The 21st century can rightly be called the century of social media. Countless posts, reposts, replies to posts and comments, hundreds of every second downloadable videos on YouTube and photos in Instagram. If you are offline, you are not in trend. Major universities (such as the Massachusetts Institute of Technology MIT) lay out online lectures and textbooks. Issues affecting a variety of topics from politics and culture to cooking and the specifics of performing a particular asana in yoga are now discussed not only and not so much in the kitchen or in the smoking room, but on the Internet forums. What's better? Is the book properly screened? Does the plot of the beloved series move in that direction? Will the new phone model be more successful and cooler than the competition? Today, these questions are answered by a big date analysis, and the systems that produce similar research on social media data, although not yet yesterday, but this morning is accurate. One of these systems was created by a software giant and bears the proud name of a faithful ally of a British detective. It is worth noting that we will discuss only the social media analytics system (IBM Watson Analytics for Social Media), and this is only part of the famous Watson cognitive system, and the pros and cons below are directly related to this service, which for simplicity of mention will be called simply Watson in the future .
1. Like many big data analysis systems, Watson's main goal is to give the user a simple view (graphs and pictures) about how often and in what way they write about social media products, companies, brands and services. Aka is the frequency of mentions on the message flow, sorted according to the results of the sentiment analysis. One of the key features of Watson was already hidden at this stage. Stream purchased. That is, information is collected by a separate company and then transferred for analysis. And if there was no tweet or a new comment, and it turned out to be not taken into account in the analytics - all questions to ... not Watson. Today, analysis is available from Twitter, forums, news, Yutyub (more precisely all those comments that people leave on the wall), Facebook Facebook pages, reviews and blogs. At the same time, messages from the above sources are used only for quantitative analysis, and according to the agreement with Twitter, the company does not have the right to let the user read what people are writing on the network and what the public is (not) satisfied with. At the same time, it’s easy and simple to do this on Twitter itself, just enter the word we need into the search bar ...
2. The positive side, directly dependent on the previous paragraph, can be considered the opportunity to start an unlimited number of topics. For example:
Machine 1, Machine 2, Machine 3, Machine 4 ... Machine N ... As well as an additional bonus to the system for creating separate topics to the topic, or in a different way, characteristics:
dimensions, fuel consumption, engine features, and so on . Inside each topic, you can specify both the necessary search words or terms that it is important for you to catch in the message flow, as well as negative words. For example, in the situation with the analysis of messages about the German OBI (OBI) supermarket, you need to exclude the character of "Star Wars". To create the most correct search query, in the case of the polysemy of the object for analysis, you can use the hint: in the right field you can see the cloud of the most frequent words used with the object. Alas, the system can not always recognize whether a multi-valued object or not, and the hints work only with a list of previously known polysemic words.

