Historically, many parts of Yandex have developed their own systems for storing and processing large amounts of data, taking into account the specifics of specific projects. With such a development, efficiency, scalability and reliability have always been a priority, therefore, as a rule, there was no time left for convenient interfaces for using such systems. A year and a half ago, the development of large infrastructure components was separated from product teams into a separate area. The goals were as follows: start moving faster, reduce duplication among similar systems, and lower the entry threshold for new internal users.
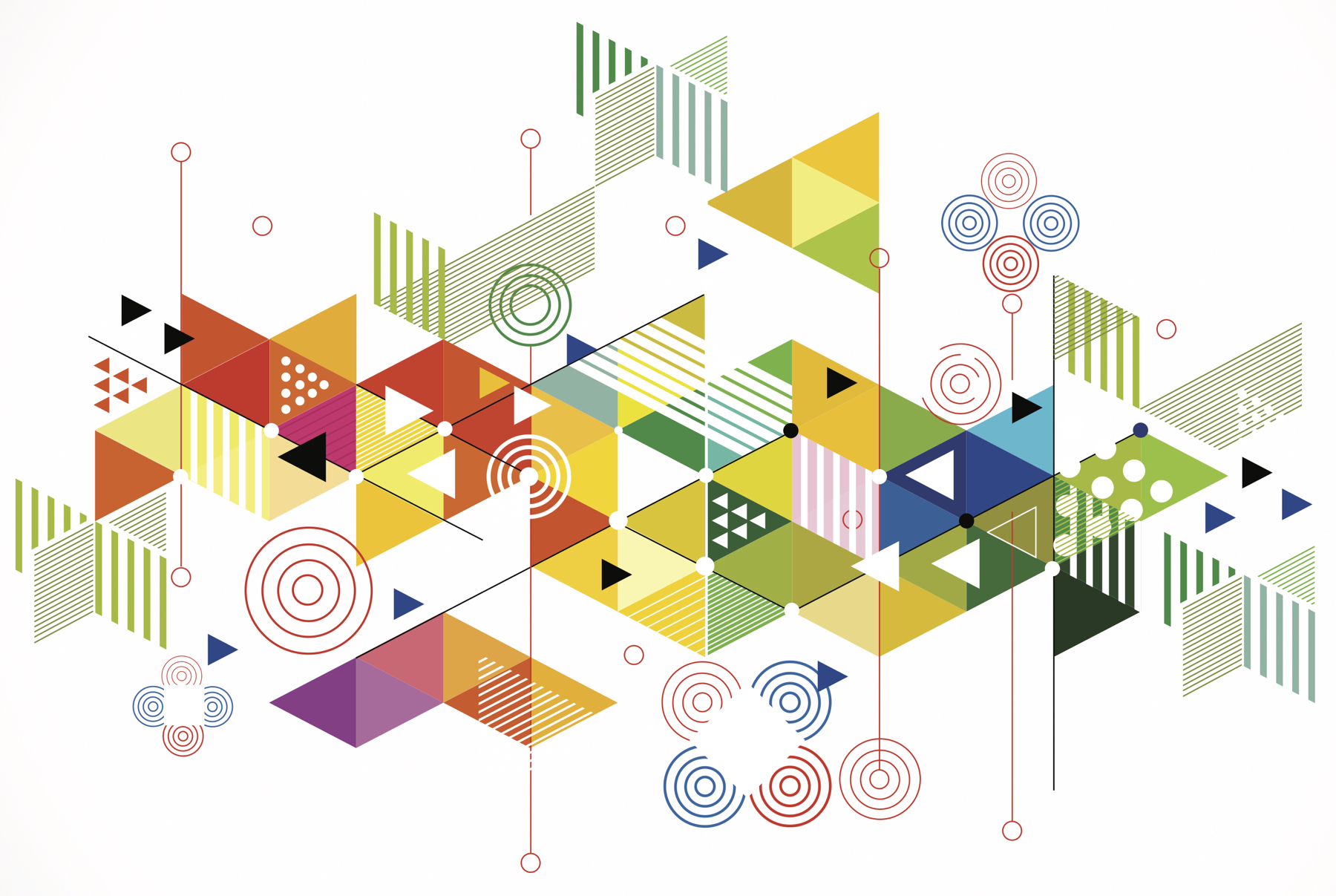
Very soon, we realized that a common high-level query language could help here, which would provide uniform access to existing systems, as well as eliminate the need to re-implement typical abstractions on low-level primitives adopted in these systems. Thus began the development of Yandex Query Language (YQL) - a universal declarative query language for data storage and processing systems. (I’ll say right away that we know that this is not the first thing in the world, which is called YQL, but we decided that this does not interfere with business, and left the name.)
')
On the eve of our
meeting , which will be devoted to the Yandex infrastructure, we decided to tell the readers of Habrahabr about YQL.
Architecture
Of course, we could look towards the popular in the world of open source ecosystems - such as Hadoop or Spark. But they were not even seriously considered. The fact is that support was required for the data warehouses and computing systems already distributed in Yandex. Largely because of this, YQL was designed and implemented extensible at any level. All the levels, we take turns below.
