This article focuses on the data warehousing architecture. What is guided in its construction, what approaches work - and why.
"The tale is a lie - yes, there is a hint in it ..."
Planted grandfather ... storage. And the large-very-large vault has grown. But I didn’t really know how it works. And grandfather started reviewing. Grandfather called grandmother, granddaughter, a cat and a mouse on the family council. And they say the following topic: “Our vault has grown. Data from all systems are flocking, the tables are apparently invisible. Users are preparing their reports. It seems to be all right - live and live. Yes, only one sadness - no one knows how it works. Drives require apparently-invisible - you will not get enough! And then there are more users to go to me to get used to with complaints different: the report hangs, the data is outdated. And even the trouble - we come with reports to the king-father, and the figures somehow do not converge. One never knows - the king is angry - then do not demolish the head - neither me nor you. So I decided to collect and consult you: what shall we do? ”.He cast his eyes over the meeting and asked:
- Here you are, grandma, you know how it is arranged in our vault?
- No, grandfather, I do not know. Yes, and how do I know something? There are some brave lads guarded by him! One Usichi what! Do not approach. I went to see them somehow, I made pies. And they ate the pies, wiped out his mustache and said: “Why did you come, grandma? What is your vault? Tell me what report you need - we'll do it for you! You are the main pies bring more often! It hurts you they are delicious. "
- And you, granddaughter favorite, do you know how our vault is arranged?
- No, grandfather, I do not know. They gave me access to it somehow. I connected, I look - and there tables - apparently invisible. And in the schemes are different hidden. Eyes scatter ... I was confused at first. And then she looked - some of them are empty, others are filled, but only half. And the data seems to be repeated. No wonder that you will not get enough disks, with such redundancy something!
- Well, and you, cat, what do you say about our storage? Is there anything good in it?
- Yes, how not to say, grandfather - I will say. I, by grandchild's request, tried to put in a separate sheet of a pilot — a small window case. In order to understand what kind of trade is profitable for our state — what products the merchants go well for, they pay tribute — they replenish the treasury. And what - out of hand badly. And I began to select the data from this storage. Facts gathered. And he began to try to compare them against the products. And what, grandfather, I saw - they seem to be the same products, but you look at the signs - they are different! I took them then combing grandchildren comb. Chesal-scratching - and led to a certain uniformity, caressing the eye. But early I was glad - the next day I launched my scripts to update the wonderful data in the window - and I went away with everything! “How so?” - I think, - the granddaughter would go and upset - today we would have to show our pilot to the minister. How do we go something - with such data?
- Yes, sad tales, a cat, you tell. Well, and you, little mouse, never really tried to find out about the repository? You have a girl brisk, nimble, sociable! What are you telling us?
- Yes, how, grandfather, do not try - of course, I am a quiet mouse, but nimble. I asked the cat's granddaughter to get the data model of our state repository. And the cat, of course, came to me - on you, he says, mouse, all hope! Well, what a good deed good people (and cats) do not do? I went to the lock, where the storage manager hides the data model in the safe. And lurking. She waited for him to take out that model from the safe. Only he came out for coffee - I jumped to the table. I look at the model - I can not understand anything! How so? I do not recognize our repository! We have thousands of tables countless data - flows irrepressible! And here - everything is slim and beautiful ... He looked at this model - and put it back in the safe.
- Yes, absolutely strange things, you told us, mouse.
My grandfather thought hard.
- What will we do, my friends? After all, you will not live with such a storage facility for a long time ... Users will be out soon - they will completely lose their patience. 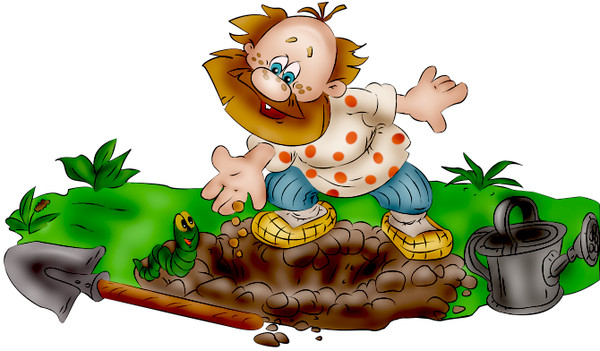
No matter what our grandfather decides from a fairy tale - to build a new vault or try to reanimate the existing one - it is necessary to draw conclusions before “rolling up our sleeves” again.
Let us put aside organizational aspects, such as the danger of concentrating expertise in a certain narrow closed group, the lack of control processes and ensuring the transparency of the systems architecture used in the enterprise, etc.
Today I would like to focus on building the architecture of a specific system (or group of systems) - data warehouses. What needs to be kept in the focus of attention first of all, when an organization is planning to build such a complex and expensive system as a repository.
')
Debriefing
None of us, working on the creation and development of a system, wants it to be a “temporary building”, or a solution that will “die” in a year or two, because It will not be able to meet the requirements and expectations of customers and business. No matter how strong a lurch in the direction of “flexible methodologies” is observed today, it is much more pleasant for a person to feel like a “master” who makes violins rather than a craftsman who planes sticks for disposable drums.
Our intention sounds natural: to make systems, sound and high-quality, that will not require us to have regular “night vigils with a file”, for which we will not be ashamed before end users and who will not look like a “black box” for all “uninitiated” followers.
To begin with, we list a list of typical problems that we regularly encounter when working with repositories. Just write down what is - so far without trying to streamline and formalize.
