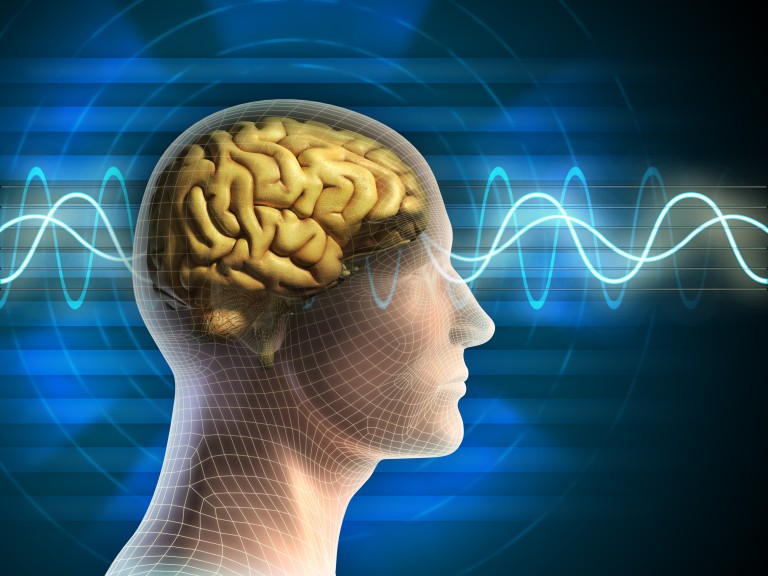
With age, a person’s cognitive abilities are reduced. Neurobiologists have long known that this decline correlates with physical changes in the brain. You can see the first signs of aging or even determine the age of the brain using MRI, and the difference between the age of the brain and the chronological age of a person helps to identify neurodegenerative diseases in the initial stages.
This analysis is often very long, since MRI data needs to be processed in detail before you start automated aging recognition processes: remove the skull bones from the image, separate the gray and white matter and other tissues, and remove image artifacts, including various ways to smooth the image. The whole treatment may take more than 24 hours, and this is an obstacle for doctors who hope to take into account the age of the patient’s brain in the process of clinical diagnosis. Scientists from
King’s College London found a way to speed up the process.
')
Giovanni Montana and his team trained a convolutional neural network (
CNN ) to measure brain age using raw data from an MRI scanner. The
depth learning method takes only a few seconds and can give doctors an accurate picture of the age of the brain while the patient is in the scanner.
The method is a standard technique of deep learning. Montana and his team used brain tomograms from over 2,000 healthy people between the ages of 18 and 90 years. None of them had neurological diseases that could affect their brains. Therefore, the age of the brain must correspond to the age of participants in the experiment in the passport.
Each scan is a T-1 weighted image. In such an image, fluids in the brain appear darker in color, the white matter is lighter, the gray matter gray, and the fat bright color. Such a scan is usually carried out for studies of the chest, musculoskeletal system, abdominal cavity and brain.
An example of neuroimaging input data for use in age prediction models. A - gray matter in axial projection, B - white matter in axial projection, C - “raw” or minimally processed T1-weighted images.In each picture, scientists noted the chronological age of the patient. The team used 80% of the image to train a convolutional neural network to determine a person’s age, taking into account the scan of their brain. Another 200 images went to check the performance of the network. Finally, they tested the neural network in 200 images, which she had not yet seen, to determine how well she coped with the determination of the age of the brain.
At the same time, the team compared the results of the in-depth approach with the traditional method for determining the age of the brain. It requires significant image processing for identification, including white and gray matter in the brain, followed by statistical analysis - regression based on
Gaussian processes .
The results were quite interesting. Both in-depth training and statistical analysis accurately determine the chronological age of patients when data is entered for analysis in
preprocessed form . Both methods somehow work with an error of less than 5 years in determining the exact age of the brain.
The accuracy of CNN and regression based on Gaussian processes in the analysis of 200 images. Chronological age (X axis), estimated brain age (Y axis). And - results of CNN, B - results of regression on the basis of Gaussian processes, C - results of CNN in the analysis of raw T1-weighted images. r is the correlation coefficient of brain age and chronological age from all graphs.However, in-depth study demonstrates its clear superiority in analyzing the initial MRI data, where the network determines the correct age with an average error of 4.66 years. Unlike CNN, the standard regression method based on Gaussian processes coped worse with the problem: the average error was almost 12 years.
Moreover, in-depth training takes only a few seconds compared to the 24 hours of preprocessing required for the standard method. The only data processing required for a convolutional neural network is to determine the sequence of image orientation and the size of the
voxel between them. For doctors, this is important: after the introduction of appropriate software, data on the patient's brain age can be obtained while it is still in the scanner.
The team also compared images taken using different scanners to show that the method can be applied to scans collected from different machines in different parts of the world. In addition, they compared the age of the brain of 62 female twins to show how the age of the brain is related to genetic factors. In the course of this study, scientists have identified a high heritability of the level of aging of the brain, which inevitably decreases with age. It is believed that environmental factors become more significant over time, and here scientists have identified a promising direction for future research.
The outcome of Montana and his team has the potential to influence how physicians determine the correct diagnosis. There is considerable evidence that diseases such as diabetes, schizophrenia and traumatic brain damage correlate with faster aging. Thus, a quick and accurate measurement of brain aging can significantly affect how physicians deal with these diseases in the future.
Scientific work published on arXiv.org (
Arxiv: 1612.02572 [stat.ML])