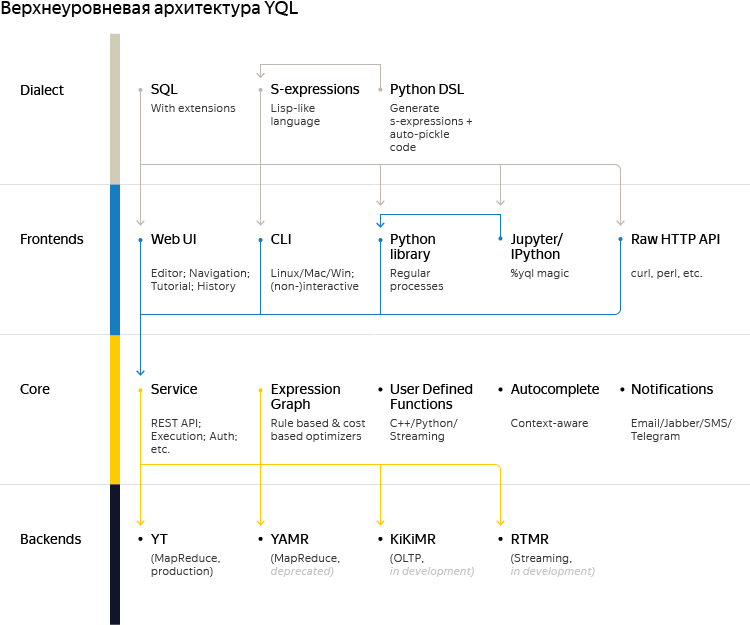
In the diagram, user requests are moved from top to bottom, but we will discuss the affected elements in reverse order, from bottom to top, so that the story is more coherent. First, a few words about currently supported backends or, as we call them, data providers:
- It so happened that in Yandex, for more than five years, two implementations of the MapReduce paradigm, YaMR and YT, have been developed, which you can read more about in a recent post . Technically, they have almost nothing to do with each other, nor with Hadoop. Since the development of systems of this class is quite expensive, a year ago it was decided to hold a “MapReduce-tender”. YT won, and now the YaMR users are finishing up on it. The development of YQL began almost simultaneously with the start of the tender, so one of the main requirements was the support of both YT and YaMR, which needed to be implemented to facilitate the lives of users during the transition period.
- About RTMR (Real Time MapReduce) also once was a separate post . His support is now at an early stage of development. First, this integration project will allow new users to implement RTMR without special training. Secondly, they will be able to consistently analyze both the fresh data stream and the archive collected over a long period and located in the YT distributed file system.
- In Yandex, data storage systems with an OLTP pattern use even more than those based on the MapReduce paradigm. KiKiMR was chosen as a pilot project for integration with YQL. In many ways, this choice was made because the need for a friendly interface KiKiMR was formed at the same time with the active growth of the popularity of YQL. Another reason was that KiKiMR had resources for this project. A detailed story about KiKiMR does not fit here, but if in brief, it is a distributed, fault-tolerant strict consistent-data warehouse, including distributed between data centers. It can be used in installations consisting of several machines and thousands of nodes. A distinctive feature of KiKiMR is the built-in ability to perform operations efficiently and transactionally with an isolation level of serializable both on individual objects (single-row transactions) and on groups of distributed objects of storage (cross-row / cross-table transactions).
- This list contains only what is already implemented or is under construction. The plans are to expand the range of systems supported in YQL and beyond. For example, a very logical development of events will be support for ClickHouse , which is now somewhat delayed only due to limited resources and the lack of urgent need.
Core
Technically, YQL, although it consists of relatively isolated components and libraries, is primarily provided to internal users as a service. This allows, from their point of view, to look like a “one-stop service” and minimize labor costs for organizational issues such as issuing accesses or firewall settings for each of the backends. In addition, both implementations of the classic MapReduce in Yandex require the presence of a client process synchronously awaiting the completion of a transaction, and the YQL service takes it on itself and allows users to work in the “launched and
forgotten mode came after the results later. But if you compare the model of service with the distribution in the form of a library, there are also disadvantages. For example, you should be much more careful about incompatible changes and releases - otherwise you can break user processes at the most inappropriate moment.
The main entry point to the YQL service is the HTTP REST API, which is implemented as a Java-based application on
Netty and not only runs incoming computation requests, but also has a wide range of supporting duties:
- Multiple authentication options.
- View a list of available clusters with backends, as well as lists of tables and charts, navigate through them.
- The repository of user-saved queries, as well as the history of all launches (historically lives in MongoDB, but this may change in the future).
- Notifications of completed requests:
- Next to the REST API, there is a WebSocket endpoint, with which user interfaces (we’ll talk about them a little later) are able to show pop-up messages in real time;
- Integration with internal services for sending letters, SMS and messages in Jabber;
- Alerts via bot in Telegram.
Using Java made it possible to quite quickly implement all this business logic due to the presence of ready-made asynchronous clients for all the necessary systems. Since there are no too strict requirements on latency, there were few problems with garbage collection, and after switching to
G1, they almost disappeared. In addition to the above,
ZooKeeper is used for synchronization between nodes, including the publisher-subscriber pattern when sending notifications.
The execution of user requests for computation is orchestrated by separate processes in C ++ called yqlworker. They can be run either on the same machines as the REST API or remotely. The fact is that there is a network communication between them using the MessageBus protocol developed and widely used in Yandex. A copy of yqlworker is created for each request using the fork system call (without exec). This scheme allows you to achieve sufficient isolation between the requests of different users and at the same time - thanks to the mechanism of
copy-on-write - not to waste time on initialization.
As can be seen from the diagram with high-level architecture, Yandex Query Language has two views:
- The basic syntax is based on SQL and is intended for people to write.
- The s-expressions syntax, in turn, is more convenient for code generation.
From the query, regardless of the selected syntax, a calculation graph (Expression Graph) is created, which logically describes the necessary data processing using primitives that are popular in functional programming. Such primitives include: λ-functions, mapping (Map and FlatMap), filtering (Filter), folding (Fold), sorting (Sort), applying (Apply) and many others. For SQL syntax, the lexer and parser based on
ANTLR v3 build the Abstract Syntax Tree, which is then used to build the calculation graph. For the s-expression syntax, the parser is almost trivial, since the grammar is extremely simple, and programs operate on these abstractions anyway.
Further, to obtain the desired result, the request goes through several stages, returning to the already passed if necessary:
- Typification . YQL is a fundamentally strongly typed language. There were many arguments in favor of this, starting from the roots in SQL, where schematization is implied, and ending with a wider scope for acceleration - for example, by generating native code on the fly. In addition to simple data types, several types of containers (Optional, List, Dict, Tuple, and Struct) and special types are supported, for example, an opaque pointer (Resource).
