We have already mentioned the Data & Science series of events several times, where data analysts and scientists tell each other about their tasks and look for ways to interact. One of the meetings was devoted to bioinformatics. This is an excellent example of an industry where there are a lot of unsolved tasks for developers.
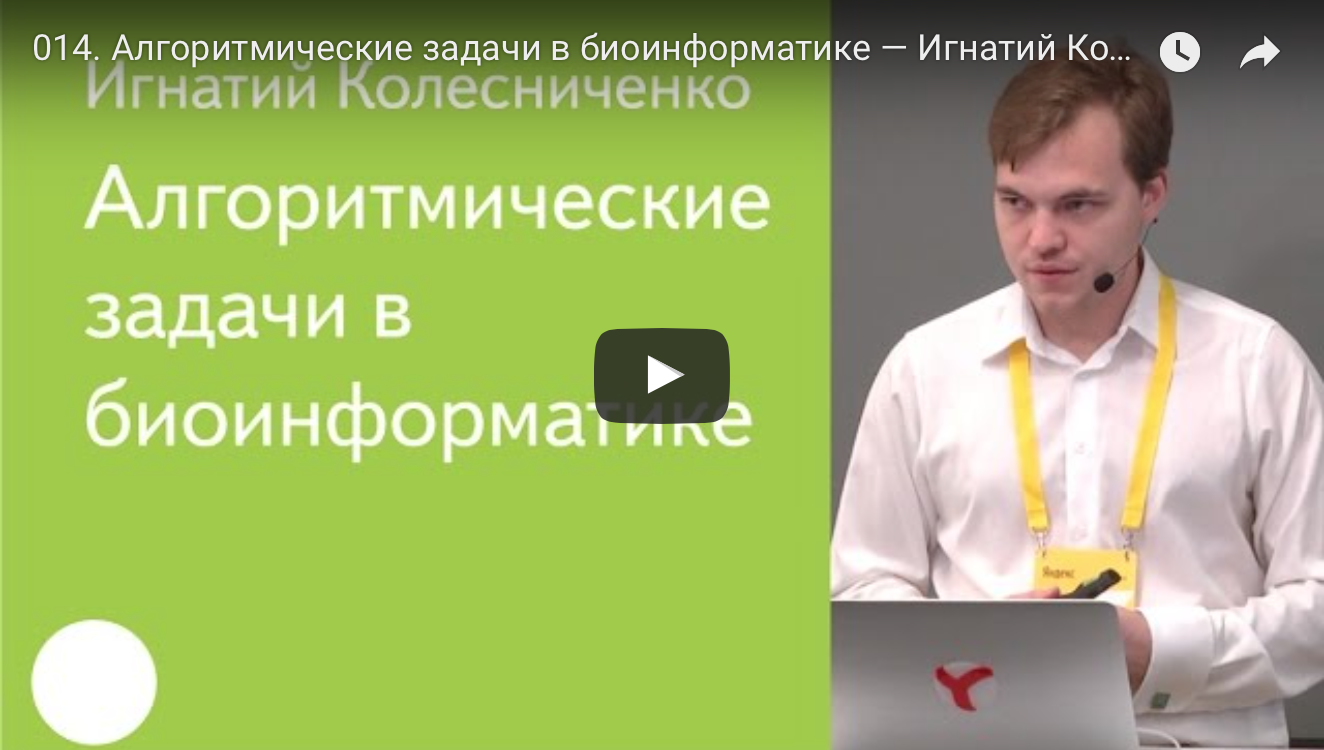
Under the cut you will find the decoding of the lecture by Ignat Kolesnichenko - a graduate of the Moscow State University and the School of Data Analysis. Now Ignat is working as a leading developer of the Yandex distributed computing technology service.
')
Who is the programmer here? About half the audience. My report is for you.
