top, like slices of watermelon, cut from top to bottom. Interns quickly flipped through the layers of images, as if flipping through the pages of a notebook, calling the anatomical structures: cerebellum, hippocampus, central lobe, striatum, corpus callosum, ventricles. Then one of the students, whose age was approaching 30 years old, stopped at one of the pictures and showed a pencil on the area from the right edge of the brain. “There is something spotty here,” he said. “The borders look blurry.” From my point of view, the whole image looked spotty and blurred - like a porridge of pixels - but he clearly saw something unusual.
“Blurred?” Lineli-Dipl supported him. "Describe, please, more."
Intern began to mumble something. He stopped, as if sorting out the anatomical structures in his head, weighing the possibilities. “It’s just heterogeneous,” he shrugged. - I dont know. It looks weird. ”
Linel-Dipl opened the next set of CT images taken after 24 hours. The area, the size of a grape, which pointed out to the ordinator, was dull and bloated. A few follow-up shots taken a few days apart told the rest of the story. A gray wedge-shaped area appeared. Shortly after entering the emergency room, a neurologist tried to clear the clogged artery with a drug that dissolves clots, but it arrived too late. A few hours after the initial picture, she lost consciousness, and she was transferred to the intensive care unit and intensive care. Two months later, she was still in her room. The left side of her body, including her arm and leg, was paralyzed.
Linelle-Dipl and I went to her office. I had to study the process of education in the hospital: how do doctors learn to make diagnoses? Can machines learn this?
My dedication to diagnostics began in the fall of 1997 in Boston, when, after medical school, I went to practice. To prepare, I read a classic medical textbook that divided the process of making a diagnosis into four distinct phases. First, the doctor uses the patient's medical history and physical examination to gather facts about her complaints or condition. Information is then critically reviewed in order to create an exhaustive list of potential causes of problems. After this, questions and preliminary checks help to exclude some hypotheses and strengthen others - this is so-called. "
differential diagnosis ". Such parameters as the prevalence of the disease, medical history, risks, possible effects on a person are taken into account (as the famous [American] medical aphorism says, “the hoofs of hoofs emit horses rather than zebras” [“
zebra ” in US medicine is the slang name of an exotic diagnosis in the case when a more simple diagnosis is most likely]). The list narrows down: the doctor clarifies his assumptions. In the final phase, laboratory tests, X-ray or CT scan confirm the hypothesis and diagnosis. Variants of this step-by-step process were reproduced in medical textbooks for decades, and the idea of ​​a diagnostician, methodically and tediously moving from symptoms to reason, was inspired by generations of medical students.
But the real art of diagnosis, as I learned later, is not so straightforward. My mentor in medical school was an elegant
New England resident in polished moccasins and with a stiff accent. He considered himself an expert diagnostician. He asked the patient to demonstrate a symptom — for example, a cough — and then lean back in his chair and roll adjectives in his tongue. “Rattling and metallic,” he could say, or, “low, with a strum,” as if describing a vintage bottle of Bordeaux. Everyone seemed to be the same kashli, but I played along with him: “Rattling, aha,” - feeling like an impostor at a wine tasting.
A cough specialist could immediately narrow down the diagnosis. “Sounds like pneumonia,” he could say. Or: "wet wheezing with congestive heart failure." Then he gave a stream of questions. Has the patient recently gained weight? Did he have contact with asbestos? He asked the patient to cough again and leaned over, listening with a stethoscope. Depending on the answers, he could issue a new series of possibilities, as if intensifying and weakening synapses. Then, with the pomp of an itinerant magician, he proclaimed a diagnosis: “heart failure!”, And wrote out tests to prove his case. Usually he was right.
A few years ago, Brazilian researchers studied the brains of expert radiologists to understand how they give their diagnoses. Did these experienced diagnosticians address the mental “rule book”, or did they use “pattern recognition and non-analytical reasoning”?
Twenty-five radiologists were asked to evaluate X-rays of the lung, and at that time they were monitoring their brain activity with an MRI. (There are many wonderful recursions: to diagnose the process of diagnosis, you need to take a photo of people studying the photo). Before the eyes of the subjects flashed X-rays. Some showed common pathological lesions - for example, a brush-shaped pneumonia shadow, or a gray opaque wall of fluid accumulated behind the membrane. The second group of images included contour drawings of animals. In the third there were the outlines of the letters of the alphabet. Radiologists were shown these pictures randomly, asking them to speak out loud the name of the damage, animal or letter as quickly as possible, while at that time the MRI device was tracking their brain activity. On average, radiologists required 1.33 seconds for the diagnosis. In all three cases, the same part of the brain was “lit up”: a wide delta of neurons near the left ear and a strip in the form of a moth at the posterior base of the skull.
