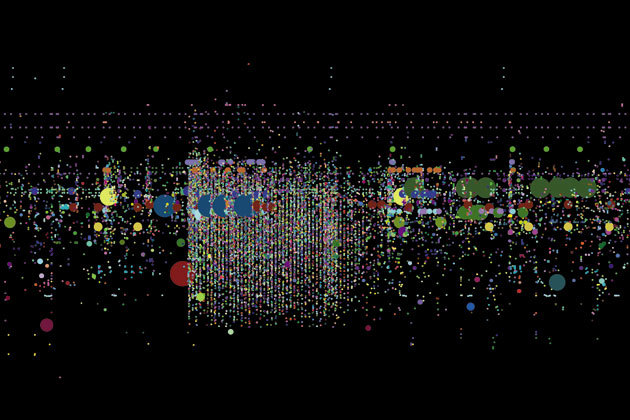
Nowadays, the situation on the stock markets is increasingly influenced by technology and special trading algorithms. As automation grows, social relations between bidders change.
The analysis of the sociology of financial algorithms is the subject of a
study by the professor at the Graduate School of Social Sciences, Edinburgh Donald McKenzie. We present to you the most interesting thoughts of this work - the first part deals with the prerequisites for the emergence of HFT trading and the classification of its application schemes.
')
Note: The material presented below belongs to the category of “large-scale pre-holiday reading” - this must be taken into account when taking time to study it. Introduction
With the growth of trade automation and the number of trading algorithms, social relations between market players are beginning to change. Neil Johnson and his colleagues (Neil Johnson, 2012) believe that there was a transition from “mixed interaction of people and machines” to “pure machine ecology”, in which “prices are dictated by machines”. In their opinion, falls or price hikes, lasting, for example, 25 milliseconds, are caused by the operation of algorithms.
All these are just assumptions, since it is not easy to determine which transaction was made by man and which by algorithm. [1] However, there is evidence that some — but not all — financial markets operate in one of three ways:
- The players in the market are people, and the market is a system of direct interaction between people.
- The market is automated (supply meets demand through an electronic system), but players still use people who use computers to interact with each other.
- The market is automated, and its players are algorithms.
The social aspects of e-commerce are devoted to the work of researchers such as Caitlin Zalum (Caitlin Zaloom, 2006), Alex Preda (Alex Preda, 2009, 2013), Sergey Saavedra and his colleagues (Serguei Saavedra, 2011). Most scientific papers on this topic deal with the second scheme or interaction between people in the third. Donald MacKenzie (Donald MacKenzie, 2006) and Martha Poon (Martha Poon, 2007, 2009) in their writings describe the general principles of the algorithms, but their work also deals mainly with direct communication between people. The same applies to the consideration of Ken Hillis, Michael Petit and Kylie Jarrett of the principles of the operation of PageRank algorithm in Google.
The third scheme helps describe the actor-network theory developed by Bruno Latour and Michel Collon. Within this theory, the concept of “agent” is used to describe the algorithms [eng. actor]. Although this term is considered controversial, it’s still silly to assume that the algorithms are just executing human orders. The unpredictability of algorithms often caused known catastrophes. Suffice it to recall the financial collapse of Knight Capital, which occurred on August 1, 2012, when the company lost $ 440 million in 45 minutes. [2]
However, in order to consider automatic trading from the point of view of sociology, it is necessary to move from the general provisions of actor-network theory to more specific issues. In their work on the relationship between “markets” and “technologies”, Karin Knorr Cetina (Karin Knorr Cetina) and Alex Preda (2007) raise the challenge that all economic systems face: how to organize economic interaction in the market. They offer two solutions that resemble the first two of the three schemes described above:
- "Network structure", in which "the interaction is carried out by transferring data through the channels connecting the network nodes." As an example of such a “channel”, Alex Preda (2006) cites the exchange ticker, which has radically changed the structure of stock markets.
- “Stream structure” or “instant interaction”, based on “collecting and simultaneously presenting data to a large number of people”, in particular, on computer screens. In this structure, “various operations, analytics and data presentation are performed in one place, which should guide the players and limit how they will react.”
