By this time, you should have developed a good understanding of predictive modeling and its application to games. If not, see:
part 1 and
part 2 of this article. We have formed a good base, and in the final part we will try to predict forecasting and see what the future holds for a rapidly developing industry.
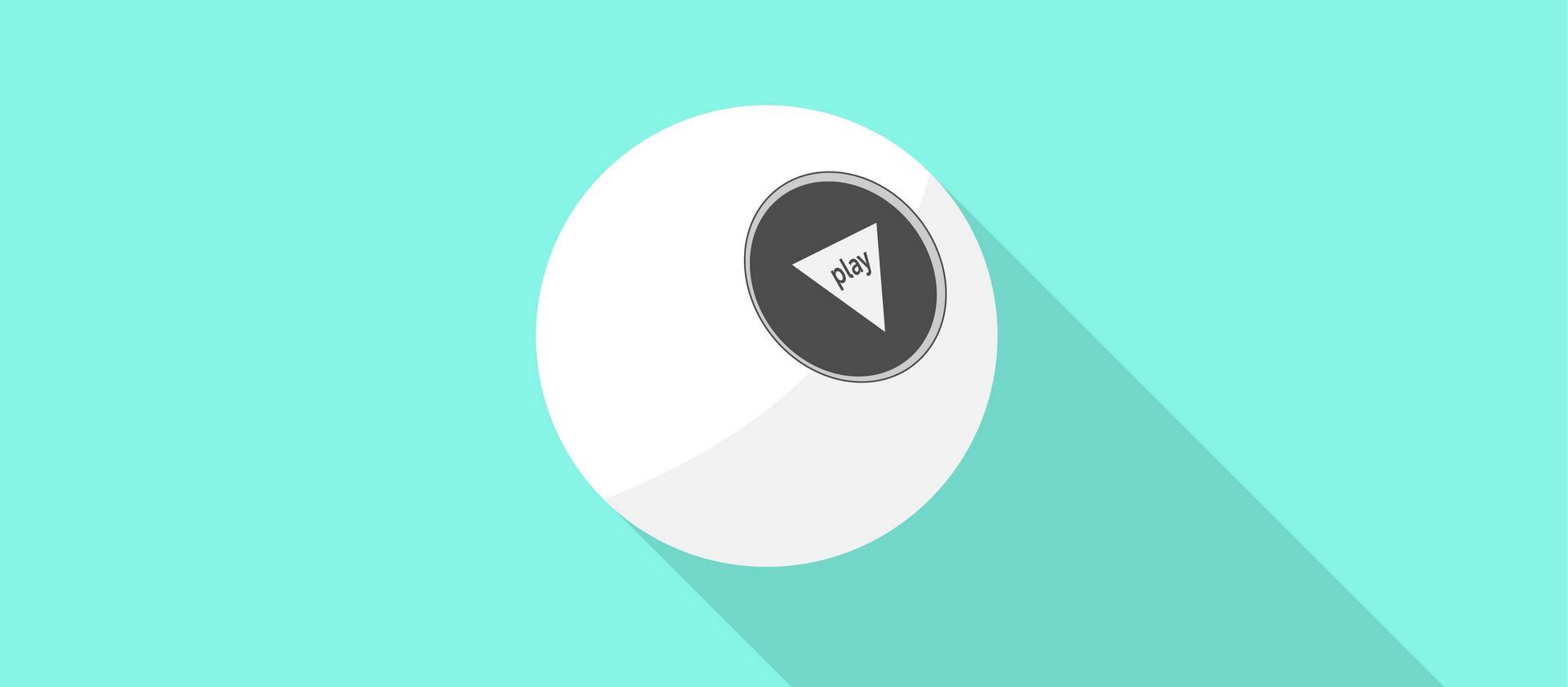
Even after everything has been said and done, the prediction of big data remains something unexplored. We are only in the early stages of unlocking the potential of this technology. Therefore, if you are in doubt, whether to apply prediction in your gaming company or not, I have great news for you: you have only stepped on the first rung, but you are already ahead of many of your competitors.
