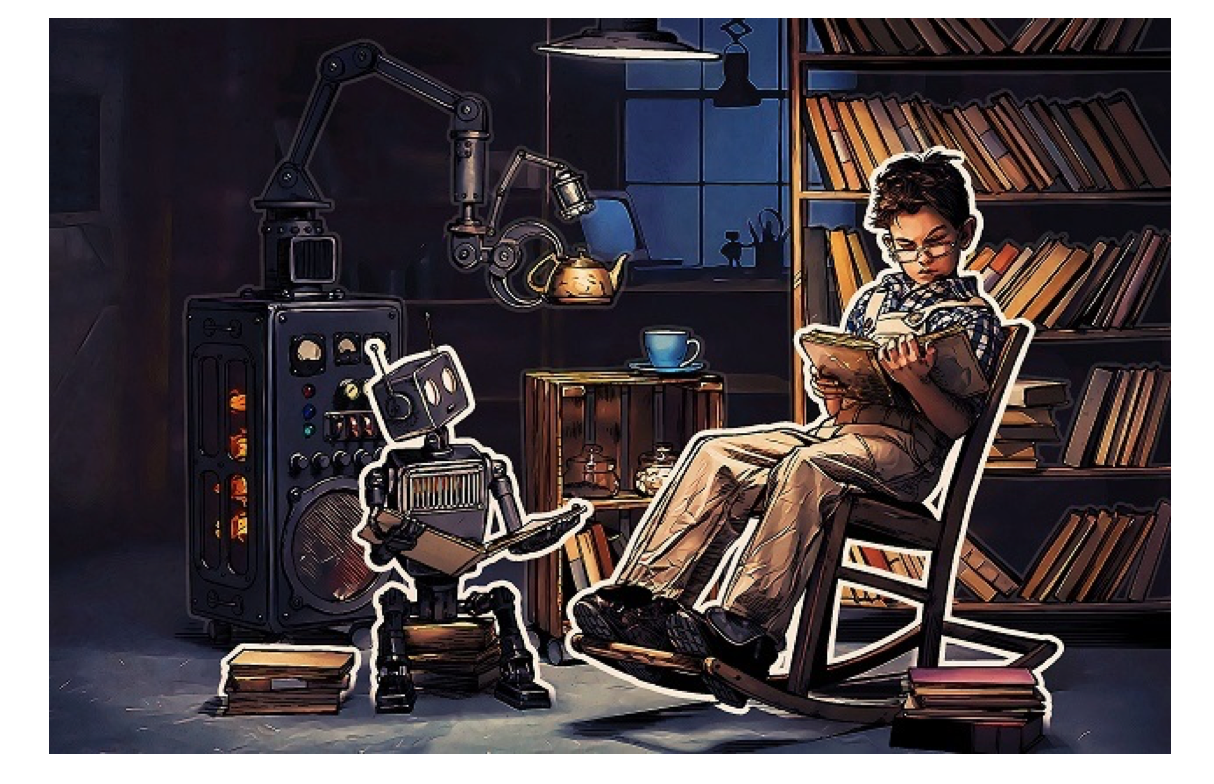
Business analysis has evolved from static reports telling what happened to interactive dashboards, with which you can delve into the data and try to understand why this happened. New sources of big data, including devices of the Internet of things, are pushing businesses to move from passive analytics - when we look at a certain period in the past and look for trends, or check for problems once a day - to active analytics that can warn of something advance and allows you to create information panels with the update in real time. This helps to make better use of operational data, which is much more useful if they are obtained “just”, while the conditions have not changed.
Many companies are interested in such active analytics, allowing them to keep abreast of their business. But even the dashboards show only what has already happened.
For this reason, various areas of in-depth analytics, including predictive, are developing most rapidly. According
to the Gartner report, by 2018, in order to maintain their competitiveness, more than half of large organizations around the world will use advanced analytics techniques (and algorithms based on them).
')
In-depth, predictive analytics is the computation of trends and future opportunities, the prediction of outcomes and the development of recommendations. It goes beyond familiar queries and reports in familiar tools like SQL Server Reporting Services, Business Objects and Tableau. It uses complex statistical calculations, descriptive and predictive in-depth data analysis (data mining), machine learning, simulations and optimization. And all this for the sake of finding signs of likely trends and patterns in the data, both structured and unstructured.
These tools are now used by marketers and analysts to understand customer churn processes, customer life cycles, cross-selling opportunities, customer preferences, assess borrowers, and identify fraudsters. For example, many telecommunication companies today are trying to move from passive to active analytics in order to determine which subscribers want to switch to competitors based on user profiles and call history. Companies from almost any market segment are interested in such tools.
