Cellular utilization indicators for office workers, the unemployed, retirees, teachers and students. For example, the neural network has defined such a specific indicator for office employees as the length of outgoing calls.Thanks to social networks and cellular metadata, specialists have obtained a convenient and fairly accurate tool for studying society. People publish some information on social networks consciously, and some of the important data is given out involuntarily. For example, the analysis of anonymous cellular metadata shows traffic on the roads, the speed of cars, traffic jams, passenger traffic of public transport. These are fairly logical data mining options. But a group of scientists from the Telenor Group Research, the MIT Media Lab, the Flowminder Foundation and the Stockholm School of Economics
found a very unusual option . Researchers have shown that the cellular logs can predict ... employment. The unemployed and representatives of 17 more kinds of occupations are determined quite accurately.
According to scientists, this is the world's first study of this kind, when the unemployed or a person's profession is calculated at the individual level with the help of in-depth training on the logs of the cellular network. Previously, researchers tried to predict only the total unemployment rate for mobile data, but not the profession of specific people.
Researchers emphasize how important it is to have accurate statistics on the unemployed in society. This is an important economic indicator for the study of the labor market, which helps build economic forecasts and manage the economy. Although the surplus of free labor resources is pleasant for employers, but the state usually aims to reduce unemployment below a certain level.
')
Finding accurate information about the unemployed is hard. This requires periodic large-scale polls. In some countries, the actual unemployment rate is much higher than the rate officially registered with the bodies of employment services.
Such surveys take a lot of time and resources. For example, in the United States, indeed, such surveys are constantly conducted in households and publish statistics. In less developed countries, due to the high cost of surveys, they are conducted irregularly and with insufficient coverage. Now, researchers have found an alternative option that fundamentally solves the problem.
Even homeless people now have cell phones, so metadata analysis provides almost complete coverage of the working-age population in many countries (in general, more than 50% of the world's population has mobile phones). Sociologists can only dream of such coverage. Engineers have shown that cellular network metadata provides sufficient spatial coverage and accuracy in time to conduct effective data mining.
Scientists have used unprecedented coverage and accuracy of cellular network metadata in past years to calculate auxiliary indicators of poverty, illiteracy, population estimates, migration, and the spread of viral epidemics. At the individual level, cellular network metadata helps to predict
a person’s socioeconomic status ,
income level ,
demographic characteristics, and
personality type . Now it came to the status of employment.
The researchers applied the depth learning model on a massive dataset from a poor South Asian country. For training the program used the results of a survey of 200,000 people in households, conducted by a local mobile operator. People reported on their employment status and profession, choosing from 18 kinds of occupations.
In addition, for in-depth training mobile logs were taken over a period of six months, 76,000 of these 200,000 people surveyed. The information was carefully anonymized, the program did not have access to telephone numbers, subscriber names, the contents of conversations and text messages. Naturally, having such access in the style of SORM, you can profile people with almost one hundred percent accuracy. In this case, the task was set to conduct scientific research without violating human rights.
Researchers identified three types of mobile logs: financial (amount of account replenishment, communication expenses, frequency of replenishment, ratio between maximum and minimum amount of account replenishment, etc.), movement in the area (home area / cell, entropy of places of visit, inertia radius sections, number of places visited, etc.) and social functions (number of conversations with a contact, entropy of contacts, duration of a conversation, number of SMS, volume of Internet traffic, number of MMS, number and duration of video calls onkov, frequency of use of additional services of the operator, etc.).
The model with all variables was tested on several algorithms, including GBM (gradient boosted machines), RF (random forest), SVM (support vector machines) and kNN (K-nearest neighbors). As a result, a multilayered neural network was compiled. More precisely, 18 models for each type of profession (including the unemployed). Training and testing was carried out with the distribution of data 75% and 25%.