- In principle, we have a good storage: if you don’t touch it, everything works. However, as soon as a change is required, “local landslides” begin.
- The data is downloaded daily, according to the regulations, in one large process, for 8 hours. And it suits us. But if suddenly there is a failure - it requires manual intervention. And then everything can work unpredictably for a long time, because human involvement in the process will be required.
- Roll out the release - wait for problems.
- Any one source could not give the data in time - all the processes are waiting.
- The integrity of the data is controlled by the database — so our processes fail when it is broken.
- We have a very large storage - 2000 tables in one general scheme. And 3000 more in a variety of other schemes. We have little idea how they are arranged and for what reason they appeared. Therefore, it can be difficult for us to reuse something. And you have to solve many problems again. Because, it's easier and faster (than to understand "in someone else's code"). As a result, we have discrepancies and duplicate functionality.
- We expect the source to provide quality data. But it turns out that it is not. As a result, we spend a lot of time reconciling our final reports. And quite succeeded in this. We even have a streamlined process. True, it takes time. But users are used to ...
- The user does not always trust our reports and requires the justification of a particular figure. In some cases, he is right, and in some not. But it is very difficult for us to justify them, because we do not have the means of "end-to-end analysis" (or data lineage).
- We could attract additional developers. But we have a problem - how do we enable them to work? How to most effectively parallelize the work?
- How to develop the system gradually, without going into the development of the "core system" for a whole year?
- The data warehouse is associated with the corporate model. But we know for sure (we saw in XYZ Bank) that it is possible to build a model indefinitely (in XYZ Bank, we went for six months and discussed business entities, without any movement). And why is she at all? Or maybe it's better without her, if there are so many problems with her? Maybe it somehow generate?
- We decided to drive a model. But how to systematically develop the data warehouse model? Do we need “rules of the game” and what can they be? What will it give us? And what if we make a mistake with the model?
- Should we save the data, or the history of their changes, if "business does not need it"? I would not want to “store garbage” and complicate the use of this data for real tasks. Should the repository save history? What is it like? How does storage work with time?
- Should we try to unify the data in the repository if we have a control system for the NSI? If there is MDM, does this mean that now the whole problem with the master data has been solved?
- We are expected to replace key accounting systems soon. Should the data warehouse be ready for a source change? How to achieve this?
- Do we need metadata? What do we mean by this? Where exactly can they be used? How can you implement? Do I need to store them "in one place"?
- Our Customers are extremely unstable in their requirements and desires - something is constantly changing. In general, our business is very dynamic. While we are doing something, it is already becoming unnecessary. How do we make it so as to produce the result as quickly as possible - like hotcakes?
- Users require speed. But we cannot launch our main loading processes often, because this loads source systems (badly affects performance) - therefore, we hang up additional data streams - which will pick up the point - what we need. True, it turns out a lot of threads. And we will throw out some of the data later. In addition, there will be a convergence problem. But no other way ...
Already got quite a lot. But this is not a complete list - it is easy to add and develop. We will not hide it in the table, but hang it in a prominent place - keeping these questions in the focus of our attention during the work.
Our task is to work out a comprehensive solution as a result.
Anti-fragility
Looking at our list, you can make one conclusion. It is not difficult to create a kind of “database for reporting”, add data to it, or even build some routine data update processes. The system begins to live somehow, users appear, and with them obligations and SLA, new requirements arise, additional sources are connected, methodologies change - all this must be taken into account in the development process.
After some time, the picture is as follows:
“Here is the vault. And it works if you do not touch it. Problems arise when we have to change something. ”A change arrives to us, the impact of which we cannot assess and comprehend (since we didn’t put such tools into the system initially) - and in order not to risk it, we don’t touch what we have, but do another extension from the side, and another yet - turning our decision into slums, or as they say in Latin America, “favela”, where even the police are afraid to go.
There is a feeling of loss of control over their own system, chaos. It takes more hands to support existing processes and solve problems. And making changes is harder. In other words, the system becomes unstable to stresses, non-adaptive to changes. And besides, there is a strong dependence on characters who "know the fairway," because no one has a "map".
This property of the object - to collapse under the influence of chaos, random events and shocks -
Nassim Nicholas Taleb calls
fragility . And also introduces the opposite concept:
anti -
fragility -
when the object is not destroyed by stress and chance, but receives a direct benefit from it . (
"Anti-frailness. How to benefit from chaos" )
Otherwise, it can be called
adaptability or
resistance to change .
What does this mean in this context? What are the "sources of chaos" for IT systems? And what does it mean to “take advantage of the chaos” in terms of IT architecture?
The first thought that comes to mind is the changes that come from outside. What is the outside world for the system? For storage in particular. Of course, first of all - changes by the data sources for the repository:
- changing the formats of incoming data;
- replacement of some data source systems with others;
- change of rules / platforms for system integration;
- changing data interpretations (formats are saved, the logic of working with data changes);
- changing the data model, if the integration is done at the data level (parsing the database transaction log files);
- data growth — as long as there was little data in the source system, and the load was small — it was possible to take it anytime, arbitrarily heavy query, data and load increased — now there are strict limitations;
- etc.
The source systems themselves, the composition of the information and its structure, the type of integration interaction, as well as the logic of working with data can change. Each system implements its own data model and approaches to work with them that meet the goals and objectives of the system. And no matter how they strive to unify industry-specific models and reference practices, all the same, nuances will inevitably emerge. (And besides, the process of sectoral unification itself, for various reasons, is not much advanced.)
