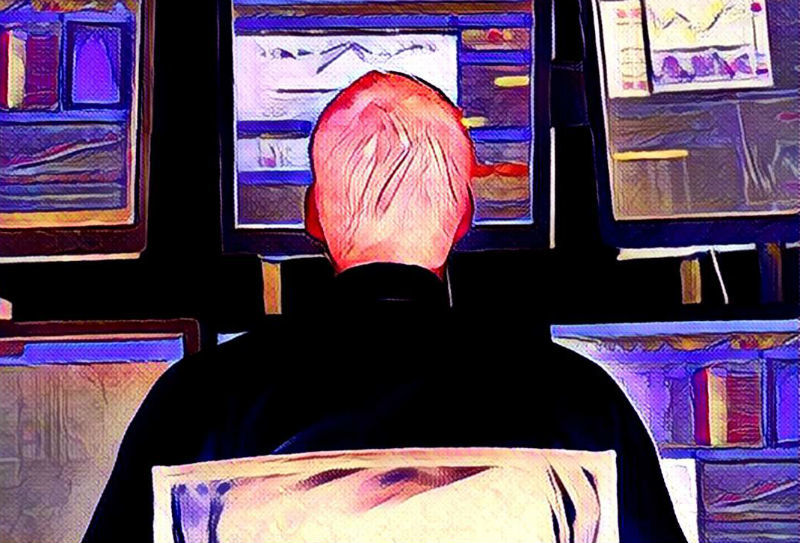
Turing Finance’s blog editor and, in combination, hedge fund financial analyst Stuart Reed published a practical
guide for those who see their future in the financial market, based on their experience in the profession. He promises to tell not about how to become a quantum, but how to be it in any of the sectors of the financial industry in which you are currently working. In his opinion, this is not even a question of schooling, but, rather, a question of ideology. We present to your attention an adapted translation of this material.
Quantitative methods or quantitative analysis in the finance industry is a science, but not a profession at all, says Reid. This means that knowing how to become a quantum adds nothing to your skills. Need to know how to be it. In the second case, we are talking about the principles and ideology underlying the quantitative analysis. In the first version, it’s about showing some practical activities to get a job, where the word “quantum” is in the description.
')
At a basic level, being a quantum does not mean the ability to derive sophisticated formulas for evaluating the capabilities of a curious stochastic model. This does not mean creating, training and testing trading strategies that use a statistical regression model. To be a quantum is to believe that scientific models are suitable for a general analysis of financial markets.
A similar ideology gained weight in evaluating derivatives, then switched to risk management, asset management and exchange trading. Probably in the next decade we will be able to observe the spread of this approach in the field of corporate finance, in venture capital and banking investments.
Philosophy of science
In my last year in computer science, the author, along with the rest of the students, spent a year creating a module called “research methodologies”. It was based on two textbooks: "The philosophy of science: from problems to theory" and "The philosophy of science: from explanation to justification." Both are written by Mario Bunge. Despite all the attempts of the teacher to make this subject as boring as possible, the author liked to study philosophy. Many ideas of the module were in demand in future practice.
Actually, the subject “philosophy of science” itself tells about the logical foundations, methods and conclusions of science. Simply put, he is trying to answer the questions: what is science? How it works? What are the limits of scientific knowledge? History has given many answers to these questions. Often the opposite. They can be reduced to
several areas : realism, empiricism, instrumentalism and induction, logical positivism and falsifiability.
Realism versus empiricism
Realism is sure that science deals with the real world in its research, and not with its construction, which is given only in sensations. On the contrary, empiricism, proceeds from the position that we know everything that we know through the senses. This means that our knowledge is temporary and limited.
The main argument in favor of realism can be the accuracy of scientific theories. If we have a theory sufficiently accurate to be able to predict the future state of the system with its help, then we describe the real world. To a greater extent this is true for the physical sciences. In the financial world, any theory will be limited in its ability to predict and subject to distortion.
Deduction, induction and abduction
In the philosophy of science, we distinguish several forms of logical reasoning.
- Deduction means deducing inference, based on one or more prerequisites, to a single consistent conclusion. It is often used in mathematics and formal computer science methods.
- Abduction is a somewhat simplified form of deduction, which states that, obtained through the latter conclusions, may not be true by necessity. But in any case, this is the best we can do, based on the available data and prerequisites.
- Induction draws conclusions on the basis of one or more strong assumptions based on evidence and observations in order to obtain probabilistic truth, which can be verified by the method of falsification.
The disadvantage of the latter method is that any conclusions here will be unreliable. This can be demonstrated by the well-known example of a black swan. As long as all the swans that come across to us are white, we can be guided by the statement “all swans are white.” The first black swan, the appearance of which the model cannot predict, will refute this truth. This example is considered in more detail in his writings
Nassim Taleb .
