What is its measure? And what kind of useful conversion do we want to get from video analytics?
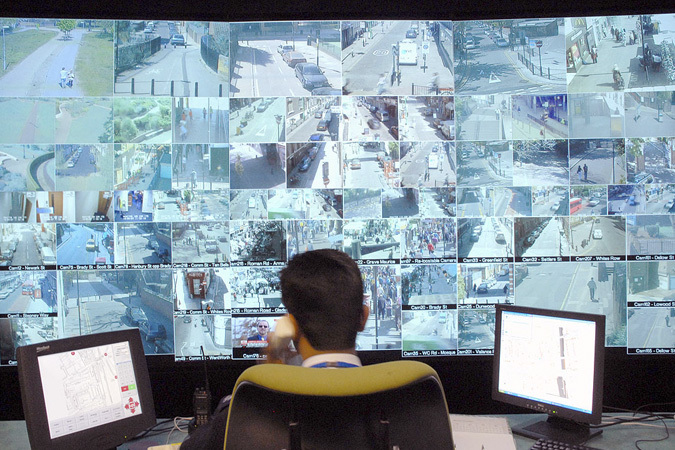
Surprisingly, all these analytical topics of articles are set by local users. Apparently, this is holding. So, to answer the previous question, which direction of video analytics is more effective (rigid or flexible), you need to decide what we will “hang”?
')
Here is a post from the user ErmIg:
"
When testing the accuracy of video analytics algorithms, as a rule, they always set an unambiguous criterion by which one can judge the correctness of video analytics in a given situation ...
For the so-called tough analytics (I will use the author’s terminology, although I only heard it from his lips), the accuracy criterion is unequivocal - different experts usually have no doubt that someone crossed the signal line or that the object is a machine or a person. A question on flexible video analytics: what is considered non-standard behavior? Different experts may have opposing views on this issue. How to quantify such analytics? ".
Thank you very much for the comments! He gave reason for this article. And I had a passing question: if we even prove as a result of tests that a tough video analyst fully performs its functions, will this indicate that it has a useful effect?
I think that in order to set the measurement criteria, you need to understand what kind of useful transaction we want to get from video analytics? According to
Wikipedia , video analytics algorithms "are most often used in video surveillance and other areas of security." What is a useful component in the most relevant area in this case - in video surveillance? How can this technology help in a specific area?
The answer is almost obvious. A person cannot capture a stream of video information falling from a dozen cameras on it every second and a fraction of seconds. Even if nothing happens, the operator should still carefully look at the monitors so as not to miss anything important. A person is simply not physically capable of doing this for 8 hours in a row. And, according to many "analysts", and 10 minutes per hour.
So (as an answer option), the
purpose of video analytics is to bring the capabilities of a person with his tasks to monitor what is happening on the screens of monitors . But is the task of the surveillance operator to keep track of everyone who crossed a line? If this task is hard video analytics useful meaning?
For example, people constantly go through the line back and forth. In front of the operator, alarm messages pop up all the time, for example, once per second. Will this solve the problem - to reduce the amount of information flow?
I agree, they took an extreme variant, we simplify. The office employs only 50 - 100 people, they go in and out only at 9 am, from 13 to 14, and at 18.00. In these three periods, the hard video analytics will not be able to simplify the life of the operator. The rest of the time, perhaps, it comes in - 3-5 people per hour. And then we have a good chance to justify the use of this expensive and nontrivial technology.
Only in the case of tough video analytics, as many have already said, we will face a high amount of interference. Dogs, cats, tree shadows on the ground, bugs on the cameras, birds, glare ... - they can all cross this line. To filter them, you need to set hard parameters of a human figure. But they are based on a contrasting video detector, which sees frame changes only relative to the background and, depending on the color of the clothes, the activity of a person’s limbs, the number of moving targets, falling shadows and much more, can perceive the human figure as a large car and as many small bird type targets. Conversely, a group of pigeons on asphalt will create a detection frame - identical to a human one.
I would like, of course, to assume that all these problems arise only on some bad days or at night, but even the sun can give such drops of shadows from trees that you cannot filter them out by any means: the sun does not have a permanent location - the shadow is always There are no regularities in the speed of appearance due to clouds in different places - there are many clouds in Russia. There are no exact parameters of the shadows - from different sides the trees will have different sizes and shapes of the shadow. And with the breath of breeze over the foliage, the picture is complicated hundreds of times.
Naturally, video analyst developers declare all sorts of filters of all kinds of interferences, but in reality, interfering questions pose a dilemma: get away from interference as much as possible and respond only to a clearly defined figure and close your eyes to some part of people or lose less useful goals, but at the same time take more interference (crap).
In fact, the above partly says that a tough video analytics on the street is generally useless, because will score the operator with false positives at the same level as regular video surveillance. But we also have interior rooms, how will it behave there?
