Almost all the achievements of AI known to you are connected with the breakthrough of thirty years ago
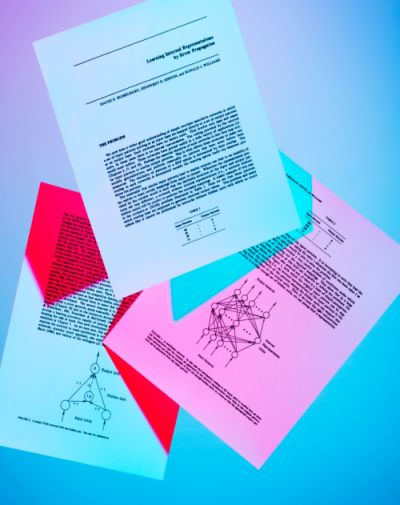
I am standing in a room that will soon become the center of the world — or just in a very large room on the seventh floor of a glittering tower in downtown Toronto. Jordan Jacobs, the co-founder of this place suits me the trip: the emerging Institute "Vector", which opens its doors in the fall of 2017, and seeks to become the global epicenter of artificial intelligence.
We are in Toronto, because
Jeffrey Hinton is in Toronto, and Jeffrey Hinton is the father of “depth learning” (GO), the technology behind current enthusiasm for AI. “After 30 years, we look back and say that Jeff was Einstein in AI, in-depth learning, in what we call AI,” says Jacobs. Among the researchers at the forefront in the field of civil defense, Hinton has more quotes than the following three combined. His undergraduate and graduate students launched AI labs at Apple, Facebook and OpenAI; Hinton himself is the lead scientist on the Google Brain AI team. Practically all the achievements in the field of AI of the last decade — translations, speech recognition, image recognition, games — in one way or another are based on Hinton's work.
The Vector Institute, a monument to the take-off of Hinton's ideas, is a research center, where companies from the USA and Canada — such as Google, Uber, and Nvidia — will finance attempts to commercialize AI technologies. Money poured into him faster than Jacobs had time to ask them; Two co-founders studied companies in the Toronto area, and it turned out that the demand for specialists in AI is ten times higher than the capacity to produce them in Canada. "Vector" is the starting point of a global attempt to mobilize around civil society: make money from technology, train people in it, hone it and apply it. Data centers are being built, skyscrapers are being filled with startups, a whole generation of students is joining the region.
The impression of being in the “Vector” office, empty and echoing, awaiting filling is as if you were at the beginning of something. But what is strange about GO is how old its key ideas are. Breakthrough work by Hinton, made jointly with David Rumelhart and Ronald Williams, was published in 1986. The work understood the technology called "reverse error propagation" (ORO). ORO, in the words of John Cohen, a specialist in computational psychology at Princeton, "this is what all deep learning is based on - literally everything."
')
In fact, the AI ​​today is GO, and GO is ORO, and this is surprising, considering that ODP is more than 30 years old. It is worth understanding how this happened - how the technology can lie in the standby mode for so long, and then lead to such an explosion. Understanding the history of ORO, you will begin to understand the current development of AI, and in particular, the fact that perhaps we are not at the beginning of a revolution. Perhaps we are at its end.
Proof of
The walk from the Vektor Institute to Hinton’s office on Google, where he spends most of his time (he is now an honorary professor at the University of Toronto) is a kind of live city advertising, at least in the summer. You can see why Hinton, who was born in Britain, moved here in the 1980s after working at Carnegie Mellon University in Pittsburgh.
Going outside, even in the business center near the financial district, you feel as if you were in nature. I think it's all about the smell: it smells of wet humus. Toronto was built in a wooded ravine, and it is called the “city in the park”; during urbanization, local authorities imposed strict restrictions to maintain tree growth. On the way to the city, its outskirts seem covered with cartoon lush vegetation.