The culture of working with corporate data - the availability and control of the information architecture, a single semantic model, master data management systems (MDM) somewhat simplify the task of consolidating data in the repository, but do not exclude its necessity.
No less critical changes are initiated by the storage consumers (changing requirements):
- previously, there was enough data to build a report - now it was necessary to connect additional fields or a new data source;
- previously implemented data processing techniques are outdated - you need to rework the algorithms and everything that affects it;
- Previously, everyone was satisfied with the current value of the directory attribute on the information panel - now the value that is relevant at the time of the occurrence of the analyzed fact / event is required;
- there was a requirement for the depth of data storage history, which was not there before - to store data not for 2 years, but for 10 years;
- Previously, there was enough data on the “end of day / period” state — now the state of the data is “intra day” or at the time of a certain event (for example, decision making on a loan application - for Basel II)
- we were previously satisfied with reporting data as of yesterday (T-1) or later; now we need T0;
- etc.
Both integration interactions with source systems and requirements from the data warehouse users are external factors for the data warehouse: some source systems replace others, data volumes grow, incoming data formats change, user requirements change, etc. And all this is typical external changes to which our system - our storage - should be ready. With the right architecture, they should not kill the system.
But that is not all.
Speaking about variability, we, first of all, recall external factors. After all, inside we can control everything, so it seems to us, right? Yes and no. Yes, most of the factors that are outside the zone of influence are external. But there is also “internal entropy”. And precisely because of its presence, we sometimes need to return “to the point 0”. Start the game again.
In life, we often tend to start from scratch. Why is this characteristic of us? And is it so bad?
With reference to IT. For the system itself - this may turn out to be very good - the opportunity to review individual decisions. Especially when we can do it locally. Refactoring is the process of disentangling that “web” that periodically arises in the process of system development. Returning “to the beginning” may be useful. But it has a price.
With proper management of the architecture, this price is reduced - and the process of developing the system itself becomes more controlled and transparent. A simple example: if the principle of modularity is observed - a separate module can be rewritten, without affecting external interfaces. And this can not be done with a monolithic structure.
Anti-fragility of the system is determined by the architecture that is incorporated in it. And it is this property that makes it adaptive.When we talk about adaptive architecture - we mean that the system is able to adapt to changes, and not at all that we are constantly changing the architecture itself. On the contrary, the more stable and stable the architecture, the fewer the requirements that entail its revision, the more adaptive the system.A much higher price will have solutions that involve the revision of the entire architecture. And for their adoption you need to have very good reasons. For example, such a basis may be a requirement that cannot be implemented within the framework of the existing architecture. Then they say - there is a requirement that affects the architecture.
Thus, we also need to know our “boundaries of anti-fragility”. The architecture is not developed “in a vacuum” - it relies on current requirements and expectations. And if the situation is fundamentally changing - we must understand that we have gone beyond the current architecture - and we need to reconsider it, work out a different solution - and think over the transition paths.
For example, we assumed that we will always need data at the end of the day in the repository, we will do the data collection every day using standard system interfaces (through a set of views). Then from the risk management division came the requirements of the need to receive data not at the end of the day, but at the time of the decision on lending. No need to try to “stretch the tension” - you just need to recognize this fact - the sooner the better. And begin to work out an approach that will allow us to solve the problem.
A very fine line arises here - if we take into account only the “requirements at the moment” and do not look at a few steps forward (and a few years ahead), then we increase the risk of facing the requirement affecting the architecture too late - and our price The change will be very high. Looking a little ahead — within the boundaries of our horizon — has never harmed anyone.
An example of a system from a “fairy tale about storage” is just an example of a very shaky system built on fragile design approaches. And if this happens - destruction occurs rather quickly, precisely for this class of systems.
Why can I say that? The repository theme is not new. The approaches and engineering practices that were developed during this time were aimed precisely at this - maintaining the viability of the system.
A simple example: one of the most frequent reasons for the failure of storage projects “on take-off” is an attempt to build a storage above source systems that are under development without coordinating integration interfaces — an attempt to take data directly from the tables. As a result, they went into development - during this time, the source database has changed - and the download threads to the storage became inoperable. Redoing something late. And if you are still not secured by making several layers of tables inside the storage, then everything can be thrown out and started anew. This is just one of the examples, and one of the simplest.
The criterion of the fragile and anti-fragile according to Taleb is simple. The main judge is time. If the system withstands the test of time, and shows its "vitality" and "indestructibility" - it has the property of anti-fragility.
If, when designing a system, we take anti-brittleness as a requirement into account, then this will encourage us to use such approaches to building its architecture that will make the system more adaptive both to “chaos from the outside” and to “chaos from the inside”. And ultimately the system will have a longer lifespan.
None of us would like to make a "temporary". And do not deceive yourself that it is impossible today. Looking a few steps forward is normal for a person at any time, especially in a crisis.
What is a data warehouse and why we build it
An article on the repository architecture suggests that the reader is not only aware of what it is, but also has some experience with similar systems. Nevertheless, I thought it necessary to do this - go back to basics, to the beginning of the path, because this is where the “fulcrum” of development is located.
How do people come to the conclusion that data storage is needed? And how do they differ from just a “very large database”?
Long ago, when the world simply lived “business data processing systems”, there was no separation of IT systems into such classes as frontal oltp systems, back-office dss, text data processing systems, data storage, etc.
