
Big data today, well, BIG. The IDC study for 2016, entitled “
Semi-Annual Guide on Big Data Expenses and Analytics, ” predicts that global big data turnover
will grow from $ 130 billion in 2016 to more than $ 203 billion in 2020 , that is, cumulative annual growth will be at the level of 11.7%. According to IDC, growth is facilitated by three factors: an increase in the availability of huge amounts of data, a rich assortment of developing open source technologies for working with big data, a cultural shift in the business environment towards making decisions based on analyzing the data set. It sounds right, huh? And if we assume that this is not entirely true. Everywhere there are many reports of failures that comprehend big data initiatives. In this article, we will discuss the reasons for these failures, why decisions taken to remedy the situation are only temporary measures, and why autonomous data-processing platforms are a viable long-term solution.
Big data in “Getting Rid of Illusions”
In 2016,
PricewaterhouseCoopers (PwC)
research results state that the Information Value Index (Information Value Index), which characterizes the ability of large and small businesses to profit from big data, is on average 50.1 out of 100 possible points.
')
These obviously unsuccessful ratings place big data in the “Freedom from Illusions” zone, where certain technologies end up after a decline in inflated interest in them. In fact, in the PwC ranking, only 4% of companies scored more than 90 points. To achieve such results, they had to implement robust data management frameworks, create strong data-based decision-making cultures, and ensure the security of access to corporate data for those who need it.
The fact that so few companies are able to operate at a similar level indicates the immaturity of most corporate initiatives to use big data. To be mature in this area, companies need to come to terms with the complexity of big data.

The main reason for failure is complexity.
Big data technology is complex. Very complicated. There are a number of reasons for this. First, you are trying to solve difficult problems using huge - almost overwhelming - arrays of data. At the same time, open source technologies used to embed big data are usually designed with flexibility in mind. These are not commercial products, they have a lower level of support and service. They are not created as ready-made solutions to specific problems. And precisely because of their flexibility, they can be difficult and difficult to deploy.
In addition, when using open source tools one has to deal with numerous “moving parts” (moving parts). Six years ago, we only had to worry about Hadoop. Today we have Spark, Presto, Hive, Pig, Kafka and many other tools. All of them change frequently. Spark has had six significant releases since the beginning of 2015. Hive in recent years has also gone through several iterations of major changes.
Therefore, to keep the big data infrastructure at the latest level in a timely manner and to fix the bugs of all these open source products requires a lot of man-hours, which is why the productivity of work suffers.
Finally, as problems grow, you will not be able to take advantage of purchasing products from a single supplier. When it comes to support, training, troubleshooting, you have to work with multiple sources and depend on the open source community. And sometimes you can be left alone with your problems when using open source tools for working with big data.
Modern solutions to the challenges faced by corporate big data initiatives
Of course, the industry is trying to solve all these problems. But modern solutions for the most part are temporary measures and are ineffective in the long term. Let's look at some common solutions to problems with the complexity of big data.
Redevelopment of open source technologies
Some vendors reconfigure open source technologies to reduce their complexity for the business. For example, they can build their solutions based on specific uses. This reduces the complexity, because the proposed solution is designed to solve a specific problem, and is not a universal tool for working with big data. But this approach has a downside: to create and launch a project, you may need several such specialized solutions. For example, if you are going to be engaged in data processing, as well as their conversion and analysis, then you will have to buy three smaller reconfigured solutions. And their total complexity may be no less than that of one universal solution.
