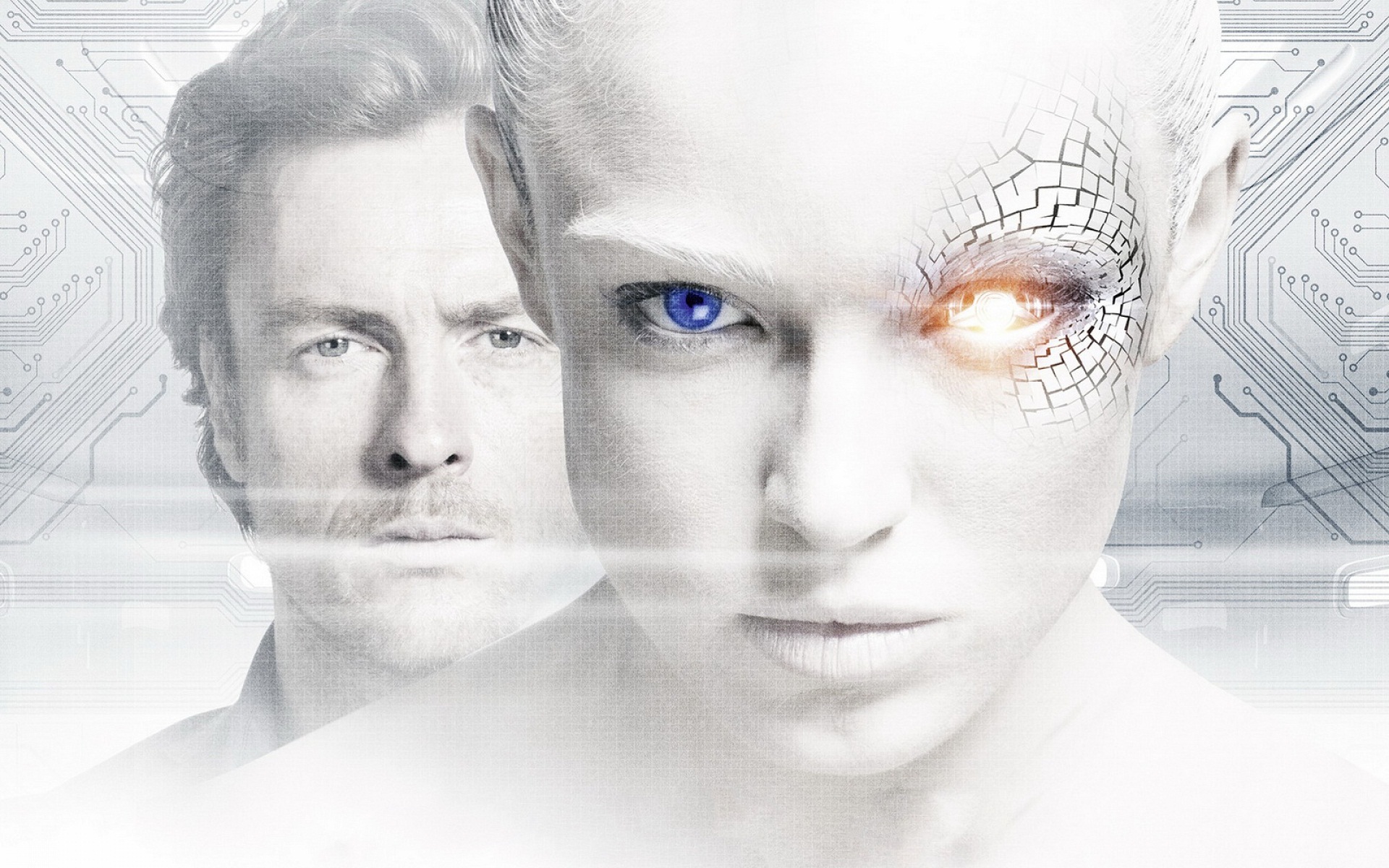
As many of you know, in 2015, Stephen Hawking, Elon Musk and hundreds of scientists, developers of artificial intelligence and major businessmen
signed an open letter in which they stressed the danger of AI for the existence of mankind and called on the community of engineers and scientists not to create artificial intelligence that cannot fully controlled by man. In 2016, at the Code Conference conference, the founder of Space X and Tesla was asked a question: which companies developing AI today are of concern to him? He replied that now
only one of them is afraid of him. What - did not say. And despite all the assurances of the techno-adepts that the AI ​​is exclusively good, the price of neglecting the security mechanisms may turn out to be exorbitantly high.