')
3. At this point, we analyze the actual analytics produced by Watson. One of the most important and main points of the analysis of social media messages is demography. That is, distribution by gender, age and geography. It should be noted here that this analysis is carried out thanks to linguistics, which means that a number of problems are associated with this.
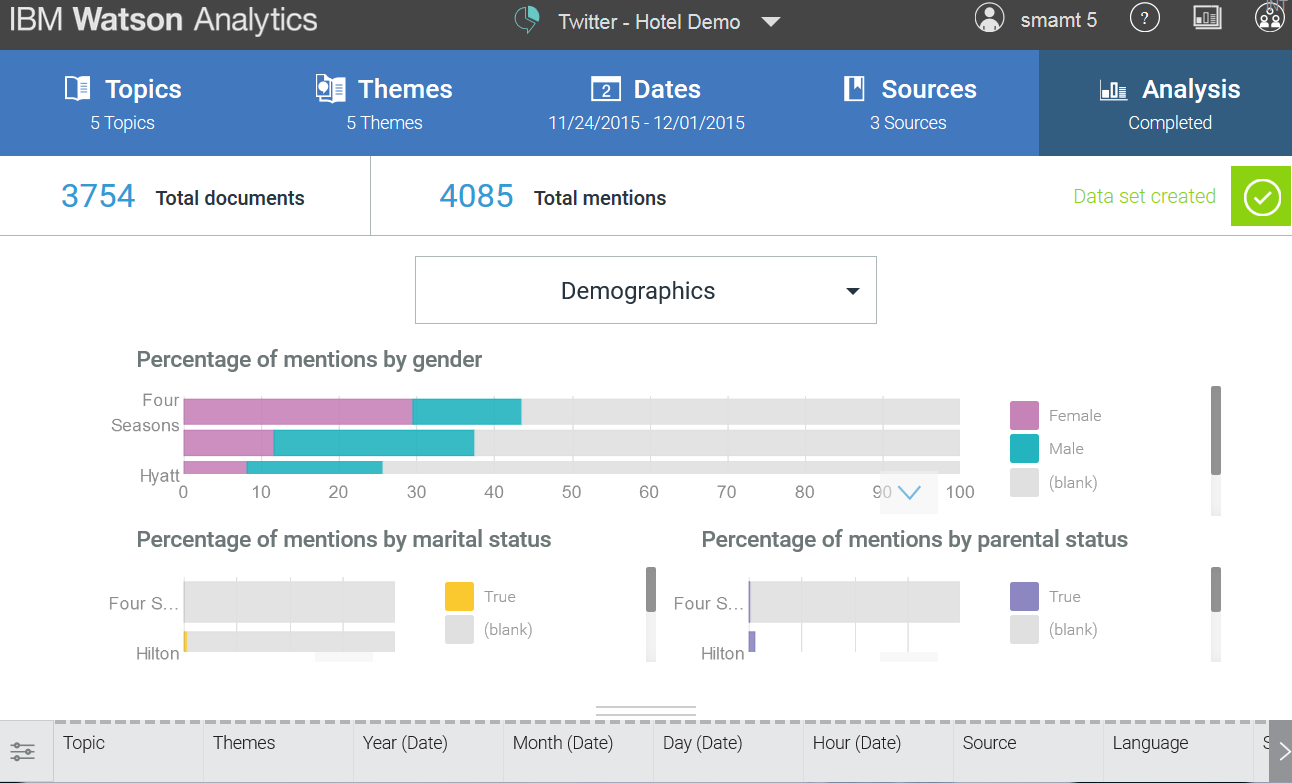
- The distribution by gender is male / female , mainly by names (dictionaries), by nicknames ( Mr. X becomes an indicator of a man, and “ Little Mermaid 99 ” is a woman), and also according to certain words used in the text of the message “ I became a mother ” - female , " I became a father " - male. Whether it always works correctly is a separate question. Some nicknames do not allow one to ascribe unequivocally a certain gender to a person; moreover, nobody forbade the ironic use of the opposite sex in the comments.
- There is also a “married / single” metric - information is taken from the profile and message text. That is, if the expression “ my wife ” is found, then the status is “married” .
- Similarly, the “childless / with children” metric works. The last two metrics in my opinion are the most controversial. In Watson does not distinguish direct speech. This means that messages like: “ Next from the words of a girlfriend / friend ... My husband / son did this ” will be processed incorrectly. Information from a friend / girlfriend will be attributed to the speaker.
- And what about the most important metrics: age and geography? But there is no data on age. Totally. None And geography is determined by the mention of the names of cities and countries in the message. And how many of us wrote that we were in Moscow or St. Petersburg, while in Saratov or Voronezh? So, without comment.
4. The spectrum of languages ​​Watson owns is quite wide, but if we are interested in sentiment analysis of messages (the main ingredient of the cake called Analysis of Social Media), then the situation is worse. It takes about 9 months to add one language. At the moment, English (who would doubt), French, German, Spanish, Dutch, Chinese (traditional and simplified versions), Russian, and Portuguese are already working quite well.
Watson distinguishes the following types of tonality: positive, negative and ambivalence. If the first two types are clear and without explanation, then the latter refers to the case when it is unclear whether the statement is positive or negative. Example: “
This camera has good color, but the sound is lame ”. If we take into account only the camera, and not its individual characteristics, then the tonality becomes ambivalent. One of the advantages of Watson’s tonality is the ability to personally add to the “tonal dictionary” those words that should not be processed positively or negatively. An example is the slogans of advertising campaigns (“
Tanks are not afraid of dirt! ”). If you do not add a slogan to “ignore” - we will get unreliable data on tonality, an abundance of positive, which in fact is not. It works only for specific customers, that is, changes the tonality only for a particular product, and not globally, for everything. One of the big drawbacks of the used dictionaries is that the power of tonality is not recorded. That is, the words “
bad - terrible - disgusting ” are equally negative for Watson. But any person will say that it is not.