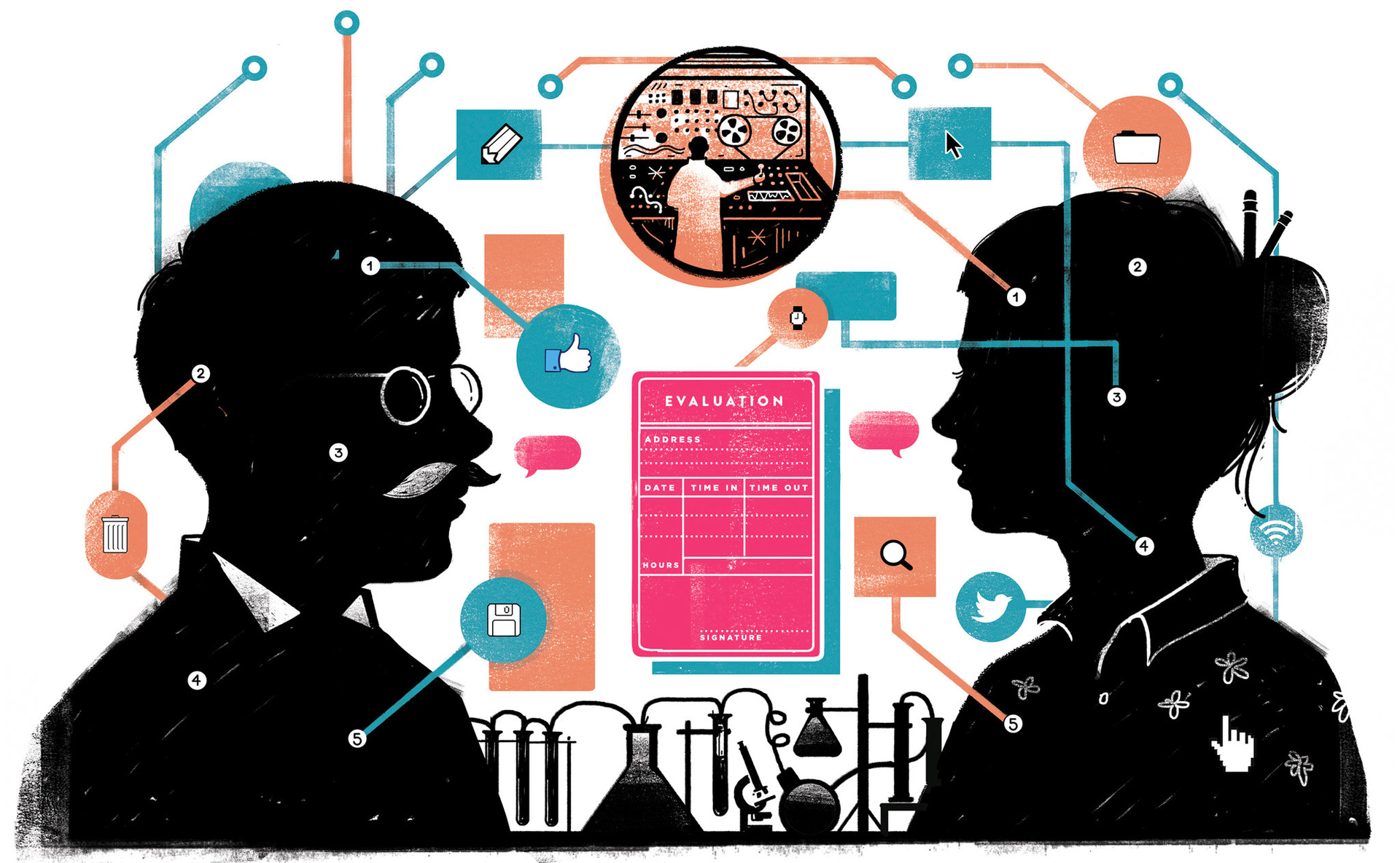
Quick Start Programs
The so-called quick start programs are generally developed to help you get started faster. But they are intended only for initial deployment. Alternatively, some vendors offer deployment templates. These are specific reference books for specific cases of working with big data, in which some proven techniques are described step by step.
The problem is that such solutions are designed to run the work, but will not help you cope with the further complexity of big data. And they will not help you in keeping your infrastructure up to date as technology advances.
Clusterless solutions
There are vendors who are trying to hide the complexity by separating the core infrastructure from the workload - they exclude the very concept of clusters.
If you use on-premise infrastructures for working with big data, you usually use several large clusters (for example, one for development / testing, the second for production). But it is very difficult to plan the joint execution of several tasks in a cluster for the sake of maximizing the efficiency of use, and hence ROI. On the other hand, if you bet on the cloud, you can, for example, use its adaptability: form a 20-server cluster, use it for four hours, and then disband it. So you can more flexibly manage costs and productivity.
But such an approach requires appropriate qualifications. You need to know what the optimal cluster size is for a particular workload. You must understand the types of servers, install and test the appropriate software.
As a solution to this problem, many vendors today offer "clusterless" solutions.
Amazon Athena , the cloud-based data store
Snowflake and
Cloudera Impala (currently the “incubator” Apache project) - they all abandoned the concept of clusters. You just need to specify the product for your data and start to perform requests.
