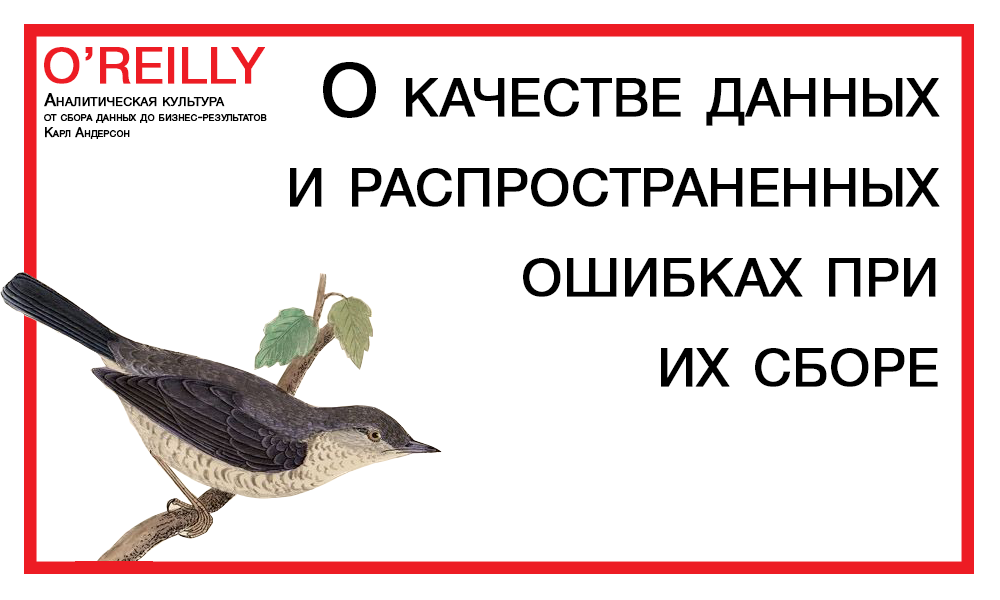
Many companies believe that they work and make decisions based on data, but often this is not the case. After all, in order for management to be conducted on the basis of data, it is not enough to collect and compile them, these same data, into statistics.
It is much more important to conduct a proper analysis, and for this they must be “clean”.
To understand the purity of the data and the basic quality parameters, I will start with this article.
For reliable analytics, all “P” data must be observed: correct, correctly collected, collected in the right form, in the right place and at the right time.
If one of the parameters is violated, this can affect the reliability of all analytics, which means that you need to understand what is important to pay attention to when working with data.
Key Data Quality Aspects
AvailabilityAnalysts should have access to the necessary data, but, in addition, access should be to the tools used in the analytics.
AccuracyAll data must be reliable, and the permissible errors are indicated.
Exact temperature - good data, and outdated address, phone or e-mail - bad data.
')
InterconnectednessIt should always be possible to associate some data with others. For example, information about a customer, his address, contact and payment information should be attached to the order number.
CompletenessThe data must be “fat” and with all parts. "Disabled" with the missing part of the information may interfere with getting high-quality analytics.
ConsistencyIf the data is not consistent and contradictory, then an error has crept in somewhere.
So if the client's address is present in two databases, then it must match. Otherwise, it is necessary to select one source that is reliable and ignore the rest before correcting errors.
UnambiguityEach field with information should have a full description, which does not allow ambiguous values.
RelevanceData must be consistent with the nature of the analysis.
For example, the statistics of seasonal migrations of lemmings are weakly related to seasonal fluctuations in exchange rates.
The same lemming that does not affect stock prices.ReliabilityReliable data is both complete and accurate information.
TimelinessThe scourge of Russian business is untimely data.
It often happens that the data have not yet had time to process and analyze, and they are already outdated.
Obsolete data cannot be used to build a short-term strategy; they can only be used as a basis for long-term strategic planning and forecasting.
Another disadvantage of outdated data is that they have become almost useless, and the company bears the costs of their storage and processing.
An error in any of the points may lead to partial or complete unsuitability of the data for use, or, worse, to incorrect conclusions made on the basis of erroneous data.
Data with errors
Basilisk - an error has clearly crept into his description.Errors appear at any stage of working with data, and often analysts can no longer influence their correction, since these specialists are the final link in the work with the material and cannot control the collection and processing of information.
Let's look at the main causes of errors and ways to help avoid them.