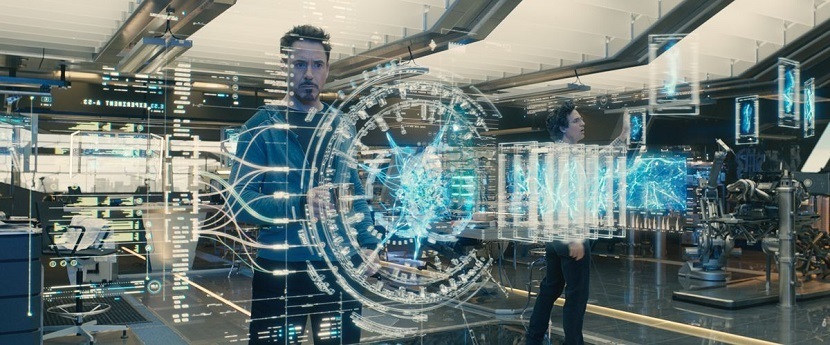
')
Perhaps one of the reasons for the controversy regarding the risks associated with
AI is the skepticism of scientists and IT-businessmen regarding each other. To one, it seems that others are completely ignorant. There is also a third party - government agencies, in which they believe that business does not see beyond its nose, and scientists are in the clouds. Moreover, both businessmen and scientists perceive officials as a bureaucratic swamp. So all three communities do not understand each other and pull the blanket over themselves.
Nevertheless, among representatives of various communities today, there is a growing understanding that we cannot deploy and use intelligent solutions, machine learning systems or cognitive computing platforms if their “thinking” process is not transparent to us. We need
to know what they think .
Today we tend to blindly trust the results of the systems, the principle of the majority of which we do not understand at the algorithmic level. This is valid for the Facebook identity feature, but completely unacceptable for systems that develop solutions within the framework of very important and valuable business logic, goals and priorities. And our livelihoods depend on the latter.
Today, for some of us, the value of AI-technologies is determined by the creation of machines that can explain the existence of themselves and the world around them. And one of the most important moments is the transparency of thinking of intellectual and analytical systems. Some time ago, it seemed that many developers were neglecting this criterion in favor of performance, but fortunately, in recent months, the issue of AI transparency has been increasingly raised.
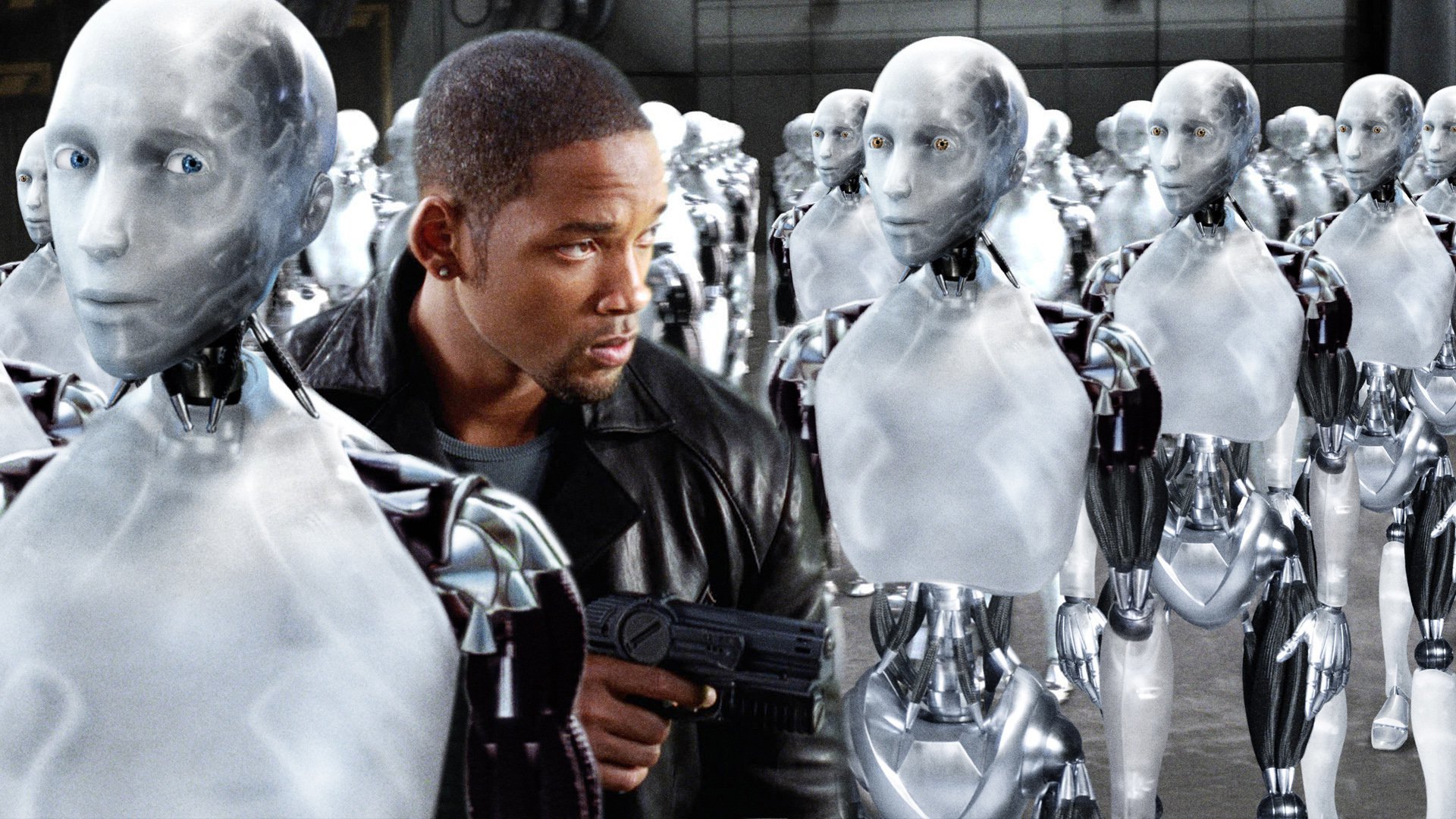
For example, in an academic environment, models for unpacking the results of the work of in-depth training systems are being discussed, as well as ideas related to evidence-based approaches to the process of thinking of AI. In the business environment, top managers are increasingly asking how to deploy training and decision-making systems, whose line of reasoning is completely unknown. Essentially, they ask how they can use software that no one can understand? And the last straw was a recent announcement from DARPA, in which the interest of the agency in the work on the "explainable AI" was voiced.
Obviously, more and more people are aware of the need for AI transparency. But how can we achieve this? Some technologies, such as depth learning models, are so incomprehensible that even among practitioners there are disagreements about how they work, if we go a bit beyond the limits of the specifics of their algorithms. What can be done in such situations?
