Ksenia Suvorova, development director at Fontanka.ru , and Andrei Miroshnichenko, coordinator of the offline Data Scientist program, specifically for the Netology blog, prepared interviews with Avito and Spice IT HR agency about what the market is waiting for from the Data Scientist profession .Now almost every article on data science or machine learning begins with the fact that “three years ago, an American research company calculated that in four years a million data scientists would be needed.” And even in Russia, there is an acute shortage of people with expertise. At the same time, there are many opportunities to master this area: online and offline courses, simulators and books - that is, it is real to correct the current situation. Interviewed by Andrei Miroshnichenko - coordinator of the offline program "Data Scientist".
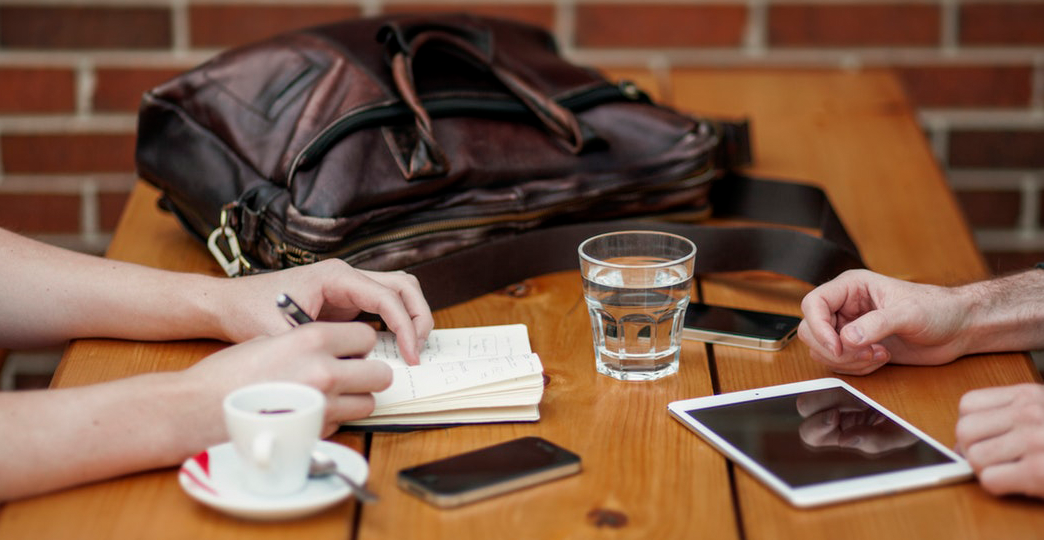
')
Last time, we made a
brief squeeze of two interviews , and talked about how the profession of a specialist with big data looks like from an employer, not an employee. Today we publish full interviews with Avito and Spice IT.
Avito
Alexandra Golovina told about the perspectives of the data science profession and Avito's work on Netology.At what stage, in your opinion, is the data science market? Is it growing, sufficiently developed, or even declining, and after six months, people who have studied will understand that they have wasted time?
The need for data science specialists is very high and will continue to grow. However, there are also many opportunities for learning: anyone who understands that he lacks an academic education can take courses and get the necessary base.
The question is, rather, who and why comes into the profession. Now there are quite a few people who are interested in the area of ​​big data and who study it, but do not yet understand what they really need it for. At the interview, applicants say that they are interested in machine learning, and when you begin to ask why, they answer: "This is fashionable." And that's all. Understanding how to apply knowledge, no. That is, in most cases, this research is for the sake of research, an attempt to test its own hypotheses.
And in the business is not so. There is a task for which it is necessary to find the optimal solution method. The specificity is that this solution is practically realizable. And this is probably the main problem that we face in selecting people. Some applicants believe that it is enough to come up with a beautiful algorithm, and the fact that it cannot be applied anywhere is a tenth thing. That is, the problem is that many people study data science, but where and why to use the data, not everyone understands.
Examination, in turn, can be divided. There are people who have worked in the field close to us - klassifaydah, IT. They perfectly understand where and how to apply knowledge. People who come from another sphere - from banks, yesterday's graduates or who have worked in the laboratory - in this regard lose, but for us this is not an indicator. Such candidates may not know the specifics of our business, but they can develop fully working algorithms without complicating the task. This, by the way, is the second problem of the candidates. They go into deep learning, deep networks, trying to find something more complicated. Although in reality the model that will work can be much simpler.
