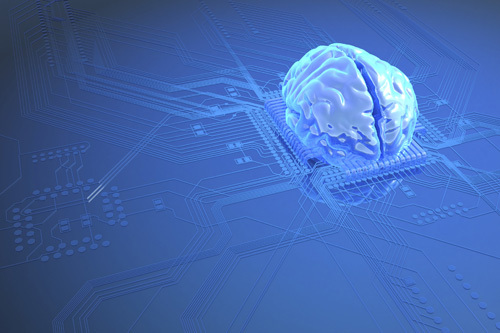
Hello! Last time we talked about how the technology of understanding and analyzing natural language texts ABBYY Compreno. Many people ask us - how much is already possible to develop technology and where, finally, are products based on Compreno. As promised, today's material is devoted to the products and exactly what business problems they are solving today.
Based on our technology, you can create a number of solutions for different types of tasks. But the focus of our attention today is the corporate market, companies that need to receive significant information from data sets in a short time. This direction is promising for us both from the point of view of the demand for such technologies by customers, and from the point of view of the earliest return of our investments in technology.
Immediately, we note that solutions based on Compreno technology are application or technological modules that are embedded in any solutions, adding opportunities to them.
For example, Compreno-based solutions can be used with ABBYY FlexiCapture streaming technology.
')
Documents of any organization contain important business information, for example, persons, organizations, dates, events and other material facts. With the help of our technology, you can highlight specific objects in the text and establish links between them. For this task, we made the ABBYY InfoExtractor SDK.
For example, ABBYY InfoExtractor SDK can automatically solve such an urgent task for many organizations as the distribution of payments by items of payments. The field “Purpose of payment” in the invoice describes in free form, from whom and for what the payment was received, as well as its period. Accordingly, this information should be reflected in the accounting or billing system, which takes a lot of time from the accounting staff. The ABBYY InfoExtractor SDK solution "understands" the meaning of what is written in the invoice, and then extracts the necessary data: the contract number, the paying organization, the amount, VAT, etc. Then you can transfer this data to the ERP system or billing system for accounting and receivables debt. Thus, the final decision can automatically compare the extracted data with the base of contracts and “tie” orders to the payment document, compare the values ​​of VAT in the purpose of the payment with the VAT specified in the contract.
By automating the extraction of data about the borrower from the materials provided to the bank, ABBYY InfoExtractor SDK also allows, for example, to optimize the risk assessment process when issuing loans. And this is very important for banks. Banking professionals need to receive full and high-quality information about borrowers (individuals and legal entities) in a timely manner to make a decision on granting a loan, as well as to respond quickly to financial problems of clients.
Solutions that integrate the ABBYY InfoExtractor SDK can:
• Analyze information about a potential borrower to assess its solvency, as well as about persons and organizations associated with it (to assess additional risks or sources of funding). For a complete picture of the borrower, information from various bank information systems — electronic customer files, ABS, etc., and external resources — can be analyzed from the Supreme Arbitration Court website, bailiffs, courts of general jurisdiction, media and social networks.
• Check for compliance with the same type of data from various sources (this allows you to identify the risks of falsification of documents).
• Analyze the state of the collateral: cost, location, facts of encumbrance or arrest, and other factors;
Thus, you can create a solution that will track important information about the activities of the borrower.
How does ABBYY InfoExtractor SDK solve this problem?