Clusterless solutions are easy to manage. However, they all have a multi-client (multitenant) structure, that is, all users are served by one common infrastructure. So even though they disguised the idea of a cluster, in fact, it’s still the same set of servers running software that many users use. And most of them are not even your colleagues. Therefore, vendors of noncluster solutions impose certain restrictions so that your workload does not interfere with the tasks of numerous environments. This reduces the flexibility of application: it is necessary to limit the number of requests so that they do not consume too many resources. In essence, complexity is hidden from users, but manageability from an IT point of view is reduced. This affects both cost and service-level agreements.
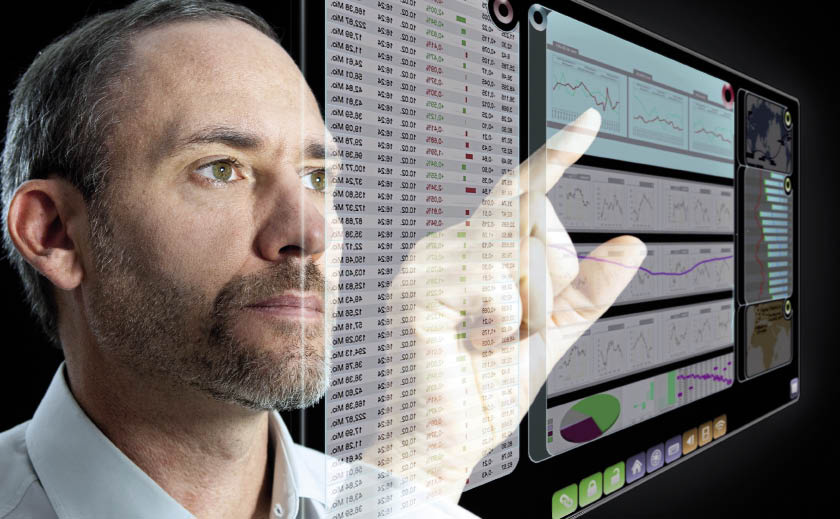
People
At first glance, the only viable long-term solution to the problem of complexity that does not require sacrificing flexibility is to hire more employees as your initiatives using big data grow. But for two reasons, this in itself is a problem.
Today in the industry there is a strong shortage of qualified professionals. There are not enough big data specialists and database administrators. They are hard to find. They are hard to attract. And it's hard to keep them if you hired them.
In 2015, a study was conducted, according to which 79% of companies noted a shortage of specialists to large data. In 2016, the
situation worsened . Already, 83% of respondents noted difficulties in finding the necessary talent.
Even if you run a big data project, and you have a team with the right knowledge and skills, as you increase the number of users, expand your application park, increase the number of departments you serve, workload and application methods, you will need more people to maintain the infrastructure.
Big data itself is a scalable technology. You get a commensurate benefit from adding to the infrastructure of any computing power, storage or other resources. And because of the scalability of big data, companies can now think in terms of the linear cost of processing unlimited amounts of data.
However, due to the complexity of big data, you will have to hire additional employees each time you add a new type of workload, or introduce a new method of using technology. And people - the resource is not scalable. By adding one person to the team, you won’t get an increase in efficiency as from one person. You will get less because of the overhead of communication, collaboration, processes, management, administration, and HR.
Difficulty is a silent killer of big data, and the reason why in the long run you will not be able to rely on the use of human resources.
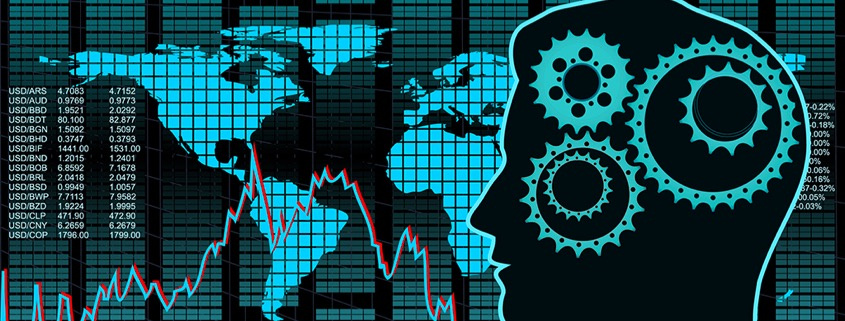
The growth of intelligent, autonomous data processing platforms
Intelligent automation of data processing platforms can be another long-term solution.