Toronto is the fourth largest city in North America (after Mexico City, New York and Los Angeles), and the most diverse: more than half of its inhabitants were born outside Canada. This can be seen when walking around the city. People in the technology quarter look different from San Francisco residents — young white guys in sweatshirts — but more internationally. There is free medicine, good public schools, people are friendly, the political climate is stable and leaning to the left; all this attracts people like Hinton, who says he left the United States because of the
Iran-Contra scandal. This is one of the first topics that we raise at the first meeting, shortly before lunch.
“Most people at Carnegie Mellon University believe that it was wise for the US to invade Nicaragua,” he says. “They thought the country belonged to them.” He says that he recently had a big working breakthrough: “A very good young engineer is working with me,” a woman named Sarah Sabur. She is from Iran, and she was denied a work visa to the United States. The Google office in Toronto took her to him.
Hinton, 69 years old, has a kind, thin, British-type face of a “
big good giant ”, with thin lips, large ears and a prominent nose. He was born in Wimbledon, in England, and his voice in conversation sounds like the voice of an actor reading a children's book about science: you can hear curiosity, charm and a desire to explain everything in it. He is funny, a little showman. All the time he is talking, because, as it turned out, it hurts him to sit. “In June 2005, I sat down and it was a mistake,” he tells me, after a pause, to explain that he has problems with the intervertebral disc. Because of this, he could not fly, and earlier that day he had to carry a surfboard-like device with him so that he could lie on it in the dentist’s office, which worked with a cracked tooth root.
In the 1980s, Hinton was an expert on neural networks, a simplified model of a network of neurons and brain synapses. But at that time everyone decided that neural networks are a dead end in AI research. And although the very first neural network, Perceptron, which he began to develop in the 1950s, was called the first step towards human-machine intelligence, the 1969 book from the authors from MIT, Marvin Minsky and Seymour Papert, called “Perceptrons”, mathematically proved that such networks can perform only the simplest functions. Such networks had only two layers of neurons, input and output. Networks with a large number of layers between incoming and outgoing in theory could solve a huge number of tasks, but no one knew how to train them, so in practice they were useless. And besides a bunch of dodgers like Hinton, Perceptrons caused people to put a cross on neural networks.
Hinton’s breakthrough in 1986 was that he showed how an ODP can train a deep neural network — that is, a network with many layers. But it took another 26 years until the increased computing power was able to take advantage of this discovery. In
2012, Hinton, with
two students from Toronto, showed that deep neural networks trained with ODP overtake the best image recognition systems. Depth learning began its spread. For the outside world, the AI ​​woke up in one night. For Hinton, this was a long time favor.
Reality distortion field
A neural network is often drawn in the form of a club sandwich, in which layers are superimposed on each other. The layers contain artificial neurons, obtuse small computational units that can be excited — like a real neuron is excited — and transmit the excitation to other neurons with which they are connected. The excitation of a neuron is represented by a number, such as 0.13 or 32.39, denoting its magnitude. On each of the connections between neurons, there is another critical number that determines the excitation of which force can be transmitted through it. This number simulates the power of synapses between the neurons of the brain. The greater the number, the stronger the bond, the more excitement is transmitted to others.

One of the most successful applications of deep neural networks is pattern recognition, reminiscent of the scene from the series Silicon Valley, in which the team creates a program that can tell if there is a hot dog on the image. Such programs exist, and ten years ago they would have been impossible. To make them work, you first need to get the picture. Suppose this is a small black and white image of 100x100 pixels. You feed this image into your neural network by adjusting the excitation of each neuron on the input layer so that it is equal to the brightness of each pixel. This is the bottom layer of the club sandwich: 10,000 neurons (100x100) representing the brightness of each pixel of the picture.
Then you connect this large layer of neurons with another large layer of neurons above it, which, say, several thousand are recruited, and they, in turn, with several thousand more neurons, and so on, in several layers. Finally, in the upper layer of the sandwich, the outer layer, there are only two neurons - one means “there is a hot dog”, the other means “no hot dog”. The idea is to teach a neural network to excite only the first of these neurons, if there is a hot dog in the image, and only the second one if it is not there. ORO, the technology on which Hinton built his career, is the method for accomplishing this task.