It was at the time when the first relational DBMS Ingres was created by
Michael Stonebreaker .
And it was a time when the era of personal computers whirled into the computer industry and forever turned all the ideas of the IT community of that time.
Then it was easy to meet corporate applications written on the basis of the desktop class DBMS, such as Clipper, dBase and FoxPro. And the market of client-server applications and DBMS only gained momentum. One after another, database servers appeared that would permanently occupy their niche in the IT space - Oracle, DB2, etc.
And the term "database application" was extended. What included such an application? Simplified - some input forms through which users could simultaneously enter information, some calculations that were run “by button” or “on schedule”, as well as some reports that could be seen on the screen or saved as files and sent to print.
"Nothing special - a regular application, only there is a database," - said one of my mentors at an early stage of the work path. “Is it anything special?” - I thought then.
If you look closely, there are some peculiarities. With the growth of users, the volume of incoming information, as the load on the system increases, its designers and designers, in order to keep their performance at an acceptable level, go to certain "tricks". The first is the separation of the monolithic “business data processing system” into an accounting application that supports users on-line, and separately allocate an application for batch data processing and reporting. Each of these applications has its own database and is even placed on a separate instance of the database server, with different settings for different load patterns - OLTP and DSS. And between them are lined up data streams.
It's all? It would seem - the problem is solved. What happens next?
And then companies grow, their information needs are multiplying. The number of interactions with the outside world is growing. And in the end there is not one big application that fully automates all processes, but several different ones from different manufacturers. The number of systems generating information - the system of data sources in the company is increasing. And sooner or later, there will be a need to see and compare among themselves the information obtained from different systems. So in the company data warehouses - a new class of systems.
The generally accepted definition of this class of systems is as follows.
Data Warehouse (or Data Warehouse) - a domain-specific information database specifically designed and developed for preparing reports and business analysis to support decision making in an organization
Thus, the consolidation of data from different systems, the ability to look at them in some “single” (unified) manner is one of the key features of data warehouse class systems. This is the reason why repositories appeared during the evolution of IT systems.Key features of data warehousing
Let's take a closer look. What are the key features of these systems? What distinguishes data warehouses from other IT enterprise systems?
First, these are large volumes. Very big.
VLDB - this is what leading vendors call such systems when they give their recommendations on how to use their products. From all systems of the company, data flows into this large database and is stored there “forever and permanently”, as they are written in textbooks (in practice, life turns out to be more complicated).
Secondly, this is historical data -
“Corporate memory” - this is how data warehouses are called. In terms of working with time in storage, everything is quite interesting. In accounting systems, the data is current at the moment. Then the user performs a certain operation - and the data is updated. At the same time, the change history may not be preserved - it depends on the accounting practice. Take, for example, a bank account balance. We may be interested in the actual balance for "now", at the end of the day or at the time of a certain event (for example, at the time of calculating the scoring score). If the first two are solved quite simply, then the latter will most likely require special efforts. The user, working with the repository, can refer to past periods, compare them with the current, etc. It is precisely these possibilities associated with time that essentially distinguish data warehouses from accounting systems — obtaining the status of data at various points on the time axis — to a certain depth in the past.
Thirdly, it is
consolidation and
unification of data . In order to make their joint analysis possible, it is necessary to bring them to a common form - a
single data model , to compare the facts with the unified reference books. There may be several aspects and difficulties.
First of all - conceptual - under the same term different people from different departments can understand different things. And vice versa - to call differently something that in essence is one and the same. How to ensure a “unified view” and at the same time preserve the specifics of the vision of a particular group of users?Fourth, this is work with data quality. . , . – . , - – , « » , . , –
, .
Anyone who has encountered a repository, most likely observed some kind of "layered structure" - because It is this architectural paradigm that has taken root for systems of this class. And not by chance. The layers of the storage can be perceived as separate components of the system - with their tasks, area of ​​responsibility, "rules of the game."The layered architecture is a means of dealing with the complexity of the system - each subsequent level is abstracted from the complexities of the internal implementation of the previous one. Such an approach allows to single out tasks of the same type and solve them in a uniform way, without inventing a “bicycle” from scratch every time.A schematic conceptual architectural scheme is presented in the figure. This is a simplified scheme that reflects only the key idea - the concept, but without the “anatomical details” that will arise with a deeper study of the details.
As shown in the diagram, conceptually select the following layers. Three main layers that contain a data storage area (indicated by a filled rectangle) and data loading software (conventionally indicated by arrows of the same color). As well as an auxiliary - service layer, which, however, plays a very important connecting role - data loading control and quality control.Primary Data Layer - a layer of primary data (or staging , or an operating layer ) - is designed to load from source systems and save primary information, without transformations - in the original quality and support a complete change history.The task of this layer- to abstract the subsequent layers of storage from the physical device data sources, methods of data collection and methods for isolating the delta of changes.Core Data Layer — the core of the repository — is the central component of the system that distinguishes storage from simply “batch integration platform” or “big data dump”, since its main role is to consolidate data from different sources, leading to uniform structures, keys. It is during loading into the kernel that the main work is done with data quality and general transformations, which can be quite complex.The task of this layer- to abstract their customers from the logical structure of data sources and the need to compare data from different systems, to ensure the integrity and quality of data.Data Mart Layer — analytic showcases — a component whose main function is to convert data to structures convenient for analysis (if BI works with showcases, then this is usually the dimensional model) or according to the requirements of the consumer system.As a rule, storefronts take data from the core - as a reliable and verified source - i.e. use the service of this component to bring the data to a single form. We will call such windows regular . – ( ). , , , , .