A standalone platform is a self-managing and self-optimizing infrastructure for working with big data. She learns how to perform these or other tasks as best she can, using examples from users. The platform keeps track of which queries you make, which tables you use, how many clusters you create and how effectively you exploit them. In general, the standalone platform examines everything related to using the big data infrastructure to solve business problems, and analyzes the information gathered to help you further improve.
This is much like unmanned vehicles. A car that can drive in a variety of conditions - in heavy traffic, in snow, rain, with a strong side wind - and partly compensate for the difficulty of creating truly autonomous cars. But manufacturers reduce this complexity by highlighting specific aspects of driving that they can automate. The simplest solution is cruise control, which has long been used in production cars. And today, cars have already appeared on the roads, capable of operating independently in very difficult road conditions.
A fully autonomous data processing platform is also an unreached goal. But vendors slowly automate the solution of individual problems and tasks.
Autonomous platforms will develop in three stages. At first warnings, conclusions and recommendations will be automated. Then come policy based automation. Finally, we get completely autonomous platforms. Yes, today it is only a concept. But it will be implemented.

Warnings, conclusions and recommendations
•
Warnings - a passive form of intelligence. The platform will provide information about the infrastructure, but it will not do anything. Warnings can be: “Do you understand that the cluster is working, but the data is not processed?”. You can answer: "Yes, you need to kill the cluster" or "Now I will start using it, so I left it working." The warning only refers to a situation that looks extraordinary. And you have to decide what to do with it.
•
Inference is the result of an analysis. The system takes an extraordinary situation, analyzes it and comes to a conclusion. For example: "Sergey makes the most resource-intensive requests among all users." Like warnings, the conclusions are passive: the system only offers you information, but does nothing.
•
Recommendation - the next level after the withdrawal. The system takes the result of the analysis and on the basis of it produces a recommendation for action. For example: “This field is often used as a filter in queries or sort fields. Indexing it can improve performance. ” But this is only a recommendation - the platform will not automatically take any action.
Policy based automation
Policy-based automation is when you give the infrastructure a set of guidelines or configuration parameters, based on which the system performs some actions. That is, you configure the policy, and the system acts in accordance with it.
For example, you can customize policies for the “purchase” of computing power to ensure optimal spending. In most cases, clusters are formed on the basis of a single type of server (by a combination of processor, memory, and disk space). The reason is that the underlying technology was originally designed for on-premise deployment, when IT acquires identical servers for the data center.
In the clouds, however, providers offer many configurations from different machines, each of which has its own recommended prices. AWS in the spot market sells unused capacity at auctions and you can place your bets on nodes. Often, the demand for AWS is so low (for example, in the middle of the night) that you can buy nodes for 10% of the normal price. Cool, yeah? But there are two "but." First, if the demand is high, you may not find suitable servers on the spot market, because availability can vary greatly. Secondly, AWS may confiscate spot nodes from you if another customer wishes to pay an “on demand” price (normal price). Therefore, the spot market is an unpredictable thing, there are no guarantees that you will use the nodes you need for the necessary time.
Another example of policy-based automation.
Qubole has a spot agent who, on the basis of the policy you set, “buys” in compliance with the optimal ratio of price and performance. If necessary, the agent combines servers of different types and automatically places bets for you. Politicians can be very complex, and since they can run all night and work instantly, it’s better to let the machines do the work, not the people. This is not like intelligent systems for trading in the securities market.
Fully autonomous automation
Finally, we get a completely autonomous automation, in which the platform collects information, analyzes, makes decisions and acts without asking for our permission and not being guided by policies.
For example, the platform may notice that there are a number of queries using a specific field, and determine that indexing on this field can improve performance. Also, the platform can determine that if you break the table in a certain way, it will also increase performance. It can determine that the cluster is too small and does not satisfy the conditions of the SLA, and dynamically increase it. And since this is a “closed cycle”, the platform will be able to evaluate the results of its actions.
Today, teams working with big data are forced to proactively monitor such opportunities, make changes manually and manually track the results. In reality, many do not have the time or throughput for this, except for the most important or difficult situations. So a self-optimizing platform will help big data teams focus on business results, rather than on finding problems and tuning performance. They will also be able to solve problems for which they rarely managed to allocate time before, such as carrying out numerous minor improvements, the cumulative result of which will be of great importance to users.
