The problem of data quality is a fairly serious topic, and not only in connection with their processing and analysis. On the data in the modern digital world built many processes, including those related to security. Therefore, the effectiveness and the result of their work depends on the quality of the data used in state and commercial organizations.
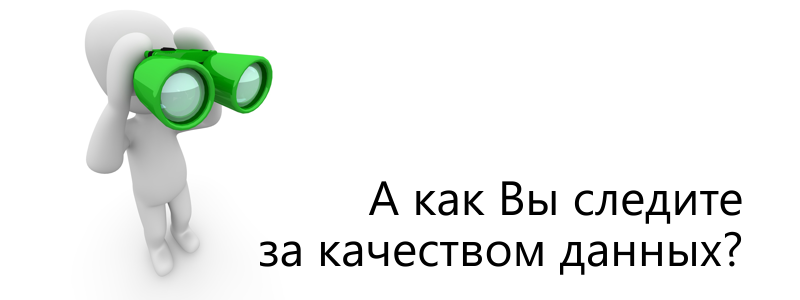
Consider several indicators that could make an integrated assessment of the quality of public (open) data.
Before you begin.This publication is a continuation of the general public data series. Many of the concepts found in the text were considered in previous articles. Despite the fact that we are talking about public (open, shared) data, the proposed set of quality indicators can also be used to evaluate other categories of data with some amendments. The proposed list is in a sense a hypothesis and does not claim to be "exhaustive."
Links to previous articles Data has a limited shelf life.')
Primary data is always relevant at a particular point in time in the past and very rarely relevant for a long period.
This is one of the problems of quality : digital data, as the registration of the historical state of an object or system constantly loses its relevance with time and has to be updated.
Data quality is a characteristic of digital data sets, showing the degree of their suitability for processing and analysis and compliance with mandatory and special requirements, in this regard, to them.And what can make up such a thing as “quality of public data”? Select nine indicators.
1. Relevance of data
The designated or indirectly determined point in time at which the data reflect the real state of the target subject (object, system, phenomenon, model, event, etc.).
The relevance of the data can also be indicated by the period of time during which they retain their significance. Given the constant changes in economic systems, public economic data have a fairly short period of relevance.
The relevance of the data is most often set by the
supplier , in addition to which he can also “promise” to periodically update them to maintain it.
The recipient of data can independently assess their relevance based on information from the supplier or other means.
2. Objectivity of data
Accuracy of the data reflecting the real state of the target subject (object, system, phenomenon, model, event, etc.).
Objectivity directly depends on the method used and procedures for collecting information, as well as on the density of the recorded data. In the process of processing digital data sets, they lose their objectivity and are enriched by aggregated, rounded, reduced and calculated indicators. However, due to this, the data is “saturated” with knowledge, thereby allowing in the future to reduce the sequence of operations to extract meaningful information from the practice.
The supplier can indicate the objectivity of public data by describing their primacy and describing the procedure for obtaining them.
The recipient has the right to critically treat the secondary data, especially if their objectivity is not proved by the applicable formulas and mathematical calculation models.
3. Data integrity
Completeness of the data reflection of the real state of the target subject (object, system, phenomenon, model, event, etc.).
In contrast to objectivity, integrity shows how complete and unmistakable the data are both in terms of semantic consistency, and in terms of compliance with a given structure or selected format. Integrity depends on the correct division into elementary indivisible units, the preservation of their indivisibility, the correct identification and interconnectedness.
The data published by a bona fide default
provider must be consistent.
The recipient determines the integrity of the special test methods evaluating the semantic content, the correctness of the definition of the structure and technically checking the format.
4. Data Relevance
The correspondence of the data on the real state of the target subject (object, system, phenomenon, model, event, etc.) to the problem being solved (goal), and the possibility of their application, taking into account the existing content, structure and format.
Understanding of relevance is directly linked to the purpose of the data user and the specific task he performs, and therefore with the source data set.