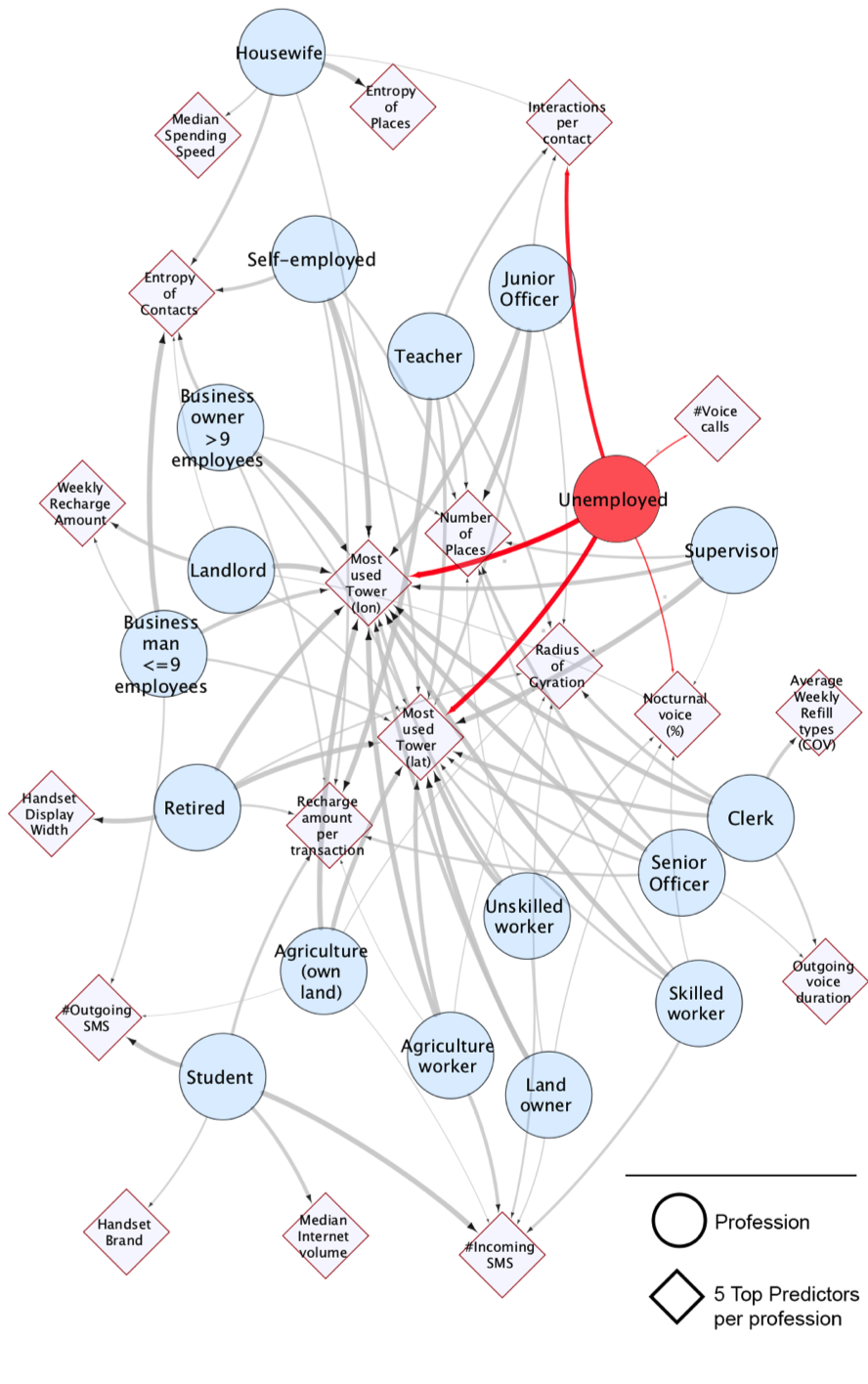
The results showed that the neural network best defines office employees (clerks). On the use of mobile communication, they give out themselves with an accuracy of 73.5%. The most difficult is to identify qualified employees by the cellular network metadata (61.9%). The average for all professional groups was 67.5%. Like office workers, the unemployed are also very well defined, with a probability of 70.4%.