The purpose of the report is as follows: I will tell you exactly how the data are taken and how they are arranged. The story will be a little deeper than
that of Andrew . For this it is necessary to remind a little about biology. I, at least, expect that programmers do not know biology.
And then I will try to tell you what algorithmically complex problems are solved in this area in order to interest you, so that you understand that it’s great and you need to go into bioinformatics and help people solve complex problems. Obviously, biologists themselves will not cope with algorithmic tasks.
I graduated from Mekhmat. I am a mathematician, I work in Yandex. I am a programmer, I work on
the YT system , we build the internal infrastructure in Yandex, we have a cluster of thousands of machines. All the data that Yandex collects is stored in these clusters, everything is processed, petabytes of data, I understand this a little. I am also a co-founder of Ebinom. There I was engaged in the same technical problem. My goal was to figure out how these algorithms, programs work, and teach them how to do it in the most automated way, so that a person could upload data to the site, poke a button, and a cluster would turn around, everything would be quickly calculated, minimized, and would have seemed a man.
While I was solving this technical problem, I had to figure out what data we were talking about and how to work with them. Today I will tell part of what I learned.
A
video of Gelfand was posted on Facebook in the announcement for this event. Who watched it? One man. Gelfand looks at you disapprovingly.

We’ll work with DNA, but I want some context around the DNA, so we’ll start with the cell. It is clear that bioinformatics as a science of molecular biology is interested in all processes in a cell, but today we will talk more about the technical side, about the data and we will be interested in, conditionally, only the core and nearby components.
The nucleus contains DNA. It has a nucleolus. It, together with the histones that Andrew showed, is in some sense responsible for regulation and epigenetics. There is a second important part - the ribosome, folds around the nucleus, the so-called endoplasmic reticulum. It has special complexes of molecules called ribosomes. It will be from your pieces of DNA, which you subtract from the DNA first, to make proteins.
The third part is chromatin: all matter in which all DNA is floating is located. It is packed tightly enough, but between it there are still a lot of different regulatory molecules. Well, there is a membrane - protection, nutrition, the way the cell communicates.
There is much more in the cell, other functions. We will not talk about them, you can read in textbooks.
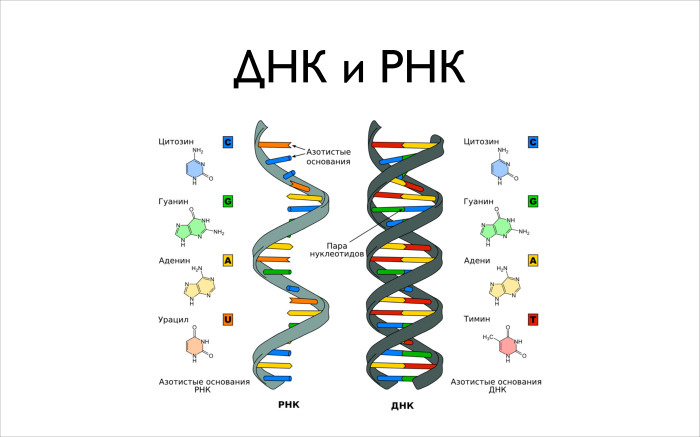
Now about the information, DNA and RNA. There are two main molecules: deoxyribonucleic acid and ribonucleic acid. These are molecules of the same type. Once the structure of this molecule was discovered by Watson and Makkrik. An interesting story, you can read the autobiography, he writes very beautifully about everything. It is a double helix, this is a popular picture, a popular buzzword.
It consists of four larger molecules, complexes of dozens of atoms. These are guanine, cytosine, adenine and thymine. And in RNA instead of thymine will be uracil. When you make RNA from DNA, instead of the letter “T” you will get the letter “U”. It is important for us to know that our DNA contains these four letters. With them we will work, by and large.
DNA has a higher structure. This is not just a spiral, which somehow lies, somehow as twisted. It twists around special complexes - histones. Then the histones are twisted into some fibers, from which the letter X is already built. Here is a beautiful picture that you saw in the biology textbooks. Usually they do not speak about histones, 15 years ago they did not speak, but show the letter X.
