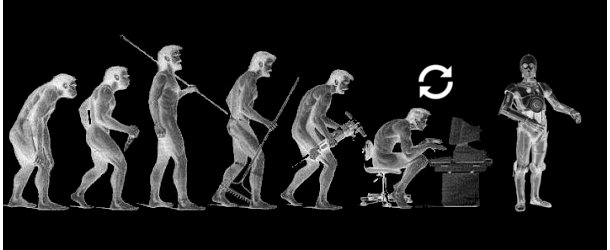
Today, only lazy does not write about artificial intelligence. For example,
Autodesk believes that artificial intelligence can take into account many more factors than a person, and thus provide more accurate, logical, and even more creative solutions to complex problems. At the University of Oxford, it is generally
suggested that artificial intelligence in the near future may replace full-time journalists and write reviews and articles for them (and that is what the Pulitzer Prize will win).
The general interest in the topic of artificial intelligence has long gone beyond scientific conferences and excites the minds of writers, filmmakers and the general public. It seems that from the future, in which the robots (or Skynet) rule the world or at least solve most of the daily tasks, it is a stone’s throw away. But what do the scientists themselves think about this?
')
First of all, it is worthwhile to deal with the term “artificial intelligence”: there are too many conjectures and artistic exaggerations on this subject. In this matter, it is best to contact the author of this term (and in combination the creator of the Lisp language and the laureate of many awards) - John McCarthy. In the
article of the same name (“What is artificial intelligence?”) McCarthy gave the following definition:
This is the science and technology of creating intelligent machines, in particular - intelligent computer programs. Artificial intelligence is associated with the task of using computers to understand how human intelligence works, but is not limited to the use of methods observed in biology.
It turns out that artificial intelligence and human intelligence are closely related? Not quite so - McCarthy himself emphasized: if the intellect "in general" is the "computational" component of what helps the subject to achieve specified goals, then the intelligence of man, animals and machines will work differently.
It turns out that artificial intelligence is not akin to human, although many futurists, writers and even scientists want to believe that this is not so. This is often repeated by
Michael Jordan , an honorary professor at the University of California at Berkeley. He believes that a lack of understanding of what artificial intelligence is, leads not only to the creation of “beautiful images” not related to real science, but to the real disinformation and various myths that flourish in this area.
Myth one: to create or improve artificial intelligence, you need to understand how the human brain works.Jordan
argues that this is not the case. The work of artificial intelligence, as a rule, has nothing to do with how the human intellect is arranged. This “myth” is deeply rooted due to the public's predilection for “beautiful ideas”: the authors of popular science articles on artificial intelligence liked metaphors taken from neurobiology.
In fact, neuroscience has a very indirect relationship (or no relation at all) to the work of artificial intelligence. For Michael Jordan, the idea that “deep learning requires an understanding of how information is processed and the human brain learns” sounds like blatant lies.
“Neurons” involved in in-depth training is a metaphor (or, to put it in Jordan language, a “caricature” of the brain in general), which is used only for brevity and convenience. In reality, the work of the mechanisms of the same in-depth training is much closer to the procedure of building a statistical model of logistic regression than to the work of real neurons. At the same time, it does not occur to anyone for “brevity and convenience” to use the “neuron” metaphor in statistics and econometrics.
The second myth: artificial intelligence and deep learning - the latest achievements of modern scienceThe opinion that computers “thinking like a person” will accompany us in the near future is directly connected with the idea that artificial intelligence, neural networks, and in-depth training are the exclusive property of modern science. After all, if we admit the thought that all this was invented decades ago (and robots by now did not take over the world), the “threshold of expectations” from scientific achievements in general and the speed of their development in particular will have to be seriously reduced.
Unfortunately, the media are trying to do everything possible to stir up interest in their materials, and are very selective in choosing topics that, in the opinion of editors, will arouse interest among readers. As a result, the achievements they describe and their prospects turn out to be much more impressive than real discoveries, and some of the information is simply “carefully lowered” in order not to reduce the intensity of passions.
