When protected digital data begin to open and become available to a wide range of experts, the information space is enriched and heated. At the same time, it is structured by topics and groups by the efforts of many analysts, researchers and experts, organized and aligned along the lines of priority trends, optimized and develops new approaches, technologies and models for solving problems.
There is no doubt that with this the number of alternative options grows, and the choice of the most effective one becomes more complicated.
Let us dwell on some interesting issues of the future development of public data.
')
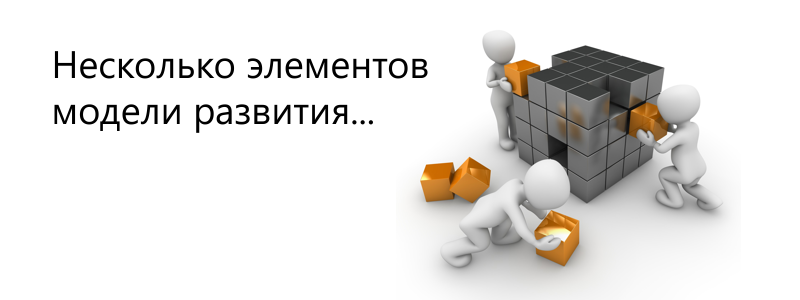
The article is based on the approach and terminology identified in the series on the subject of public data.
Links to previous articles
Competences and tools
The implementation of large-scale and full-fledged work on the delivery of public data, as well as their use, requires special competencies and tools.First of all, this is due to the peculiarities of the public data transfer scheme, which does not provide for sustainable personal interaction between the supplier and the recipient. And accordingly, as the supplier itself is forced to perform some additional work, the recipient implements a number of procedures that would simply not be necessary with the closed exchange of data via stable communication channels and with a more detailed agreement.
The required additional competences and tools for working with public data can be divided into several categories:
- Processing the data content - to bring the meaning of the data to the desired target type, including the need to reduce data volumes, change their qualitative composition, etc .;
- Upload / download data - for efficient transmission and reception of public data from the supplier to the recipient;
- Data restructuring - to change the data structure and bring it to the target working view;
- Reformatting datasets - to convert to the desired format at the level of encoding, notation or schema;
- Data quality audit - for professional assessment and verification of quality indicators of digital data sets, both at the stage of publication by the supplier and at the stage of receipt by the user;
- Metadata management - for receiving, processing, validating and reformatting metadata accompanying basic data;
- Linking to the context - to organize links to context data and formal description of the recommended context "zone";
- Organization of procedures - for planning, implementation and control of business processes for the supply or receipt of public data;
- Development of the model - for designing, changing and coordinating work with public data within the framework of a common activity, as one of its components.
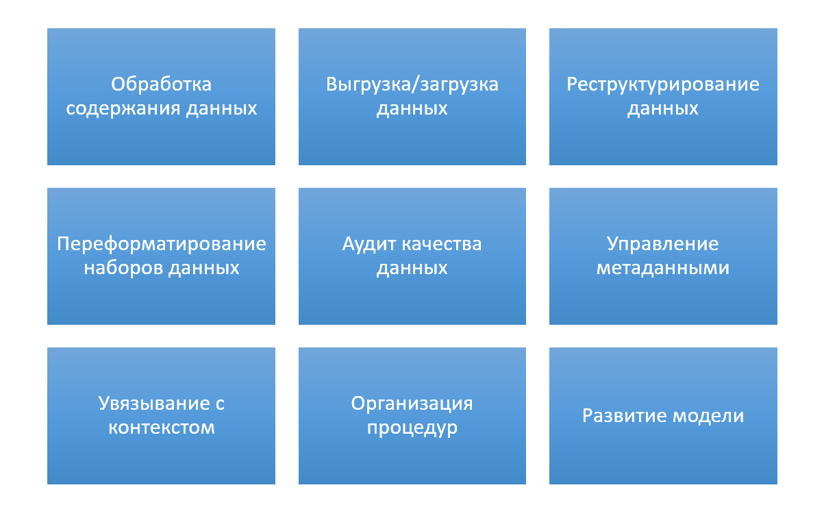
By and large, the relevant competences and tools should be based on some
full-fledged standards , since in the field of public data it is quite difficult to organize direct communications and pronounce specific moments with each of the suppliers or recipients. But the very development of standards is significantly complicated by the potentially large and unlimited number of participants. In this case, the state regulator or an organization (association) authorized by the professional community can act as an arbitrator.