An ORO works surprisingly simply, although it works best on large data sets. That's why big data is so important for AI - that's why Facebook and Google are so eager for them, and therefore the Vector Institute decided to locate its office on the same street as the four largest Canadian hospitals and developed a partnership agreement with them.
In our case, the data appear in the form of millions of images, some of which have hot dogs, and some do not. The trick is that the presence of hot dogs on the images is marked. When you first create a neural network, the connections between neurons are random weights - random numbers that determine the size of the transmitted excitation. Everything works as if the synapses of the brain are not yet configured. The goal of the ORO is to change these weights so that they make the neural network work: so that when you give it an image of a hot dog to the lower level, the neuron “is a hot dog” of the upper level is excited.
Suppose you take the first training picture, which depicts a piano. You convert the intensity of pixels of a 100x100 image into 10,000 numbers, one for each neuron in the lower layer of the network. The excitation propagates upward through the network according to the forces of the connections between the neurons in the adjacent layers, and as a result it turns out to be in the last layer, where there are only two neurons. Since this is an image of a piano, ideally a neuron “eat hot dog” should turn out to be zero, and “no hot dog” should get a large number. But let's say it did not happen. Suppose a neural network is mistaken. ODP is a procedure for shaking up the strength of each connection in a network so as to correct the error for this training example.
You start with the last two neurons, and see how badly they make a mistake: what is the difference between what values ​​for excitations should be and what they were. After that, you check all the connections that lead to these neurons — those that are in the lower layer — and calculate their contribution to the error. You continue to do this until you reach the very first set of links, at the very bottom of the network. At this point, you know exactly what contribution each individual compound made to the error, and in the last step you change all the weights in the direction that best reduces the entire error. The technique is called “back propagation of errors” because you propagate the error back (or down) across the network, starting with the output layer.
The amazing fact is that when you do this with millions or billions of images, the network starts to very well recognize if there is a hot dog in the picture. What is even more surprising is that the individual layers of these image recognition networks begin to “see” images in much the same way as our visual system sees them. That is, the first layer can begin to recognize faces, in the sense that its neurons are excited when there are faces, and are not excited when they do not exist; a layer higher can begin to recognize face sets, such as corners; a layer sees higher forms; the layer above sees already such things as an opened or closed bun, in the sense that it has neurons responsible for each of the variants. The network organizes itself into hierarchical layers, despite the fact that nobody programmed it for this.
This thing conquered all. The point is not that neural networks simply classify images of hot dogs or anything else: they are able to build ideas about ideas. In the case of text, this is even better seen. You can feed the text from Wikipedia, billions of words into a simple neural network, and train it so that for each word it displays a large list of numbers corresponding to the excitation of each neuron in the layer. If you imagine that these numbers are coordinates in a complex space, then you will, in fact, look for a point (in this context - a vector) for each word in this space. Then train your network so that the words appearing close to each other on the pages of Wikipedia have similar coordinates - and voila, something strange happens: words with similar meanings will appear close to each other in this space. That is, the words “crazy” and “crazy” will have little different coordinates, like the words “three” and “seven”, and so on. Moreover, the so-called. vector arithmetic allows you to subtract the vector "France" from the vector "Paris", add the vector "Italy" and be somewhere near the "Rome". And it works without prior explanation for the network that Rome belongs to Italy just like Paris to France.
“This is amazing,” says Hinton. “This is shocking.” Neural networks, one might say, take certain entities — images, words, call recordings, medical data — and place them in a high-dimensional vector space, where the distance between entities reflects some important feature of the real world. Hinton thinks the brain is doing the same thing. “If you want to know what thought is,” he says, “I can express it for you with a set of words. I can say „John thought:“ Opanki. ” But if you ask: “What is this thought? What does this mean for John - to give birth to this thought? ”This doesn’t mean that an opening quote appeared in his head, then an“ Opank ”, then a closing one — or even some version of these characters. A certain large pattern of neural activity appeared in his head. "A mathematician can display large patterns of neural activity in vector space, where the activity of each neuron corresponds to a number, and each number corresponds to the coordinate of a very large vector. From Hinton's point of view, this is the idea: dance vectors.