At the end of the 20th century, “instant interaction” [eng. scopic coordination] has become the main principle of the organization of the markets. According to Knorr Cetina (2013), high-frequency trading, or HFT-trading, at least partially, interferes with this interaction. HFT trading is conducted at such a high speed that a person is not able to keep track of it. As will be shown later, the study of this type of trade allows you to take a different look at certain “channels” of communication. In addition, in the process of studying HFT trading, many principles can be found on the basis of which economic interaction is built on the current market, including the two methods described above.
If a person is not able to keep track of what is happening on the market, this means that HFT traders can profitably use their advantage in speed. Michael Lewis's Flash Boys book (Michael Lewis, 2014), in which HFT traders are presented in the most unfavorable light, became a bestseller, and Columbia Pictures immediately bought out the rights to the film. [3] The author of the book doubts the “legitimacy” of the actions of HFT-traders, since their actions are not consistent with the principles of trading in financial markets. Since the stock markets became legal institutions and the concepts of “risky game” and “investing” were differentiated, finance began to be considered an area in which “success is the result of careful and continuous research” (Preda, 2009).
The idea of ​​creating HFT-traders in the market, driven by machines, scares not only young investors and the general public, but also its players themselves. In some markets, this leads to the so-called “lining up borders” [eng. the boundary work] described by Thomas Jarin (Thomas Gieryn, 1999). Some trading platforms try to distinguish “bad” algorithms from “good” ones and to separate the range of “legitimate” actions of HFT algorithms from all other types of HFT trading. Therefore, a company whose trading algorithms bring too much profit can be excluded from the trading floor and branded as “predatory”.
It is easiest for HFT algorithms to conduct operations on trading floors, and it is rather difficult for them - although in principle it is possible to trade outside the electronic system. The historical process of forming electronic trading platforms was most accurately described by Fabian Munez (Fabian Muniesa, 2011). He explains the automation of the market as a process of some kind of “deployment” or “disclosure”, as Gilles Deleuze calls it (Gilles Deleuze, 1990). Muneza describes automation as the “creative process that generates and encourages action.” However, past events - sometimes reflecting the solution of specific tasks in a given region - lead to a limitation of the available automation capabilities. Therefore, the sociology of HFT and other trade algorithms must be considered from the point of view of its development history. For this, it is necessary to study not only modern methods, but also decisions made in the past and events that have influenced them.
Such a “historical” sociology, according to Neil Fligstein (Neil Fligstein, 1996, 2001), should also be political. Socio-technical “market structures” are focused on “mitigating the effects of competition with other firms”. The existing players, in turn, were either against the automation of financial markets and the emergence of HFT trading, or tried to automate them in such a way as to minimize the negative consequences. As shown below, HFT trading has been shaped by government agencies such as the Securities and Exchange Commission.
As a basis for the historical sociology of HFT algorithms, Andrew Abbott (2005) proposes to take the idea of ​​“connected ecologies” [eng. linked ecologies]. [4] Abbott's “Ecology” is an area represented as “an interaction of many components that limit one another and compete with one another.” HFT-algorithms directly interact with other algorithms and implicitly interact with each other. HFT companies are “competing with each other” in the fight for the so-called “locations” [eng. locations] - something that “can be managed”: in our case, for a favorable position in the market and sometimes for recognizing the legitimacy of our actions.
It is important to note that HFT trading is a related ecology, that is, “a set of interacting ecologies”. As mentioned above, HFT trading is closely related to the ecology of trading platforms (it is also appropriate to use the concept of “ecology”: various sites tend to fight among themselves both for a favorable position in the market and for the recognition of the legitimacy of their actions) and the environment that regulate bodies (also competing with each other, at least in the US). At first glance, government regulation has nothing to do with HFT trading or trading platforms, but in reality this is not the case. For example, the Securities and Exchange Commission (SEC) is the most influential regulatory body in the US stock market. In amendments to the Securities Act of 1975, the US Congress ordered the SEC to organize trading platforms so that competition in the market increased. The real goal of the SEC was to undermine the authority of the New York Stock Exchange (NYSE) and its "experts" (market makers). SEC achieved its goal thanks to the seemingly unrelated development of trading platforms, which, in turn, accelerated the development of HFT trading.