- Optimization . At this stage, not only equivalent transformations occur, designed to shorten the execution time. In addition to them, the action plan is brought to the form that the backend is able to execute. In particular, logical operations that the backend can natively perform are replaced by physical ones. Thus, YQL has its own framework for optimizers, which can be divided into three categories:
- general rules for logical optimization;
- general rules specific to specific backends;
- optimization, choosing one or another execution strategy in runtime (we'll return to them).
- Fulfillment . If there are no errors left after optimization, the graph takes the form that can be performed using the backend API. Most of the time, yqlworker does just that. The logical operations remaining in the graph of calculations are performed using a highly specialized interpreter, and, if possible, on the computing power of the backends.
At any stage of the request life, it can be serialized back into the s-expressions syntax, which is extremely convenient for diagnosing and understanding what is happening.
Interfaces
As mentioned in the introduction, one of the key requirements for YQL was usability. Therefore, special attention is paid to public interfaces and they are developing very actively.
Console client
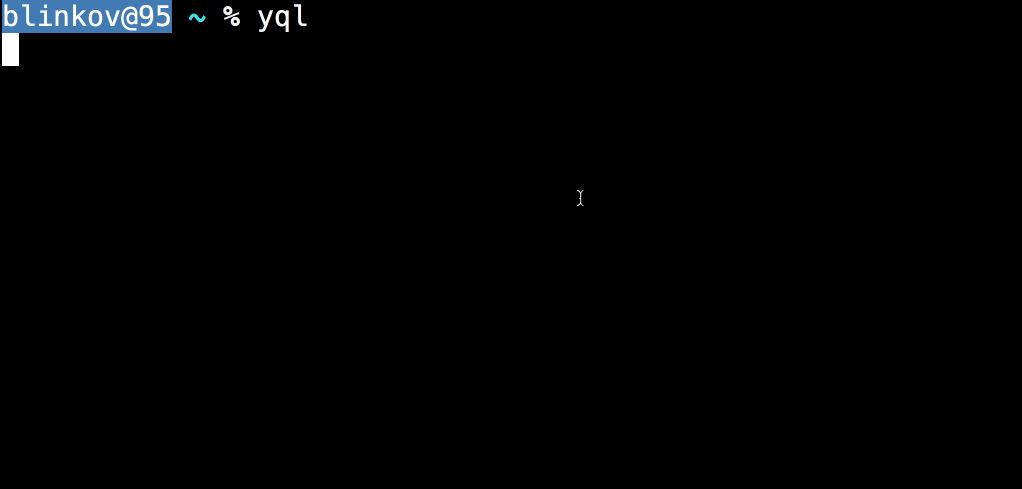
The picture shows an interactive mode with autocompletion, syntax highlighting, color themes, notifications and other decorations. But the console client can be launched in the input-output mode from files or standard streams, which allows integrating it into arbitrary scripts and regular processes. There are both synchronous and asynchronous running of operations, viewing a query plan, attaching local files, navigating through clusters and other basic features.
Such rich functionality appeared for two reasons. On the one hand, in Yandex there is a noticeable layer of people who prefer to work mainly in the console. On the other hand, this was done in order to gain time to develop a full-featured web interface, which we will talk about later.
A curious technical nuance: the console client is implemented in Python, but is distributed as a statically linked native application without dependencies with a built-in interpreter that compiles for Linux, OS X, and Windows. In addition, it is able to automatically update itself automatically - just like modern browsers. All this was just enough to organize thanks to the internal infrastructure of Yandex for building code and preparing releases.
Python library
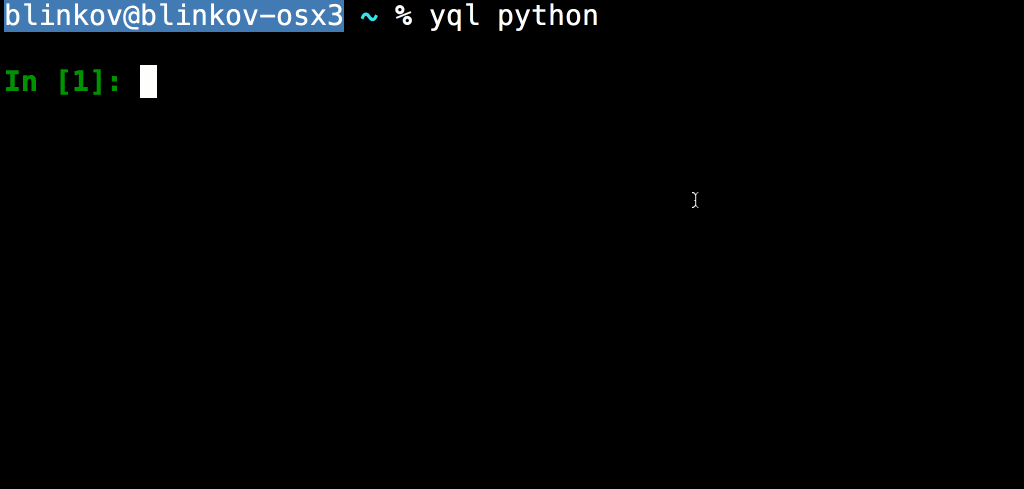
Python is the second most common programming language in Yandex after C ++,
so the YQL client library is implemented on it . In fact, it was originally developed as part of a console client, and then was allocated to an independent product, in order to be able to use it in other Python environments, without reinventing similar code.
For example, many analysts like to work in the
Jupyter environment, for which the so-called% yql magic was created on the basis of this client library:

Together with the console client, two special subroutines are delivered that launch pre-configured Jupyter or
IPython with an already available client library. It is onii shown above.