Histones are responsible for regulation. When you want to read some RNA with DNA, you may not succeed, because it is so twisted, hidden in this DNA, that it cannot be read from there. Or maybe something will change and it will start to be read.
And there are various squirrels that can attach to all this, can unwrap it, pull it out so that you can read it. The secondary structure is very important to understand which parts of the DNA, from which you can then make RNA proteins, are available for you to read.
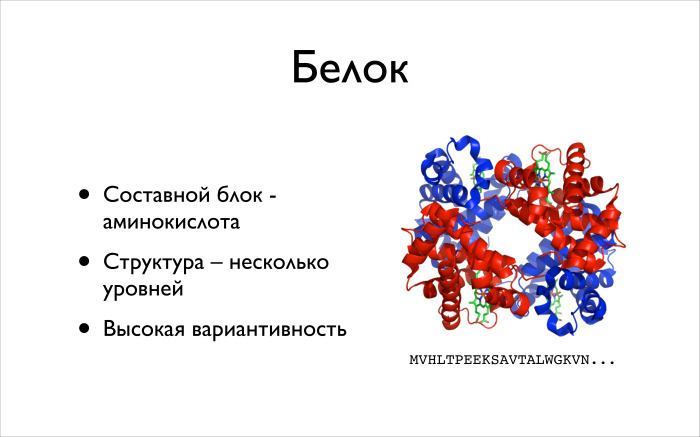
What is protein? The main vitality of the cell, the diversity of all living things is largely due to proteins. The fact is that the protein is very high variability. We are talking about a huge class of very diverse molecules that are involved in a huge pile of molecules. They have very different structures, which allows all this complex chemistry to occur inside the cell.
What you need to know about squirrels? The compound is an amino acid. Amino acids in our body, in our world, 20 pieces. They are said to have a little more, but there are 20 of them in our bodies. They also have certain Latin letters. On the right, the protein is schematically drawn, its beginning is written out, it is indicated which letters are present. I am not a biologist, I do not remember what a V or M is, but biologists can immediately say what the letters mean.
The structure of the protein has several levels, you can look at it simply as a sequence, and when you are a bioinformatist, you often look that way. Although here Arthur is interested in more complex secondary and tertiary structures.
We will look at it as a sequence of letters. Secondary and tertiary structures. There are such spirals, alpha-spirals, they are clearly visible in the picture. There are more beta lists, she knows how to line up fairly flat things, and there are special pieces that collect these alpha-helix and beta sheets.

Further, what Andrei was talking about is the central dogma of molecular biology. It consists in the fact that there is translation and transcription, and there are still many different processes of inverse, perpendicular, which also involve RNA, DNA and proteins in different directions. But we will look today only at translation and transcription. On the right there is a fairly complete picture describing this thing in eukaryotic cells, in particular in humans.
You have a gene. In the textbooks of biology they write that this is a unit of heredity. For us, when we look at DNA, a gene is some not so simple part. Not that it is translated into PH, and then protein is made from RNA. Everything happens a bit wrong. First, in the specified area there are regions, introns, which are cut. And there are exons, which then will be encoded into your protein. If you look at the DNA of 3 billion letters, then - this is a popular phrase - there are only 2% of the important areas. Only 2% are responsible for coding your genes. And we are talking about exons.
If you take more introns, you will have not 2%, but 20%. And the remaining 80% are intergenic regions involved, apparently, to some extent in regulation. But some really do not need a bottom, just as it has historically been.
The following happens: you have a certain promoter in front of the genome, a special molecule sits on this promoter, a protein that will read and make RNA from your DNA.