How do you recruit people: from the market or from internal resources?
Data science comes from different directions. We do not look for candidates exclusively from Yandex and Mail.ru. Although for high-level positions, of course, it is necessary that a person has previously worked with big data: this requires specificity.
Even in data science and machine learning, many fall from our competitions - c Kaggle, DataRing. But even this is not a decisive indicator for us. We look at how the competence of the candidate corresponds to the position, how much he will fit in the team. Because it is very important for us that a person knows how to interact with colleagues.
Do you have any special affiliate programs, such as Yandex with HSE?
Not yet, unfortunately. Despite the fact that we have a strong data science, we started talking about this outside recently. But I think in the next year and a half we will come to the partnership programs.
Speaking of professional competencies. What basic skills and knowledge do you seek from candidates? What kind of base should you have? Should it be a profile university or a profile education, for example, mathematical or specialized in data science, which also appears in Russia?
For specialists in the data we have a strict selection throughout the company: the biggest funnel and the smallest way out of it. Colleagues have formed very clear evaluation criteria. They always know what they need, and in this plan we are the same. We have a very harmonious and friendly team, which I am proud of.
Skills can be divided into hard and soft. As for hard: education is necessarily mathematical. The specialist must understand how mathematical models work. They come to us, as a rule, from leading universities: MIPT, HSE, Moscow State University. Among the graduates of the latter, in jest, there is even a competition, of which there are more in the company - those who graduated from mechmat or graduates of the VMC.
There is also a conditional division into product analysts and ML analysts. The task of product analysts is to look for the possibility of improving the product, generating hypotheses about possible problems for users and ways to solve them quickly. ML analysts automate solutions found by product analysts of tasks using various ML methods: personal recommendations, pricing, and so on.
Basic skills check on the test task. The department is large, it consists of several departments that support different systems. Therefore, each department has developed its own case, as close as possible to what it has to do in the future. When solving such a case, the candidate’s skills become obvious. After that we look at the code and decide who to invite to the meeting.
About software skills. This is the part that we will definitely pay attention to in person with the candidate. Since data science specialists are involved in cross-functional projects, it is very important for us that a person shares company values, can work in a team and build communication with colleagues.
That is, the story about a little crazy genius who sits alone in a corner and makes supermodels - does it not work?
Looking for some tasks. Theoretically this is possible, and somewhere such things work. But Avito work involves teamwork. Therefore, we analyze the skills in the complex.
Returning to the balance of expertise and core knowledge. Who is preferable in the general case: a programmer who writes cool models, but who has a tiny specialized experience, or a person who has a lot of experience, but only basic knowledge in machine learning?
Here everything is purely individual. We must look at the person, on his prospects. Colleagues are ready to take people with minimal technical experience if they see potential in them. And then everything depends on the motivation: whether the person himself wants it, how he plans to develop, and how far along the path we are in the long term.
Do you have internal training programs, mentoring?
Of course. On the one hand, we provide freedom of action. The task comes, and what tools to choose, how to build, where to look, what to use - the employees themselves decide. In this regard, we have very initiative guys. They are literally sick of their work. Every week they organize mitapes where 1–2 people perform: they say that they use, what method they use, what they work and what they don’t. They share knowledge within the team, and new employees have the opportunity to learn directly on the job.
On the other hand, we invest in hard and soft skills of employees. Avito has an academy where you can sign up for any training. That is, you can learn not only from colleagues, but also from specialists in other professional fields.
You mentioned contests. What other ways are there to get to you, except how to file with a resume?
The purpose of the contests we organize is not to close a position. They have many goals, and the main one is to find ideas for solving a specific urgent task. If at the same time you succeed in attracting an employee, this is absolutely great. But contests are not the primary recruitment method. Regular search, job sites - it all works too. We go out on some people on our own, colleagues recommend somebody.
Speaking of vacancies. Often met in Russia and abroad the names of vacancies in the field of data science with approximately the same responsibilities differ. Somewhere it was a machine learning engineer, a data scientist somewhere, a data analyst somewhere, a programmer somewhere. So what are these vacancies called?
There is no single name. These may be broad names, such as Senior Analyst, and more specific ones, for example, Pricing Analyst. It all depends on how specific the intended tasks are and how long we assume that the person will solve them before switching to others.