Web interface

We left the main tool for learning the language YQL, developing queries and analytics for a snack. In the web interface, due to the lack of technical limitations of the console, all YQL functions are available in a more visual form and are always at hand. Some of the interface features are shown on examples of other screens:
- Autocompletion and view table schema
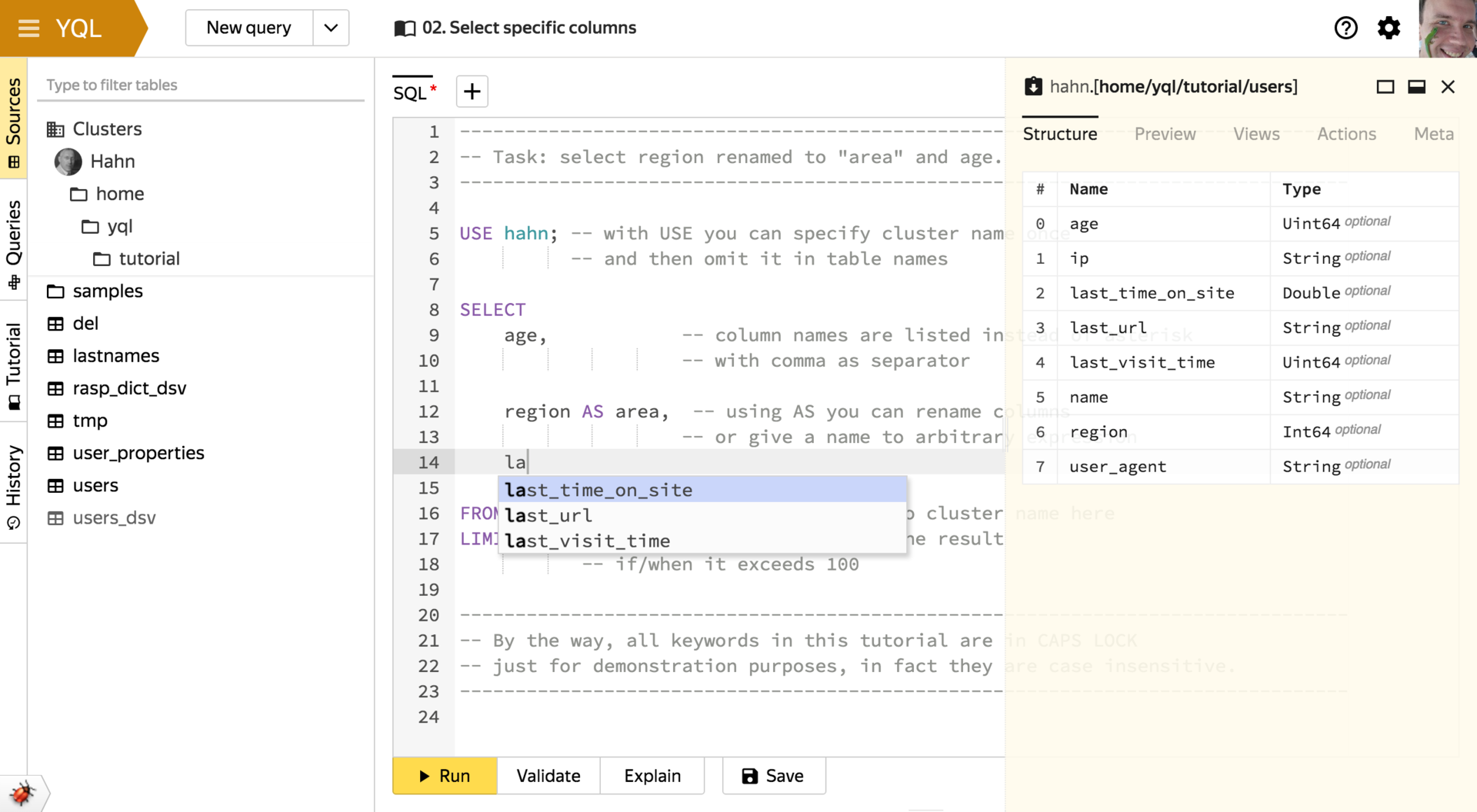
The logic of autocompletion of requests from the console client and the web interface is common. It can accurately take into account the context in which the input takes place. This allows it to prompt only relevant keywords or the names of tables, columns, and functions, and not everything.
- Work with Saved Queries
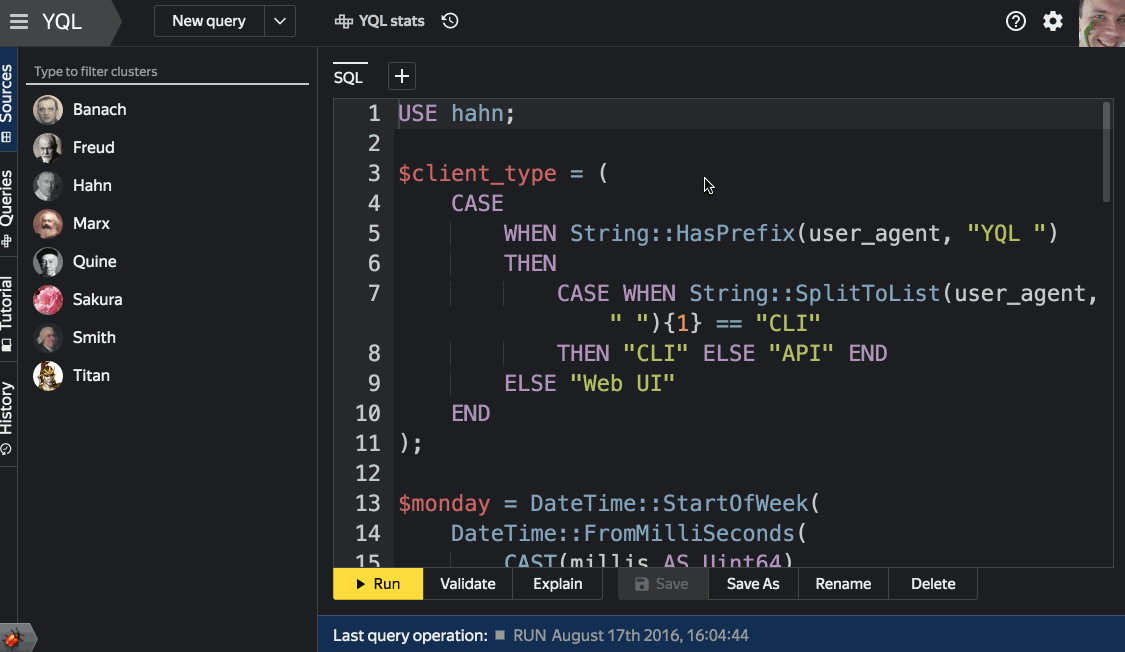
When you save the query under the name, they fall into the mini-analogue of the code repository with the ability to view the history and return to previous versions.