Further from the RNA, the transformation goes by another molecule, which cuts out unnecessary introns from it. You get a ready-made messenger RNA, from which you make your protein a translation. This cuts off 3 'and 5' - non-coded ends. When you make a protein from RNA, you actually have a triplet, some sequence with which you can start. Everything before it is just skipped. The cell sets, it skips it, and starts doing everything from the start codon.
I used the word codon. What are codons? how does coding work? The logic is simple. There are four symbols in DNA: A, C, T, G. There are 20 symbols in a protein, 20 amino acids. It is necessary to somehow make one of the four 20. It is clear that it will not work directly, it is necessary to encode them somehow. From the point of view of scientists who are engaged in coding, the device is very simple, stupid coding, we would have thought up this easily. But from a biology point of view, doing this was not easy.
You have three letters of DNA read, and each triplet of letters encodes a particular amino acid. The triplets of letters 64, and 20 amino acids can be encoded in 64 variants. Encoding will be redundant. There are different triplets that encode the same thing.
That's all you need to know about broadcast and transcription today.
Andrei talked a lot about metagenomics, about different omiki. Scientists of the last 50–100 years are very actively studying what is happening in the cell, what kind of processes are there, what kind of chemistry is there, and not just biology. And they are quite successful in this matter. They may not have a complete picture and understanding, but they know a lot of things.
There are large maps of the transformation of different substances into each other. In particular, most of the elements, proteins, which describe what generally happens in the cell.

Here is a small piece, and there is a big picture, scientists love to draw it. Before us is an exemplary simplified diagram of everything that is done in our cell.
It is important to understand that all molecules are more or less always present in our cell - the question is in concentrations. All the time, your DNA is unfolding somewhere, something is being read from it, and what is being read depends largely on the concentration of different proteins. And the whole life of the cell is regulation. You have become more, something went to do more than another process, he began to broadcast something more, a new protein appeared, he took part in some process, said that he was enough to produce me, gave birth to a new protein, which began to interfere with its production. Such processes looped with feedback are present in large numbers in the cell and are here generally represented.
Where do the data come from - these A, C, T, G? Andrew said that there are sequencers that you read them. Let's understand in more detail how these devices are arranged and what they will read.
There is a classic method of Senger, which was invented in the late 70s, and which was allowed in 1989 to start the project "Human Genome". By 2001, the project was completed, they were able to assemble a sequence of a certain “reference” person, consisting of 3 billion letters. We disassembled all 23 chromosomes and understood which letters - A, C, T, G - are in each chromosome.
Senger's method is good and reliable, scientists trust him, but it is very slow. In 1977, it was a terrible horror, you worked with some radioactive solutions and substances in gloves, being behind the lead thing, something was poured somewhere in a test tube. Three hours after these exercises, if you didn’t accidentally throw something in there, you received a picture from which you could learn 200 nucleotides of your DNA.
It is clear that the process was quickly automated, and in the course of automation, the technology changed a bit. By the mid-2000s, there was a mass of new technologies that allow sequencing to be done quickly and cheaply and not spend three hours of work of an expensive scientist for every 400 characters.
The latest technology, about which Andrew spoke, is the minanopores. It seems to me that in the scientific community there is still some doubt about whether this technology will become mainstream or not. But it definitely opens up new borders for us.
All previous technologies are considered fairly accurate, there are few errors in them, we are talking about percentages and percentages. This technology has significantly more errors, 10-20%, but you can read big ids. It seems that in large applications this is really necessary.
Whether they will be able to finish it up to a technology in which there will also be few mistakes is unknown. But if they can, it will be very cool.
The overall picture of sequencing, how it happens. A sample is taken from you, a blood test, a saliva test, or they are picked up with special tweezers, a piece of bone marrow is taken out: a very painful and terrible procedure. Further on the test, some chemistry is done, which removes all the excess and leaves, conditionally, only your DNA, which was present in the sample.
Further you do DNA fragmentation, you get small fragments that you further propagate so that there are more of them. This is necessary in many technologies. Then feed the sequencer, it reads everything in some way.