In the long run, it is necessary to focus on developing systems that not only think, but that can
think and explain . In the meantime, we are waiting for the emergence of such developments, we must adhere to several rules for the development of existing systems.
First of all, do not deploy the intellectual system if you cannot explain the course of its reasoning . You have to understand what she does, even if you don’t understand how she does it on the algorithmic level. This is a necessary, but not sufficient condition, because you will only understand what data the system needs, what it is going to make decisions, and on the basis of what reasoning it comes to these decisions. In addition, there are three more important levels of opportunity.
Explain and discuss . I wish that the systems could explain and discuss their train of thought. We need AIs that can clearly and consistently tell how they came up with a specific decision and what were the alternatives. For example, a system designed to detect vendor fraud should be able not only to issue a list of signs on which a warning was issued, but also to explain why each of the signs indicates a fact of fraud. Since some indicators may be outside the data set or not included in the system model, it is very important to be able to offer them to the system and evaluate their impact. The opportunity to ask: “What about X?” Is important both when working with people and with intellectual systems.
Formulation Even systems that cannot be manipulated by end users should be able to, at a minimum, formulate the characteristics and scope of the reasoning itself. This does not mean that simply throwing out 10 thousand of evidence that led to some conclusion. Systems should be able to at least highlight the truly relevant characteristics, as well as describe their relationships. If the system warns the user that it has detected an example of fraudulent behavior, then it should be able to identify a set of adverse transactions on the basis of which a warning was issued.
Testability If the system does not provide explanations in real time, or does not know how to formulate cause-and-effect relationships, then its solutions should at least be verified afterwards. The logic of its actions must be tracked so that you can then investigate the circumstances of any problematic or controversial situations. Even if the end user does not have access to tracking, the analyst who developed the backend should have this opportunity.