In addition to predicting customer behavior, there are plenty of other applications for in-depth analytics. For example, the timely conduct of preventive maintenance, involving the search for anomalies in the products and the work of services. But one of the most interesting directions is the creation of systems to support decision-making, answering the questions “what can happen?” And “what should I do?”.
The main difficulty for business remains the same: how to extract some deep causal relationships or unobvious information from the accumulated data, allowing you to perform some actions or move the business forward.
The usual way to move from data to a solution is through the creation of a static report. For example, the sales director wants to know how the company was trading in the last quarter in different regions. And to make further decisions, you need to perform a number of procedures for the preparation of the report. But for decision-making, you need not only to know what happened in the last quarter, but why it happened this way. Let's say sales have fallen. Because you did not burn out the last three deals? Or because the average transaction volume has decreased? What do I need to do with the available statistics to figure this out?
For example, in the Cortana Analytics dashboard, you can generate all sorts of reports that reduce the amount of manual manipulation of data. Thanks to machine learning technology, the system can predict a decline in sales in advance, for example, over a month, and not report it in fact. Also here is widely used automation recommendations and decision-making.

Such in-depth analytics systems that use machine learning technologies to predict and support decision-making should ideally inform you in advance not only what can happen, but what you can do in this regard.
For example, the system found that your sales forecast for the next week will not be met. At the same time you have two marketing campaigns planned in CRM. Due to the expected downturn, you have the opportunity to launch stocks a week earlier to support sales. You activate the shares in the system and run automated business processes so that all the necessary activities started a week earlier.
Such in-depth analytics systems can be used to get answers to questions like which client is most likely to leave you in the next quarter, and can also warn you that a large client will go to competitors with a probability of 90% over the next 30 days.
Intellectual advice
Today, companies are accumulating more and more data on which to base business decisions. And the next stage in the development of decision-making systems based on available data is automated decision support systems. That is, intelligent electronic assistants that give advice on maintaining and developing a business. But are we ready for this?
According to a
recent survey conducted among 50,000 US manufacturing companies from 2005 to 2010, the number of enterprises where business decisions were made on the basis of data has tripled. True, this is only 30% of companies. And when, in 2015, telecommunication provider Colt conducted a survey among European managers of IT companies, 71% said that intuition and personal experience in making decisions work better than data analysis (although 76% said that their intuition did not always coincide with their data).
On the other hand,
Avanade's research showed that company executives generally plan to use digital assistants and automated intelligent systems for problem solving, data analysis, collaboration, and decision making. And they expect that this will allow more than a third increase in revenue. Moreover, 54% of managers said they would be happy to work with such systems.
Pioneer companies that already use machine learning to manage customer service, financial resources, risk and compliance requirements, both in sales and marketing, and in newly developed business areas, have discovered a “
significant, even exponential increase in profit” in terms of costs, revenues and consumer properties . These campaigns use the so-called "perceptual intelligence" (perceptual intelligence) - a combination of speech recognition and voice, in-depth analytics and business decision support.
According to the study, fast tracking of customer behavior, increasing their satisfaction by accelerating and improving the accuracy of call processing, reduces costs by 70% and achieves a 20-fold increase in revenue.
The involvement of business users in their construction will also facilitate the introduction of such systems. The demand for data analysis experts exceeds supply, which means that companies that do not have their own serious development will turn to third-party analytical services. And experienced users (referred to in Gartner as “civilian data analysis specialists”) will adopt these tools and create their own in-depth analytics systems.
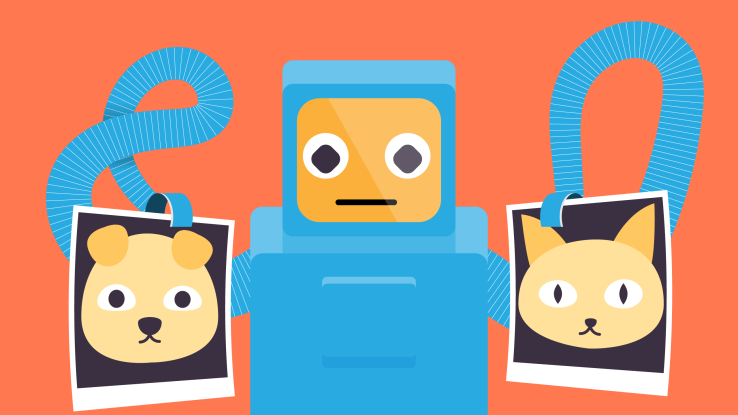