However, there are also shadows in the rooms, as well as a number - albeit significantly smaller than on the street - of their own interference. In addition, the object detector has not yet learned to recognize group targets, which is detrimental to both street and internal video surveillance. Although, it does not make sense in the premises to assume that instead of people, a car will travel, therefore, any sufficiently large detection frame can be displayed to the operator for viewing.
Only now we have forgotten that the office employs 50 or even 100 people, and they all walk around the premises during working hours. Those. the operator will be loaded with regular hits! As a result, from 9 to 18 there is no sense from such video analytics either. Although I really want to hear at least one argument against these calculations.
We now fling through a flexible video analytics - interpreted by Wikipedia as VIDEOSEMANTIC.
It allegedly does not depend on interference, because interference is part of the statistics on which the analysis is based. Those. there were interferences - there were interferences, the statistics did not change. Appeared different from interference information - drawdown. However, what is the size of the statistics database you need to keep in yourself? At a minimum, it is required to record interference in the last week and from all cameras. It is possible to record something, perhaps, but to make a selection on the fly? There is hardly any such processor power. Although indexing algorithms simplify this approach, but naturally reduce the accuracy of the data.
In the short-term mode, the statistics still, probably, win, because the recurring nature of interference on this day precisely under these weather conditions, precisely at this light and for all other factors will most often be unchanged. But this does not negate dogs and cats or birds on the pavement.
Although there are significant advantages. The triggering on the same cat will most likely be only one - no matter how much she climbed in a certain period of time. Just because the nature of the frame of detection, movement, color gamut, and other parameters on which the video semantics is based will be little changeable. Those. instead of constant duplication of interference - as in hard video analytics, only one false trigger will occur. Although only in a limited period of time, the gain can still be counted.
In general, although this can be talked about for a long time, video semantics does better with harsh video analytics - much better, but does flexible analysis have a useful component, but does it take the necessary goals? And does it reduce the flow of unnecessary information that falls upon the operator, when in front of the cameras, 100 people are constantly walking in the office or in the street?
It turns out that video semantics does not respond to them at all, just does not notice - that's the number! It is also based on a contrast video detector - simply because there is nothing else in video surveillance and there cannot be (at least in the visible spectrum). And so for her: that people, that interference is one and the same content, no difference. Well, or almost no, of course, some elements of the hard video analytics are there - the same object detector, but we already know that it works uselessly. In video semantics, in principle, there are algorithms of hard video analytics, but only for statistics, they are included in the general analysis of statistics. So who does she catch?
Does not catch anyone, does not report any line intersections. Quietly about the fact that people cross them, if they went to dinner. But any movement in the same area or in another, which was not previously met in statistics, causes a drawdown. Those. from 9 to 13 and from 14 to 18, video semantics will regularly respond to 3–5 people per hour defined by the conditions of the problem, because according to statistics, crowds do not go at this time. And it will not get hammered - to the extent that a tough video analytics.
Thus, we receive already working variant which gives out useful transaction. And what will happen at lunch and at the time of arrival - leaving from work? Yes, even the close attention of the guard to the monitors is not much help in finding troubled moments. A computer intelligence can not be higher than human. Although…
Video semantics will be:
1. Indicate to the operator at the beginning of a busy period - the appearance of a crowd.
2. At the end of such.
3. The deviation of the trajectories, speed and actions of individuals from the general flow, which theoretically could indicate danger.
Those. during this period video semantics also works to some extent. It does not alarm every second with an operator, but gives only deviations from the standard one — the character of behavior present in statistics. Yes, maybe there will be no crime there, but who spoke about crime here? At the beginning of the article, we decided that the task of video analytics is to reduce the useless flow of information.
Also analyzed and other movements around the office. To the security guard or archive viewer (security chief), video semantics displays only deviations of people's actions (and sometimes noises — much less than in hard video analytics). Thus, the operator is able to identify unauthorized behavior and even preparation for it, because it usually requires non-standard actions: movements in rarely visited areas, movements of rarely used objects, raising - lowering things from rarely used places, other trajectories, other speeds, other conditions illumination with the same actions ...
But suddenly I hear an indignant question: does video semantics solve the anti-crime task if the attacker completely adapts to the usual behavior of the crowd? And here I myself want to ask another question: will even the most keen operator notice such a phenomenon? Let me remind you, we are still solving the problem - to make video surveillance convenient for a person, and not to surpass a person.
However, attempts are being made in this direction, moreover, both from rigid and flexible video analytics, but this is the topic of the next article, if they are not banned (some karma remain — oh, not everyone likes these conclusions.
PS:
I just want to note that video semantics, besides that wins in the fight against interference, does not require complex settings, as in a tough video analytics. Video semanta is based on statistics - and therefore, on self-study. This is especially important when the camera view is shifted (from the wind), and when weather conditions change, the seasons are winter - summer, when the noise situation changes radically and everything needs to be reconfigured. Video semantics is simple and unpretentious, which makes it more practical from the point of view of the conclusions of this article. IMHO.
Go ahead?