Thus, the development of HFT trading in the US stock market did not depend on the social structure or on technological progress. Most likely, it originated as one of the components of the so-called “ligaments” [Eng. hinge] by Abbott (2005) - “strategies that work” in several ecologies. "Link" here is defined as a series of phenomena that linked processes from different ecologies - HFT trading, trading platforms and state regulation. Such a linked historical process led to the emergence of HFT trading in the US securities market.
It is necessary to clarify two points that relate to “related ecologies”. First, in accordance with actor-network theory, this article does not try to separate "social" phenomena from "technical" ones. Each of the three described ecologies is a sociotechnical area in which people write algorithms, and those, in turn, begin to replace a person and sometimes even ruin his plans. Secondly, the “topology” of the interaction of these three ecologies has not historically been fixed. For example, HFT-trading originated in a certain group of trading platforms and obeyed their laws. These laws HFT traders had to accept as a "social fact." However, as its development HFT-trading partially covered the activities of trading platforms that were already formed on its basis.
Thus, in this article the sociology of algorithms is considered as a) historical, in particular, revealing dependence on previous events; b) "ecological" by Abbott; and c) “zeliterskaya” [eng. Zelizerian, after the famous sociologist and economist Viviana Zelitser - approx. transl.], studying the alignment of boundaries and attempts to distinguish between "bad" players and "bad" algorithms from "good". Then, after the story about the research methodology and the forms of interaction between the algorithms, the functioning of the HFT algorithms is considered in detail. After this, the historical process mentioned above is described in more detail. At the end of the article there are differences in the principles of operation of HFT algorithms in three different markets [approx. Perev .: they will be discussed in the second part of the article]. This is done in order to show how the functioning of these algorithms is influenced by historical processes, various related ecologies and the construction of boundaries. The article discusses the following three types of markets:
- "Light" US securities markets. [5] Trade at these sites directly depends on two factors. The first is the “connection” between the development of trading platforms and the development of HFT algorithms and state regulation. The second is the dependence of events on previous processes. The beginning of this development was the decision to organize economic cooperation, adopted in the late 1970s. However, as a result, a strong contradiction arose, which led to the need to use market sweep orders [Eng. intermarket sweep orders].
- "Dark" US securities markets. In these markets, all algorithms are divided according to the principle of "morality" ("good" and "bad"). Here, the competition between trading floors and their desire to recognize their legitimacy are closely intertwined. This leads to tight control of HFT-firms, some of which are excluded from the auction and declared “opportunistic”.
- Trading platforms FOREX. This type of market is necessary for comparison with the US securities market. Given the lack of analogs of the SEC commission and the historical “bundle”, as well as the presence of a special related ecology, the alignment of borders in the FORCES markets is simplified. In addition, their economic cooperation is not organized in the same way as in the case of securities trading. In the process of development of this type of markets, a contradiction also arises, which leads to the emergence of a new type of algorithmic activity - “additional verification” [Eng. last look].
Research methods
In an area like HFT trading, it is difficult to carry out both quantitative and qualitative research. The complexity of quantitative research lies in the fact that market data, with rare exceptions, does not indicate exactly who made the transaction - the person or the algorithm, and if the latter, then whether it was an HFT algorithm. [6] One group of economists was able to access data indicating a characteristic HFT trading style of trading. However, access to this data, which belongs to the Commodity Futures Trading Commission (CFTC), has already been closed, and articles based on it have been deleted. As a result, despite the fact that two consulting companies, Tabb and Aite, publish data on the volume of HFT trading on the market (see Table 1), these data are still based only on the opinions of experts with whom the companies cooperate.
Table 1 - Share of HFT trading on these markets in 2012. Source: Aite Group data; Arash Massoudi and Donald Mackenzie (2013)
Market | Share hft trading on the market |
---|
US stock market | 53% |
international market futures | 52% |
international market FOREX | 40% |
international market fixed income securities (bonds and similar liabilities) | 18% |
Qualitative research of HFT trading is not any easier: HFT companies are usually private and do not report openly about their activities, and often even try to hide it. Nevertheless, 43 experts - the founders of firms, their former and current employees - agreed to give an interview about the activities of HFT companies and the difficulties associated with it, and experienced specialists also about the history of HFT trading. Interview participants are conditionally designated in chronological order: starting with AA, who gave his interview in October 2010, and ending with an interview with BQ in April 2014.
The search for interview participants was conducted in different ways. At first I turned to open sources and searched for the names of the most active HFT firms from Chicago, New York, London and Amsterdam (the four most important settlements for HFT trading). If these sources indicated the names of decision makers and their phone numbers, I called at least one representative of each such company. Other search methods were more spontaneous: meetings with speakers at events dedicated to HFT trading, a survey of potential interview participants, to which analysts in the industry or previous interview participants referred, and just casual acquaintances.
Most of the interviewees were experienced HFT traders, but among the respondents were young players whom I found by chance or through other interview participants.
There were some difficulties during the survey. At times it was not easy to focus the interviewees on HFT trading. For example, I conducted interviews with two former traders from the Chicago Pit who settled in an HFT company, and I had to stop them every time they started talking about their pits. Some questions interfered with the course of the interview itself, since the participants could not or did not want to answer them.
However, during the conversation with the first participants I was able to identify a number of HFT trading strategies, widely known and often practiced in this area, and which became one of the topics of subsequent interviews. In addition, the first participants talked about some features of the work of HFT companies that are unknown to me (for example, conducting an “additional test” on FOREX), but known to all industry players. Initially, the study was not of a comparative nature, but in the course of the survey it became clear that there are a number of significant differences in different areas of trade (especially between trading in the securities market and FOREX market). Therefore, in subsequent interviews, more attention was paid to these differences. [7]
However, the study of ecologies associated with HFT trading was simplified for several reasons. For example, it was easier to ensure the confidentiality of interviews. In the case of stock trading, the historical data of large trading floors (NYSE and NASDAQ) and regulators is fairly easy to find in official documents, and by drawing on them you can get a more general picture. In addition, during the interview, I could focus on three types of trading platforms: those that the sources and participants of the interview referred to as key ones; those that could follow the work of HFT-algorithms and build boundaries between them; and FOREX sites, which can be compared with securities trading sites.
Interview participants who are not HFT trading experts are identified in the article as “interviewees”.
Despite the problems of obtaining data from the scientific literature on HFT-trading, the conclusions reached during the interview can be verified. To do this, they need to be compared with the assumption about the possibility of HFT algorithms to predict short-term price changes. This statement goes against the “efficient market hypothesis” (EMH), however, as will be shown later, the results of quantitative studies confirm the conclusions reached during the interview.
Engines for customer and seller information, execution algorithms and HFT algorithms
When it comes to automating the market, it is usually meant that the deals are made using a special engine [Eng. The matching engine is an engine that brings together buyers and sellers], and on its basis an e-book of applications is formed. Figure 1 shows a screenshot taken by one of the interview participants during the testing process of one of the execution algorithms, which will be described below. The screenshot shows the book of applications of several trading platforms where the loan saving company Astoria Financial was trading. Applications for the purchase of Astoria shares are listed on the left - for example, an application or applications for the purchase of 192 shares placed on the NASDAQ, at a price of $ 7.74 per share. Right, respectively, the application for sale.
Figure 1 - Applications for the purchase and sale of shares of Astoria Financial Corp. on the US marketplaces, noon October 21, 2011. Source: one of the interview participantsIn addition to the maintenance of the order book, the engine is looking for opposite pairs of orders for the purchase and sale. In the order book in Figure 2 there are no such pairs. But they will appear as soon as a person or algorithm makes an application for the purchase of shares at a price not lower than $ 7.75 or an application for the sale of shares at a price not higher than $ 7.74 (an order that can be executed instantly is called a “market” [eng. Marketable order ]). If the engine finds a pair of opposing orders, it makes a deal and sends confirmation to both parties. In contrast to the “manual” trade, there are no negotiations in electronic trading. In addition, in the stock market (but not always in the FOREX market) the whole process is carried out anonymously.
Figure 2 - Applications for the purchase and sale of shares of Astoria Financial Corp. on the NASDAQ, noon October 21, 2011. Source: Figure 1In addition to traditional traders, two classes of trading algorithms interact with the engines described above. The first class is execution algorithms [eng. execution algorithms]. They are used by large investors or brokers acting on their behalf to buy and sell large blocks of shares or other financial instruments. Execution algorithms break these packages into small parts and put them on the market in such a way as to reduce the “market influence”. The second class of trading algorithms is proprietary trading algorithms, a special case of which are HFT algorithms, described in detail in the next section. [8] Unlike execution algorithms, proprietary trading algorithms do not trade in a specific volume of financial instruments. In particular, HFT algorithms are almost always programmed to extract profits without placing risky positions, when the volume of purchase orders far exceeds the volume of sales orders, or vice versa.
Exposing and canceling applications, HFT-algorithms and execution algorithms directly interact with the engines, which reduce buyers and sellers, and with the help of these engines, they implicitly interact with each other. At the same time, developers of execution algorithms try to hide the actions of their algorithms from professional traders and other algorithms. This is explained by the fact that the proprietary algorithm can track how the execution algorithm tries to acquire a large block of shares, then buy out these shares before it and profitably resell them to the same algorithm. Some algorithms are programmed to search for moments of “price momentum” [eng. price momentum], which also bring a good profit. In this case, the algorithm provokes a sharp movement in the market and “profits” on other algorithms that follow the general trend.
How does HFT trading work
It is a mistake to suppose that HFT algorithms most often use risky methods of making profit. Judging by the name, high-frequency trading involves maintaining a large volume of trades, and complex and risky strategies are unlikely to do so. The essence of some HFT-strategies are only in the search for financial instruments that can be bought cheaper on one trading platform and sell at a higher price on another. However, such “opportunities for arbitration”, as market players call them, appear infrequently and do not bring enough profit to conduct a full-fledged business. The main activity of HFT-traders is the use of strategies with a wide scope, which allow to predict price changes in a very short period of time.
To understand these strategies, consider two of them. They are used by all HFT firms in which participants of our interviews work or have worked. The first strategy is based on the “dynamics of the financial instrument in the order book” [eng. order-book dynamics]. Simply put, as AH said, it is the ability of an algorithm to determine, “whether the size of the bid exceeds [Eng. bid - buy order] size of the offer [eng. offer - application for sale] ". Take a look at the order book in Figure 2: the best bid (having the highest price) consists of 192 shares, and the best offer (having the lowest price) - out of 488 shares. The size of the best offer is larger than the size of the best bid. This means that the shares of this company in the market want to sell more than they buy, and, most likely, “the price for it will decrease by one point”. (The fact that this method helps to make forecasts and that HFT firms use it is confirmed in the financial and economic literature). [9]
In addition, valuable information from the “dynamics in the book of applications” can be obtained using the so-called “tape” [Eng. time and sales]. It provides information on transactions conducted with this financial instrument, including data on the time and price of the transaction. It should be remembered that more complex HFT algorithms will take into account the dynamics of this financial instrument in all books of orders both in the US stock market and in the FOREX market. In addition, HFT algorithms can take into account what the respondent AN calls “book pressure”, i.e. the sizes of bids located below the best bid, and the sizes of offers located above the best offer. However, in this situation, some traders and algorithms may use a "spoofing" strategy [Eng. spoofing - fraudulent action] - submit bids that will create the impression of excess demand or supply in the market. For example, Figure 2 shows large purchase orders for $ 7.72 and $ 7.71, and, until prices have dropped, these orders can be canceled before they are executed. HFT algorithms can avoid this problem if they take into account only those applications that have been in the book for quite a long time, since fraudsters usually quickly cancel their applications.
