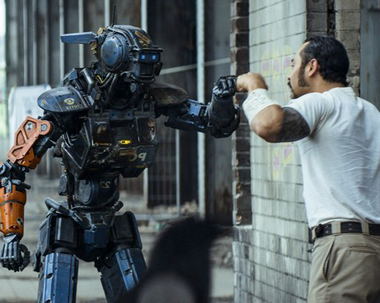
Have you ever come across artificial intelligence systems? We believe the answer of the majority of habravchan will be positive. After all, AI has already ceased to be "something beyond fiction." Speech recognition systems from Siri, IBM Watson, ViaVoice, virtual players Deep Blue, AlphaGo, and even early systems such as MYCIN, developed at the Stanford University in the 1970s, designed to diagnose bacteria that cause severe infections, as well as recommend the right amount antibiotics are all variations on the topic of AI. But, despite the fact that technology is rapidly gaining momentum, modern systems are still very “angular”, and the main problem that researchers face is language training. Making the system speak is not difficult, but no one has yet succeeded in explaining to it the “physics” of the surrounding world — what a person understands on an intuitive level.
The topic of the language problem of artificial intelligence is widely disclosed in the article by Will Knight, the chief editor of AI MIT Technology Review, which
PayOnline , an automation system for accepting online payments, diligently translated to users of Habrahabr. Below is the translation itself.
Approximately in the middle of an extremely tense game in
Go , held in Seoul, South Korea, whose participants were one of the best players of all time, Lee Sedol and artificial intelligence created by Google called AlphaGo, the program made a mysterious step that demonstrated a frightening advantage over its human opponent.
At step 37, AlphaGo decided to put the black stone in a ridiculous, at first glance, position. It seemed that this move, more like a characteristic beginner's mistake, would most likely lead to the surrender of a significant part of the playing field, then, as the essence of the game, on the contrary, consists in controlling the playing space. TV commentators wondered what was the matter: either they didn’t understand the progress of the car, or it had some kind of malfunction. In fact, contrary to the general view, move number 37 allowed AlphaGo to create a strong position in the center of the board. Google won a convincing victory by making a move that, in its place, no single person would have done.
')
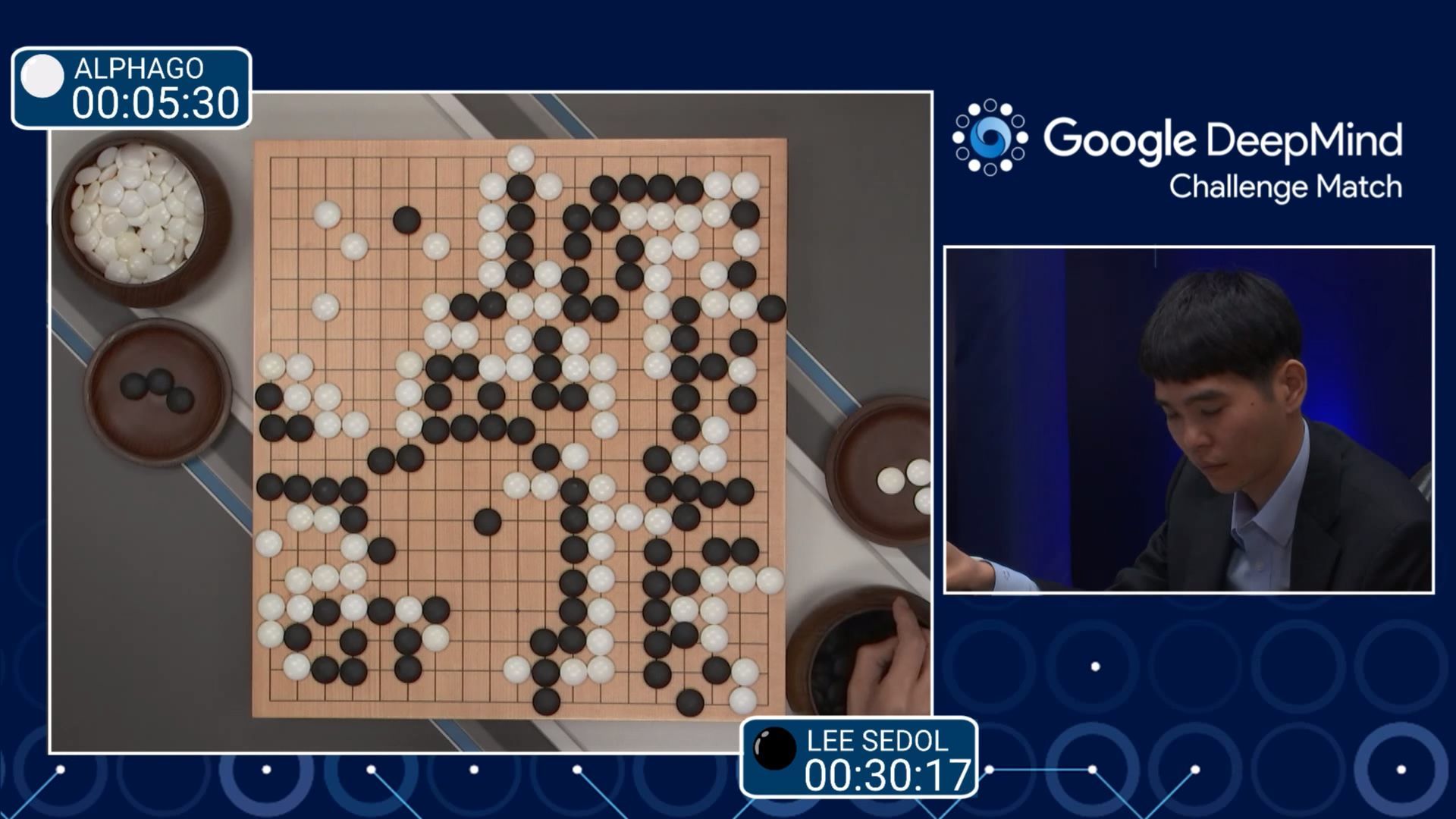
The victory of AlphaGo looks particularly impressive, as many consider the ancient game of Go as a good test for the development of intuitive intelligence. Its rules are extremely simple: two players take turns placing black and white stones at the intersections of the vertical and horizontal lines of the board, trying to surround the opponent’s stones and thereby exclude them from the game. Despite this simplicity, a good game of Go requires a lot of mental effort.
If in chess players are able to “see” a few steps forward, then in Go this process goes to a new level: miscalculation of the optimal options in each individual game very quickly becomes an almost impossible task. At the same time, unlike chess, there are practically no classical maneuvers or patterns in Go. There is no obvious way to evaluate the advantage in it, since even a sophisticated player may find it difficult to give an unambiguous explanation of why he made this or that move. All these features make it impossible to write a simple set of rules, following which a computer program could play at the same level as the professionals.