Jeffrey hintonIt is not by chance that the main AI institute in Toronto was called “Vector”. The name came up with Hinton himself.
Hinton creates some kind of distortion of reality, an atmosphere of confidence and enthusiasm in which you feel that there is nothing that a vector could not do. After all, look what they have already achieved: cars that behave themselves, computers that recognize cancer, cars that instantly translate what was said out loud into another language. And look at this charming British scientist telling about gradient descent in high-dimensional spaces!
Just leaving the room, you remember: these systems of deep learning are still quite stupid, despite their seeming ingenuity. A computer that processes a photo of a pile of donuts lying in a pile on the table automatically signs it: “A pile of donuts is on the table,” and seems to understand the world around it. But when the same program processes a photo of a girl brushing her teeth and signs it: “a boy holds a baseball bat,” you understand how shaky his understanding is, if it exists at all.
Neural networks are thoughtless recognizers of fuzzy patterns, and are only as useful as fuzzy patterns recognizers can be useful - hence the rush associated with their integration into all possible software. They represent a kind of limited intelligence that is
easy to fool . A deep neural network that recognizes images may be at a dead end if you change one pixel, or add visual noise that a person does not even perceive. And indeed, every time we find new ways to apply GO, we also find its limitations. RoboMobi can not cope with the orientation in the conditions, hitherto they have not met. Machines hardly recognize sentences that require common sense and an understanding of how the world works.
GO in a sense mimics the processes occurring in the human brain, but not too deep - this may explain why the AI ​​sometimes turns out to be so shallow. The ORO was discovered not by sensing the brain, deciphering its messages; it grew out of the models of animal learning by trial and error obtained in old experiments on the development of reflexes. And in most of the breakthroughs that came with him, there were no new neurobiological revelations; there were only technical improvements obtained over the years of work of mathematicians and programmers. Our knowledge of the work of the intellect is nothing compared to what we do not yet know about it.
David Duvenaud, an assistant professor working in the same department at the University of Toronto as Hinton, says that GO resembles the work of engineers before physics. “Someone wrote a work where he said:“ I built a bridge, and it does not fall! ”Another comrade wrote:“ I built a bridge, and it fell - and then I added columns, and it did not fall. ” After this, the columns become a hot topic. Someone comes up with arches, and as a result, everyone says: “Arches are cool!” And after the advent of physics, he says, “you can understand what works and why.” He claims that we have only recently begun to move into a phase of real understanding of the work of AI.
Hinton himself says: “Most of the conferences consist of introducing small changes, instead of thinking carefully and saying:“ What do we lack in our current activities? What does she have problems with? Let's concentrate on that. ”
From the outside it is difficult to catch when you see only one eulogy about another breakthrough after another. But the latest progress in the field of AI belongs more to engineers than scientists, and reminds me of a fuss at random. And although we already understand better what changes will be able to improve the system with civil defense, we, by and large, are still in the dark about how these systems work, or whether they can ever come closer to such a powerful system as the human mind.
It is worth wondering whether we have already squeezed everything possible from ORO. If so, it is possible that the AI ​​progress chart has a plateau.
Patience
If you want to know where the next breakthrough will occur, which will form the basis for building machines with more flexible intelligence, you should study the research process similar to what the ORO came up with in the 80s: very smart people play with ideas that not yet working.
A few months ago, I was at the Center for Mind, Brain, and Machines — a joint project of several institutions, based at MIT, to see how my friend Iyal Dehter [Eyal Dechter] defends his dissertation on cognitive science. Right before the report, his wife Amy, their dog Ruby and daughter Susanna were spinning beside him and wished him good luck. On the screen was a picture of Ruby, and next to her was a photo of Suzanne in infancy. When dad asked Suzanna to show where she was on the screen, she gladly slammed a folding pointer in her infant photo. As she left the room, she unfolded her toy pram and shouted, “Good luck, daddy!” Over her shoulder. She added: “Vámanos!” She is two years old.