The output is always the same fastq file. All devices work differently, they have different lengths, different types of errors, and if you work with this data, it is important to understand what types of errors are there, how to work correctly with such data. If you apply the same method to everything, you will get dirty data that you cannot rely on.
Briefly about the method Senger. The idea is very beautiful. You have a DNA sequence, you have bred it, it is floating in your test tube. Then you want to understand what it consists of. Special proteins are fed there. Maybe the whole DNA will even float there, but the molecule that can disassemble it will also float.
Scientists have a very simple way. How did they learn to do such things with DNA? They looked at how nature does the same thing in our cells, understand what proteins they are doing, got them, and now they can replicate DNA themselves. Just take the proteins that are engaged in replication, and throw them into the tube. Everything happens by itself.
They throw these proteins, and they throw our ACTGs. Among them there are special marked ones, which, when she stuck to our DNA while reading, does not allow me to glue anymore. Throwing the whole thing up, shaking up and letting it stand for a while, we get pieces of unfinished source DNA, which we are interested in. We randomly stick something, and the hope is that for every length we have something stuck, at this moment the stopping TTP will stick and everything will stop us.
More special marked ACTG, fluorescent. If you dwell on them in a special way, they will be green, blue, red or yellow. Then all this is thrown into the gel, there they are distributed by mass, they are highlighted and get the picture, as on the right. Further along it can be calculated from the top down: the shorter ones are higher, the heavier ones are lower.
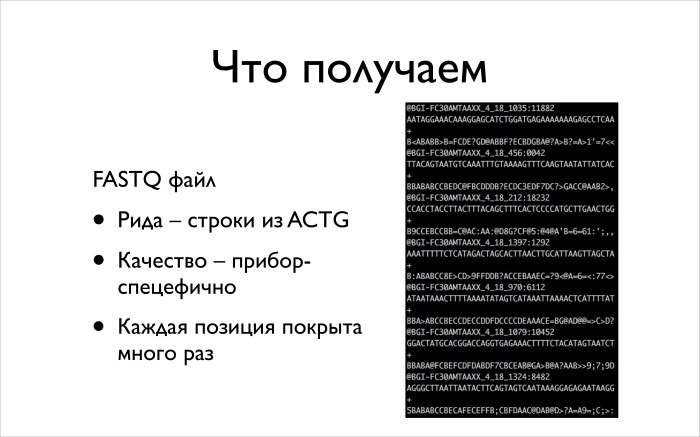
As a result, we get a fastq file. What it looks like is drawn on the right. This is such a text format that programmers should already be puzzled for - why should they be stored in text form when you can compress? So it happened. In fact, they are stored in such a file, they just press it with some KZIP. The file contains data in four lines: first comes the ID of your one read, read. Further letters that were read there. Then some technical line, and the fourth line is quality. All devices are able to give some indicators of how confident they are in reading. Further, when you will work with data, this must be taken into account.
The essence of most algorithms is that we read many, many times with large coverage random pieces. Further on, we want to understand what the original DNA looked like. It is clear that when people started the “Human Genome” project, they didn’t have any other genomes assembled. Bacteria then learned to collect. And it was a terrible task. You have the human genome, it has 3 billion characters. You have received a bunch of pieces of length 300-400, and now we need to understand from them what sequence there was from which such pieces could have come out. It looks scary.
Let's talk about the algorithms. What problems are solved with the help of NGS and how are they solved from an algorithmic point of view?

The first task is to assemble the genome. About her, you can read a six-month course of lectures, there are a lot of materials. I will outline only general ideas so that you understand what algorithms are used here, why it is difficult and interesting.
What is the task of assembling the genome? There are reads in the fastq format, there are millions or tens of millions. For example, you collect one chromosome. And you need to get the genome or chromosome that you want to collect.