“Our results support the hypothesis that when a doctor recognizes a damage known to him, the same process works, which is connected with the naming of objects,” the researchers concluded. The definition of damage is very similar to the definition of an animal. When you recognize a rhino, you are not considering alternative candidates to discard the wrong options. You do not mentally combine a unicorn, an armadillo and a small elephant. You recognize the rhino as a whole - as an image. The same thing happens with radiologists. They did not weigh, did not remember, did not differentiate. They just saw the famous object. For my mentor, wet rales were as familiar as the famous melody.
In 1945, the British philosopher
Gilbert Ryle gave a fateful lecture on two types of knowledge. The child knows that the bike has two wheels, tires are filled with air, and you need to ride it, rotating the pedals in a circle. Ryle called such knowledge - actual, propositional - "knowledge of what." But to learn how to ride a bike, you need to turn to another area of ​​study. The child learns to ride a bike, falling from him, balancing on two wheels, overcoming the pits. Ryle called this knowledge — hidden, experimental, skill-based — knowledge of how.
It would seem that these two types of knowledge are interdependent: knowledge of facts can be used to deepen experimental knowledge, and vice versa. But Ryle warned against the temptation to believe that “knowing how” can be reduced to “knowing what” - one cannot learn to ride a bicycle according to a rule book. Our rules, he argued, make sense only because we know how to use them: "Rules, like birds, must be alive before you can make stuffed animals out of them." One afternoon, I watched my seven-year-old daughter climb a hill on a bicycle. For the first time, she was stuck on the coolest part of him and fell. The second time she leaned forward, at first almost imperceptibly, and then more and more, and transferred the weight back when the curvature of the hill began to decrease. But I did not teach her the rules by which you need to climb this hill on a bicycle. I think that when her daughter will overcome the same hill, she will not teach her either. We meet a few rules according to which the universe works, but we give the brain to independently comprehend all the others.
Some time after the meeting with the radiology interns, I spoke with Stephen Heider, a young man who noticed signs of a stroke on a CT scan. How did he detect this damage? Was it “knowledge of what” or “knowledge of how”? He began to talk about the learned rules. He knew that the strokes are usually one-sided, that they lead to the “graying” of the tissue, that the tissue sometimes swells a little, which makes the anatomical borders disappear. “There are places in the brain where the bloodstream is especially vulnerable,” he said. To determine the damage, you need to look for its signs on one side and look for them not to be on the other.
I reminded him that the photograph had a lot of asymmetrical details, ignored by him. This picture on the left had a lot of gray squiggles - perhaps artifacts associated with movement, or chance, or brain changes that preceded a stroke. How did he concentrate only on this area? He was silent for a moment to gather his thoughts. “I don’t know - partly it was subconsciously,” he said at last.
“That's what happens — everything falls into place — when you grow up and study as a radiologist,” Linel-Dipl tells me. The question is whether a machine can “grow and learn” in the same way.
In January 2015, computer science specialist
Sebastian Trun [Sebastian Thrun] became interested in the mystery of medical diagnostics. Troon, who grew up in Germany - thin, with a shaved head and a sense of comic; he looks like a strange hybrid of Michel Foucault and Mr. Bean. A former Stanford professor who ran the AI ​​laboratory there, Trun went out of there to lead Google X, to lead the work on creating self-learning robots and robo-mobiles. But he found an addiction to studying medical devices. His mother died of breast cancer when she was 49 - as much as Truna is now. “Most patients with cancer have no symptoms at first,” Troon told me. “My mother didn't have them.” When she went to the doctor, her cancer had already metastasized. I became obsessed with the idea of ​​detecting cancer at an early stage - when it can still be cut with a knife. And I thought that, can the machine learning algorithm help in this? ”
What is stronger, body or mind?
- Get up.
- Not.Early attempts to make automatic diagnoses tried to work with a textbook and with accurate knowledge. Take an electrocardiogram showing the electrical activity of the heart as lines on a page or screen. Over the past 20 years, such systems have often used computer interpretations. The data program is fairly straightforward. Characteristic waveforms are associated with various problems — atrial fibrillation or vessel blockage — and rules for recognizing these waveforms are embedded in the device. When the machine recognizes a waveform, it marks the heartbeat as "atrial fibrillation."
In computer-aided
mammography , it is also becoming common. The recognition software highlights the suspicious areas and the radiologist examines the results. But usually this software uses a rule-based system, and identifies it as suspicious damage. These programs do not have a learning mechanism: a machine that saw three hundred X-rays is no better than the one that saw four. These limitations became clearly visible after a 2007 study that compared the accuracy of mammography before and after the introduction of devices with computer diagnostics. One would expect a sharp increase in accuracy - but it turns out that the effect was more complex. The number of biopsies increased many times. And the detection of small invasive tumors - just those that tend to identify oncologists - has decreased. More recent studies have shown an increase in problems with false tumor detection.