Nobody taught AlphaGo a Go game. Instead, the program analyzed thousands of games and played millions of games against itself. Among others, the AI ​​Technician program used one of the currently most popular methods called “in-depth training”. Its essence is reduced to mathematical calculations that simulate the processes occurring in the brain, when the associated layers of neurons are activated during the identification and memorization of new information. The program has learned itself through hours-long practice, gradually improving the ability to intuitively feel the strategy. The fact that, as a result, she was able to defeat one of the strongest Go players, is a truly significant event in the development of machine learning and artificial intelligence.
A Rubber Ball Thrown on the Sea - Lawrence Weiner, 1970/2014About the visual support of the articleOne of the reasons why understanding a language is so difficult for computers and artificial intelligence programs is that the meanings of words often depend on the context and even the appearance of individual letters and words. This article is accompanied by a series of images, the authors of which demonstrated examples of the use of various visual images, the general meaning of which goes far beyond the meaning of the letters used in them.
A few hours after the move number 37, AlphaGo completed the game with a victory, bringing her advantage in a series of three wins to two points. After that, Sedol stood in front of a crowd of journalists and photographers, politely apologizing for letting down mankind. “I just have no words,” he said, squinting under a flurry of flashlights.
AlphaGo's unexpected success demonstrates the significant progress in the field of artificial intelligence, which scientists have been able to achieve over the past few years, after decades of stupor and kickbacks back, often referred to as "AI-winter." Depth learning opens the way for intensive self-learning for cars, which allows them to solve complex tasks that were considered only available two years ago for people with an exceptional level of intelligence. Self-driving cars today have become a given future. In addition, very soon, AI-systems based on the use of in-depth training methods will help people diagnose diseases and recommend treatment.
But despite this impressive progress, there is one fundamental area of ​​knowledge, the fate of which in the context of AI remains unclear: language knowledge. Systems like Siri or IBM Watson can follow simple commands that are reproduced out loud or write letters and answer basic questions, but they are not able to keep up the conversation and do not understand the real meaning of the words they use. If we want to truly experience the whole transformative potential of AI, the situation in this area must change.