The solution structures the text, highlighting the entities (dates, objects, persons, etc.) and their properties. After extracting all the entities, the solution extracts all the necessary data from them and builds connections between them. Based on these relationships, a potential borrower’s card is constructed with a consolidated risk profile throughout the entire court decision base:
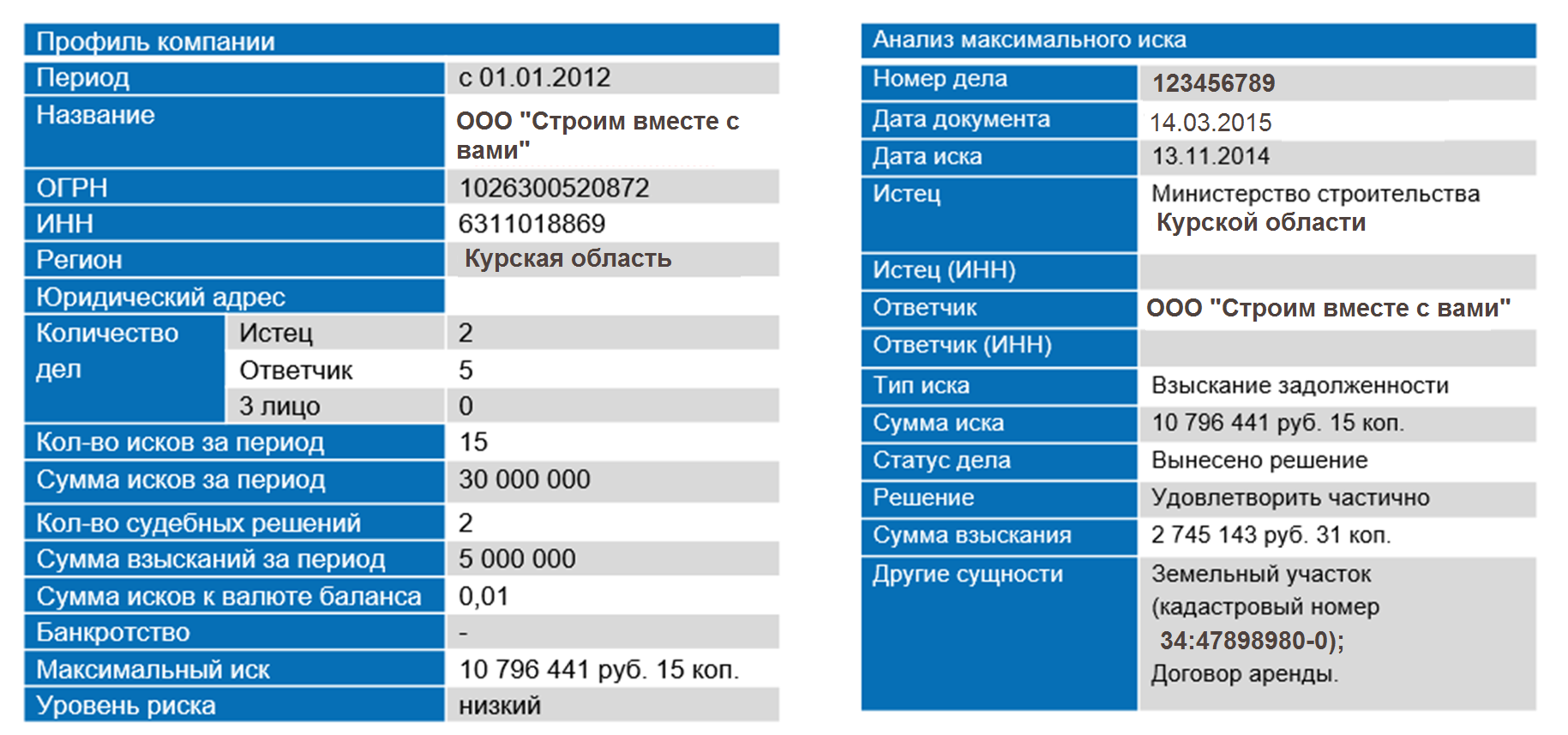
Another important task facing banks is the monitoring of transactions to counter the financing of terrorism and the laundering of proceeds from crime. At the request of Russian legislation, banks are obliged to quickly establish the economic meaning of operations and transactions. If there are suspicions that the operation is aimed at financing terrorist activities, banks should promptly send information about the violation to the authorized bodies.
Within the framework of this task, bank employees need to analyze a large flow of unstructured documents - contracts in order to enter data on payers, amounts, subject of the agreement, etc. into the Bank's IS. Regulatory bodies may request access to this information at any time, and banks need to provide it very promptly, while avoiding data errors. ABBYY InfoExtractor SDK allows you to perform this task automatically: the decision determines the type of contract, understands what data you need to extract from the text of the document. It is also possible to extract complex links when two or more objects of the same type (for example, real estate objects) with their attributes (also of the same type, for example, area, price, etc.) are scattered throughout the text of the document. After processing, all this data is exported to the target IC.
Also ABBYY InfoExtractor SDK can help you quickly handle requests from government agencies that come to credit organizations. You can create a solution with an integrated InfoExtractor module that will analyze information, automatically create request cards and provide quick access to this data. The availability of complete information will allow you to control the timing of the consideration of requests and comply with the requirements of the writ of execution. In addition, significantly reduced time for processing documents.
Now a few words about the implementation of ABBYY InfoExtractor. A couple - because clients, to our regret, are not yet ready to disclose publicly the details of the projects. With the help of ABBYY InfoExtractor, one of the largest Russian oil companies solves the problem of generating analytical reports and searching for duplicate documents in the corporate information system.
A large energy company uses the integration solution ABBYY FlexiCapture + InfoExtractor to distribute payments in billing systems. In payment documents (see illustration below) there is a column “Purpose of Payment” - it contains information allowing to correlate a payment with a specific contract, client, obligation. “Understanding” the entities identified in this field, as well as the details associated with them, simplifies the further processing of the document and speeds up important business processes for the company.