In the absence of standards, the subject independently makes the appropriate decisions and determines for himself those or other priority areas of regulation.It is also important to mention the division of competences and tools into three categories:
- General purpose are universal competences and tools for working with data, the use of which does not depend on their content and purpose;
- Special assignments are specialized competences and tools, the use of which is associated with a specific subject area of ​​public data;
- Special (one-time) assignments are deeply customizable or task-specific competencies and tools used in individual special cases related to one-time or unique jobs (their reuse is unlikely).
The problem of training, practical training of specialists and the subsequent preservation of competencies within the business, as well as the problem of finding or developing and effectively using practical and productive tools in practice, should be solved
systematically, consistently and persistently . Businesses that seek to work with public data and benefit from it should not neglect these things, but, starting with strategy and ending with operational activities, are obliged to create appropriate competencies and be equipped with tools.
An independent independent expert will experience even greater problems in these areas when he tries to get good analytics and good results on public data. Of course, the more help the data providers or professional expert communities provide him with affordable access to knowledge and tools at affordable prices and conditions, the more stable and high-quality effect will be obtained.
This is another argument in favor of creating a whole system of “feedback” of public data providers in its full scale and breadth.Involve more not only business users of public data, but individual experts, journalists, researchers by reducing entry barriers and any kind of costs, both when using public data and when it is delivered.
Littering of data and activity of suppliers
The call to open data and provide free access to them is quite understandable and justified. But imagine if all economic entities immediately follow it. In the absence of real joint agreements on the rules for publishing data and their application expressed in generally accepted formal documents such as standards or regulations, we will immediately
garbage the network space and nothing more.
Public data do not require haste and also need a high-quality professional solution, like any other information problems.
Here are two non-trivial questions with which by and large should start:
- How much and what data do we need?
- How much and what data can we supply?
Object orientation is important for public data, just as it is important to supply
quality data in a generally applicable format.
You can talk about different motives for the free disclosure of digital data. The worst of them is not an attempt to throw in fake data, but a sincere desire to put huge data arrays into the network - it is not clear who needs it and obviously the quality with high-speed publication will be low.
It is much more important to publish related data and maintain their relevance.And here we cannot do without specialized automated tools.
The activity of public data providers should not be measured by the number of sets “thrown” into the network and not by the frequency of updating, but covered by the subject area and the quality preserved during updates.
Publication of “garbage” - poor quality, poorly structured and poor in data content - causes the main reputational blow to the whole concept of free dissemination of information and digital data, and not only worsens the ratings of individual owners and suppliers of “numbers”.This suggests the need for some regulatory and auditing organization or community whose task should include authoritative expertise and assessment in the field of public data. Best of all, if the most significant participants of the process on the part of the owners, suppliers, recipients and users of public data can develop framework rules and conditions based on the results of the development of this area.
In the end, the trash has someone to clean up.Security and Public Data
If an economic entity is offered to lay out for free access any data related to its target or economic activity, it will immediately begin to worry about its commercial or reputational security. There are grounds for such concerns, but they are necessarily eliminated at the level of a
professional public data supply management system (if there is one).
On the other hand, if it is not planned to get a significant effect from the supply of free data, then it is better to take care of the peace of the security services and isolate the subject’s internal data from external users - which is what most businesses do. This is only the “internal” side of digital public data security, but there is a more common aspect for the community as a whole.
The problem of security lies not only in the public data itself, but in the possibilities to use the data for the implementation of various types of crimes.Intellectual criminals may well use a variety of data on the location of the victim, on his material condition, on the localization of places unfavorable to the victim. Public data may not be the main, but the linking information for blackmail. Leakage of important information provokes the activity of scammers and scams. In addition, the very fact of publishing false data (albeit highly qualitative) can push not only criminals into action, but also create social tensions. Manipulating public opinion with the help of “as if actual, authentic data” is quite likely and will be a more subtle, but professional and reliable (convincing) way to manage social groups. Intermediaries for this already have - social networks.
