As in many other applications, we have to store a lot of information in a mobile ICQ: messages, contacts, and the like. When the number of requests for this data reaches some critical value, the application begins to slow down. Long start, slow opening of chat, slow sending of messages, constant spinners - all this is terribly annoying. The most common cause of brakes is bad data handling. In the article, I want to share our experience in data structure refactoring, query optimization, and some convenient techniques for migration.
A few words about the original problem. The main entity we have is an ICQ profile, which has a list of contacts, and those have messages. Our application has existed for many years, developed by different people with different approaches, the version number of the main database confidently approached 30. In addition, the number of features in the product cannot be predicted in advance, this also affected the architecture. In general, the data model was originally something like this:
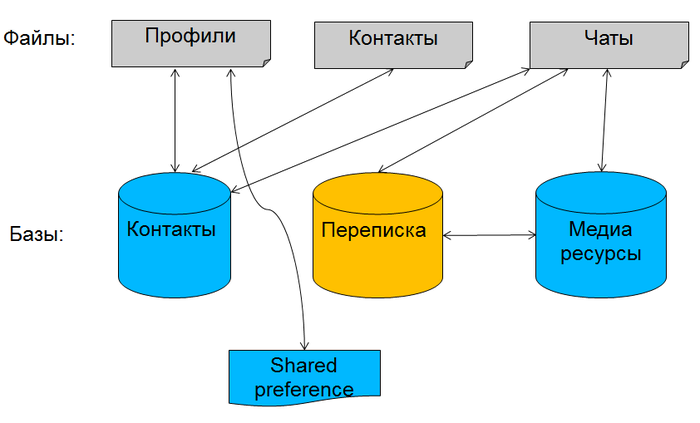
We had several files where we stored profiles, contacts and a list of active chats in binary form. The correspondence was initially stored in the database separately, for each profile it was different. At some point, contact data was transferred to a new database. Then the application added support for media messages: files, pictures and video. [irony] Logical [/ irony], a separate database was created for them. In addition, we used a piece of shared preferences, where we stored critical data about our access to the network, so as not to tie them to the main databases. Gradually, work with files began to strain. Imagine you have changed one contact, but you still have to serialize and write to the file all contacts! Once we decided that it was no longer possible to understand and develop it in this structure, synchronization problems began to appear, for example, when you need to atomically write to both the file and the database (and transactions are not available!). I wanted to put all the data into a single database and enjoy the benefits of the relational structure and the DBMS. For all data, except the history of correspondence, there were no questions with storage.
This is our approximate table with messages, we note that there are two key fields here - text and integer:
')
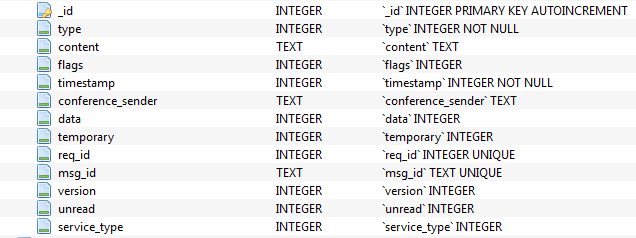
We chose between two options for storing history: a separate table was created for each contact with the structure above, or the whole history was kept in one table, and the contact information was in a separate key field. Accordingly, in the first variant, the contact id was contained in the table name. Like that:
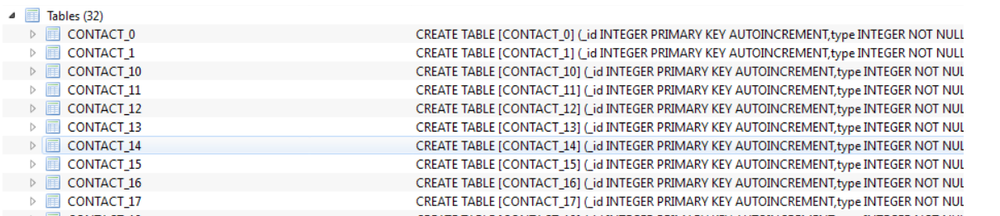
The first option is quite fast if we work a lot with one chat, i.e. when you need to read and write messages for one contact. However, the application contained a lot of code that determines which table to write to, which indexes to use, and therefore it seemed logical to transfer all of this into one big table. But worried about the performance problem. If you look for an answer to a question on the Internet, how is it better to store data in such situations, then most often the answers will be approximately as follows: “If your number of tables is small and is known in advance, it is better to break them. If you frequently access data from different tables, it is better to combine them. ” To understand exactly which structure is better, we wrote a small test application and compared the speed of the queries. Consider a real life example. The user has 30 contacts, 2000 messages in total. The active user sends an average of about 30 messages per day and receives approximately twice as much. Total 100 messages per day, that is, 20 thousand - this is about six months correspondence.