Now, from a programmer point of view, how is this task formulated? You have short lines from the characters ACTG, they have some indicators of their quality, weight. And when you collect them, there are still restrictions on the answer. When biologists collected the human genome, they knew that there were such and such genes on chromosome 11: we studied them as a result of long work and found out in other ways that they are there. And I want to take this into account. It would be foolish to collect something, and then find out - what we are sure of, for some reason is not, because the algorithm decided so.
We need to get some kind of super string over all short lines. And we want it to be as small as possible. Otherwise, we can simply glue together all our fragments and get something that is not suitable for anyone. Since all the lines at the entrance were obtained from our chromosome, it means that we need to find this chromosome. If we did it with a sufficiently large coverage - conditionally, with each shift there is some kind of line - and we will collect the shortest, this will be our genome. This is not always true, there are various reasons why you can lose or miss something in this wording. But this is a sufficient approximation that suits everyone.

A simplified example of the problem. You can build a superstring that is shorter than their length of 16, and it may look like this. We took the last line of the SSTA, pasted the penultimate one to it, the TA letters from them overlapped. We thus saved two characters. Then to the speakers pasted the first line. TGAC , . , . .
. , , , NP-. , , : . : , - ? 2 10 — .
. , - . .
: . . , , , .
, , . 100 . , , . , , scaffold'. , .
. 80- 90-, , . 60- 70-, . , . -, , . , 80- , . , . .
? Overlap-Layout-Consensus. overlay-, , , , .
Overlay- — . , — — , .
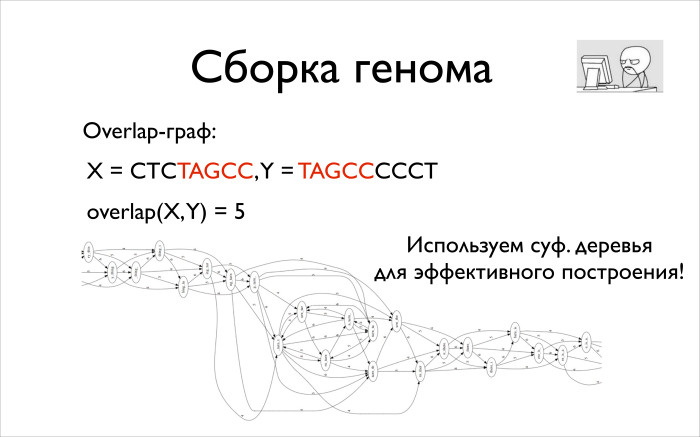
. 5, , .
, , . , , — . , overlap. , . , , , , . , .
1990 , , , . . , , overlay . , — . . overlay, .
, . , , . . , overlay.
, , , 100 1000. , , , .
, . . , , , . , . , , , .
, . , , , . , , . overlay , , , .
- . , .
. , 1989 . -, , , -, .
: , . , , , , . , .
, , . , k = 4, , , k= 4, , . - , . , , — , - - , - . .
— .
, k . , k = 2, , .
, , , , . , , .
. : , . . : , . - .
: , , , . , . , , .
, . . , , , , . , .
, . — , , , , . , , , . , .
, , , . : , . — . - , . , , . , , , , . . . , , , .
, , — . , , , , . , , .

. , . , .
? . , , , … , , . , , .

, , — . ? , . , . , , . . , , . , , . , . , .
, , - , , . , - , . , , , . , , - , - . - . .
: -, , -, . 10 . — - . , , , , , . .
machine learning. , , , .
.

. , , — . , , , , , , . - - , , , , , . - , , , , , . - , . , , , , .
, , - , . , . , , , . , , , - . . , : , — . . . .
. , , ? - , , , . , , , , , , . — , , , : , .
, . , , , -. . , , . , , , , .
, , , . , . , : , , , , , , . -, , , . -, , , . , - , , , , . , , , . , . , , .
: , , -. , . 2016 , , . « », , .
, . . , . Thanks to all.