Quantitative market theories are built on induction through empirical observations. Such theories are easily refuted in the presence of conflicting data. They may be slim on paper, but in practice they are most often the subject of the faith of their followers, who miss facts that do not fit into the model.
The reader probably already wonders how the philosophy of science helps to become a quantum? Its ideology is important for understanding the limitations of our knowledge of financial markets. No matter how deep the financial theories are rooted in empirical data, they do not seem to be infallible, they are fragile in nature. These include all the popular prerequisites of quantitative models: returns of a normal distribution, linearity, stationarity, hypotheses of random walks, and market efficiency.
Scientific method
The scientific method helps to make the thinking clearer and more rigorous, and to increase the testability of the model and the verifiability of its underlying hypotheses.
The scientific method is a continuous process that includes systematic observations, quantitative measurements, experiment, obtaining hypotheses, testing hypotheses and improving them. The author proposes to go all the way to the scientific method on the example of the theory of random walks.

Observations
In the context of financial markets, we usually extract useful information from books, articles, mass media and even sometimes, reading good (or not so good) blogs on a given topic. What do we find in them? In many academic publications there is a statement that the market behaves randomly, it has stochastic processes.
It is important to start asking questions here. The first questions that will help us to somehow evaluate this assumption can be the simplest: who, what, when, and why? Let's try to look from this point of view on the
hypothesis of random walks .
- To whom, in terms of the market, does this theory apply? Do all markets behave in the same random way? How does chance coincide with liquidity and other parameters of relative efficiency?
- What, what forces make the market random? Does it really lead to chance by efficiency? If the market is random, then there is no point for its agents to compete with it. That is, if efficiency is removed, will the market remain random?
- Where is the market random? Is there a place in the world where it is a little less random, for example, in developing countries? If so, what can be said about the differences in the dynamics of this process?
- When do markets become random? That is, are they random throughout the time or interrupted by modes where there is no randomness? If so, does it mean that at such times it becomes possible to benefit from such a state?
- Why are markets random? What processes and forces keep them in a state of chance?
- How can you measure market efficiency and test the random walk hypothesis? Can we simulate an efficient economy using the base agent model and check whether the price disclosure mechanism is random?
Some of these questions someone had already passed the answer. Therefore, it is critical to be aware of previous research. After formulating their own questions and reading the literature on the topic, competent and correct ideas will arise in my head, which ultimately should form a verifiable scientific hypothesis.
Hypothesis formation
A hypothesis is a declarative statement that substantiates the relationship between a set of variables. A good hypothesis should be concise, verifiable, taking into account all previous accumulated research experience. Let us take, for example, the following interesting ideas that one of the regular readers of his blog sent to the author.
Market returns demonstrate randomness, as the market is able to quickly adapt to get rid of any weak points.
The thought is good. But the hypothesis itself is so-so. Many terms are not defined, too much of everything is mixed into one heap, and it is not so easy to check. Let's try to break this hypothesis into several separate ones.
Hypothesis 1 . Market movements (up or down) are indistinguishable from Martin-Löf binary random sequences.
The author’s analysis of the random walk hypothesis led him to conclude that the market is not random, at least in such harsh terms. Following this, there are many other issues that require additional research. Consequently, this new hypothesis can be further broken into two parts.
Hypothesis 2 . The efficiency of the market, the temporary accessibility to all participants of any information, forces the market to develop randomly.
In one of the following materials, the author promises to tell you how to test this hypothesis for truth. In the meantime, those who are interested can read two articles on this topic: “Risk aversion and the martingale property of market prices”, Le Roy, 1973, and “Asset prices in the economy of exchange,” Lucas, 1978. For some reason, both materials are not available under the link - the translator’s comment. In them, researchers are trying to figure out how the access to information of rational market agents affects the random distribution of prices. In general terms, the conclusion is: free access to data may lead to random movement of the market, or it may not. That is, efficiency does not mean chance.
Further, all of this leads us to the third hypothesis, which is investigated relatively rarely, due to the fact that it is difficult to verify.
Hypothesis 3 . Regardless of whether the market develops by chance or not, all opportunities to earn money on it are washed out too quickly for investors to have time to build a permanent effective strategy.
This hypothesis is not too laconic and declarative. It is quite difficult to refute or confirm. That is, in all respects it is not as elegant as the previous ones. Therefore, at this stage, it can be neglected.
Development of tested forecasts
In order to build a forecast, you first need to determine whether your hypothesis is correct. Then you should understand what values to include in the forecast. For example, if we take the first hypothesis for the base hypothesis, then market returns (up or down) can be checked and calculated using the NIST statistical test suite. Their behavior must match the Martin-Lof binary sequence. In one of the past posts, the author has already done this operation using a pseudo-random number generator called “Mersenne's whirlwind”. It turned out that this hypothesis is incorrect.
