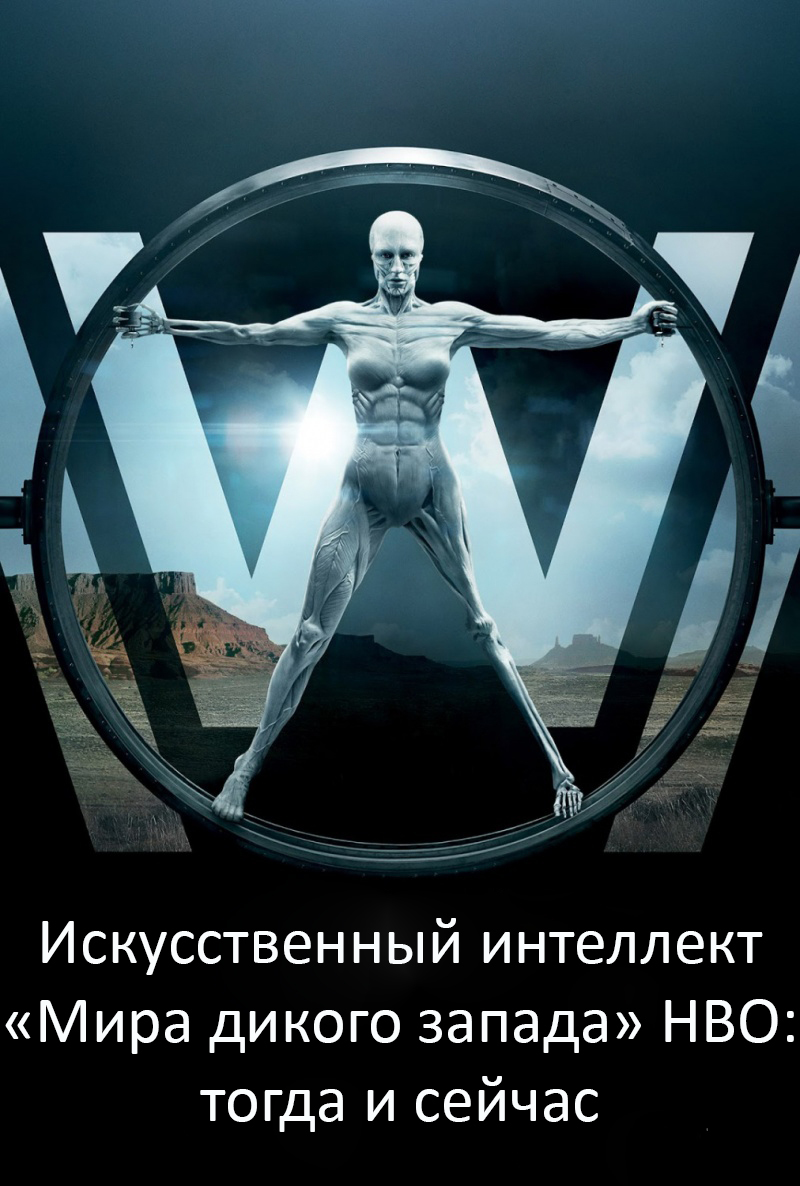
Just as today, in 1973 (the year when Michael Crichton released World of the Wild West), everyone was fascinated by the idea of artificial intelligence. The film was a huge box office success, although it was released in the same year when people began to cool down to the idea of AI: a massive depletion of AI resources, deceived expectations and, as a result, dying interest in subsequent years.
In 2016, the World of the Wild West returned to the screen, and fundamental changes in the technique of in-depth training of machines, publicly available information resources and computing power fundamentally change the future for AI. The computing power and capabilities of technologies are now sufficiently developed to enable AI to complement and push the development of society compared with the complete collapse of hopes in 1973.
')
The new version of "World of the Wild West" by HBO, created by Jonathan Nolan and Lisa Joy has become today one of the most popular TV shows. The futuristic western realities add fuel to the fire of the universal obsession with AI, and the popularity of the show proves that people are fascinated by the potential of AI. The success of the "Wild West World" reflects a sustainable AI ecosystem in which venture capital funds, corporations and consumers actively interact.
The main tasks of AI have not changed since 1973: the automation of tasks and the elimination of the constraining factors of organizations, as well as the facilitation of everyday household activities. The government, scientists and corporations moved progress in the field of AI, while consumers soared in transcendental heights (as evidenced by the box office success of the “World of the Wild West”), but were not able to acquire technology or understand how to use it. yourself restrictions.
Consumer interest was not enough to continue financing the technology, and just a few months after the film was released, James Lighthill published a rather pessimistic report on AI, prompting a subsequent 7-year lull. In the
report, Lighthill pointed to the growing gap between feverish expectations and reality, which led to the fact that universities began to cut grants, the government and the military reduced funding for AI development projects, and resources began to go to other projects.
Today, Ray Kurzweil, Ren-Sun Juan, Andrew Eun, Jan Lekun, Joshua Bengio and other experts make bold statements about the potential of AI, and corporations are urgently preparing to master the features of image recognition, voice and dialogue. The current AI revolution goes far beyond university and military studies and spills over into our daily lives. Progress is driven by 6 components that were missing or lacked in the seventies: scientific base, computing power, available information resources, specialists, and investments.