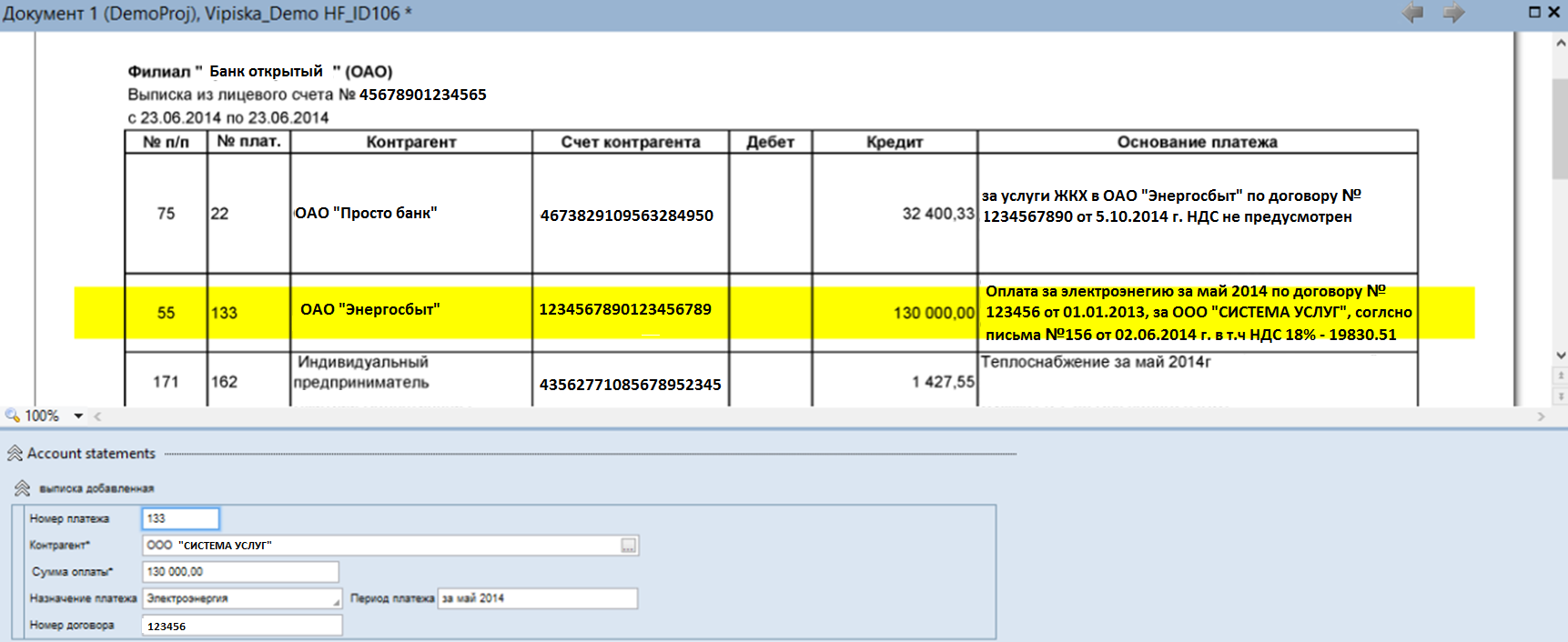
The main task of ABBYY Smart Classifier is to classify texts and documents by content. I must say, in our products before was a tool for the classification of documents. In ABBYY FineReader Engine, ABBYY FlexiCapture Engine and ABBYY FlexiCapture, the classifier separates documents both in appearance and in content (we
wrote about this in our blog earlier). However, the principle of the ABBYY Smart Classifier with the text is significantly different from how it was done in previous products.
ABBYY Smart Classifier “reads” the text in order to understand from the words, sentences and general context what this document is about and which category it belongs to. There are two types of classification in the solution: semantic - for Russian and English, textual - for 42 other languages. In this and in another case, first, the classifier is given a training set (for example, 10 categories of 50 texts each, which we ourselves have arranged in advance into these categories and assigned the name of each of them).
The classifier analyzes each category, identifies features from each text in the group: words, phrases, semantic classes that are determined based on our hierarchy (here we have a parser, which we wrote about
in the last article about Compreno ) and determines the frequency of these signs. After extracting features, the classifier builds a model that describes which features distinguish each of the categories from each other. Such a classifier is capable of resolving homonymy and analyzing the text more deeply; for a good result, it needs significantly fewer documents in the training set than the exclusively morphological one.
When, after learning, a new document arrives at the classification, the ABBYY Smart Classifier also extracts features from this document, tries on it a model for each of the categories, looks at which category the new text best matches. The output is a response similar to the following: “Document 1 belongs to category A by 60%, to category B by 35%, to category C by 5%). In the future, based on these results, developers can write their own rules that determine into which category the document should be written: simply by the highest percentage or when a certain threshold is exceeded (for example, if the classifier has determined that it belongs to category A by 80 or more percent) and so on. P. The decision accurately determines the category of the document based on the meaning of the text.