Let's create two databases: in one the data is scattered on different tables, in the second - everything is stored in one table. Immediately after creating the files, we will see that in the first case, the size of our database is almost 400 KB, and in the second - only 32 kilobytes. Why it happens? The file size of the database is always a multiple of a certain size - the size of the page. The database stores data inside the page. The page size can be configured in the system with the command Pragma page_size, in Android the default is 4 kilobytes. We experimented with this parameter, tried to increase and decrease it. The maximum page size according to the documentation is now 64 kilobytes, the minimum is 512 bytes. Any changes to this parameter led to deterioration in any tests. In fact, the size of the page in each operating system is optimized for disk operations. It is known that the data is read by sector, and this page size is best tailored to work with the disk. For more information about the file format - in the
official documentation .
After adding the test data, the sizes of our files in the pages were matched, but the approach with a bunch of tables is expected to lose.
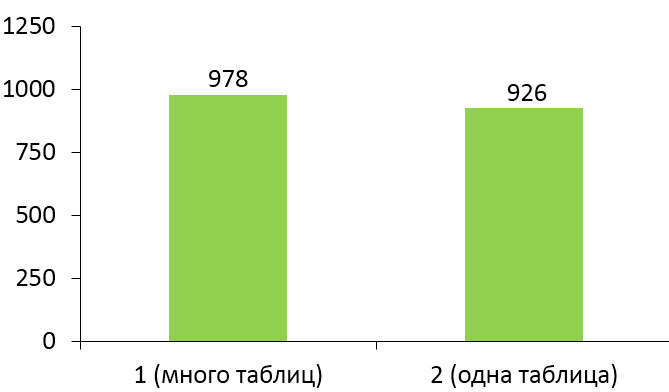
Insert optimization
Let's look at inserts options. The roughest option: messages are added in a cycle without any transactions, in both cases it will take indecent 5.5 minutes. If in our example we group up 1000 records into one transaction, then the time spent will be significantly reduced. What else can be done besides transactions? When we call the insert () method from the SQLiteDatabase class, it creates a prepared statement - a compiled SQL statement - inside the database and executes this statement for each query. This is quite expensive in terms of performance. Therefore, the SQL Statement that we use for inserts can be brought out. Every time we need only fill it with new data. If we apply this approach, we will win about 20%.
To get even greater performance gains, we need to tinker a bit with the database settings. A small technical digression on transactions. A transaction in a database should have the following properties: atomicity, consistency, independence, reliability (
https://ru.wikipedia.org/wiki/ACID ). To maintain all these properties, the database cannot write transactions directly directly to the database file on the disk. She needs to keep a separate structure - the transaction log. This is quite a powerful tool that allows you to maintain the above transaction properties.
SQLite has many interesting transaction logging modes, such as in-memory. In this mode, the transaction log is kept directly in memory, and tremendous database performance is achieved. But if the power is accidentally turned off, the data will be lost. Learn more about
transaction logs .
We will be interested in two modes of operation, in which data is securely stored. The first is the default Android mode — journal_mode rollback. If you configure it through "pragma", then it is defined as "journal_mode = delete". In this case, the database creates a special db-journal file. It writes the data needed to roll back the transaction if something went wrong. And the transaction data is written to the main database file. If the transaction is successfully completed, the journal file is flagged with a special flag that it is not used. It is important for us that in this mode the database writes to two files at once: the rollback file and the main database file.
In SQLite version 3.7 (this is Android API 16), a new transaction log mode, Write Ahead Logging, has appeared. In this mode, data is not written to the main database file, but to a temporary one, which is called db-wal. And so that the application can work with it the same way as with the main database, a file with indices is created - db-shm. It works like this. Transaction data is written to a temporary WAL file that has the same page structure. When the number of pages reaches a certain threshold value, the data from the WAL file is transferred to the main database. If the power is turned off at this moment and the data is not transferred, the next time SQLite starts, it will find this file and restore the structure. As for our test, when you turn on this mode, the performance will increase. Here we win a little, because we have only 20 transactions. But if there are a lot of them, then in different tests the acceleration can reach 40%. Therefore, the use of wal on new models of gadgets will be a good solution.