. . ,
.
– – BI-, , .
The layers described above consist of a permanent data storage area, as well as a program module for loading and transforming data. This division into layers and regions is logical. Physically, the implementation of these components can be different - you can even use different platforms for storing or transforming data on different layers, if it is more efficient.Storage areas contain technical (buffer tables) that are used in the data transformation process and target tables. , -. «» . . (-), , .
There is also a special component (or a set of components) that provides service functions for all layers. One of its key tasks, the control function, is to ensure “uniform rules of the game” for the entire system, leaving the right to use various options for implementing each of the layers described above - including use different data loading and processing technologies, different storage platforms, etc. We will call it a service layer (Service Layer) . It does not contain business data, but has its own storage structures - it contains a metadata area, as well as an area for working with data quality (and possibly other structures, depending on the functions assigned to it).Such a clear separation of the system into individual components significantly increases the controllability of the development of the system:- , , ( , , ) – , delivery;
- ( , ) – .. , ;
- , , , ( – , );
- ( , ) , – , ;
- the selection of window displays allows you to take into account the differences and specificity of data understanding that users of different departments may have, and designing them for the BI requirements allows not only to produce aggregated figures, but to ensure validation of the data by providing drill down to primary indicators;
- the presence of the service layer allows you to perform end-to-end data analysis (data lineage), use unified data audit tools, common approaches to isolating the delta of changes, working with data quality, loading management, monitoring tools and error diagnostics, speeds up the resolution of problems.
This approach to decomposition also makes the system more resilient to change (compared to the “monolithic structure”) - ensures its anti-fragility:.
« » — .
Core Layer. — , , . – , , .
, , - , , , .
, , -, .
– . . « ». , , , .
1. – , ().
. , -, , , – 20-30.
2. « » — – . – .
. , .. . , , . « » , - . « » — . , – ERP- ( CRM) - « ». , .
3. , . , .
. , . « » «» — .. « ». , - 4 , « », ( ) . .
. , « » — , , , . , , -. , . , ..
– , . : ( ) , , - .. . , – « » .
Developing a data model is not a process of inventing and inventing something new. In fact, a data model already exists in the company. And the process of its design is more like an "excavation". The model is carefully and carefully extracted to the light from the “ground” of corporate data and is clothed in a structured form.Myth 4. In our company, the business is so dynamic, and everything is changing so quickly that it is useless for us to make a model - it will become obsolete before we put this part of the system into operation.In fact. Recall that the key factor in the core is stability. And above all, the topology of the model. Why?
Because it is this component that is central and affects everything else. Stability is also a requirement for the kernel model. If a model becomes obsolete too quickly, then it is incorrectly designed. For its development, the wrong approaches and “rules of the game” were chosen. And it is also a matter of qualitative analysis. Key entities of the corporate model change extremely rarely.But if it comes to our mind to make for a company trading in, say, confectionery, instead of the directory "Products" make "Candy", "Cakes" and "Pies". Then when pizza appears in the list of products - yes, you will need to enter a lot of new tables. And this is just a matter of approach.Myth 5. Creating a corporate model is a very serious, complex and responsible business. And scary to make a mistake.In fact.The core model, although it should be stable, is still not “cast in metal”. Like any other design decisions, its structure can be revised and modified. Just do not forget about her quality. But this does not mean at all that “one should not breathe” on it. And this does not mean that temporary solutions and “stubs” that should be planned for recycling are unacceptable.Myth 6. If we have a data source, for example, the NSI system (or the master data management system - MDM), then it should correspond to the corporate model in an amicable way (especially if it was recently designed, and did not have time to collect it). , "Traditions" and temporary shelters). It turns out that for this case - we do not need a kernel model?In fact. , – .. . . Why? – ( ), , , ( ) ..
, – , , « » . , , – – .
( staging )
In the
diagram, it is designated as Primary Data Layer. The role of this component: integration with source systems, loading and storage of primary data, as well as preliminary data cleansing - checking for compliance with the rules of format-logical control, recorded in the “interface interaction agreement” with the source.
In addition, this component solves a very important task for the repository - identifying the “true delta of changes” - regardless of whether the source allows tracking changes in the data or not and how (by what criterion you can “catch” them). As soon as the data got into the staging - for all other layers, the issue of delta selection is already clear - thanks to the labeling with meta-attributes.
The data in this layer is stored in structures as close as possible to the source system — in order to keep the primary data as close as possible to their original appearance. Another name for this component is “operational layer”.
Why not just use the well-established term “staging”? The fact is that earlier, before the “era of big data and VLDB”, disk space was very expensive - and often the primary data, if saved, then a limited time interval. And often the name “staging” is called the buffer to be
cleared .
Now technologies have stepped forward - and we can afford not only to store all primary data, but to historize them with the degree of granularity that is only possible. This does not mean that we should not control the growth of data and does not eliminate the need to manage the life cycle of information, optimizing the cost of storing data, depending on the “temperature” of use - that is, taking away “cold data”, which is less in demand, to cheaper carriers and storage platforms.