Troon was convinced that he would be able to surpass the first-generation devices in accuracy, moving from rule-based algorithms to students — that is, from making a diagnosis based on “knowing what” to setting using “knowledge of how.” Increasingly, such learning algorithms with which Trun works use a computer strategy called a neural network, inspired by the work of the brain itself. In the brain, nerve synapses are strengthened or weakened through periodic activation; Digital systems are trying to achieve this result through mathematics, correcting the "weights" of connections to obtain the desired output. The most powerful of the networks resemble layers of neurons, each of which processes the input data and sends the results to the next layer - hence the name “depth learning”.
Troon began with skin cancer; Specifically, keratinocyte carcinoma (the most common class of cancer in the US) and melanoma (the most dangerous type of skin cancer). Is it possible to teach the machine to distinguish between skin cancer and its more favorable state - acne, rash, mole - by scan of the photo? “If dermatologists are capable of this, then the machine should handle it,” argued Troon. “Perhaps the machine will do better.”
Usually, dermatologists are taught to recognize melanoma based on a set of rules, denoted by the mnemonic abbreviation ABCD. Melanomas are often asymmetric (A), their borders (border, B) are uneven, their color (color, C) is uneven and variegated, and the diameter (D) is usually more than 6 mm. But when Troon looked at examples of melanomas in medical textbooks and on the web, he found instances to which none of these rules were applicable.
Trun, an associate professor at Stanford, formed a team with two of his students, Andre Esteva and Brett Kuprel. Their first task was to create a training set: an extensive collection of images used to teach machine recognition of malicious education. In the Internet, Esteva and Kuprel found 18 repositories of images with skin lesions, classified by dermatologists by type. These galleries contained nearly 130,000 images — acne, rashes, insect bites, allergic reactions and cancers — that dermatologists divided into almost two thousand diseases. There was also a set of two thousand skin lesions for which pathologists made a biopsy, in connection with which they were diagnosed with high accuracy.
Esteva and Kuprel began to train the system. They did not program the rules in it, they did not teach it the ABCD system. They fed the neural network pictures and their diagnoses. I asked Troon to describe what this network was doing.
“Imagine a classic program trying to identify a dog,” he says. - The programmer would have to enter into her a thousand “if / then” conditions: if she has ears, muzzle, hair, and if it is not a rat, etc. - to infinity. But the child learns to recognize dogs differently. First he sees dogs, and he is told that they are dogs. . , – , , . – , – . , , . , . , . , – , ». , .
2015 , , : 14000 , , ( ). : , ? 72% ( – «/», ). , , : 66% .
, , , , . , : . « -», –
, Nature .
« , , », – . «» . , , - ( , ), . , . – , , – , , .
, . , - , . , . , , : « . , ».
: « », , , . , « » . , . , . « , – . – , . , ».
« » . . – . , – ? , . . . . , , , . , , . , , ? . , , : , .
, . , . . , , , , . , : . – , .
. , , , ? ? , : . , - . , ? , . , , . , .
« , – , , , . – ? , . , . . , . -, – , – . ? , – . . , , ». , . , .
, , . – , . ; 70-, .
«, , , Wile E. Coyote. , . ».

. « , , – . – , . - , ».
: « ». -, , «/». , . - , , . , .
. « , , , , », – . : « , , . ». , , – . , « ».
, .
. , , , , , , « – , ». , . «, , – . – , , , , , . , . ».
. , . , . « , – , - – . , ? ? . - , , , . , ».
( ) , . IBM Watson Health , , DeepMind . , .
, . , . , , —
, . « , – , . – – . . ?»
, , 51- . [Lindsey Bordone], , 49 . 10 . 60 , . .
. – « , , » – .
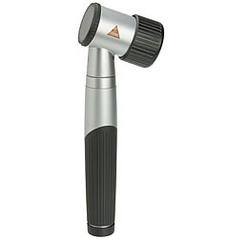
30 . . , .
« ?» – .
. «», – .
« , – . – . , , . - ? ?»
« , », – . , .
« , – . – ». .
, , . , . « », – , .
, . «» . . 20 , , , . , .
« », – . .
: , , . , -, , . , : , , , . , , , 200 000 . 130 000 . , , .
. « , , – . – , ».
, . , , ? , : , ? « ». , – . , –
, . , , . , ?
. , . , , . . - . , , , .
, , . , «» «», : , , , . . , . ? ? ? - ? ?
« » « » – , . : « ».
. , . ,
; . , – , – , , . . , , – , . - – , , «». – ?
« - », – . . : « - , . , . – ». , .
, , , , . . . ? ? , (, – , ). . , – « », « » « » – , , , , , ?
, . «, , – . – , , . ? , , , . ? ? , - , ? , ?»
. , , . « , – . – , . , ».
, «» diagnosis, dia «» + gnosis «». «» – . , . , , .