1. How to recognize cat snoutsAlthough the basic components for creating AI have existed for 50 years, today's obsession with the idea was generated by the research of Andrew Un in 2012 (Stanford). Eun and his team made a breakthrough in the field of uncontrolled learning with the help of neural networks - prerequisites for in-depth training (a series of algorithms that remotely imitate the brain). Eun, a visiting professor at Stanford (founder and leading specialist of the Google Brain team,
leader of a team of 1,200 AI developers at Baidu ) decided to test unsupervised learning, or a data set for learning without using the model, but through a neural network. He and his team used YouTube as an array of data, and with the help of the luminaries, the AI field and 16,000 computers checked whether his depth learning model would be able to recognize faces. It can, even the cat snouts can, what became known as the “cat experiment” Only due to the improvement of depth learning algorithms, which resulted in decades of scientific research, it became possible to conduct such a test.
Until 2012, traditional machine learning implied the use of algorithms to obtain the final variable. The UNA tests have shown that in-depth training (as well as the design of neural networks) has enormous potential.
In 1973, both budget financing of AI, computing power, and in-depth training methods were limited; there was no understanding of how to process the data with complex algorithms. The processing of natural language was only in its infancy, and the notion of Turing completeness appeared only a few years ago. Researchers in the 70s were mistaken about the progress of AI, as discussed in the work of Lighthill.
2. Graphic processors and computing powerIn-depth training or data processing via neural networks requires tremendous computing power, which was not available during the creation of the “Wild West World” by Krayton. Even before the beginning of the in-depth learning process, it is necessary to collect, synthesize, load, and distribute data across huge databases and distributed computing systems.
Scientists and enthusiasts today use graphics processors for learning using arrays of data. Neural networks must train on processor chips for 400 hours, driving research data of decades into algorithms. In-depth training uses huge amounts of data, processing of which requires highly scalable performance, high memory bandwidth, low power consumption and speed of arithmetic operations. The Hadoop and Spark frameworks offer acceptable databases, and Nvidia, in turn, is 20 years ahead of the production of graphics chips, which are ideal for complex algorithms and calculations. Nvidia chips, including graphics, are used in most “smart” hardware, such as car robots and drones, and have allowed the use of in-depth training in new areas.
In 1973, the computing power of computers was logarithmically weaker than it is now (as seen in the
image of Jonathan Kumi)
3. Amount of data versus storage costToday we generate a huge amount of data that can be loaded into learning models and which give a more accurate result in image recognition, speech and natural language processing. The data is obtained from such devices as fitness bracelets, Apple watches and from the Internet of Things devices. And the amount of data is many times more at the level of corporations. Increasing the amount of big data (big data) from mobile devices, from the Internet, the Internet of things creates whole mountains of data, just right for AI.
With all the modern cloud technologies, large companies can store data and have constant access to them without spending fabulous money. The growing popularity of the Box and Dropbox exchangers, offers from Google, Amazon and Microsoft have reduced the cost of data storage.
In 1973, there was incomparably less data — hours of users did not track sleep cycles or health indicators — nothing that our favorite applications can do today. In companies, all data was stored in the field, and if you did not have money for servers and their maintenance, then you were left out.
4. Interaction and public information resourcesAI specialists are attracted to open IT resources that are accessible through corporations represented in today's hypercompetitive market. The IBM Watson super computer has become the first swallow, and competitors are now seeking to offer their own services. Google provides the infrastructure through Google TensorFlow, and Microsoft offers the CNTK framework for in-depth training. Universities open access to their research: the University of Berkeley shares its Caffe framework, and the University of Montreal has opened access to its Python-Theano library.
While many organizations are collaborating and sharing research in the field of AI, in order to attract the best specialists, some are preparing for potential negative consequences if a single organization takes the lead. Some of the players in this field are OpenAI, the Open Academic Society and Semantic Scholar libraries. At the conference on neural network information processing systems, held in December, Apple announced that it would open access to its developments on AI, and allow staff researchers to publish their work.
At the time of the “World of the Wild West”, there was practically no interaction in the exchange of research, since all research was mainly carried out in government structures and defense enterprises. Organizations shared programming languages such as LISP, and scientists compared their work during face-to-face meetings, but the Internet didn’t have communication capabilities that hampered collaboration and the development of AI.
5. Specialist II- king and godStudents jumped at the AI field, and are taking courses in data analysis and natural language processing, and universities, in turn, draw the appropriate resources to create such courses. The number of students in computer science, mathematics, engineers and research courses is also increasing due to scholarship programs that provide adequate funding.
In 1973, there were few such programs, and European universities closed many projects on the study of AI after the publication of the pessimistic report of Lighthill.
6. Investment insanityToday, investment in AI is growing by leaps and bounds. Since 2011, the NGTR (cumulative annual growth rate) has reached 42%, according
to Venture Scanner . Large venture capital funds and technology companies are bored by AI, and invest in professionals, companies and initiatives. Several acquisition deals were concluded, which were in fact a beautiful purchase of talented employees to create their own or strengthen an existing team of AI specialists.
In 1973, investments came mainly from defense and government organizations, such as DARPA (Agency for Defense Advanced Research). When the fever began to subside, DARPA significantly cut its budget for the development of AI and means, to large researchers, were impoverished. At that time, venture capital was just beginning to emerge, and the funds were more interested in the production of semiconductors, rather than in AI.
AI is already present in our lives (for example, Prisma, Siri and Alexa). It will infiltrate into all areas of activity of organizations: software operation and development, security, sales, marketing, customer support, and many others. The above 6 components will be strong evidence of the potential of AI, and the wave of AI development will be similar to the Internet boom of the 90s, and the boom of mobile development of zero. Many people are still aware of this potential, which is presented in image recognition technology, video, speech and machine translation.
To prepare for the coming changes, organizations need to clearly understand the scope of the technology, its limitations and future potential. Facebook companies see AI as more of a philosophy, not a technology, as Facebook technical director Mike Shröpfer put it at a web summit in November.

In the photo: Anthony Hopkins and Jeffrey Wright in "The World of the Wild West"
HBO Photos