What gives us the presence of "historicized styling":
- the possibility to make a mistake (in structures, in transformation algorithms, in granularity of history management) - having completely historical data in the accessibility area for the repository, we can always reload our tables;
- opportunity to think - we can not rush to study a large fragment of the core in this iteration of the development of the repository, since in our pricing, in any case, they will be, and with an even time horizon (there will be one “reference point of history”);
- possibility of analysis - we will save even the data that is no longer in the source - they could wipe there, go to the archive, etc. - here they remain available for analysis;
- the possibility of an information audit - thanks to the most detailed primary information, we can then figure out - how the download worked for us, that we eventually got these numbers (for this we need to have labeling with meta-attributes and the corresponding metadata for which the download works - this is solved at the service layer).
What difficulties may arise when building "historicized styling":
- it would be convenient to set the requirements for the transactional integrity of this layer, but practice shows that this is difficult to achieve (this means that in this area we do not guarantee the referential integrity of the parent and child tables) - integrity alignment occurs on subsequent layers;
- This layer contains very large volumes (the most voluminous in the storage - despite all the redundancy of analytical structures) - and you should be able to handle such volumes - both in terms of load and in terms of queries (otherwise you can seriously degrade the performance of the entire storage).
What else interesting can be said about this layer.
First, if we move away from the paradigm of “through loading processes”, then the rule “the caravan goes at the speed of the last camel” no longer works for us, or rather we abandon the principle of “caravan” and go to the principle of “conveyor”: took data from source - put in your layer - ready to take the next batch. It means that
1) we do not wait until processing occurs on other layers;
2) we do not depend on the schedule for providing data by other systems.
Simply put, we schedule the download process, which takes data from one source through a certain way of connecting to it, checks, allocates a delta - and puts the data into target styling tables. And that's all.
Secondly, these processes, apparently, are arranged very simply - it can be said trivially, from the point of view of logic. And that means - they can be very well optimized and parameterized, reducing the load on our system and speeding up the process of connecting sources (development time).
For this to happen, you need to know very well the features of the technological features of the platform on which this component runs - and then you can make a very effective tool.
Analytical showcase layer
The Vitrin layer (Data Mart Layer
in the diagram ) is responsible for preparing and providing data to end users - people or systems. At this level, the requirements of the consumer are taken into account as much as possible - both logical (conceptual) and physical. The service should provide exactly what is needed - no more, no less.
If the consumer is an external system, then, as a rule, it dictates the data structures that it needs and the rules for collecting information. A good approach is considered one in which the consumer is responsible for correctly collecting the data. The data storage prepared, formed the storefront, provided the possibility of incremental data acquisition (marking with meta attributes for the subsequent allocation of the delta of changes), and the consumer system itself controls and is responsible for how it uses this storefront. But there are some peculiarities: when the system does not have an active component for data acquisition, you need either an external component that performs an integrating function, or the storage will act as an “integration platform” - and will ensure correct incremental data shipment later - outside the storage. Many nuances are coming up here, and the rules of interface interaction should be thought out and understandable to both parties (however, as always, when it comes to integration). Regular cleansing / archiving of data is usually applied to such showcases (it is rarely necessary for this “transit data” to be stored for a long time).
From the point of view of analytical tasks, the greatest value is represented by “for people” showcases - more precisely, for the BI tools with which they work.
However, there is a category of “highly advanced users” - analysts, data researchers - who do not need either BI tools or regulatory processes for filling external specialized systems. They need some kind of “common storefronts” and “own sandbox”, where they can create tables and transformations at their own discretion. In this case, the responsibility of the repository is to ensure that these common storefronts are filled with data in accordance with the regulations.
Separately, you can select such consumers as the means of Data Mining - deep data analysis. Such tools have their own requirements for data preparation, and data research experts also work with them. For storage, the task is reduced - again to the support of the service for loading certain display windows of an agreed format.
However, back to the analytical showcases. They are of interest from the point of view of developers, storage designers in this data layer.
In my opinion, the best approach to the design of data marts, time-tested, which almost all BI platforms are now “sharpened” is the approach of
Ralph Kimball . It is known as
dimensional modeling - multidimensional modeling. There are a great many publications on this topic. For example, the basic rules can be found in the publication
Marga Ross . And of course, we can recommend
the best-selling book from the guru of multidimensional modeling. Another useful resource is
Kimball Tips.The multidimensional approach to the creation of storefronts is described and worked out so well - both by the “evangelists of the method” and by the leading software vendors that it makes no sense to dwell on it here in any way — the source is always preferable.
I would like to make only one accent. "Reporting and analytics" is different. There are “heavy reporting” - pre-ordered reports that are generated as files and delivered to users through the delivery channels provided. And there are information panels - BI dashboards. At its core, these are web applications. And to the response time of these applications are the same requirements as for any other web application. This means that the normal update time of the BI panel is seconds, not minutes. It is important to remember this when developing a solution. How to achieve this? Standard optimization method: we look, what is the sum of the response time and what we can influence. What is the most time spent on? On physical (disk) readings of a DB, on data transmission on a network. How to reduce the amount of read and transmitted data in one request? The answer is obvious and simple: you need to either aggregate the data, or put a filter on large tables of the fact tables involved in the query, and eliminate the joining of large tables (references to fact tables should be made only through measurements).
What is BI for? How is he comfortable? Why is the multidimensional model effective?
BI allows the user to perform so-called "ad hoc requests." What does it mean? This means that we do not exactly know the request beforehand, but we know which indicators in which cuts the user can request. The user creates such a query by selecting the appropriate BI filters. And the task of the BI developer and the storefront designer is to ensure that the logic of the application works so that the data is either filtered or aggregated, avoiding the situation when there is too much data being requested - and the application is “hung”. Usually they start with aggregated figures, further delving into more detailed data, but at the same time setting the necessary filters.