Despite the fact that AlphaGo does not know how to talk, it contains a technology that can bring the machine understanding of the language to a higher level. Within companies such as Google, Facebook and Amazon, as well as leading academic laboratories for the study of AI, researchers are attempting to fully solve the problem, which seems to be unsolvable. Among the developments they use are in-depth training and some other AI-tools that ensure the success of AlphaGo and the general revival of interest in AI. The success of their work will allow to realize the scale and nature of the phenomenon, defined as a revolution of artificial intelligence. From the results of their activities will also depend on how sociable the machines of the future will be and whether they can become close friends of people in their daily lives, or will they remain mysterious black boxes seeking greater autonomy.
“It’s just impossible to create a humane AI system based on the work of which there would be no language,” said Josh Tanenbaum, a professor of cognitive science and computer engineering at MIT. “This is one of the most obvious characteristics of human intelligence.”
Perhaps the same methods that allowed AlphaGo to conquer primacy in Go would one day allow computers to master the language, or maybe it would take more than that. In any case, if artificial intelligence programs do not learn to understand language, the impact that AI will have on society will be different. Of course, we will still have incredibly powerful and smart programs, such as AlphaGo. However, our relationship with AI is likely to be characterized by a much lower degree of cooperation and friendliness.
“From the very beginning of the research, one question didn’t give rest to the scientists:“ What if we had entities that are reasonable in terms of efficiency, but different from us, in the sense that they are not able to understand our human nature and realize it? ” - says Terry Grape, an honorary professor at Stanford University. “Imagine machines whose existence is not based on human intelligence, but on“ big data, ”and which, at the same time, control the world.”
Caster machines
A few months after the triumph of AlphaGo, I went to Silicon Valley - the very heart of the last boom in artificial intelligence. I wanted to pay a visit to the researchers who have made significant progress in the practical application of AI and are now trying to bring the machine to a higher level of understanding of the language.
I started with Winograd, who lives in the Palo Alto area, right near the southern part of the Stanford campus, near Google headquarters, Facebook and Apple. Curly white hair and thick mustache make it even more like a reputable academician, radiating also infectious enthusiasm.
In the distant 1968 Vinograd made one of the very first attempts to teach the car a reasonable conversation. Being a gifted mathematician and a fascinated scholar of linguistics, he found himself in a new MIT laboratory for the study of artificial intelligence to write a doctoral work and decided to develop a program capable of conducting textual conversations with people in the language of everyday communication. At the time, this task did not seem too ambitious. The AI ​​field developed in leaps and bounds, while other MIT employees worked on creating complex computer vision systems and futuristic robotic manipulators.
“We then felt as if we were exploring the unknown and were absolutely not limited in possibilities,” he recalls.
Four Colors Four Words - Joseph Kosuth, 1966Yet, not everyone was convinced that learning a language was a simple task. Some critics, including the influential linguist and MIT professor
Noam Chomsky , believed that AI researchers would inevitably run into problems in their attempts to teach machines to understand human language, simply because the mechanics of human language were then poorly understood. Grapes even recalls that once at a party one of the students of Chomsky stopped communicating with him, barely hearing that he was working in an AI laboratory.
But there were reasons for optimism. A few years earlier, Joseph Weisenbaum, a German professor at MIT, created the first chat bot in history. A virtual interviewee, named ELIZA, was programmed to behave like a caricature therapist, repeating the key phrases of statements or asking questions to develop a conversation. If you, for example, told her that you are angry with your mother, then in response she would ask: “What else comes to your mind when you think of your mother?” This cheap, at first glance, trick, worked surprisingly well. Weisenbaum was shocked when some of the test participants began to tell the car about their darkest secrets.
Grapes wanted to create something that would be able to understand the language. He began with a general simplification of the problem. He created a primitive virtual environment, the “block world,” consisting of a handful of imaginary objects located on the same, imaginary, table. He then created a program called
SHRDLU , capable of handling all the nouns and verbs, applying the simple grammar rules needed to address the bare virtual world. SHRDLU (a set of letters, without any sense repeating the sequence of the second column of
the linotype keyboard ) could describe objects, answer questions about their interrelationship and execute typed commands, making corresponding changes to the block world. She even had some semblance of memory. That is, if you told her to move the red cone and after that you mentioned the "cone", then it automatically meant a red figure, and not any other.
SHRDLU everywhere was demonstrated as one of the symbols of fundamental progress in the field of AI. But it was only an illusion. When Grapes tried to expand the block world of the program, the rules necessary to account for the words used and grammatical connections became too cumbersome and uncontrollable. A few years later, he stopped working on the program and, ultimately, quit working with AI in order to focus on other areas of research.