The graph below shows how much we accelerated the insertion of records.
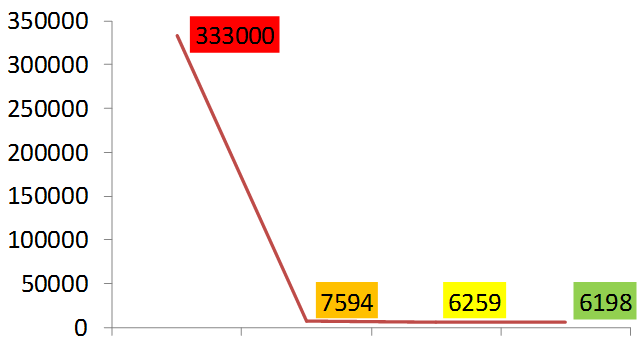
Sampling optimization
Let's look at data sampling. For our application, this is perhaps more important, since applications most often read the data. Here I will tell you pretty obvious things that most of you probably use. However, for many applications this is still relevant. First, you must use indexes. This is a special structure that is stored separately from the table, associating data by some key with an entry in the main table. When using an index, the DBMS, instead of a full scan of the entire table, first searches for the id of the desired records in the index by the key field behind O (log N), and then reads the necessary data from the table in a dotted manner.
However, even with the use of indices, much more can be accelerated. Consider this example: when launching an application, you need to read one message for each contact in order to display chats. If you simply read each message in a loop, then, for example, with 100 iterations, the total reading time will be approximately 5-6 seconds. Instead of separate requests, it is better to group the required keys into one request. For example, we can use the where in construction and pass in a list of id. However, the most effective option is to read the data from the index itself. You can store more than one field in the index. Such indices are called composite. This slows down inserts somewhat, but when selecting fields that make up an index, the database will not read two structures — the index and the main file — but only the index. If you get the data on the ID, which are already in the index, you can get a significant acceleration.
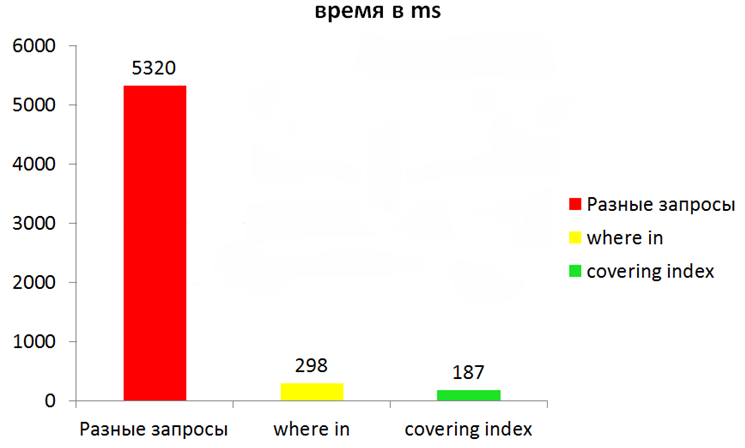
It would seem a good result, but if you turn on the wal, then under certain conditions you can speed up the application even more. The reason is that this mode uses separate data channels for writing and reading (writing does not block reading:
www.sqlite.org/wal.html ).
Not to mention a very handy tool for debugging requests. In SQLite there is a command “explain query plan”. Consider an example of this command.