- Query execution plan

Here is shown the most simple and universal implementation of JOIN in terms of MapReduce.
… and not only
All pens in the REST API itself are annotated by code, and based on these annotations, detailed online documentation is automatically generated using Swagger. From it you can try pozadavat requests without a single line of code. This makes it easy to use YQL, even if the options listed above for some reason did not fit. For example - if you like Perl.
Opportunities
It is time to talk about which plan the tasks can be solved with the help of Yandex Query Language and what opportunities are provided to users. This part will be rather abstract, in order not to lengthen the already long post.
SQL
- The main dialect of YQL is based on the SQL: 1992 standard interspersed with newer versions. All basic designs are supported, but full compatibility in subtleties that were not very much in demand is still in development. Thanks to this, many new users who have previously worked with any databases with a SQL interface have to learn a language far from scratch.
- On backends working in the MapReduce paradigm, target tables (for simplicity) are created automatically. Requests most often consist of a
SELECT
an arbitrary level of complexity and optionally contain an INSERT INTO
. - Full-featured DDL (
CREATE TABLE
) and CRUD (plus UPDATE
, REPLACE
, UPSERT
and DELETE
) are available in OLTP scripts. - For many situations that are either not supported in standard SQL, or would be too cumbersome, various syntax extensions have been added to YQL, for example:
- Named Expressions
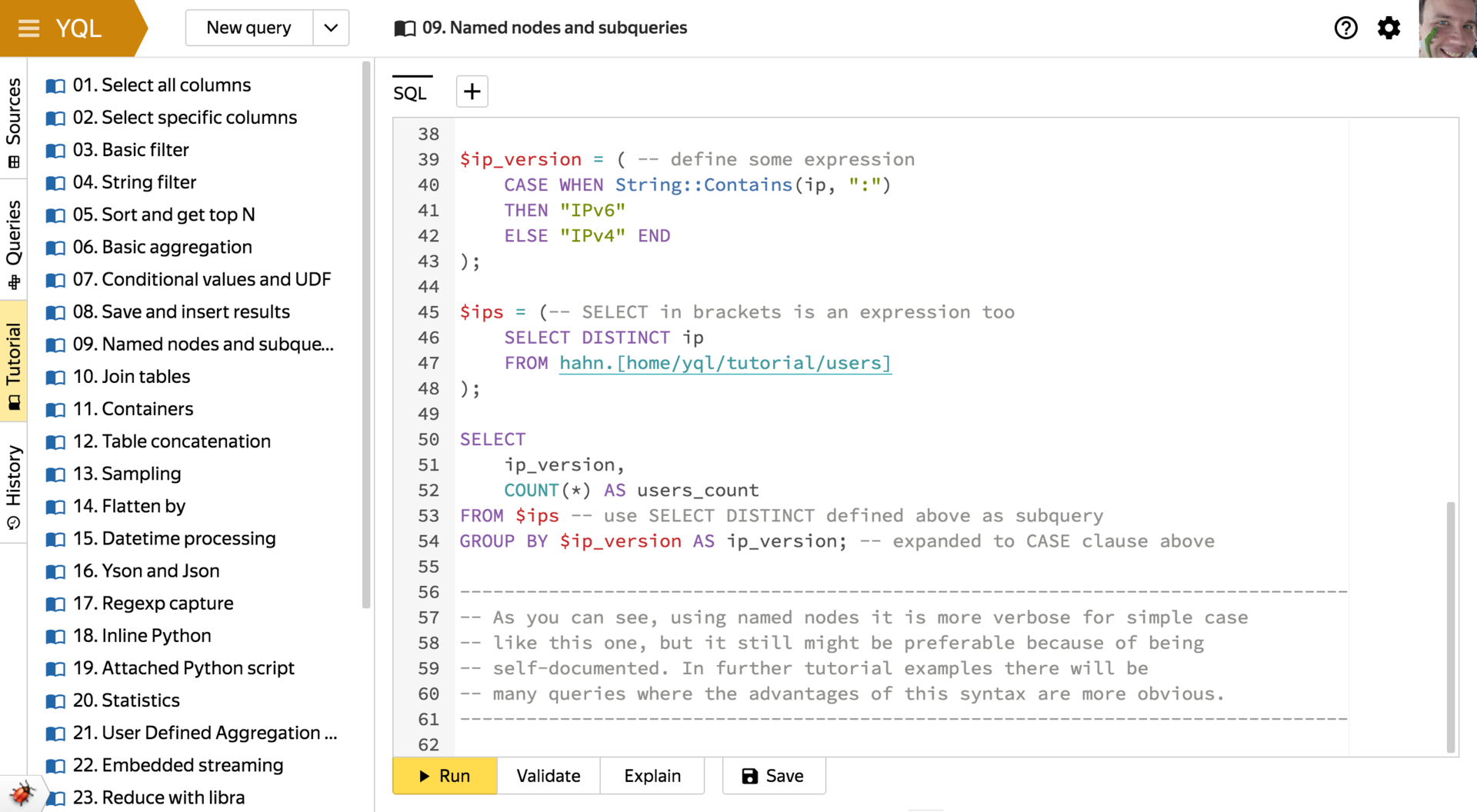
Allowing a large number of levels of nesting subqueries to write them in turn, and not in each other according to the standard. They also make it possible to not copy-paste frequently used expressions.
- Work with container types

Available as a syntax for getting items by key or index, and a set of specialized built-in functions.