The second widely used HFT strategy takes into account the price movements of financial instruments that affect the movement of this instrument. As an example, the so-called "futures log" [Eng. futures lag]. Its essence lies in the fact that, based on the price movement for index futures, HFT algorithms can fairly accurately predict how the value of traded securities or shares of exchange-traded investment funds will change [Eng. exchange traded funds, ETF]. [ten]
Another more specialized source of information - and it is used by all companies without exception - is the news of the world economy or individual companies. Today they are distributed in such a form that they can be considered a regular computer. As a result, an algorithm that reacts to these news even before they affect prices, can bring a substantial profit to its owner. As a rule, HFT-companies' algorithms collect information from several sources at once, taking into account the dynamics of the order book, data from related financial instruments, and market news. At the exit, such algorithms usually give the theoretical value of a stock or other tool that can be expected in the near future - from a split second to a couple of minutes. This theoretical value, according to the respondent BF, is easiest to present as a variable depending on such factors as the difference between bid and offer, prices of related tools, etc. Algorithms of other companies collect data in other ways. For example, AN talks about the automatic “polling” system, where the number of “votes” for various factors changed depending on market conditions.
In the stock market, the difference between the theoretical and actual value of shares is often less than a cent. “If the algorithm considers that the price should be equal to $ 2,396, and the book contains bids for the purchase of shares at $ 2.40, the algorithm will sell this share at $ 2.40,” says BJ. “Sometimes I lose money on such deals, but on average I win 55% of the time, and that's enough for me.”
However, there is a significant difference in how HFT-algorithms make their transactions. Some algorithms can act “passively” or, as market players say, “add liquidity”: they submit an application with such a price that it cannot be executed immediately. Other HFT algorithms can act "aggressively" or "take liquidity": they can send a market request that will be executed immediately after it reaches the engine. For example, in the order book in Figure 2, an order to sell shares at $ 7.74 is a market, “aggressive” or “taking away liquidity”; an application to sell shares at $ 7.75 is non-market, “passive” or “adding liquidity”. [eleven]
As can be seen from the example, adding liquidity has a number of economic advantages. With other things being equal, the liquidity-adding order is executed at a more favorable price compared to the liquidity claim. In addition, for the increase in liquidity, trading platforms pay "rebates" [eng. rebate] - a small fee of about 0.3 cents per share - a company that submitted a “passive” application. Such companies sometimes call themselves “electronic market makers”, as they, like ordinary traders, can act both as a seller and as a buyer.
The main task of the HFT algorithm that adds liquidity is to place buy orders at a price equal to or close to the best bid ($ 7.74 from the example in Figure 2) and sell orders at a price equal to or close to the best offer ($ 7, 75), waiting until they are executed. Such an algorithm wins on the "spread" [eng. spread] between the bid and the offer (usually it is one cent, as in the example above) and receives a double rebate, giving a total of 1.6 cents for the shares sold and bought. But there may be difficulties: when prices change, the algorithm that adds liquidity, you must constantly cancel current bids and put up new ones, as well as make predictions on how prices will change in the future. For example, if prices soar, the offers of such an algorithm “become obsolete”, and aggressive algorithms can execute them at a favorable price. «» «» , «» .
. , – ( ). , . , «» , .
HFT- , : , , , – . BE , « ».
, , , HFT-, , HFT- . - , HFT- , . [12] , HFT- , «» [. co-location – ]. , , . [13] HFT-, , «» . «» . – , -.
HFT- « ». , , , « » [. order gateway] – , «» ( ). , (Scott Patterson, Jenny Strasburg, Liam Pleven, 2013) Wall Street Journal , «» CME 1-10 . : , , CME.
, HFT- . , $1. . , 53% . , 0,06 . , 0,05 .
, , . HFT- - , (2014) Knight Capital. [14]
. , . HFT- , . , HFT- - – 5 2013 – . [15]
«»: HFT-,
HFT- : HFT-, ( , [. electronic communication system, ECN], 1990- ) . , 1970- , .
. , ( , (FDIC), (OOC), (OTS), (CFPB), CFTC SEC) (, -) . [16] SEC «» , NYSE (NASD), NASDAQ. [17] , . SEC, , .
