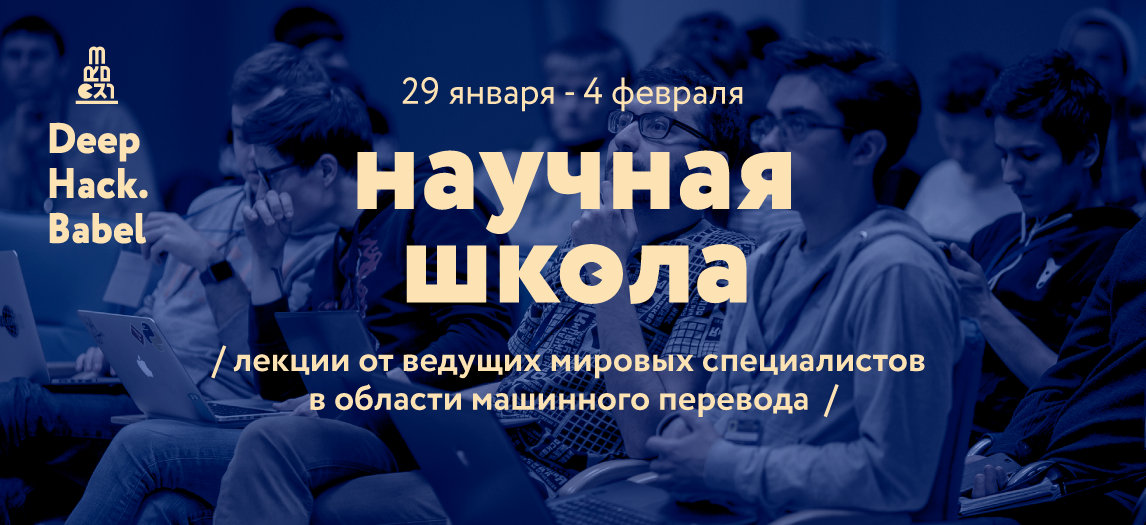
Before the new year, we were
told why we need a hackathon for machine translation. Next week, the 50 participants selected will come to Dolgoprudny to participate in training the system on non-parallel data. In addition to many hours of brainstorming, the scientific school will help in the search for solutions - a
series of lectures from leading world experts in machine translation . Habr, we invite you to visit interesting performances! They will be held on Fiztekh from January 29 to February 4, do not forget to
register . Well, if you don’t want to leave the house on a frosty winter evening, you can watch the broadcasts on
the DeepHack channel .
"Attention is all you need"Ilya Polosukhin , founders of the startup
Near.AI and ex-Google employee
January 30, 20:30
Today, most natural language processing relies on convolutional and recurrent models in a coding / decoding scheme. The most successful models connect the decoder with the encoder through the mechanism of attention. One of the founders of the Near.AI startup, Ilya Polosukhin, will talk about the breakthrough Transformer machine translation model he worked on at Google. It is a simple network architecture built only on attention mechanisms. As experience shows, experiments with neural machine translation demonstrate an improvement in the quality of translation, with significant savings in training compared to the previously proposed recurrent models.
')
"Deep learning for reading comprehension"Ruslan Salakhutdinov (Carnegie Mellon University)
February 2, 7 pm
Ruslan Salakhutdinov, Associate Professor at the Faculty of Machine Learning at Carnegie Mellon University, will talk about the use of in-depth training for text understanding. His research is aimed at understanding the computational and statistical principles that allow the structure to be detected in large amounts of data.
More about the work experience of RuslanIn 2009, Ruslan received his Ph.D. in computer science (specialization: machine learning) at the University of Toronto, after which he worked for two years in the laboratory of artificial intelligence of the Massachusetts Institute of Technology. He then returned to the University of Toronto as a senior lecturer in the departments of computer science and statistics.
Ruslan is editor in the Journal of Machine Learning Research and, moreover, he was a member of the program committees of several specialized conferences, including the Conference on Neural Network Information Processing Systems (NIPS) and the International Conference on Machine Learning (ICML). Ruslan received scholarships from the Alfred Sloan Foundation and Microsoft, and participates in the Canada Research Chair program for machine learning. He is a winner of the Early Researcher Award, a Google award for members of the academic community, and the Nvidia Award for AI Pioneers. Ruslan is a senior research fellow at the Canadian Institute for Advanced Study.
"Neural Machine Translation"Kyunghyun Cho (New York University & Facebook)
February 2, 5:30 pm
Gyeonghyun Cho, a senior lecturer in computer science and data science at New York University and a fellow at
Facebook AI Research , will talk about his research over the past 2.5 years in the field of neural machine translation. Using as a starting point the concept of neural machine translation on the mechanisms of attention, Kyunghyon Cho will also touch upon the topics of multilingual translation, non-parametric neural machine translation based on search engines and machine learning without a teacher. He will also briefly talk about his current work in the laboratory, including non-autoregressive neural machine translation and trained greedy decoders.
"Neural easy-first taggers"Andre Martins , Researcher at Unbabel Inc.'s Lisbon office
February 4, 11:00
André Martins will talk about his recent work on a new text processing model called neural easy-first tagger. This model is trained to solve problems of marking the sequence - for example, annotation of grammatical and lexical features of words in the text. The model solves the task of marking the sequence, regardless of the order of the objects in it. The decoder updates the “sketch” of the predictions in several iterations. His work is based on the attentional mechanism, which determines how much of the incoming data is strategically beneficial to process the next. When solving sequence marking problems, this model is superior to taggers using bidirectional networks with long short-term memory (BILSTM).