FLATTEN BY
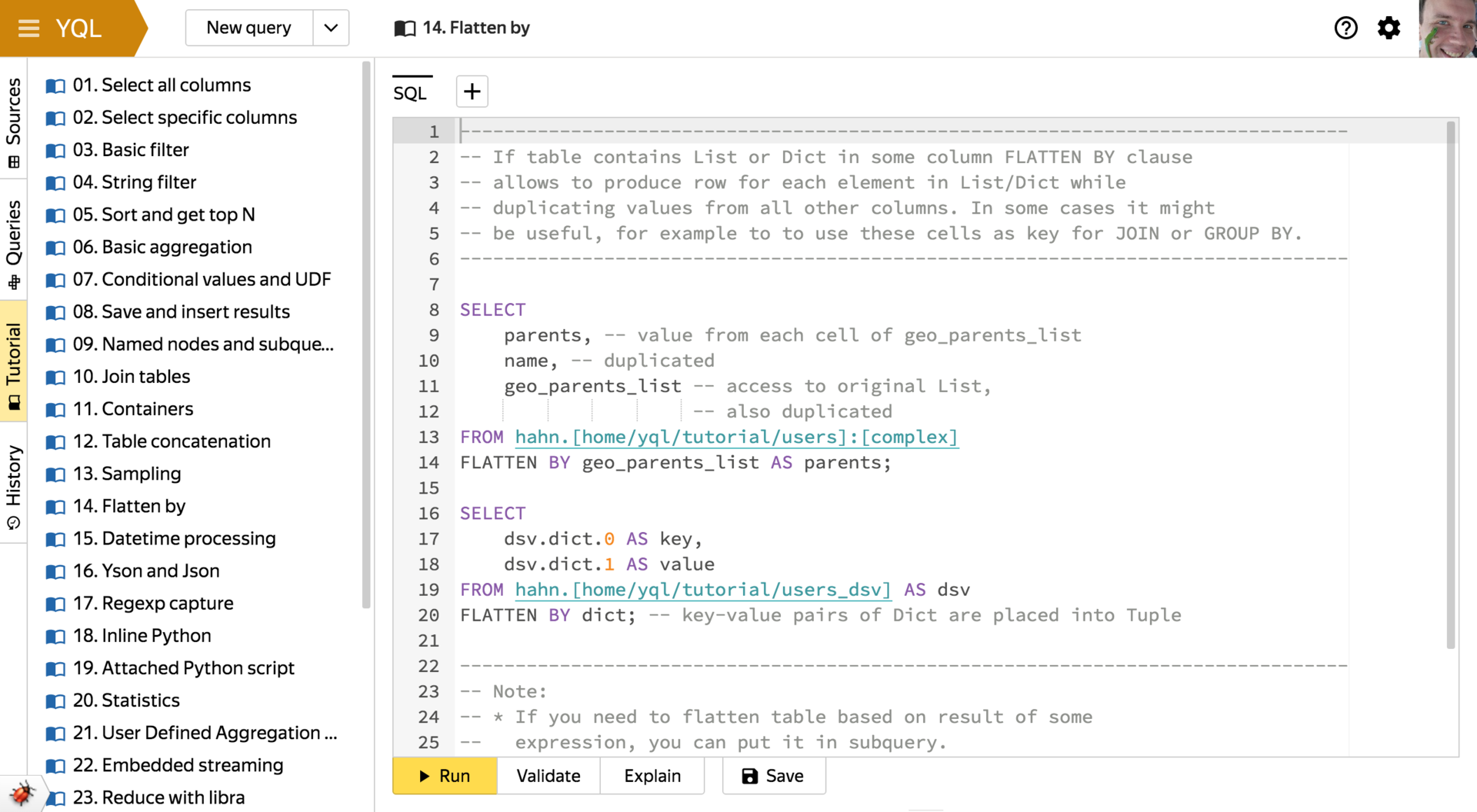
This keyword is used to multiply the rows of the source table with vertical unfolding of containers (lists or dictionaries) of variable length from a column with the corresponding data type.
It sounds a bit confusing - it's easier to show with an example. Take a table of the following form:
Applying FLATTEN BY
to the left column, we get the following table:
Such a conversion can be handy when you need to calculate some statistics for cells from a container column (say, through GROUP BY
) or when cells contain identifiers from another table with which you need to make a JOIN
.
The funniest thing about FLATTEN BY
is this: it is called differently in all systems that can do this. From what we found, there is not a single repetition:
ARRAY JOIN
- ClickHouse,unnest
- PostgreSQL,$unwind
- MongoDB,LATERAL VIEW
- Hive,FLATTEN
- Google BigQuery.
- Explicit
PROCESS
(Map) and REDUCE
(Reduce).