As a rule, when I communicate with candidates, I’ll talk in detail about the department where we are looking for a person, about his specifics. Because the data scientist is a data scientist. In different companies, it has different functionality, and it is better to immediately clarify what to do.
About the portfolio. How important is its presence?
This is optional. Colleagues may be interested to see previous projects of the applicant, but they are not the decisive argument. We give the candidate a case that is as close as possible to what is to be done. And we look at how a person will work in the field - this is the most important.
HR Agency Spice IT
At what stage is this market segment? Should we expect a recession in the near future?
Jobs more and more. Data is the most valuable product on the market. Recession in the near future will not be. There are not enough specialists, especially if we are talking about such vacancies as Head of Predictive Analytics or Lead / Chief data scientist. Candidates are busy on serious projects and do not want to quit. Plus, these positions imply the presence of special qualities required by a company.
With interns and junami easier. Data science is beginning to gain momentum, and many are happy to try their hand in this area. The guys themselves send us a resume with a request to consider them on the existing positions. We are only for.
I think in a couple of years Data science will become one of the leading vacancies, the market will be full of offers, but is there enough for all candidates ...
What professional competencies are you looking for? What should be the base?
Professional competencies depend on the requirements of the client company. From the main one can be distinguished: R, Python, Machine Learning, databases, such as MSSQL, MySQL, Postgresql. Candidates for the position of Data Scientists should be well versed in mathematics, statistics and programming.
What non-professional skills are you looking at?
Soft skills. Jobs, where soft skills are one of the key requirements, are quite rare, unless it is a leadership position. Due to the fact that there are not so many strong specialists in the market, the emphasis is on the technical component. Of course, many companies would like to see in their ranks specialists with a proactive attitude, prepare presentations, make up beautiful reports and know how to establish contact with colleagues and management, but again, in practice, most customers rely on these requirements, preferring to communicate sociability with good technical experience.
Of course, there are universal things that are expected by default. Responsibility, independence, if we are talking about the position above the junior. If a candidate is trying to be an intern, then the ability to google and ask senior specialists in time is very important. Well, following the current trends in the industry for all. Although this is no problem. People in this position are usually not curious.
Do I need to have a special university education to get into the company, or are self-taught people also considered?
Of course, this question is more designed for an inhouse, but I want to note that higher technical education is a point that is often found in the basic requirements.
Usually, after analyzing the market and organizing a selection of relevant resumes, we agree with our clients that education is not a key factor in the selection. But there are quite specific customers, for whom the presence of a narrow profile education, corresponding to the direction of the company, is important. For example, a couple of months ago we were looking for a scientist with the education of a psychologist, but this is rather an exception to the rule. Due to the dampness of the market, education goes into the background when compiling a job description.
How important is the core experience in the company?
Today, I had only one position where this experience was important: just that same psychologist-scientist. Basically this criterion is rare. Although, perhaps, this is due to the fact that in our work most of the positions on data science come from startups that have a unique or rarely encountered line of activity. It would be almost impossible to find specialists of this type.
Are data scientists hired with little or no experience?
Such positions are often found. Companies are ready to train, accepting candidates as interns or junior analysts. Ready to watch the guys who lead projects on freelancing or doing something for themselves in order to gain experience.
What is the average experience you need to have to get to work as a data scientist?
We have jobs at various levels, where we need specialists with minimal experience or a strong technical background. There are lots of options, everyone can pick something up.
What problems do you most often encounter with candidates for data science posts?
It often happens that the junior position lacks only a theory - it takes experience, at least some. Companies want candidates who have already tried to do something on their own, even if it is a practical task from a training course or an experience of participating in competitions held by Kaggle.
What advice and recommendations can you give to candidates during the interviews at the data science post?
The main thing is the preparation for the interview. Usually I ask the candidate to prepare for the meeting with the technical team. Sometimes it happens that the guys point out a lot of technologies in the resume, and in fact they work with only a small part of this. I ask you to honestly describe what and to what extent you had to face. This avoids embarrassing situations in an interview where people cannot answer questions because they have a superficial idea of ​​things that they themselves have voiced. You can look at the basic requirements and tighten up what is possible.
From the Editor
Courses "Netology" on the topic: