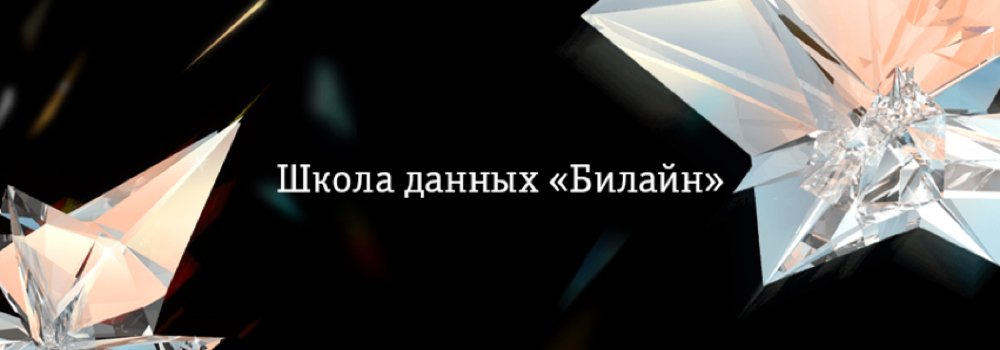
Hi, Habr!
So, we launched the third year
of the Beeline Data School . A detailed report on the lessons from one of the participants can be read
here .
')
We will also post reports on the School’s work on the School’s official
Facebook page . In the same place, we will answer questions that can also be sent to dataschool@beeline.digital.
We type the 4th course, which starts from April 4. Record, as always, on the
page of the School .
However, this post is not only about this. So far in the School of Data we have taught analysts, taught how to use machine learning methods to solve practical problems. However, practically any practical task begins with a business need and a business statement.
We will not say now that at the dawn of big data, it was believed that the main insights and applications of analytics come more from data. This is certainly there, but in our practice it occurs at a ratio of 80 to 20, where 80 percent of all tasks for the analyst or even more are born from business.
However, how does a business generate these tasks if it, the business, does not understand data analytics? Yes, very simple. In our company, we spent some time explaining to the business the possibilities of data analytics, and now various departments are flooding us with orders, inventing new applications for these tools.
On the other hand, data and their analytics, once the prerogatives of exceptionally large companies, now penetrate everywhere, and even startups today often think about what to do with this data.
How to use data to personalize offers and create an individual product, how to deal with outflows or minimize risks of non-payment, how to use analytics to choose the right place for the store, how to segment company employees to select motivational schemes or predict dismissals, how to effectively recommend products like profile customers, how to work with programmatic advertising.
All these issues are increasingly rising in different areas of business along with others. For example, a company has a lot of data, for example, because it works with telematic device data: what to do with this data, how to make money with it? Or how to make a data-driven company so that all decisions are made based on the data: where to start?
Previously, everyone was chasing after cases: successful applications of analytics to solve business problems. But, the fact is that each business is quite unique and what works for some may not work for others, but on the other hand, the success of any case lies in the details, and nobody will tell you these very details and, again, business to business just these details may differ significantly.
Therefore, you will have to reinvent all successful applications of analytics in your business. And in order to successfully do this you need to know about the possibilities and limitations of this analytics, and you, as business owners, and employees of your departments, since most of the applications will be generated by them, as close as possible to business objectives.
At the same time, it is important to understand not only the applications of analytics, but also how this analyst works, as well as in the formulation of the problem. How long does it take to build a model, what data is needed, what accuracy is achievable, what accuracy is required taking into account business sense?
Consider this simple example: you predict a call to a call center, or fraud, or another rare event. Suppose that you need to receive a list of candidates for this event once a day, in the case of calls for early contact with your customers, and in the case of fraud to prevent it.
Suppose your analysts have made you a model with a probability of a false positive classification of a call or fraud 10%. This means that with a probability of 10% a client who was not going to call the call center would be classified as going, and a client who did not commit fraud as a framer.
At the same time, let us assume that the probability of correct classification of those who call the call center or make fraud is 87%.
At first glance, the model is not bad. You save a lot of money by reducing the number of calls to the call center or fraud in 87% of cases. At the same time, you classify falsely those who were not going to call or make fraud only in 10% of cases.
However, it can be remembered that a call to a call center per day is relatively the entire customer base, although it is quite a rare event, however, like fraud, in a normal situation. Suppose that these actions somehow relate to 1% of all customers, which is pretty close to the truth.
Meanwhile, our error of 10% should be imposed on 99% of the entire customer base. Suppose you have 1 million customers. Then, it turns out that you contact on the day in order to prevent a call to the call center or deny service on the basis of suspicion of 1 million * 99% * 10% = 99,000 customers. And if your base is 10 million customers? And if 100?
It turns out that such accuracy does not suit you at all and you would prefer to sacrifice the accuracy of guessing those who actually call in order to underestimate as much as possible the errors of false inclusion in the forecast of those who would not call. Since these two quantities are interrelated.
Consider another example. You want analysts to build you an outflow model. First of all, you will need to agree on what is considered an outflow. In most cases, customers obviously do not inform the company that they have left; they simply stop using the services. Accordingly, if they did not use your services for 2 weeks, is this an outflow? And a month? And two? It is necessary to discuss in advance, because what you define as a target variable, your model will predict.
And at what point should the model predict the outflow drift? At the moment when the client has not used the service for a month already? Or at the beginning of this period, or maybe in advance, so that you have time to contact with the client and try to keep him?
These and many other subtleties determine the success or failure of data analytics in each specific case.
There are also more global issues: where in the company's structure to place the unit for working with analytics, should it be a unit or can it be scattered across different functions, what should be the organizational structure of the unit for its work to be most effective, what processes are needed, what roles .
In order to answer you all these and other similar questions, we have made a data analytics course for managers,
Data-MBA .
In this course, we talk about all the basic data analysis tools, as well as their application in different areas of business using specific case studies, the intricacies associated with this, capabilities and limitations, processes, technologies and many other things necessary for successful using data analytics to solve business problems.
The first lesson is February 16, recording until February 12. No special pre-training is required, we will tell everything in the classroom. Sign up
here .