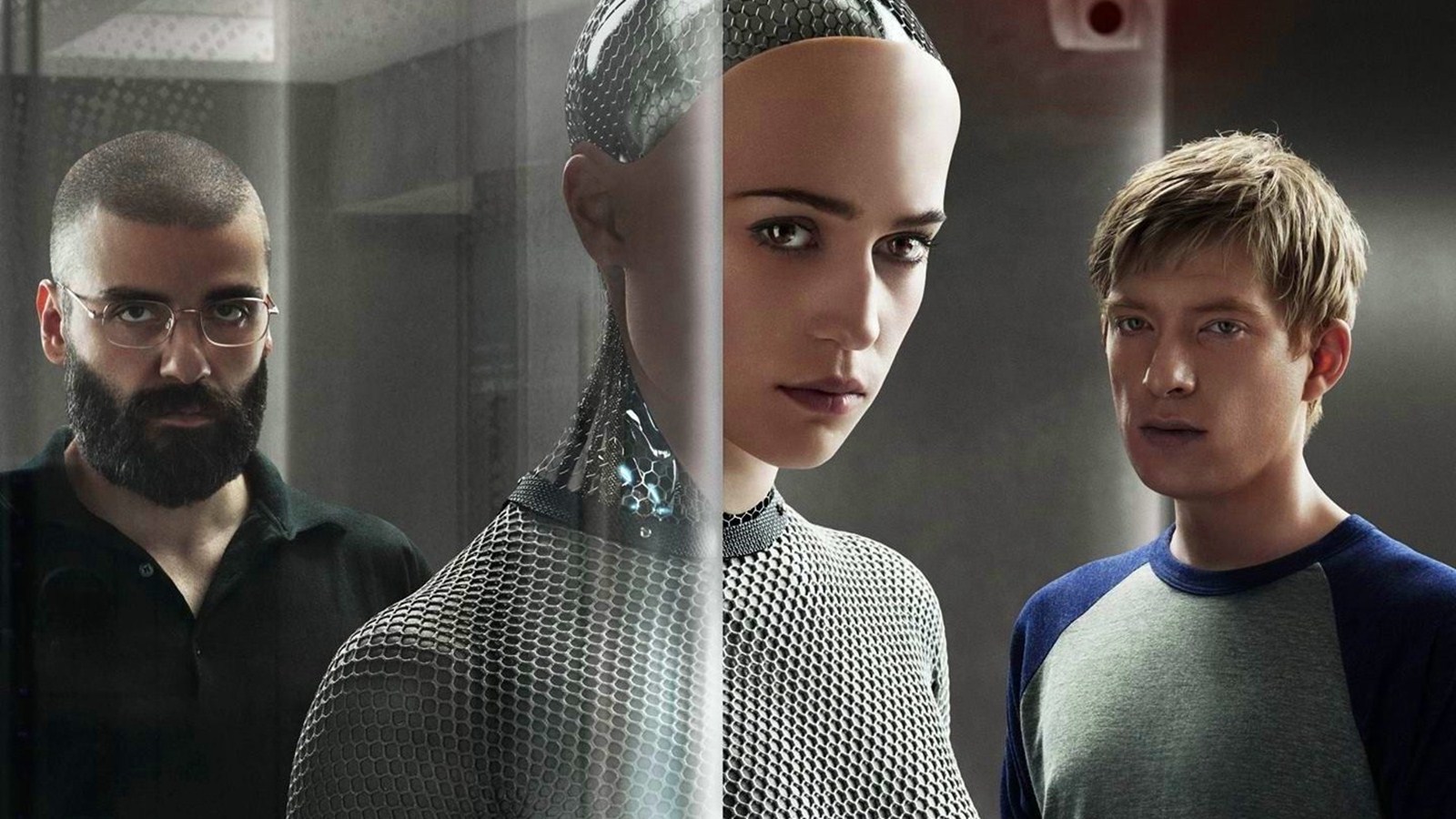
Given the underdevelopment of AI technologies, many systems are still simply not able to support the functions of explanation, discussion, formulation, or verification. They work quite effectively, but should be used only in those areas where there is no need for the above functions. It seems the same recognition by photos for automatic tagging on Facebook. But the same system cannot be applied, say, to assess creditworthiness when analyzing applications for mortgage loans, because despite the accuracy of the work, it will not be able to provide a useful explanation for why it approved or rejected this or that application.
As in the case of people, at home and in the workplace, we want to be able to work with, and not for AI systems. But for this they should be able to explain to us the course of their thoughts. Otherwise, we will come to a situation where we will only have to listen and obey. We are facing a choice: to create artificial intelligence, which will be our partner, or which will only indicate what we should do.
Although the transparency of AI thinking looks like a purely technical task, it has broad socio-economic consequences. Without this transparency, users will be forced to trust and respect the AI ​​systems. And without trust and respect, the introduction of such systems will falter, and we will not receive the advantages that AI-technologies could give us.
ZY As a bonus, a
link to a selection of films on the topic of AI.