The supplier cannot affect the relevance of the data, but can significantly simplify the understanding of this quality indicator with the help of extended metadata, the use of common formats and traditional structures, as well as the indication of recommendations for their use.
The recipient in each case evaluates the relevance of the data sets on the basis of the themes and working format (ie, the tools used).
5. Data Compatibility
Joint processing of data on the real state of the target subject (object, system, phenomenon, model, event, etc.) with the existing ones within the framework of the problem to be solved (set goal).
Unlike relevance, compatibility is a procedural indicator that characterizes the ability to include data in the processed array for further analysis and is not directly related to the essence and criteria of the current task. On the other hand, compatibility at the substantive level with the subject of the task being executed is important for efficient processing of digital data. Public data should be especially carefully evaluated for compatibility, including in terms of their variety. Whether for the specific purposes of combining - the mutual use - of open data and shared data, or shared and delegated data depends on the assessment of the analyst. Most often it is necessary to comply with the conditions of separate storage and control of different types of public data.
The public data provider specifies compatibility through metadata and context references.
The recipient determines the possibility of sharing data for each set, both in content and structure, and in format. But unlike relevance, incompatible data can be attempted to be compatible with various operations of transformation, transcoding, translation, etc.
6. Measurable data
The presence in the data of the processed qualitative or quantitative characteristics of the real state of the target subject (object, system, phenomenon, model, event, etc.), as well as the calculated final volume of the set of digital data.
The substantive measurability of data is the basis for the subsequent procedures for their processing and analysis. Measurement of the total amount of data is necessary for the selection of tools and control of their integrity during processing and the results of the analysis.
The supplier may explicitly state the “measurements” included in the data, both quantitative and qualitative. At a minimum, the maintenance of public data sets with a record of their total or by file size in bytes is almost a generally accepted standard.
The recipient of public data restores the measurability in the content of the data by analyzing them and examining the structure and always always accurately or fluently checks how their physical size corresponds to the declared one.
7. Data Manageability
The ability to process, transmit and monitor data on the real state of the target subject (object, system, phenomenon, model, event, etc.) in a targeted and meaningful way.
Manageability is caused by the need to change, correct, structure, organize, filter, save, forward, evaluate, distribute data. It is largely based on the correct structure and format.
A supplier can declare that data is manageable through accompanying them with special metadata, but the
recipient , as a rule, independently conducts its assessment on the basis of his competences and tools.
8. Binding to the data source
Associated and reliable identification of the supply chain of data on the real state of the target subject (object, system, phenomenon, model, event, etc.).
At the same time, in the description of the “supply chain of public data” it is better to include references to all the actors who performed the main data transfer roles: generator (author), owner, supplier. Binding to the source allows the supplier and the recipient to refer and restore authorship, legal relations, the reliability of the source, the credibility of the distributors.
Public data is almost always distributed with indication of the owner and
supplier . And moreover, one of the limitations of using data is the need to specify the source when they are published or used. It should be borne in mind that good data binding allows you to get it again, if necessary, with clarifications, additional updating or with restored integrity, i.e. - with high quality.
9. Trust in the data provider
Evaluation by the recipient of the business qualities of a public data provider on the target state of the subject (object, system, phenomenon, model, event, etc.) as a responsible, authoritative, organized and relatively independent publisher of high-quality digital information.
This indicator is some kind of integrated retrospective assessment of all previous supplier data transfers - the reputation of the publisher of public data.
The recipient always proceeds from inner conviction when determining such an indicator of data quality, but the supplier has several ways to form and maintain the level of trust he needs. These include, for example, careful preparation of data for public transfer, a high level of organization of the “digit” publication processes, support for feedback from recipients, timely updating and notification of problems found in these problems, special events, participation in independent evaluation and associations.
Any of the specified indicators of data quality is subjective , both in terms of the semantic content of the data, and in terms of its perception by different suppliers and recipients.