“The restrictions turned out to be much stricter than we thought at the very beginning,” the scientist admits.
Grapes came to the conclusion that it was simply impossible to provide the machines with the ability to truly understand the language using the tools available at the time. The problem was that, as Hubert Dreyfus, a professor at the University of California at Berkeley, wrote in his 1972 book What Computers Cannot Do, many things that people do require a kind of instinctive thinking that cannot be reproduced using hard rules. . That is why, before the match between Sedol and AlphaGo, many experts expressed doubts about the fact that cars could master the game of Go.
Pure Beauty - John Baldessari, 1966-68But even while Dreyfus was developing his theory, the research team was working on an approach that ultimately had to endow machines with just this type of thinking. Drawing a little inspiration from the discoveries of neuroscience, they experimented with artificial neural networks — layers of mathematically simulated neurons that could be taught to activate in response to certain incoming data. The first such systems were terribly slow, and the approach was abandoned due to the impracticality of its logical apparatus. It is extremely important, however, to note that neural networks could be trained in such behavior, which was not possible to program in advance, and later this skill was very useful for solving simple tasks, such as recognizing handwritten characters. Work in this direction acquired a commercial character in the 90s when it was used to
read numbers on checks . Proponents of the approach were convinced that neural networks would ultimately allow the machines to produce much more significant results than what they could in those years. One day, they said, technology could even understand the language.
Over the past few years, neural networks have become many times more complex and efficient. The approach has been strengthened by advances in mathematics and, importantly, by the emergence of faster computer hardware and the availability of vast amounts of data. By 2009, researchers from the University of Toronto showed that a multi-layer network of in-depth training is able to recognize speech with record accuracy. And in 2012, the same group of scientists won the competition in machine vision, presenting an incredibly accurate algorithm for deep learning.
The neural network of depth learning uses a simple trick to recognize individual objects in an image. The layer of simulated neurons receives input in the form of an image, and some of these neurons are activated in response to the intensity of individual pixels. The resulting signal passes through many other layers of connected neurons before reaching the output layer, which signals that the object has been identified. To adjust the sensitivity of neurons and the subsequent reproduction of their correct response, a mathematical technique called “
back propagation of error ” is used. This step allows the system to learn. Each layer of the network responds to different elements of the image, such as edges, colors, or texture. Such systems today are able to recognize objects, animals or faces with an accuracy comparable to the capabilities of any modern person.
Attempts to apply in-depth learning to languages ​​face an obvious problem, the essence of which lies in the fact that words are conditional symbols and in this respect they are fundamentally different from artistic images. Two words, for example, can be similar in meaning and consist of completely different letters, and the same word in different contexts can mean completely different things.
In the 1980s, researchers proposed an interesting way to turn the process of learning a language into a type of task that a neural network can handle. They showed that words can be represented as mathematical vectors, which allows us to calculate the similarities between related words. For example, “boat” and “water” are close to each other in a vector space, despite the fact that these two words look completely different. Google , , , , « ».
, , , .
Meaning of life
-, - Google -,
, , -, , . , .
« , — . — , , ».
The Answer/Wasn't Here II — , 2008Google . , Parsey McParseface, , , . , . Google -. , RankBrain, , , . . , , SmartReply, Gmail . , , Google .
, . 18900 . . , , : « ».
« , — . — , , ».
, . : « ?», : «, ». : « ?». : «». , . , , . , , . , , , , . . Google, , . , , .
, - , . - , , , .
«, , , — , , . — , , ».
, . , . , , , . : « », « », « » . , .
« , , , — . — ».
, , , . . , . - , - , . , , .
« — , — . — [] , , ».
, , , , . , , , ?
-, MIT, , , , . .
« , , . , , : , - - », — .
, , .
,
, , . , -, . .
« , , , », — .
Webppl, , , , , , . webppl , — . , - « » , , , . , , , -, .
. « » — , — , .
, , , , , , , . , .
, , , . , , , .
« , , — , MIT, , — , , ».
Toyota, , MIT , . — , , . — .
« , , — - , — , Toyota MIT. — , , , ».
, , Google DeepMind, AlphaGo, -. , , . , AlphaGo . 37- . , , Google : «» , , 1 10 . , AlphaGo , , , .
, .
, Google , . , .
« , — . — . , ».
, , , , , : «?». , , - . , — , , . , .
PayOnline .