And the bad news is that it is so unexplored area that at the moment there are not enough qualified specialists who can correctly and fully interpret the data. And the data itself, which can work, not so much. To create prediction models, we need experienced users. In the social predictive analytics described in the previous part, their role is played by social "whales". These are users who have major social connections, and thus they are able to significantly influence other players. Confirming or refuting one or another prediction, “whales” are able to teach a lot to game developers when creating models of one kind or another. Forecasting is improved due to player actions (recurring scenarios), and the model is aimed at advanced users. Without them, it is impossible to improve the forecasting model.
')
For many developers, the stumbling block is not a lack of data, but a lack of qualified analysts. In our university (Dmitry Williams - Ph.D. at the University of Michigan. - Approx. Ed.) This is simply not taught. We prepare either good technical specialists who can work with data, or good interpreters. And it is very rare for those who can do both.
Some researchers have gone further in this question. In my opinion, most of all there are not enough people who understand the right questions. Finding people with technical skills is easy, but taking all the business processes and adapting data to them is much more difficult. This is where sociologists would be useful.
However, the main problem is the lack of simple and effective tools for creating models. These tools do not work as “half-an-hour setup” analysis applications, which are installed quickly and easily. They are voluminous and bulky, and time is needed to create them. Game developers are not so easy to take and start using these tools: you need to thoroughly examine your players and spend a lot of time collecting useful data before the return becomes visible. You will have to deal with the integration or SDK (software development kit), and the output developed tools must be understandable and accessible at all levels of management. Easier said than done, but still this is possible.
It doesn't sound very fun, does it? As I said earlier, prediction is not magic. Game forecasting in particular is a mixture of science and hard work. However, not everything is so gloomy: a lot of good online tools appear and the process of experience accumulation is going on. At the forefront, researchers are now trying to interpret the data and build better models to bring this industry to a competitive level. And there is no turning back.
In the end, we will come to the conclusion that forecasting will turn into a commodity and become a familiar part of any information panel. I hope
social value will come close in terms of K-Score or LTV.
You may ask: if forecasting is really so valuable, should all analytics companies switch to it?
Let's say this: there is a constant struggle between performance and accessibility. Simply put (as you might have learned from previous publications), analytics become more difficult. As it develops, we get more meaningful and accurate results, but these results often go to the detriment of the ability to understand.
For example, the new models of computer science, which we are talking about, overshadow the usual sociological methods and principles of business school. Believe me, we achieve a level of accuracy of 90% using some models that you would not have created using traditional methods (for example, logit models, regression).
But there is a downside. These models are unconventional, so we do not get clear results: they are displayed in tables, conditional statements, arrays of rules and other long and incomprehensible formats. Literally no one is able to comprehend this data. I spent most of my career trying to understand the data and understand the human nature of gamers. And yet, after reading two pages full of if-then expressions, I did not understand anything.
So how can you trust models that we don't understand? After spending a lot of time researching and testing, we already just know that it works. It doesn't matter how important the result is. These models are fairly accurate, confidently tested and re-tested. This is a black box that really works.
Let's go back to the games. Imagine that you need to find out if Player A spends the money next month. You have two models: one says with an accuracy of 85% that it spends, but you cannot find out the reason, and the other with an accuracy of 40%, but it explains why. What concept will you choose?
From a practical point of view, this is a rather simple question. In each case I will choose the model with the highest percentage of accuracy. If you are persistent enough and are going to check them, you will be able to develop an algorithm during the testing process that will help you find out the cause.
Don't get me wrong: I'm an experienced model developer, and I also want to know all the answers. But if I can get models with a degree of reliability of 80% without any explanation, it will be worth it. I would like a separate area on the toolbar to answer my question “why?”, And I will work hard to make it accessible to development teams and community managers. They understand the game better than anyone else, so they need tools that can be easily learned. This is a more reasonable and practical entry into the rabbit hole.
As I said at the very beginning of a series of articles, no one can predict the future. But if we take all the available information about repetitive scenarios and forecasts, adding all the experience in data processing, we will become much closer to doing so.