It is not always enough just to build the “right star” - and get a convenient structure for BI. Sometimes it will be necessary to apply denormalization somewhere (looking around at the same time as it will affect loading), and somewhere to make secondary showcases and units. Somewhere add indexes or projections (depending on the DBMS).
Thus, by “trial and error” you can get a structure that is optimal for BI - which will take into account the features of both the DBMS and the BI platform, as well as the user requirements for data presentation.
If we take data from the “core”, such window processing will be local in nature, without affecting the complex processing of primary data obtained directly from source systems - we only “shift” the data into a format convenient for BI. And we can afford to do this many times, in different ways, in accordance with different requirements. It is much easier and faster to do this on the kernel data than to collect from the “primary organization” (the structure and rules of which, as we know, can also “float”).
Service layer
The service layer (
in the diagram , the Service Layer) is responsible for implementing common (service) functions that can be used to process data in various storage layers — load management, data quality management, problem diagnostics and monitoring tools, etc.
The presence of this level provides transparency and structured data streams in the repository.
This layer includes two data storage areas:
- metadata area - used for data loading control mechanism;
- data quality domain — for implementing off-line data quality checks (that is, those that are not embedded directly into ETL processes).
You can build the process of managing the load in different ways. One of the possible approaches is as follows: we divide the entire set of storage tables into modules. Only one layer tables can be included in the module. The tables included in each module are loaded as part of a separate process. Let's call it the
control process . The launch of the control process is placed on its schedule. The control process orchestrates the calls of atomic processes, each of which loads one target table, and also contains some common steps.
Obviously, it is enough just to divide the staging tables into modules — by source systems, or rather by their connection points. But for the kernel to do it is already more difficult - because there we need to ensure the integrity of the data, and therefore we need to consider dependencies Those. there will be collisions that need to be resolved. And there are different methods for resolving them.
An important point in managing the load is to work out a common approach to error handling. Errors are classified by severity level. If a critical error occurs, the process should stop, and as soon as possible, because its occurrence indicates a significant problem that may lead to data corruption in the storage. Thus, management of loading is not only starting processes, but also stopping them, as well as preventing late start (by mistake).
For the operation of the service layer, a special metadata structure is created. In this area, information will be stored on loading processes, loaded data sets, control points, which are used to maintain the increment (which process I read to which point) and other service information necessary for the functioning of the system.
It is important to note that all target tables in all layers are marked with a special set of meta-fields, one of which is the process identifier that updated this line. For tables inside the repository, such marking by the process allows the use of a unified method for the subsequent allocation of a delta of changes. When loading data into the layer of primary data, the situation is more complicated - the delta extraction algorithm for different loaded objects can be different. But the logic of processing the accepted changes and their rolling on the target tables for the kernel and windows is much more complicated than for staging, where everything is rather trivial - it is easy to parameterize and think up reusable typical steps (procedures).
I don’t set myself the task here to fully cover this topic - organizing downloads - I only place accents that you should pay attention to.
The above approach is just one of the options. He is quite adaptive. And its “conceptual prototype” served as the Toyota pipeline and the “just-in-time” system. Those. here we are moving away from the widespread paradigm of exclusively “night data loading”, and we load it in small portions during the day - as data is available in various sources: what has come is uploaded. In this case, we have many parallel processes. And the “hot tail” of the fresh data will constantly “blink” - and after some time level off. We have to take into account this feature. And if necessary, create custom showcases with “slices”, where everything is already complete. Those. it is impossible to simultaneously achieve both efficiency and consistency (integrity). A balance is needed - one thing is important somewhere, another is somewhere.
It is imperative to provide logging and monitoring tools. A good practice is to use typed events, where you can set different parameters and set up a notification system - subscribe to certain events. Since it is very important that when the intervention of the system administrator is required - he would know about it as soon as possible and receive all the necessary diagnostic information. Logs can also be used to analyze post-factum problems, as well as to investigate incidents of system malfunctions, including data quality.
Design and maintain data warehouse models
Why is it important to pay attention to the design of data models when developing any system where the database is involved (and especially in the repository)? Why not just throw a set of tables, anywhere - even in a text editor? Why do we need these pictures?
Oddly enough, such questions are posed even by experienced developers.
Actually, yes, nothing prevents you from scribbling the tables - and start using them. If ... if at the same time in the head (!) The developer has a harmonious overall picture of the structure that he sculpts. And what if there are several developers? And what if someone else uses these tables? And what if time passes - a person leaves this area, and then comes back to it again?
Is it possible to understand without a model? In principle, it is possible. And to understand, and "estimate the pictures on a piece of paper," and "shuffle - figure out" the data. But it is much simpler, clearer and faster to use a ready-made artifact - a data model. And also to understand the "logic of its device" - that is, It would be nice to have general rules of the game.
And the most important thing is not even that. Most importantly, when designing a model, we are forced (simply without options!) To more thoroughly and deeply study the subject area, the features of the data device and their use in various business cases.