Special measures are required to protect respectable businesses, non-profit organizations and citizens from attempts to use public data for illegal purposes in relation to them. Government regulators are already following this path, starting with personal data. Of course, the institute of state and commercial secrets existed before global informatization and will exist, but with digital public data the situation is somewhat different: it is not the protection of information from dissemination that is required, but the protection of subjects from the unauthorized use of free data. And this is a completely different story.
For their part, in order to improve their own security, businesses can and should even expand the range of tasks and
risk management technologies, especially those that are actively involved in the supply or receipt of public data. There are new risks that should be identified, assessed, monitored, compensated and eliminated.
Compliance with the legislation in the field of data publication is a complex security issue, which is tied to a number of NLAs of specialized, non-core, subject and other. In fact, the likelihood of violating a regulatory framework is large enough. And the question is not to do everything cleanly and correctly the first time, but to respond as quickly as possible to the claims and eliminate the violations until they cause serious material and moral damage.
Knowledge-mining on the public data field
The development of a data publicity system and the gradual saturation of the global information network with digital sets suitable for work poses a rather complicated but interesting question about the
extraction of knowledge . This is a kind of technology and at the same time a strategy for the gradual collection, processing and analysis of digital data to gain new knowledge.
Approximate scheme:
- We determine the problems - we formulate the subject area, point of view, targets, criteria, etc.
- Find the “entry point” - we define a limited number of data sources and target sets of public data that are subject to processing.
- We receive and process data - we accept data and perform all the necessary procedures for preparing and analyzing data.
- We formalize the knowledge gained - following the results of processing and analyzing data, we construct hypotheses, check them whenever possible, build conclusions and formulate rationales, record facts and phenomena, visualize the knowledge gained and describe them formally.
- We define “problem points” - we find in the knowledge gained controversial places that require proof or extended study and select the most important and interesting.
- We find “extension points” - we select additional sources of digital data and the sets themselves, which allow us to supplement previously collected in the same subject area
- We supplement the available data - we accept new data and re-perform the procedures for preparing and analyzing data using previous or new schemes
- We expand formalized knowledge - following the results of repeated processing and analysis, we complete hypotheses and prove them on a larger data set, finalize conclusions and substantiations, expand the depository of facts and phenomena, update knowledge and visualize them at a new qualitative level.
- We again define the “problem points” - we return to the cycle in step 5 , but at a more competent level.
One way or another, this is how the process of extracting knowledge from public data in the global network looks like. Of course, the linearity and conciseness of the description does not in any way characterize the simplicity of the extraction of the useful effect from the "layers of numbers."
The most interesting thing is that the extraction of knowledge from public data in the global information space is an endless process with the possibility of its repeated implementation on the same resource. If for the usual extraction of resources there is only the only possibility of processing primary raw materials, then for the extraction of intangible knowledge, nothing prevents to use the same data sets many times - and, grinding as many times as you like, the “figure”.
Perhaps even the term “knowledge extraction” gives an incorrect idea about the described process. The concept of mining is usually associated with material resources (raw materials) and forms some kind of mechanistic picture, while the extraction of useful knowledge is based more on methodology and scientific creativity, on the talent of a researcher and a certain amount of luck. Nevertheless, as a process of extracting something useful from a certain aggregate volume, from a solid and complex mass, the idea of ​​knowledge extraction is quite convincing.
In order for the extraction of knowledge to be carried out constructively and not turn into a "hellish work in the quarries" a lot of effort should be made to suppliers of "raw materials".
The publication of high-quality public data helps to avoid many problems , but the main thing is to avoid unproductive costs of bringing the data to the desired correct state.
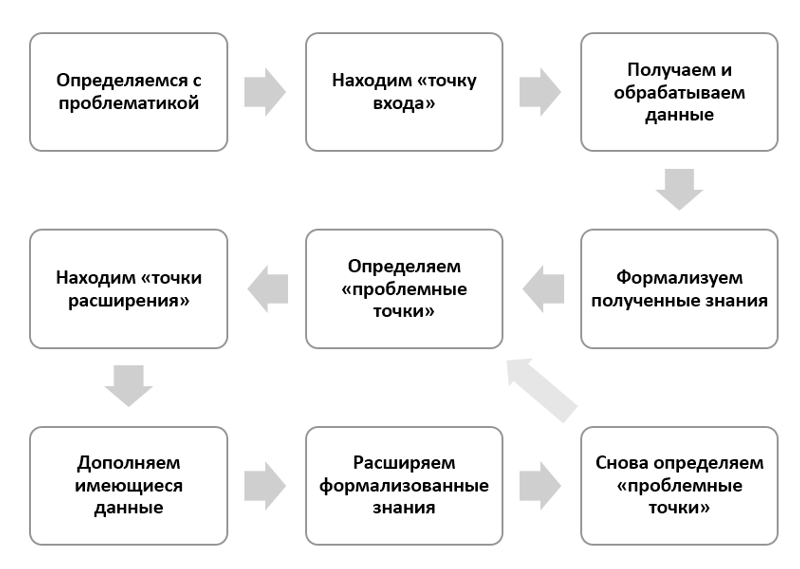
And when we touched upon public data providers, we have to immediately recall the
main motivation for free disclosure of data , which forces us to speak not only of direct knowledge, but also indirectly.