Data generationThe most frequent and obvious cause of errors: there may be both technical reasons and the influence of the human factor.
In the case of technical reasons and failures, everything is solved by calibrating and properly setting up information collection tools.
When repair and calibration do not help in resolving the issue and the data continues to arrive unreliable, then one of the possible reasons is the unreliability of the instruments.
So IR sensors that measure the distance to the nearest wall when mapping an area, can give an error of a meter or more or reset the collected data. Trust the testimony of such unreliable sensors can not.
The human factor can also manifest itself in different ways. For example, employees may not know how to correctly collect data or may not be able to work with the tool, may be inattentive or tired, not know the instructions or misinterpret them.
â–Ť The most reliable and simple solution is to standardize as many stages of the data collection process as possible.
Data inputWhen manually generating data you need to fix them, at this stage many errors occur.
No matter how the electronic document circulation is expanded, many data pass through paper before entering the computer.
Errors often occur when decrypting handwritten data. Most of the research on solving decoding errors is carried out in the medical field, because the patient’s health and life are at risk due to the slightest inaccuracies.
So the
study showed that 46% of medical errors due to inaccuracy in deciphering handwritten data. And the level of errors in medical databases
reached 26% , there is an assumption that this is due to the fact that the staff misunderstood or could not make out what was written by hand.
For example, some results of medical surveys of the population show that the growth of an adult can be 53 cm or 112 cm. And if in the first case it is clear that the error crept in, and most likely the growth of the recipient was 153 cm, then in the second case it can be both correct and erroneous. In surveys, occult errors often occur, such as “window allergies” or a weight of 156 kg instead of 56 kg.
On average, errors are divided into four types:
- Record
An error where the data was originally written incorrectly.
- Insert
The appearance of an additional symbol.
For example: 53,247 â–ş 523,247.
- Deletion
Loss of one or more characters.
For example: 53,247 â–ş 53,27.
- Change of places
Just take and change two or more characters in places.
For example: 53,247 â–ş 52,437.
We should also consider dittography (random repetition of a symbol) and haplography (omitting duplicate characters). These errors are often encountered by scientists involved in the restoration of damaged or rewritten by ancient texts. And this is another problem associated with poor-quality data.
Often mistakes are found in the spelling of dates, and even more often when different standards are encountered, such as American (month / day / year) and European (day / month / year).
And if it is sometimes clear that this is a mistake (March 23 - 3/25), in other cases it may not be noticed (April 3 - 3/5 or 5/3?).
How to reduce the number of errors
Hippogriff is a proud and majestic mythical animal, a species of griffins. Yes, in the engraving, too, he, but with errors in the description.The first step is to reduce the number of data generation steps to input. If you can avoid participation of the paper carrier as a transfer link, exclude it.
In electronic forms, you should enter the verification of values, this is especially important when entering structured data: index, phone number and city code, BIC, SNILS and p / s.
Many data have a clear structure that helps reduce errors - it can be the number of characters, their breakdown into groups, and other types of formats.
â–Ť If possible, exclude manual data entry and prompt the operator or user to select a value from the drop-down list.
If the number of options is large, then you can use the question-answer form with final confirmation of the correctness of the entered data.
Ideal is to eliminate the human factor during data entry and automate the process.
When decrypting data, the “double entry principle” has proven itself well.
When using this method, two employees are independently deciphering each other, and after the results, the data in which discrepancies are found are compared and rechecked.
An interesting data verification method is used when transferring data in digital format.
For example, in bank account numbers a control number (amount) is used.
The check number is when a number is added after the number being transferred, which is used to verify the data and detect errors.
So for the number 94121 the control number will be 8, with successive folding of the digits we get the sum of 17, we continue folding and we get 17 = 1 + 7 = 8.
We transfer 941218, and upon receipt, the system carries out reverse calculations and, if the amount does not match, the number will be marked as erroneous.
There can be several control numbers, one for each block of digits.
There are drawbacks to this method due to the symbol permutation error, but this is better than nothing.
This concludes the introductory article on data collection and quality control. If the information was useful to you, I will be glad to feedback.
Perhaps you disagree with something or want to share your methods and practices - I invite you to comment and hope for an exciting and useful discussion.
Thank you all for your attention and have a nice day!
A source of information
Posted by: Carl Anderson
Analytical culture. From data collection to business results
Creating a Data-Driven Organization
ISBN: 978-5-00100-781-4
Publisher: Mann, Ivanov and Ferber