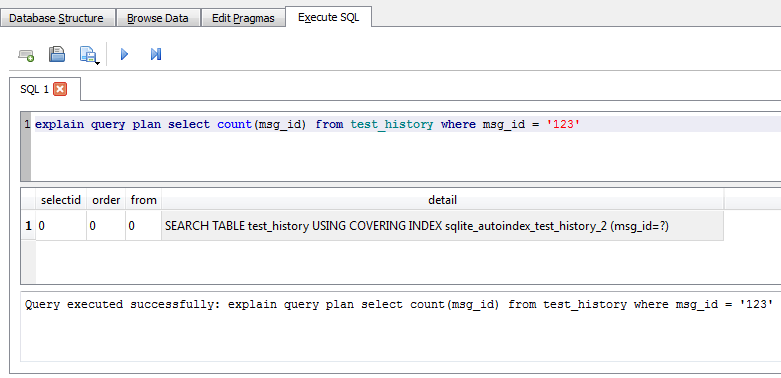
When executing this query, the DB Browser shows what the DBMS does when executing the SQL query. In this case, we see that SQLite searches the index (using covering index). This team can give us four different results:
- scan table - the database will search each record with full-text search and look for a match, this is the most inefficient option;
- search table using index - the necessary index exists for searching records, but the search data is not included in it;
- search table using covering index is the most efficient case, the search data is already in the index;
- use temp B-TREE - if we use a group by, order by type in a query, and there is no index on this field, then most often the database should do the following: get all the records that meet the criteria, and build in memory a tree for sorting this data. This is usually a slow process. If you need to group or order something, then it is better to keep an index on this field too.
I repeat: each query that you want to optimize, it is desirable to run using "explain query plan" and see what exactly the database does. We now turn to migration: it is necessary to transfer the bulky structure into a single database so as not to lose data.
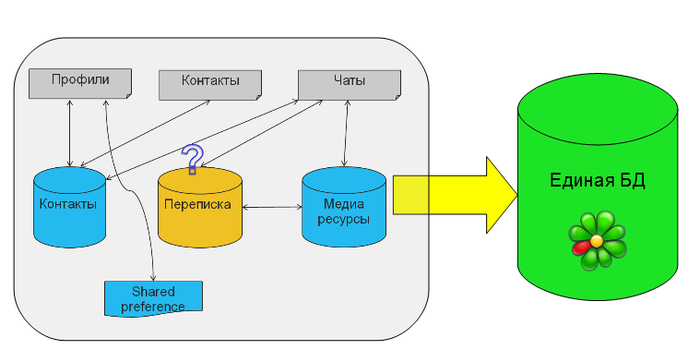
Probably, many people use the SQLiteOpenHelper class, which allows you to track changes in the database version and execute code that changes your structure in the onUpgrade method. I must say that this method is called within a single transaction. If you try to copy data in the onUpgrade method or perform any complex actions that can throw exceptions, you risk not updating anything. I recommend using this method only to change the structure. And to transfer the data itself, it is better to call a separate method.
By the way, in one of the upgrades we had an annoying mistake. We used constants in the SQL code, and at some point the constants changed. And the versions of the application from which we tried to upgrade the structure naturally worked with the old names of tables and fields. At the same time, everything in the code worked in the current upgrade from the previous version, but not in the upgrade from the version before last, because we changed the constant, and it was completely unclear where to look for the error. Therefore, we decided not to use constants in SQL queries at all.
After we updated the database structure, we needed to copy a bunch of records from different old databases. The simplest solution is to read all the old data into the cursor, parse each record and add it to the new database, but it is terribly slow. If we have a large correspondence history, tens of thousands of messages, this process takes a few seconds. The user will start to get bored, might think that the application has hung and, in the worst case, will refuse it altogether. Therefore, it was important for us to speed up this procedure. It turned out that SQLite has a very convenient “Attach database” command, which allows you to connect third-party databases to your database. To access tables from attached databases, you only need to add the name of the database to the table name. Thus, it is possible to use SQLite tools for migration without any parsing.
This approach has some limitations. The Attach database command itself, which is an extension of SQLite, does not work inside a transaction, so it will not be possible to call it within the onUpgrade method. In addition, transactions within the Attach database work only when querying for a single database. In our variant, when it was necessary to merge several databases into one, we had to work with each database into a transaction. But on the other hand, it works much faster than cursors, we could accurately transfer all the data in less than a second!
Also, after the upgrade, it's time to think about enabling effective modes of working with the database, WAL, for example. If your application supports the old API, then you need to check that the current API is higher than version 16.
It will also be useful to conduct some defragmentation of data inside the database. SQLite has a very convenient Vacuum command, it allows you to delete all unused pages and defragment data. It is no secret that if you simply execute the delete command, then the physical data from the disk will not be deleted, and records or entire pages will be marked as deleted. This command allows you to delete unused fragments and rebuild indexes.
Conclusion
So, in this article we looked at some approaches to optimizing the database on the example of the mobile application ICQ. Of course, almost all the recommendations can be found on
www.sqlite.org and other thematic resources, but the solution to a specific problem allows you to better understand what is happening in the depths of the database.