SEC 1934 , -. , . ( - . , . , ). [18]
SEC [ 1970- ] «» , . NYSE NASDAQ , . SEC , , . 1975 SEC – « ». [19]
, . . « » [. Composite limit-order book]. , , , « », « », (Joel Seligman, 1982). , «» . , , . [. Intermarket trading system, ITS] 1978 .
ITS NYSE, (NASDAQ 2000 ). [. Consolidated quotation system], 1978 .
, . «» , , «». [. trade through], ITS, « ». – 2002 , 30 – , . , ( « » ).
, , SEC «» . , . Island, 1995 . Island , « SOES» (SOES – NASDAQ, «» , , , «» NASDAQ). Island «» « » . , . Island, , , ITCH. , OUCH, . – – 1/8 1/10 , Island 1/256 , « SOES» . , Island – , .
Island ( ) - NASDAQ . [20] , , -.
, Island , «» . Island , , HFT-, – . HFT- : Island . BE SuperBot NYSE. «», . Island : AG1, , OUCH , . «» HFT-, .
Island ECN- , SEC , – . «» , . ECN- 1997 [. Order-handling rules]. - NASDAQ , NASDAQ. , Island HFT- .
HFT-, ECN- SEC «», . SEC HFT-, , SEC ( 2005 HFT- ). SEC Island, .
, SEC , «», . AQ, « » SEC Island . Island , , , « -». , «», , 1970- .
To be continued...Notes- The “algorithm” in the article means not just a set of instructions, which can be written in the form of a program, but this program itself.
- SEC 2013 , , , - . , 1 , , . Knight Capital , , , .
- IEX – , «» «» ( «- »). IEX, , ( ) - ( ). HFT- .
- , «», «», , « » «» , . , «».
- , «» , ; «» .
- NASDAQ 120 , 2008-2009 2010 . - , HH, NN, HN NH. , HN, , HFT- (H) , , HFT- (N). , .
- - HFT- – . . (CME). , « », .
- . HFT-, , – . , . , , , , . HFT- .
- NASDAQ, 6, , (Jonathan Brogaard, Terrence Hendershott, Ryan Riordan, 2013) , HFT-.
- Index futures are a derivative financial instrument whose value depends on the corresponding stock index, for example, S & P 500 or NASDAQ 100. ETF stocks are securities whose value also depends on the total stock price affecting this index.
- In the future, the concept of “adding liquidity” instead of “passive” is more often used, as there can be confusion: HFT algorithms that present “passive” applications often operate very actively.
- Absolute speed is also important, for example, in the process of changing market conditions, when the “passive” algorithm needs to reduce risks by quickly changing orders.
- Interview participants also reported that in some data centers you can pay even more and place your servers in the part of the building where there are engines for the attention of players.
- Brogaard, Hendershot and Riordan (2013), based on information on rebates and data from note 6 for 2008-2009, estimate the average profit of HFT companies at about 0.4 cents per share. Based on the calculations in the Lewis book (2014), the same figure is 0.29 cents per share. Knight Capital's annual reports for 2009–2011 estimate the profitability of HFT companies in the range of 0.14–0.19 cents per share.
- According to James Angel, Larry Harris and Chester Spatt (James Angel, Larry Harris, Chester Spatt, 2013), in 2013, about 5 billion shares were traded daily in the US stock market, that is, 5 billion shares were sold and 5 billion bought shares. Based on the data of McKenzie (2012) and Table 1, the share of HFT companies, constituting 50-55% of all market players, accounted for a total of about 5 billion shares sold and bought daily. If we assume that the average profitability of a HFT company was 0.1 cents per share, then we can assume that HFT trading generated $ 5 million per day, or 1.25 billion per year. This is not so much compared to the profitability of any large bank.
- For example, the furious opponent of HFT trading is New York State Attorney General Eric Schneiderman.
- In 2007, the Financial Industry Regulatory Authority (FINRA) was formed as a result of the merger of the regulatory divisions of the NYSE and NASD.
- The full text of the speech of La Guardia.
- Amendments to the Securities Act.
- According to a survey conducted in 2000 by Bernstein & Co, "58% of respondents believe that the speed of execution of the application and the best price are equally important."