Difficulty choking big data, but the future seems bright
The ultimate goal of any company is to benefit from all the data it has, make it available to all employees who need this data to make decisions, and use these solutions to improve the company's business.
With the growth of automation, teams working with big data will be able to solve more important, more valuable problems. They will be able to concentrate on the data, rather than the platform, to extract the most value from big data.
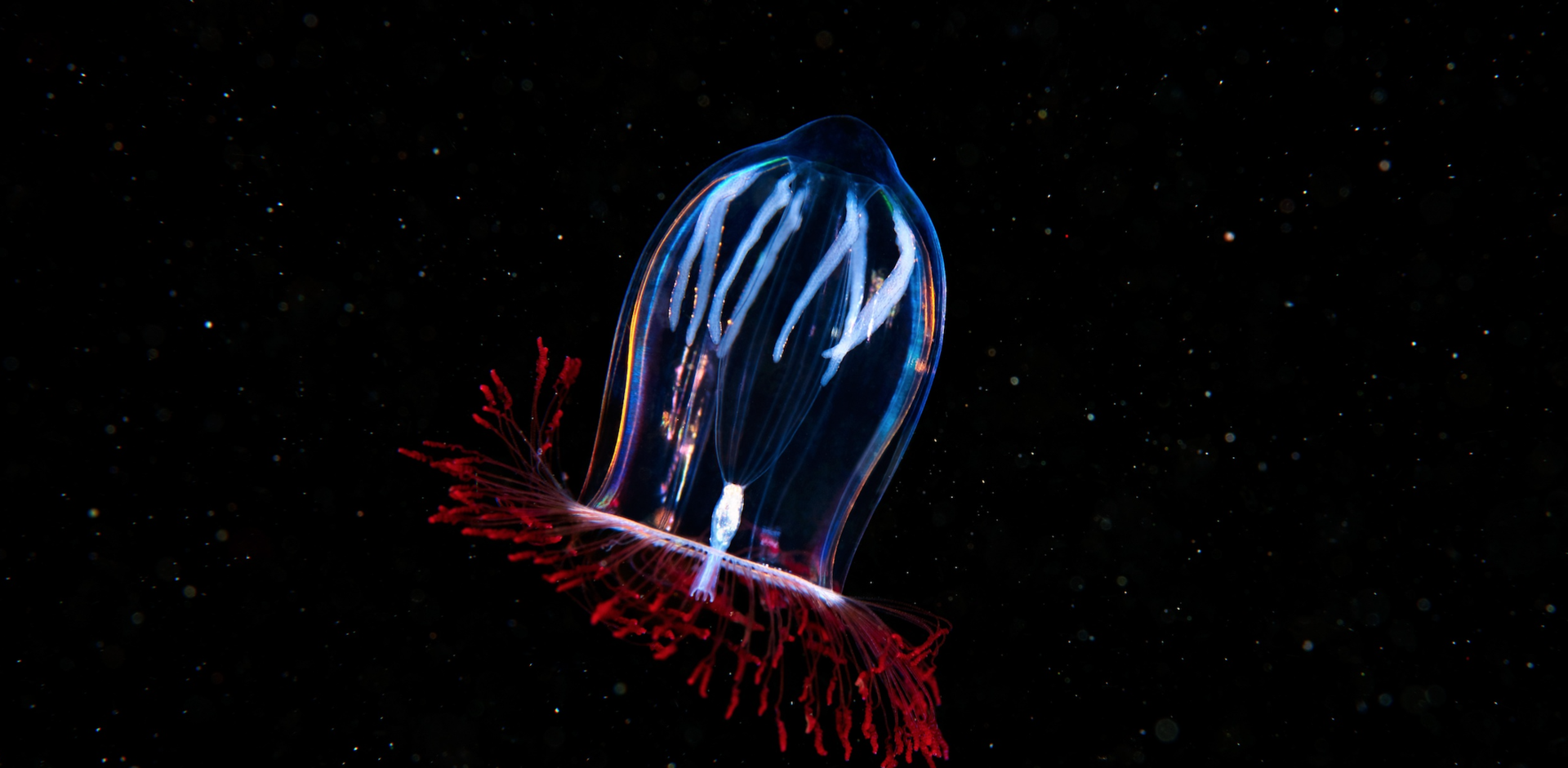