Many people make the same mistake: they are sure that research is all about objectivity. In fact, it is not so important whether the hypothesis is true or false. It is important that in any case we bring new information to scientific knowledge on a specific problem.
In order to test the second hypothesis, you will have to go one step further: create
a basic agent model in which efficiency will be guaranteed. From this model, we will then retrieve the return sequences and test them for randomness. On the models will be discussed below.
Data collection for prediction verification
The name of this stage speaks for itself. The only thing to be warned about is that data for verification must be taken from the real world (empirical data). In the extreme case, they can come from a model conforming to the hypothesis. Both approaches have their pros and cons. With empirical data, much depends on how you measure, collect and store them. Simulating data suggests that the model was done correctly.
Refinement or refutation of the hypothesis
Based on the collected data, we can answer the question of whether the predictions were correct and whether the evidence confirmed our hypothesis. It is important to note that with a positive version, we are talking only about the support of the hypothesis. Prove that it is true, we can not. In the second case, the hypothesis is considered false.
Creating a general theory
So, we collected a sufficient number of hypotheses, thoroughly tested them. Now we can collect from them one common theory. For example, portfolio portfolio theory took decades of research into the relationship between risk and profit before it was accepted by the scientific community. At the time of publication of the doctoral dissertation by Markowitz, practically no one believed in the theory of the portfolio. He even wanted to refuse to award the degree of doctor of economic sciences.
The story, in truth, is very instructive. If your idea is unpopular, it does not mean that it is incorrect. The community of financiers is very conservative. Outdated ideas and approaches are defended here with almost religious fervor and seriousness. The skills of a quantum just include the ability to look at this world objectively and constantly to deal with the myths about financial markets. Forget about consensus, search for truth.
Think with models
How to become a quantum? Design your ideas in the model. Then use these models to streamline your thinking, test and substantiate your ideas, reveal hidden patterns.
A model is a representation of individual objects or processes existing in the real world. To build models, quanta use the methods of mathematics and computer science. A quantum, for example, can compile a risk model associated with a specific portfolio of assets. Why are the models put in the forefront? Moreover, some believe that this type of thinking
was the basis of the 2008 financial crisis.
Models help us think more clearly.
Developing an idea to the level of a suitable model, made in code or written in mathematical formulas, it does not matter, it makes you see more clearly the meaning, advantages and disadvantages of the idea itself. We look at speculative things in terms of inputs, outputs and technological processes. Through the model and processes, missing parts can be detected and inaccuracies corrected.
Models are checked, intuition is not
Once an idea has been systematized and coded into the model, it becomes verifiable. Ultimately, we can see how the idea corresponds to the real layout of things. Take, for example, the stochastic model of the Brownian motion as applied to the stock market. How does it relate to the real world? Do they account for falling markets? Does it take into account periods of high and low volatility? The answer, of course, is negative. All this forces us to develop a better stochastic model: the Merton diffusion jump model and the Heston model of stochastic volatility.
Another common way to test a model is to see how it behaves on historical data. Take the usual offset value investment strategy. While it is based on the intuition of the broker, it is impossible to check whether it could bring good returns in the past. One can only believe the words of the financial manager that he is good in his business.
Models help to find hidden patterns.
Forget about finding patterns using machine learning and neural networks. Even outdated simple models can find hidden patterns and discover new insights into familiar things.
Take an example from another area. In your opinion, how many people in a separate city should be racist in order for racial segregation to reach 80%? Scientists have figured out that it is enough that 30% of people are racist for the emergence of racial segregation in a relatively isolated society. All this can be calculated using the old Schelling segregation model. And there are plenty of such examples when the model reveals to us new knowledge about familiar things. For those who are interested, the author advises to undergo a
course of understanding models on the Coursera .
Mathematical or computer models help us to move away from speculative constructions and remove the cognitive load on the individual. Simply put, they make us smarter. Much smarter.
Conclusion
Quantitative finance is an ideology, and being a quantum means much more than being just a mathematician or knowing how to write code. This is a story about the commitment to the scientific method and the ability to use it to study financial markets in general. Given this, the author gives the only advice to those who intend to become a quantum: just be it, regardless of the name of your position. There is no reason why fundamental principles and quantitative methods could not be applied to other areas of financial services or even to non-financial companies. It is likely that in a decade both quantitative banking investment and quantitative venture capitalism will become familiar. Even if you can just correctly write down the principles of this ideology at your interview, this will already be a big plus.
Other materials on the topic from ITinvest :