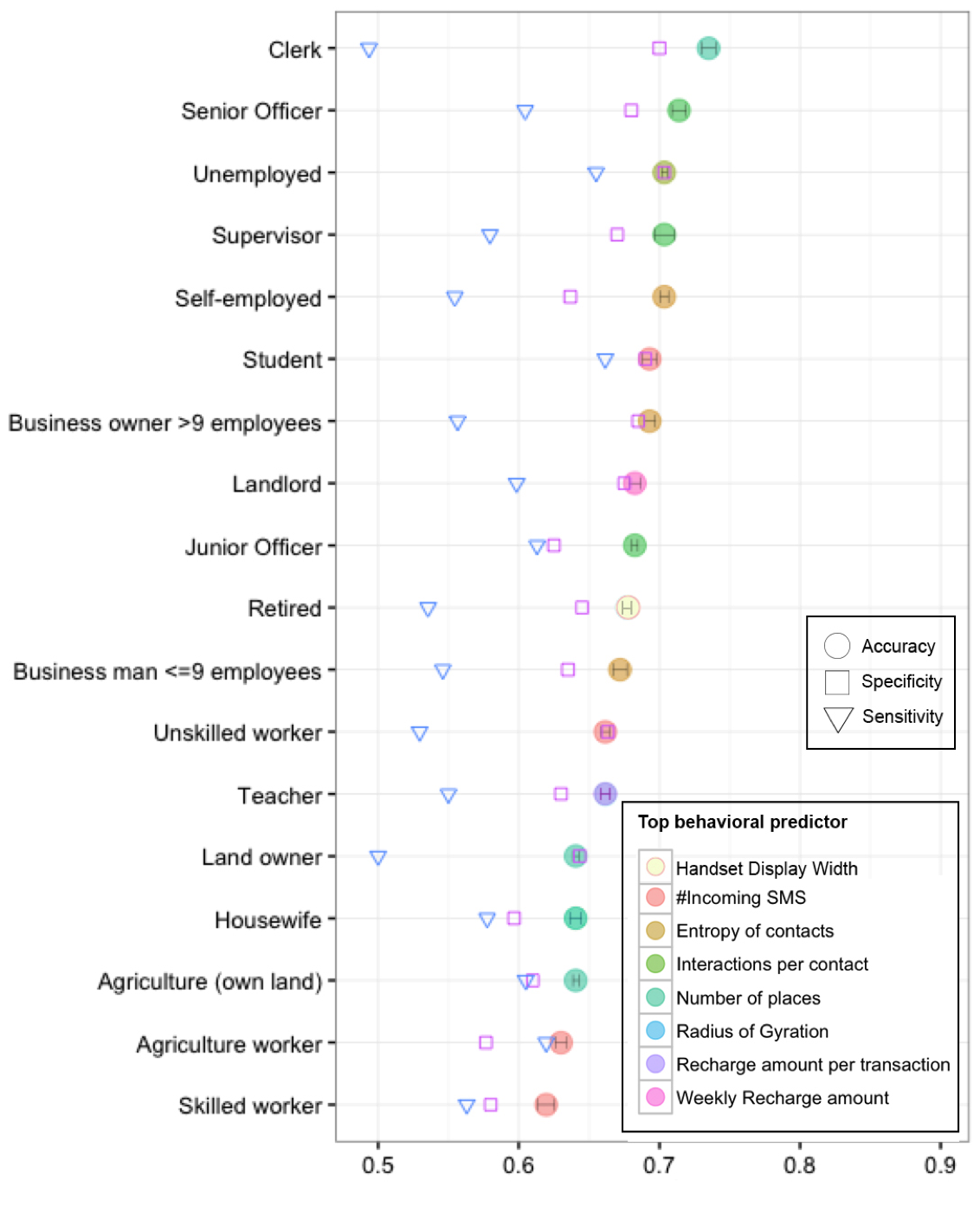
Surely this scientific work will find application in practical programs for data mining. Incidentally, if someone receives information about changes in the level of employment for 1-2 weeks before the appearance of official statistics in the United States, it can make good money on the stock exchange. So employees of mobile operators have an option for a small “hack”, if they are not afraid to go to jail for using insider information.
In countries where they introduced or intend to impose a tax on parasites, such a neural network will help replenish the budget. Will identify unregistered unemployed who are hiding from the tax office. For the intended profession of a person, you can still target advertising.
The scientific article was
published on December 12, 2016 on the arXiv preprints website (arXiv: 1612.03870) and has not yet received an expert assessment.