And those questions that we would easily “push aside” as complex, “muddy”, throwing our tablets without trying to design a model - we will have to put and solve now, when analyzing and designing, and not later - when we build reports and think about “how to reduce incompatible” and “reinvent the wheel” every time.This approach is one of those engineering practices that allow you to create anti-fragile systems. Since they are clearly arranged, transparent, convenient for development, their “limits of fragility” are immediately visible - you can more accurately estimate the “scale of disaster” when new requirements appear and the time required for redesign (if needed).Thus, the data model is one of the main artifacts that must be maintained during the development of the system. In an amicable way, it should be “on the table” with every analyst, developer, etc. - everyone who participates in system development projects.Data model design is a separate, very extensive topic. When designing storage facilities, two basic approaches are used.For the core, the “entity-relationship” approach is well suited - when a normalized (3NF) model is built on the basis of a study of the subject area, more precisely, its selected area. Here is the very "corporate model", which was discussed above.When designing analytical storefronts, a multidimensional model is suitable . . - – .. , – () , . – « », . – « »: « » , . .
, . , Oracle , .. Vertica – , , .
— , , (.. ). , , -, Vertica.
BI, .
, :
- () — — , « » ;
- – () ;
- – , .
, , . , – .
.
- – « », – . . – . . , « ».
- – , . ( ) ().
- , . . , – , , .
- () , – .. , .

, . . , . , , .
–
Data storage is always “custom development”, not a boxed solution. Yes, there are industry-specific BI applications, including a reference data model, pre-configured ETL processes from common sources (for example, ERP systems), a set of typical BI panels and reports. But in practice, the repository is extremely rarely introduced - as a “box”. I have been working with repositories for about 10 years, and have never seen such a story. They always emerge their nuances associated with the unique features of the company - both business and IT landscape. Therefore, it is hoped that the architecture will be provided by a “vendor” supplying the solution somewhat recklessly. The architecture of such systems often "matures" within the organization itself. Or it is formed by specialists of the contracting company, which is the main executor of the project.Data storage is an integration project.
-. « » . , -, « », .. . «» , .
, « ». – . .
–
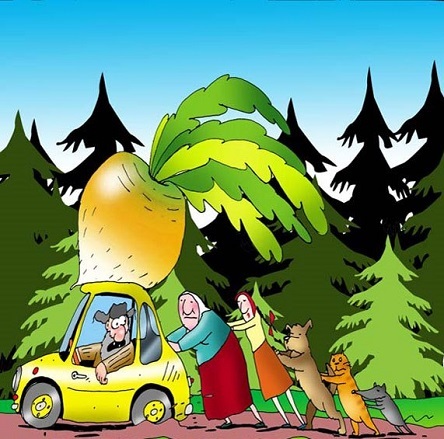
. , , .
, , . .
, , – : . – , . « », №1 – (. ).
I will clarify - the statement is true for a “live” working repository, integrated with key sources, possessing historical data and providing information and analytical services to many divisions of the company.What are my reasons to believe so?Firstly, building a storage facility is a very resource-and-cost process: in addition to the actual hardware costs, licenses for the necessary technological software and for development, almost all the systems and departments of the company are also involved. To repeat this whole process from scratch again is a very daring undertaking.Secondly, if the storage has the correct architecture, then it can quite easily survive both the change of source systems, the emergence of new requirements from the end users, and the growth of data volumes., – .
, , – -, , ..
– , . , « » - - « ». .
, , «» , .
, « » — «» . – , – , . , , - « », .
– «- »
-. ( ), – .
. , , . , .
Despite the fact that the topic of repositories is very “ancient” (if such a word is applicable for such a young industry as IT) and is quite conservative. Nevertheless, progress does not stand still - and those limitations that previously existed due to expensive and slow disks, expensive memory, etc. - now withdrawn. And at the same time, it is time to revise some architectural approaches. And this applies both to technology platforms and to the architecture of application systems that are based on them.
It is important to maintain a balance here - and to maintain a rather “eco-friendly” approach both to resources and to stored information. Otherwise, you can very quickly turn the storage into a weakly structured “trash”, in which, if it can be sorted out, it can be done through quite a lot of effort., , , , , « » «».
– , , – , .
? , , , , .
– .
, «», . – (OLTP, DSS & DWH). , , - ..
— , – .. . , . , «» « » , .
, – , , . () . , 90-
III , , . 10
, – .
, «» (one-size-fits-all) , , , , .
. –
C-Store – ,
shared nothing (SN) , .
HP Vertica .
It seems that now the topic of data warehousing has slipped into a new round of development. New technologies, approaches and tools are emerging. Their study, approbation and reasonable application allows us to create really interesting and useful solutions. And bring them to the introduction, enjoying the fact that your development is used in real work and benefit.Epilogue
In preparing this article, I tried to focus primarily on architects, analysts and developers who work directly with data warehouses. But it turned out that inevitably "took the topic a little wider" - and other categories of readers came into view. Some moments seem controversial, some are not clear, some are obvious. People are different - with different experiences, backgrounds and positions.For example, the typical questions of managers - “when should architects be involved?”, “When should architecture be engaged?”, “Architecture — wouldn't it be too expensive?” Sound quite strange for us (developers, designers) because the system architecture appears to us with her birth, it doesn’t matter whether we realize it or not. And even if there is no formal role for an architect in a project, a normal developer always “includes his own internal architect”.By and large, it does not matter - who exactly plays the role of the architect - it is important that someone puts such questions and investigates answers to them. If the architect is clearly distinguished, it only means that he is primarily responsible for the system and its development.Why did the topic of “anti-fragility” seem relevant to this subject?:
“ , , - , , , – ” / ./
, – , , .