Nevertheless, all indicators can be divided into:
- conditionally objective - these are indicators whose values ​​are weakly dependent on the opinion of the supplier or the recipient of the data and are set in accordance with controlled and partially verified criteria,
These include: relevance, integrity, measurability, compatibility, binding to the source . - conditionally subjective - these are indicators whose values ​​are directly dependent on the opinion of the supplier or recipient of the data and are established in accordance with internal “conviction” as some acceptable criterion assessment,
These include: objectivity, relevance, manageability, trust in the supplier .
Formal assessment of each of the indicators of quality can be carried out both in points (in a given interval) and in percent. Moreover, the score can be given by expert means, and the percentage can be calculated as the proportion of data corresponding to a given quality indicator to the total volume of data. In the latter case, the task looks much more complicated and requires special tools, although it will give a balanced, but still expert assessment of quality. One of the important aspects of a formal assessment of quality indicators is their control as they work with digital data sets. In dynamics, the quality of data should not deteriorate, i.e. expert evaluation of data should not be uncontrolledly reduced after individual operations or a series of treatments.
The general problem of the quality of public data depends both on each of the listed indicators and on the
integrated subjective assessment of the recipient . In any case, quality is important first of all to the recipient, as the person performing the processing and analysis operations.
In case of completion of feedback from a third-party effective data user to the supplier, the “problem” of data quality is returned to the last “boomerang”. If the data were provided “bad” or with errors, then one cannot expect any good and adequate results from those who used them. Then the whole point of efforts to select, prepare and publish data is lost - the supplier does not receive any new useful solutions and knowledge (products or services).
The most important indicator of data quality is their integrity.It has a strong effect on data
compatibility and
manageability . And the repeated publication of data with violation of integrity will necessarily affect the confidence in their supplier. The integrity of the data is not something separate from the meaning, structure or format and must comply with all levels of digital information.
Violation of data integrity is possible:
- at the semantic level - when collecting, an error was made in completeness or data recording in such a way that the very meaning that such data describe becomes incomprehensible;
- at the structural level — when ordering data elements or processing data, an error was made in completeness or writing data so that a part or the whole structure becomes “incomprehensible”;
- at the coding level - when writing, storing or reading data, an error was made at the level of converting individual characters and concepts so that the data cannot be read and / or there are gaps;
- at the notation level - when writing, storing or reading data, an error was made at the level of converting individual elements of digital data or their joint recording so that it is impossible to correctly establish isolated individual units and the connections between them;
- at the schema level — when writing, storing or reading data, an error was made at the logic or format level of individual digital data elements or their interrelationship so that meaningful information about the subject area cannot be extracted.
Similarly, for each of the levels — meaning, structure, format — each measure of data quality can be considered.For the quality of the published data, of course, the supplier is responsible. But the recipient is forced to perform verification and, if necessary, correct the data itself.
If public data is of poor quality, then it makes sense to abandon their use and send a detailed notification to the supplier. A conscientious and interested supplier will definitely make efforts to correct the situation. At a minimum, he should close access to poor quality data at the time of the proceedings and label them accordingly.
A complaint addressed to the supplier regarding the quality of the data, in conditions of maximum openness of the network communication, forces a special declarative refusal to accept the claim with justification for such refusal without fail, or improve the quality of the data and re-issue them with appropriate explanations. And if address communication with recipients is supported, notify them in a special way.
The supplier who is not ready to be responsible for the quality of the data quickly goes into the category of "irresponsible" and loses all the advantages provided by the community of analysts and experts in the relevant subject area.
The foregoing implies the need for continuous quality control of data from both the recipient and the supplier. Which in turn compels the development and use of special measurement and control instruments.
Research into the quality of digital data, and especially the quality of open, shared and delegated data, should be carried out by analysts and experts, both at the micro level of interested businesses and at the macro level of communities and government structures. In many ways, the security of the future digital economy will be based on active monitoring of the quality of the data used.