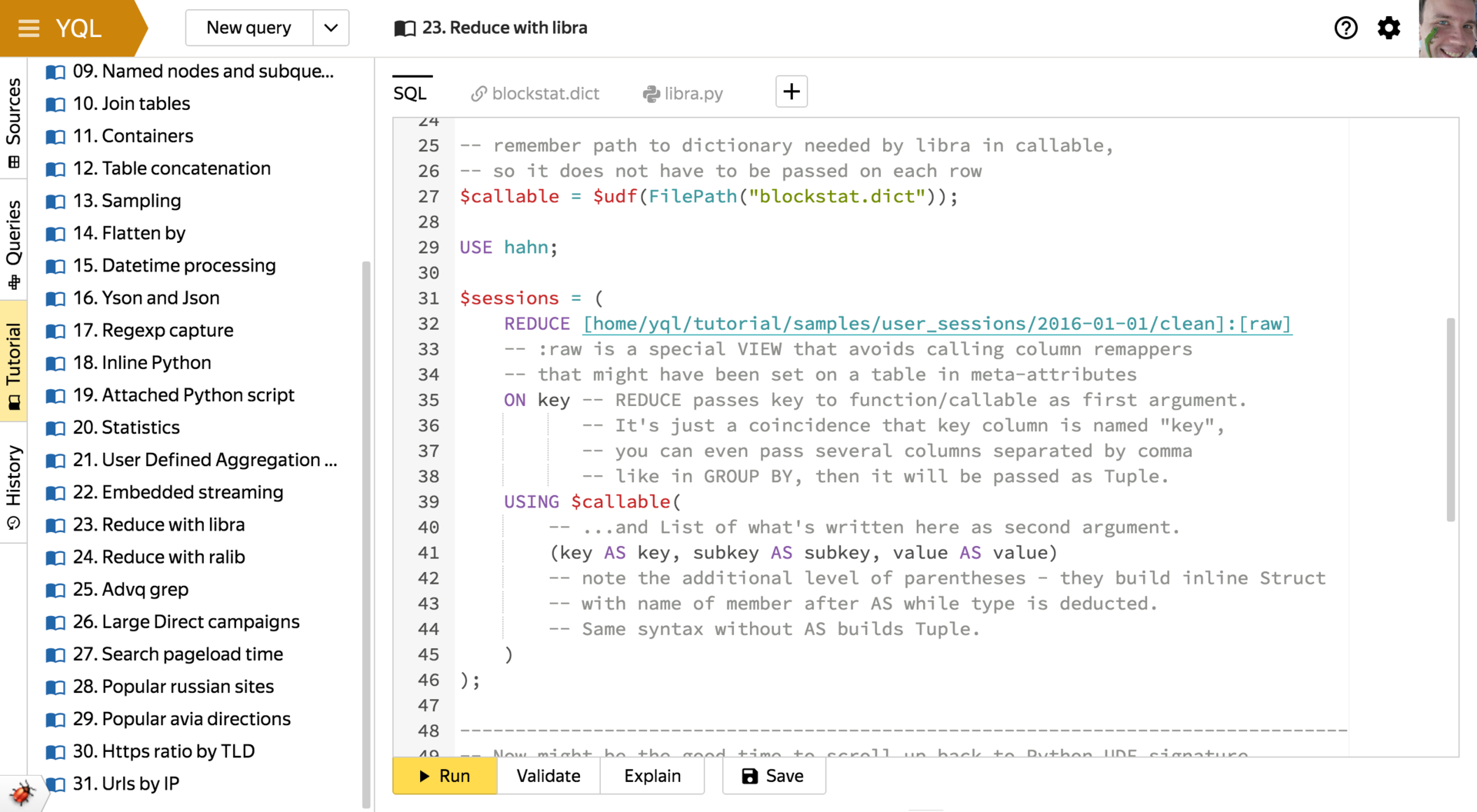
They allow embedding into existing queries in YQL the existing code written in the MapReduce paradigm in conjunction with the user function mechanism, which will be discussed below.
User Defined Functions
Not all types of data transformations are conveniently expressed declaratively. Sometimes it's easier to write a loop or use some kind of ready-made library. For such situations, YQL provides the mechanism of user-defined functions, they are also User Defined Functions, they are also UDF:
- C ++ UDF
- "Out of the box" is available more than 100 functions in C ++, divided into more than 15 modules. Examples of modules: String, DateTime, Pire, Re2, Protobuf, Json, etc.
- Physically, C ++ UDF are dynamically loaded libraries (.so) with an ABI-safe protocol for calling and registering functions.
- It is possible to write your own C ++ UDF, compile it locally (the build system has a ready-made set of build settings for UDF), load it in the standard way into the repository and immediately start using it in requests by attaching it by URL.
- For simple UDFs, it is convenient to use ready-made C ++ macros that hide parts, and, if necessary, you can use flexible interfaces created for various needs.
- Python UDF
- When performance is not so important, and to solve the problem, you need to quickly make an insert with imperative business logic, it is very convenient to dilute the declarative query with Python code. Most of Yandex employees know Python, and if someone does not know, at the basic level it is studied in units of days.
- You can either write the Python script as inline mixed with SQL or s-expressions, or attach it to the request as a separate file. In general, the mechanism for delivering files to the place of computation from a client or via a URL is universal and can be used for everything necessary, for example, for dictionary files.
- Since Python uses dynamic typing, and YQL uses static typing, the user is required to declare the signature of the function on the border. Now it is described outside with the help of an additional mini-language: the fact is that at the typing stage I do not want to run the interpreter. In the future, perhaps we will add support for Python 3 type hints .
- Technically, Python support in YQL is implemented via C ++ UDF with a built-in Python interpreter and a small syntactic sugar in the SQL parser to call it.
- Streaming UDF. So that you can smoothly switch from other technologies, and for some special cases there is a way to run an arbitrary script or executable file in streaming mode. As a result, we obtain a UDF that converts one list of strings to another.
Aggregation functions
Internally, the aggregation functions use a common framework with support for
DISTINCT
and execution both at the top level and in
GROUP BY
(including with
ROLLUP/CUBE/GROUPING SETS
from the SQL standard: 1999). And these functions differ only in business logic. Here are some examples:
- Standard:
COUNT
, SUM
, MIN
, MAX
, AVG
, VARIANCE
, VARIANCE
; - Additional:
COUNT_IF
, SOME
, LIST
, MIN_BY/MAX_BY
, BIT_AND/OR/XOR
, BOOL_AND/OR
; - Statistics:
MEDIAN
and PERCENTILE
(according to the TDigest algorithm);HISTOGRAM
- adaptive histograms for numerical values ​​that do not require any knowledge of their distribution (according to an algorithm based on the Streaming Parallel Decision Tree ).
- User Defined Aggregation Functions: for very specific tasks, you can transfer your business logic to the aggregation functions framework by creating several callable values ​​with a specific signature using the UDF mechanism described above, for example, in Python.
For performance reasons, a Map-side Combiner is automatically created in terms of MapReduce for aggregation functions with the combination of intermediate aggregation results in Reduce.
DISTINCT
now always works exactly (without approximate calculations), so it requires an additional Reduce for marking up unique values.