Much of what is now being presented “under the sauce” of artificial intelligence is simply processed information about neural networks that have been known to mankind since the 80s.
And in the eighties, everyone repeated what was known in the 1960s. It feels like every 20 years there is a wave of interest in the same topics. In the current wave, the main idea is the convolutional neural network, which was already spoken about twenty years ago.
- Michael Jordan
The third myth: an artificial neural network consists of the same elements as the "real"In fact, specialists involved in the development of computing systems operate on neurobiological terms and formulations much more boldly than many neuroscientists. Interest in the work of the brain and the device of human intelligence has become a breeding ground for the development of such a theory as “neural realism”.
In artificial intelligence systems, there are no spikes or dendrites, moreover, the principles of their work are far from not only the work of the brain, but also from the notorious “neural realism”. In fact, there is nothing “neural” in neural networks.
Moreover, the idea of ​​“neural realism”, based on the assimilation of the work of artificial intelligence systems to the work of the brain, according to Jordan, does not hold water. According to him, it was not “neural realism” that led to progress in the field of artificial intelligence, but the use of principles that were completely at odds with the way the human brain works.
As an example, Jordan cites a popular depth learning algorithm based on the “reverse transmission of a learning error.” Its principle of operation (namely, signal transmission in the opposite direction) is clearly contrary to the way the human brain works.
Myth Four: Scientists understand well how "human" intelligence works.And this is again far from the truth. According to the same Michael Jordan, the deep principles of the brain’s work do not just remain an unsolved problem of neurobiology - in this area scientists have been separated from the solution of the problem for decades. Attempts to create a working imitation of the brain also do not bring researchers closer to understanding how human intelligence works.
It's just an architecture, created in the hope that someday people will create algorithms suitable for it. But there is nothing that reinforces this hope. I think that hope is based on the belief that if you build something like a brain, you will immediately understand what it can do.
- Michael Jordan
John McCarthy, in turn, stressed: the problem is not only to create a system in the image and likeness of human intelligence, but that scientists themselves do not agree on what he (the intellect) is and what specific processes are responsible.
Scientists are trying to answer this question in different ways. In his
book , Neural Networks and In-Depth Learning, Michael Nilsen cites several points of view. For example, from the point of view of connectomics, our intellect and its work are explained by how many neurons and glial cells our brain contains, and how many connections are observed between them.
Given that there are about 100 billion neurons in our brain, 100 billion glial cells and 100 trillion connections between neurons, it is extremely unlikely to say that we can “recreate” this architecture and make it work in the near future.
But molecular biologists studying the human genome and its differences from close relatives of people in the evolutionary chain, give more encouraging predictions: it turns out that the human genome differs from the chimpanzee genome by 125 million base pairs. The figure is big, but not infinitely huge, which gives Nielsen reason to hope that based on these data a group of scientists will be able to create if not a “working prototype”, then at least no matter how adequate an “genetic description” of the human brain or rather basic principles, underlying his work.
It should be said that Nielsen adheres to “generally accepted human chauvinism” and believes that the significant principles determining the work of human intelligence lie in the same 125 million base pairs, and not in the remaining 96% of the genome, which coincide in humans and chimpanzees.
So will we be able to create artificial intelligence, equal in human capabilities? Will it be possible in the foreseeable future to understand how exactly our own brain works? Michael Nilsen believes that it is quite possible - if you arm yourself with faith in a bright future and that many things in nature work according to simpler laws than it seems at first glance.
But Michael Jordan gives advice that is closer to the practical work of researchers: not to succumb to the provocations of journalists and not to seek "revolutionary" solutions. In his opinion, being tied to the human intellect as the starting point and the ultimate goal of their research, scientists working on the problem of artificial intelligence limit themselves too much: interesting solutions in this area may lie in directions that are in no way connected with how our brain works ( and how we see his device).
PS We in
1cloud consider a variety of topics in our blog on Habré - a couple of examples:
And we talk about our own
cloud service :