Iyal began his presentation with a question: how is it that Suzanne, having two years of experience, learned how to talk, play, follow the plot in history? How can a person’s brain learn so well? Will the computer ever be able to learn as quickly and smoothly?
We interpret new phenomena on the basis of what we already understand. We break the whole into parts and study the parts. Iyal is a mathematician and programmer, and he imagines tasks - like, say, preparing a soufflé - as very complex computer programs. But you don’t learn how to make a soufflé through studying trillions of microinstructions like “turn your elbow 30 degrees, look down at the tabletop, stretch your index finger” ... If you had to do this with each new task, learning would be too difficult and you would left with what you already know. Instead, we write a program with high-level steps like “beat up egg whites”, consisting of routines like “break eggs” and “separate yolks from proteins”.
Computers do not, and this is one of the main reasons for their stupidity. To get the GO system to recognize hot dogs, you need to feed it 40 million hot dog images. To teach Susanna to recognize a hot dog, you show her a hot dog. And very soon she will already understand the language at a deeper level than the realization that certain words often appear together. Unlike the computer, she has a model of the whole world in her head. “It is amazing for me to hear people fear that computers will take their jobs away from them,” says Iyal. - Computers cannot replace lawyers, because lawyers do very hard work. Lawyers read and talk with people. We have not come close to this. ”
True intelligence does not break when you slightly change the requirements for the task that it is trying to solve. The key part of the work of Iyala is to demonstrate how, in principle, you can make a computer work like this: it is easy to apply existing knowledge to new tasks, quickly move from a state of almost complete ignorance in an area to an expert.
In essence, this is a procedure that he calls the "learn-compress" algorithm. A computer works in a manner similar to a programmer, building a library of reusable, modular components with which you can create more and more complex programs. Having not gained knowledge about the new area, the computer tries to structure knowledge about it, simply playing with this area, consolidating the received information, playing again - the way the child does.
His curator, Joshua Tenenbaum, is one of the most frequently cited AI researchers. His name came up in half of the discussions that I had with other scientists. Some of the key people from DeepMind - the team behind AlphaGo, who shocked computer scientists, beating the world champion in go in 2016 - worked with him as a supervisor. He is associated with a start-up trying to impart robomobili with intuition about the basic laws of physics and the intentions of other drivers so that the AI ​​can better predict the outcome of situations he has never seen before, such as a wagon carrying a trailer or an aggressive rebuilding attempt.
Yial's thesis is not applicable to practical applications, not to mention writing any programs that can beat a person and get into the headlines. It’s just that the problems Iyal is working on are “very, very complex,” says Tenenbaum. “They will take many, many generations.”
Tenenbaum has long, curly, graying hair, and when we sat down to drink coffee with him, he was wearing a buttoned-up shirt and slacks. He said that he turned to the history of the development of ORO for inspiration. For decades, ORO was just a cool math, unfit for anything. When computers become faster and programming is more difficult, it suddenly came in handy. He hopes that something similar will happen with his work, and with the work of his students, "but this can happen in a couple of decades."
Hinton himself is convinced that to overcome the limitations of AI, it is necessary to “build a bridge between computer science and biology.” From his point of view, ODP was a triumph of biology-inspired computing; the idea originally came not from programming, but from physiology. So now Hinton is trying to do a similar trick.
Today's neural networks consist of large flat layers, but in the cerebral cortex, real neurons are organized not only in horizontal layers, but also in vertical columns. Hinton believes that he knows why they are needed - let's say they are crucial for his eyesight and his ability to recognize objects even when the field of vision changes. So he creates an artificial version of them — he calls them “capsules” —to test his theory. So far, it does not work - the capsules could not significantly improve the performance of the network. But the exact same situation was with ORO for almost 30 years.
“This idea just has to be right,” he says about capsule theory, and he laughs at his own confidence. “And the fact that it does not work is a temporary nuisance.”
James Somers - journalist and programmer from New York