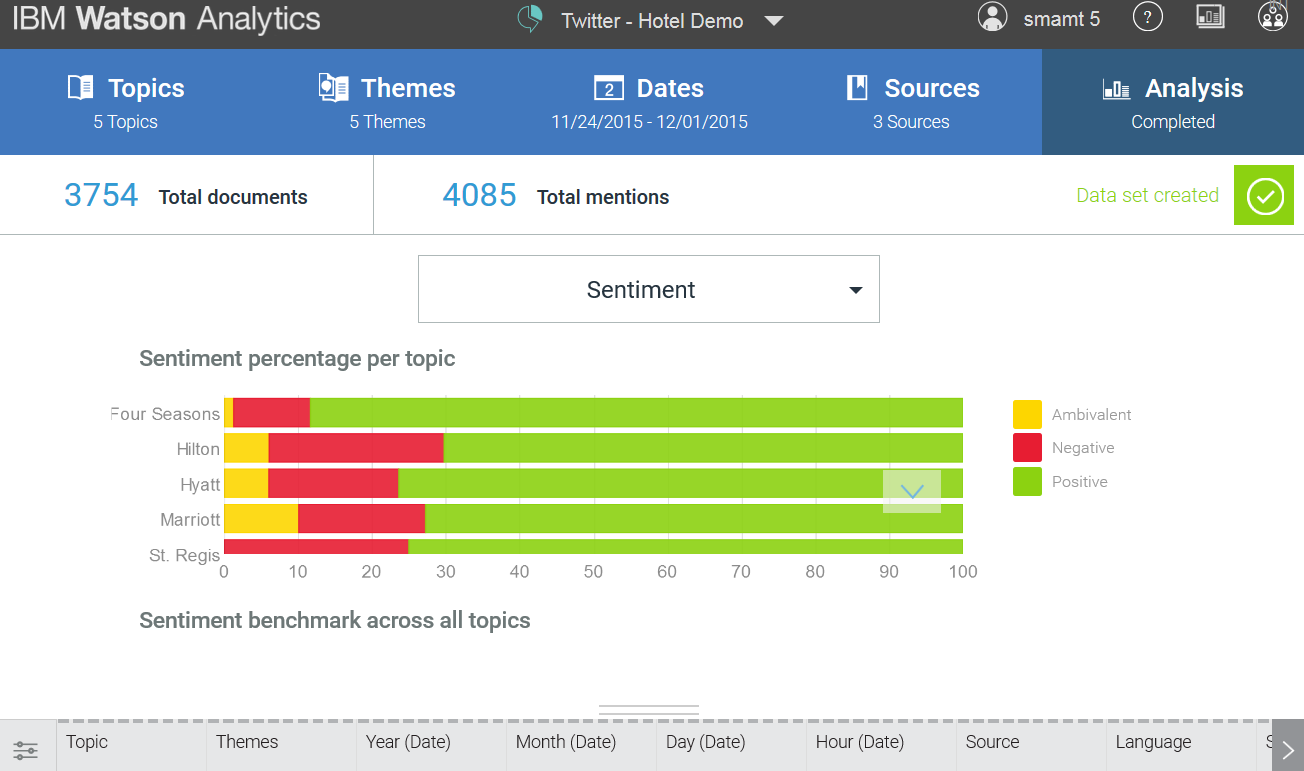
Further, linguistics (sentiment analysis, the division of sentences into parts and the above mentioned demographics) works on rules. AQL (annotation query language) is used to describe the syntax and operation of the key. See how it works, you can on the official website of
IBM .
The advantage of the approach is on the rules : with sufficient assiduity, 85–90
% of cases and features of the use of certain phrases in the language can be described.
Disadvantages : sometimes a significant layer of messages may fall out that were not taken into account when creating the rules. And if the machine algorithm can be simply retrained, then writing new rules (so that they do not conflict with the previous ones, so that the priority of execution is not violated) requires much more costs. In addition, the rules vary from language to language. If for the related languages ​​of one group, you can use the same “formulations” with minor adjustments, then to describe more rare languages ​​this will not work. No, some kind of base will remain, but ...
Alas, Watson does not allow uploading his messages to check the operation of the tonality and nowhere can you find data on the accuracy of the module. Even when working with the analysis system, the user sees not every statement, but only a small part, and only the one that is clearly defined, as positive or negative messages.
SummaryThe system pleasantly pleases the eye with an abundance of bright and colorful graphs and charts, as well as a kind of comparison criteria (demography, tonality). Easy to use, that is, you do not need to be Sherlock, just be Watson to work with her. Significantly more languages ​​offered. But the lack of data on age, questionable data on distribution by gender and geography, the inability to find out exactly what the customers are dissatisfied with (say, ambivalent expressions are the most interesting, the ones that can be turned into positive) raises some concerns about the reliability of the system. That is, Watson still has room to grow and develop, to say that he is much better than the Russian social media analysis systems, I, alas, cannot yet.