All that has been described above is the direct extraction of knowledge from public data by direct users.
The indirect extraction of knowledge from public data is to obtain a supplier of new knowledge created by recipients based on its data. Indirect knowledge mining is implemented by user feedback mechanisms and technologies. In this case, the supplier is forced to develop the community and the system not so much working with its data, as knowledge in the target domain based on access to public data.
Accordingly, for the user and for the supplier of public data, the concept of “knowledge-mining” is equally important, but it is implemented in other ways and is gradually being formed into powerful technological directions of development.
Data Lab
Since public data is useful and attractive, it is likely that they are able to form new markets with new consumers and products. We denote conditionally the business in the field of public data by the term
“data laboratory” .
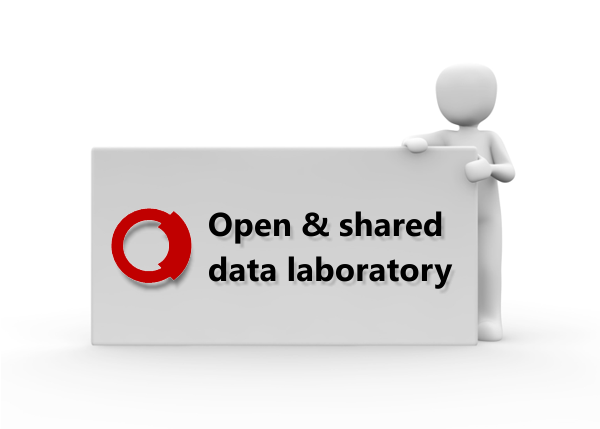
What could be the range of services provided by such a "laboratory":
- development and sale of specialized competencies and tools for working with public data;
- research of sources of public data and individual sets, development of expert recommendations on sources and sets;
- auditing public data on quality, quantity, metadata and context, as well as auditing the sources of digital data sets;
- formation of competences and knowledge base in the field of public data;
- maintaining a registry of open and shared data (with verification by parameters and the ability to apply for inclusion);
- preparation and training of specialists in the field of discovery and data sharing;
- recommendations for individuals on the delegation of data;
- aggregation and consultation on regulatory acts;
- formalization of the basis (regulations, standards, model documents) in the field of public data;
- direct subject analysis of data on order and on its own research program;
- reduction of entry barriers to the public data business (both for suppliers and recipients);
- building batch services for obtaining public data through the API;
- initiating projects based on data packages, illustrative examples and ideas, proposals and evaluation of initiatives;
- a study of the risks associated with the publicity of the data, and the development of recommendations, ways of minimizing, methods of eliminating the consequences of adverse situations, etc .;
- the initiative to create and actively participate in the development of the public data community as one of the areas of the digital economy;
- development of the public data concept and its popularization.
Based on the above, it becomes clear that the “data laboratory” is rather a functional subsystem, although it can be implemented through an independent business. And this is a business of creating and / or supporting new projects (initiatives) in the field of public data.
The focus is not the sale of data, but the sale of knowledge about public data, tools for their publication and use in a full or specialized set: software applications, technical documentation, training, templates, techniques, best practice, etc.Data Laboratory is a new type of research economic entities that base their activities on intangible assets and work in the field of open knowledge. In order to make public data truly accessible to everyone, services for their processing and presentation, their audit and localization are needed. This task of the laboratories is based on the need to reduce transaction costs in this area. What is possible only on the centralization and automation of some of the mandatory interactions of objects within the business model.