Applications for the ABBYY Smart Classifier may vary. The solution based on our technology allows us to automatically classify requests from users and, in particular, contact technical support (no matter whether they are submitted electronically or paper) in various workflow systems or mail systems and send them to the responsible department or specialist. The decision automatically determines the category of the incoming request, based on the analysis of its content (this decision, for example, is used by the State Duma in the Electronic Parliament project when classifying incoming citizens' appeals).
Further, on the basis of the category, again automatically, you can search for information about the incident in the knowledge base and immediately offer an existing answer to the user. Due to this automation, the processing time of incoming messages and the load on specialists is significantly reduced.
In addition, the solution using our classifier allows you to send an auto answer to the user without the involvement of a technical support specialist on the basis of the request. This post contains several links to the most relevant knowledge base articles that can solve the client problem.
Employees of many companies are faced daily with difficulties in finding the necessary documents - from contracts to letters. Often we only remember the essence of the document, but forgot its name or date. In this case, the search for the necessary information is delayed, and the work does not move at all. ABBYY Intelligent Search allows you to cope with this problem - a solution that can be embedded in the corporate system, and thus expand and complement its capabilities.
ABBYY Intelligent Search searches for documents by meaning, taking into account not only all forms of words, but also their meanings, semantic links between words and the context of usage. This is achieved through a ranking system and a complete semantic-syntactic analysis of the text, which we wrote about in a previous post. All this makes the solution more effective than traditional full-text search systems. Now a pilot project using Intelligent Search has been launched in T PLUS GROUPS.
How does ABBYY Intelligent Search work in the corporate information system?
Step one: we start indexing, that is, we do a full semantic-syntactic analysis of the entire collection of documents (for example, financial ones). So we get the "index" - information about each word and its meanings, about how this word is associated with others, as well as in what documents it is located. At the same stage, data is extracted. Metadata is automatically collected (author, creation date) and are found in the text of entity documents (names of organizations, persons, geoobjects, dates and sums of money). These metadata are saved along with information about the text of documents and can supplement documents (for example, in MS Share Point they are saved in the "tags" field), so that later it would be easier to work with them: to classify, sort or filter.
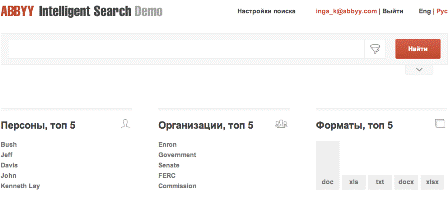
Step two: start the search. Since we have all the information indexed, we can specify the search parameters, specifying the desired value of words in the query and building filters. The user can choose whether he wants to find among documents, for example, the word “application” in the meaning of “software”, “request” or “use”. The user can specify a request in a natural language: for example, “the duties of the head of an enterprise” and receive documents that are close in meaning to the request. For example, a document in which there will be such a proposal: “The investigation was also conducted in relation to a number of other city officials of Duisburg, the manager of Lopavent, who was responsible for letting guests to the event, as well as the then head of the police’s operational activities.”
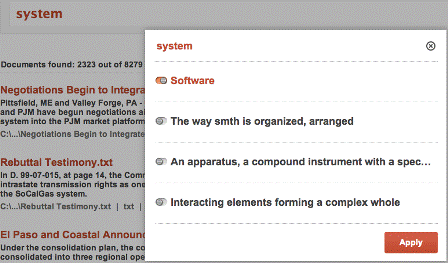
We can also search for information using “facets” - the most important and frequently occurring document attributes, formed on the basis of document metadata, entities extracted from it (i.e., the list is always specific) that can be used to filter the collection: mentioned persons, geographic objects and dates.
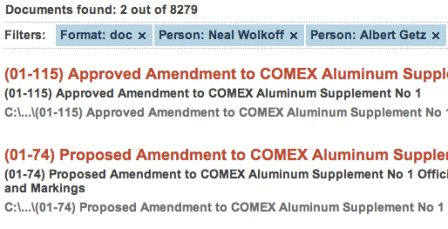
Step three: the user gets the results ranked using various attributes (that is, the documents are sorted in such a way that the most relevant are shown first).
That is, in fact, all that we wanted to tell about Compreno based solutions in the first approximation. Today, more than 25 large Russian companies in various industries have launched pilot projects using ABBYY InfoExtractor SDK, ABBYY Smart Classifier or ABBYY Intelligent Search. Most customers do not yet want to disclose cards, because we are talking about improving the competitiveness of their business, and in this sense they can be understood. We think in the foreseeable future we will still be able to tell you about the implementations with all the details, but for now - ask questions if you have them left.