JOIN tables
The fusion of tables by keys is one of the most popular operations, which is often needed to solve problems, but to implement it correctly in terms of MapReduce is almost a science. Logically, all standard modes are available in Yandex Query Language, plus several additional ones:
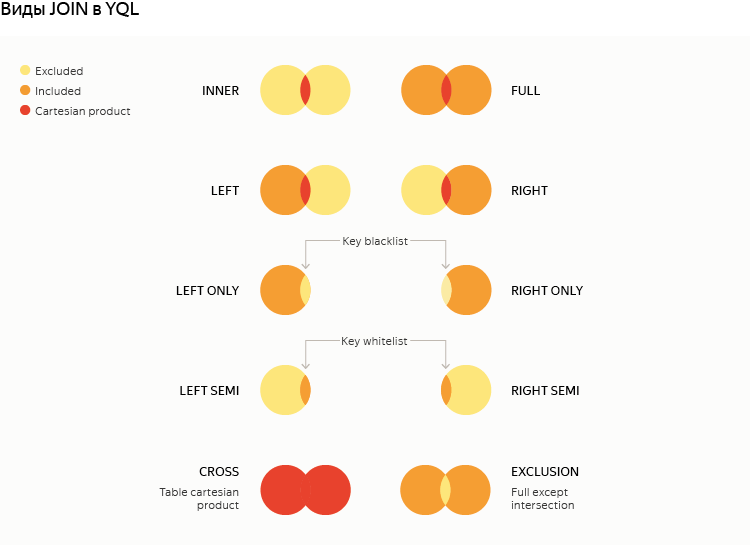
To hide details from users, for MapReduce-based backends, the JOIN execution strategy is selected on the fly depending on the required logical type and physical properties of the participating tables (this is the so-called cost based optimization):
Strategy | Short description | Available for logical types |
Common join | 1-2 Map + Reduce | Everything |
Map-side join | 1 Map | Inner, Left, Left only, Left semi, Cross |
Sharded Map-Join | k parallel maps (k <= 4 by default) | Inner, Left semi with unique right, Cross |
Reduce Without Sort | 1 Reduce, but requires pre-properly sorted input | in developing |
Development directions
Among our immediate and medium-term plans for Yandex Query Language:
- More backends in production status.
- Native code generation and vectorization instead of a specialized interpreter.
- Continue optimizing I / O and choosing execution strategies on the fly depending on the physical properties of the tables.
- Window functions based on the SQL: 2003 standard.
- SQL support: 1992 in full, creating ODBC / JDBC drivers with subsequent integration with popular ORM and business intelligence tools.
- A clear demonstration of the progress of operations.
- Extended range of available programming languages ​​for UDF - we are looking at JavaScript ( V8 ), Lua ( LuaJIT ) and Python 3.
- Integration with:
- distributed fault-tolerant service to start tasks on a schedule (a la cron) or the occurrence of events
- visualization tools (internal analogue of Yandex.Statistics ).
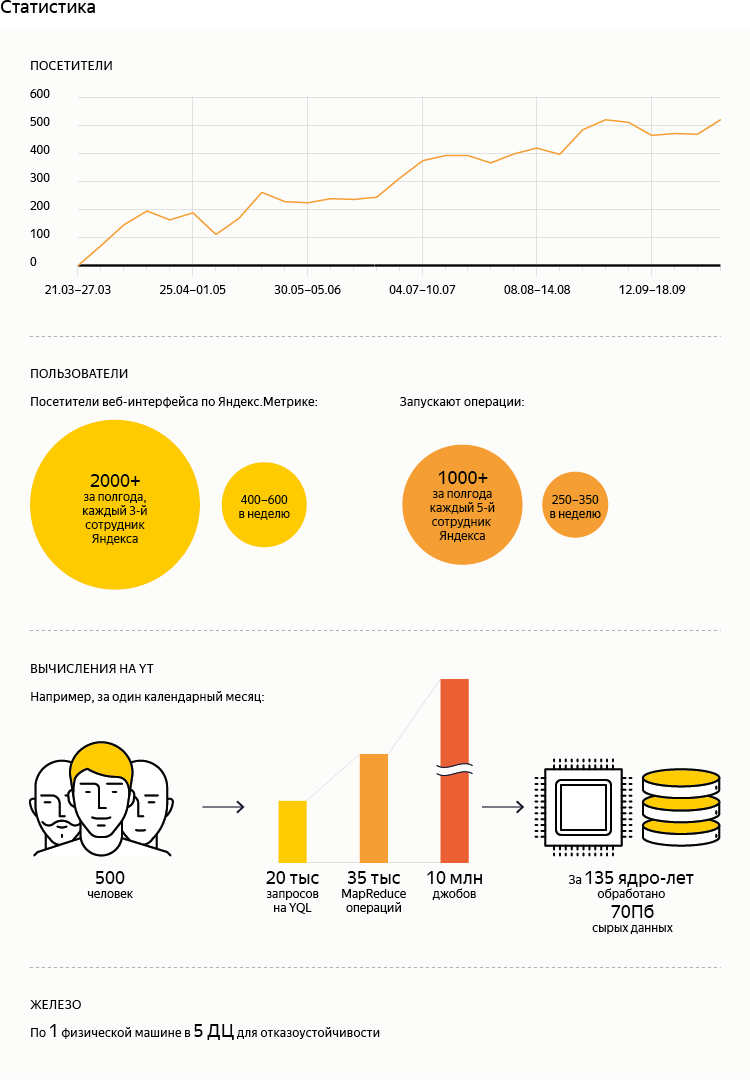
Summing up
- As the figures show (see statistics), YQL has become a product that is in high demand among Yandex employees. However, the volume of data processed with its help is not so large. This is due to the fact that historically all production processes run on low-level interfaces that are suitable for the requirements of the respective systems. That is, their gradual translation to YQL is just beginning.
- Inside Yandex, we initially encountered the following type of resistance: working in the MapReduce paradigm for many years, many are already so used to it that they do not want to relearn. In Arcadia, the main monolithic repository of the Yandex code, each employee has his own corner. There historically are literally hundreds of C ++ programs written exclusively to filter out some specific log or just a table in MapReduce for a specific task. .
- , « Hive , Spark SQL
SQL over ***
»: . , / . open source-. , , Java- , C++-, open source- — . , . YQL - 10 , full time. - SQL-, , - . Python Nile: runtime ( API) YQL- s-expressions. . , , , , , Java.
- YQL - open source — Apache Software Foundation: Hadoop Spark. , : , . .
—
, 15 , .