Another feature of such an organization is informational openness in the processing, analysis and dissemination of secondary data (information). The functionality and results of the data laboratory can be closed only in terms of the development of specific competencies and tools, but in general, in order to maintain active development, it will have to be made quite public, i.e. information open to an unlimited or conditionally limited circle of people on a wide range of issues.
Organizations like
data laboratories will reduce costs for other participants in targeted processes for linked transactions for publishing digital data sets and / or their implementation. They will allow creating a single high level of competitiveness using open, shared and delegated data, thus setting a certain margin for the market in the relevant direction.
Open and Shared Data Portal
Public data, especially open and shared, it would be good to collect in one place. Some kind of general orderly and even managed storage is a portal of digital open and shared data.
The portal, as the centralization of public data, is an important resource that must be actively developed and widely. But the question of who will develop it is not entirely obvious. Implementation at the state level will be limited only to open data, which will of course be correct. There is no need on behalf of government agencies to publish the data of individual commercial organizations or even to provide them with a place for this. Taking responsibility for the creation of a single portal of shared data by a separate business will be quite an ambitious and costly task.
It is possible to post on the public data portal:
- copies of sets of published digital data;
- links to published digital data on other network resources;
- API links for which you can get data;
- intermediary APIs by which you can get data stored on the portal or data published using third-party program interfaces.
Moreover, access to the portal data can also be implemented alternatively in static and / or dynamic ways. That is, users who are ready to receive data using special software tools will use the API, and all others simply upload files.
Obviously, the value of the portal increases many times, if it does not just list some hypothetical digital sets, but gives a detailed description of them.In other words, portals must accompany each set
of data
passports with a description of the quality of the data set, indicating the source, key metadata and in relation to the context. If at the same time all this is accompanied by an expert assessment of public data and an audit of not only the structure and format, but also the content, then such a portal will certainly be a success. However, this is quite laborious, and the option of storing copies of public data directly on the portal also requires corresponding technical resources.
One of the possible ways to optimize the functionality of the public data portals is a
directory . In contrast to the full portal, the catalog only includes some links to other network resources and accompanies them with the necessary limited description. As one of the options for organizing digital data sets, the catalog can be successfully used, but as a complete system that opens access to various collections and digital data packages, it loses much to the portal model.
Unfortunately, the search engines are still quite well looking for simple textual information on demand, but are not focused on finding data sets or data within sets. However, at the present time, not quite fully functional public data portals (as understood by this publication) have appeared, but rather their prototypes and experimental samples. The information environment in the global network is developing quite quickly and it is possible when the public data gains full strength, we will see several large projects combining them according to a thematic or regional principle.
Nevertheless, even public data organized in catalogs or collected on portals remains the field of activity of large business or individual expert analysts. For their effective use in medium-sized or small businesses it is not enough to provide direct access to the “open figure”, you will need to equip managers with productive tools and, equally important, to transfer the relevant competences to them.
Public data network
Sooner or later, but public data will be forced to move to another quality level.They will transform into standard structures. They will be cut to standard formats. For them, create a convenient productive storage sites. And, of course, they will be linked together by a variety of managed links. With the growth of linking links between different sets of public data, both general and intra-structural, a whole network of digital public data will emerge. For the development of such a network, it will be necessary to develop a number of practically significant standards, at least in terms of the format and content of inter-package and intra-structured links. Standards will also be needed to create high-quality metadata and link to contextual information.
The network is an order of magnitude more complex public data model, and its requirements are raised mainly in terms of providing high-quality and complete metadata.This in turn requires the development of such a direction as “digital metadata”.
Unlike the portals, the network not only groups public data, but they are connected, supplemented, enriched. Moreover, the binding is possible and legitimate for any data type: open, shared and delegated. The main thing is that this connection should be carried out according to the set rules and solved on the basis of clear procedures.
But the network is a data interconnection model, but not an “entry point” to related data sets. Portals should provide such “entry points” and give a slice on the subject, structure and format of public data.Communities of public data is a necessary driving force in the formation of a system of public data and the search for new knowledge, it is the embodiment of the idea of ​​a network of public data at the level of interaction of interested parties.
: . , , .
., .– , . «» , , , . () . , .
–
. - : , . .
. . , , . .
. «» , , , .
– . , , ., . «» «».
– , .