It so happened that in recent years I personally have to deal more and more with various government texts, especially laws and financial documents, such as budget justifications, as well as trying to translate them from non-human clerical language into words or visual schemes that would be understandable to me and To whom I then talk about what it is.
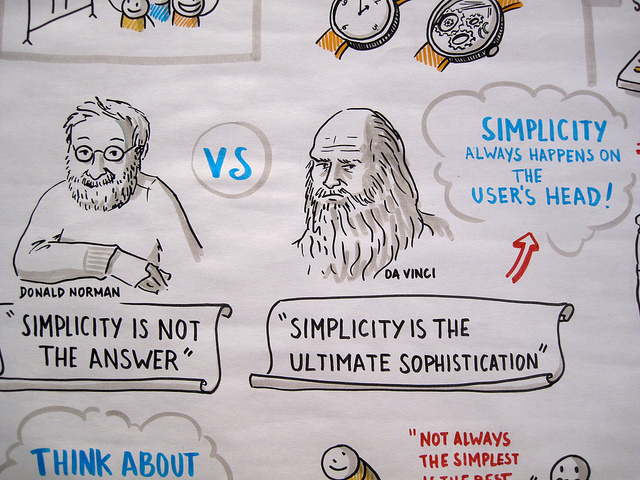
For all this time, I personally had the persistent feeling that the Russian language was divided into two of its subspecies - dry office legal official language and the "language of the Internet", with newly-formed words, jargon and significant personification. Together with the constant feeling that “life cannot go on like this”, all this led me, first to search for the correct name for what it is called, and then to what people who can program can do with it.
')
A bit of history
On January 18, 2011, President Obama issued a new decree, Executive Order, “EO 1356 - Improving Regulation and Regulatory Review” (“Improving Regulation and Regulatory Considerations”). It states that "[our regulatory system] must ensure that the rules are accessible, consistent, written in
simple language , and easily understood.".
Written in
simple (understandable) language is not a common term and not a turn of speech. This is an approach formulated over decades to translate official texts, documents, speeches of politicians, laws, and everything that is filled with official meaning, in a form understandable to mere mortals.
Understandable language is a clear, concise writing intended for the reader to understand the text as quickly as possible and as fully as possible. It avoids excessive detail, confusion and jargon.
While “plain” in English means “simple”, but in Russian, the word “understandable” is closer to translation, you can also say “clear” or “simple” language.
An understandable language, in English -
plain language , a phenomenon that initially sounded like “plain English” in English-speaking countries, but very quickly developed into a global phenomenon with international ones.
Now in the world there are several dozen organizations that are engaged in the dissemination of the ideas of language comprehensibility. In many countries, laws are passed, books are published, official state instructions are published on how to write in plain language, and annual awards are held for the most comprehensible and most incomprehensible text.
But all this is not in Russia and, in order to understand that it is in the world, we will try to figure out how it works.
What is the clarity
All that is around the intelligibility of the language fits into two terms - measurement and change.
Measurement is the evaluation of a text for simplicity (“readability”, “readability”). It is necessary in order to understand whether it is necessary to simplify the text in the future or not, or to check how successfully the text has been simplified. Examples of measurement are readability formulas, special tests for schoolchildren / students on how much they can retell the read text in their own words and other ways of determining how much the text was understood by the readers.
Change is the next step after measurement. This is editing the text according to the rules, approaches and recommendations so as to simplify it as far as possible without losing meaning. Examples of change are special programs that automatically replace some verbal turns, these are books of instructions on how to correctly rewrite complex texts, these are dictionaries of “simple language”, these are approbations of texts on an age audience before publication.
Of course, almost everyone can appreciate the clarity-incomprehensibility of the text for themselves subjectively, and many can even correct complex texts.
But we will talk about what is closer to us. About the methods of measuring and modifying texts that can be automated. First, on this method of measuring complexity as readability indexes
Readability indices
Readability indexes are mathematical formulas created to assess the complexity of the readability and understanding of texts. As a rule, these formulas use textual metrics that are simple in measurement - the number of sentences, the number of words, the number of letters and syllables, on the basis of which they give a numerical estimate, of either the complexity of the text or the expected education of the audience.
Test Flash Kinkaid (Flesch-Kinkaid Readability Test)
This test was originally based on the
Rudolph Flash test for assessing the complexity of English texts and was developed by
Peter Kinkaid under a contract for the US Navy.
The test is based on the thesis that the fewer the words in sentences and the shorter the words, the simpler the text is.
The calculation formula is as follows.

and uses 3 parameters:
- total words
- total sentences
- total syllabes - total syllables.
The result is the number of years of study required by the American graduation of education to understand the text.
Please note that this is only an assessment of words and sentences, but not their meaning. This formula, like all subsequent ones, is made for the natural texts found in life. Because you can always write complete meaninglessness from short words and sentences that no one needs.
Coleman-Lian Readability Test
This test was developed by Meri Coleman and TL Liau for a simple and mechanical assessment of the complexity of texts. Unlike the Flash-Kinkaid test and the many ways to assess readability, it does not use syllables, but the letters and calculation formula take into account the average number of letters per word and the average number of words per sentence.

L is the average number of letters per 100 words.
S - the average number of sentences per 100 words
SMOG test (SMOG grade)
The SMOG formula was developed by Harry McLaughlin in 1969 and published in
SMOG Grading - a New Readability Formula.The idea was that the complexity of the text is most influenced by complex words that are always words with many syllables and the more syllables the harder the word.
The final SMOG grade formula took into account the number of polysyllabic words with 3 or more syllables and the number of sentences. In fact, this is an estimate of the proportion of complex words to the number of sentences.

It so happened that the SMOG formula was most widely used in assessing the complexity of medical texts and in subsequent studies it showed greater accuracy compared to the Flash-Kinkaid formula.
Formula Dale-Chall (Dale-Chale readability formula)
This formula was developed in 1948 by Edgar Dale and Joan Chall on the basis of a list of 763 words with 80% of which most of the 4th grade students were familiar with, thus defining difficult words. In 1995, an updated formula of the same test appeared, which already took into account 3,000 recognizable words.
The formula itself is calculated quite simply.

However, due to the specifics of the assessment, it was mainly used and used to check texts for schoolchildren from the 4th grade.
Automated Readability Index (Automated Readability Index)
This formula was published in 1967 and, like the Coleman-Liau formula, was built on an assessment of the complexity of texts by the number of letters. This made it possible to use the formula in electric typewriters to measure the complexity of texts in real time.

Other formulas
In addition, there are many formulas behind the brackets that are also actively used:
- Fry readability formula
- Gunning fog index
- Spache Readability Formula
- Raygor Readability Estimate
- Linsear write
- Lexile
- LIX
- Flesch Reading Ease Readability Formula
- FORCAST
All of them are calculated on similar principles and many of them are actively used in practice.
Learn more about the basic readability formulas on Wikipedia:
https://en.wikipedia.org/wiki/Category:Readability_testsWhile the readability formulas are for different languages ​​- for German, Japanese, Swedish, Portuguese and others, but there is no such diversity for them as for English.
On practice
- The US Social Security Administration has released a special compliance report on language comprehensibility and, in particular, their employees use special software - StyleWriter to help evaluate and simplify texts. SSA-2013 Plain Writing Compliance Report
- The Oregon State Administration checks and verifies all texts they publish to the level of grade 10 school - Oregon Readability
- The Virginia Code contains mandatory readability requirements for all life and accident insurance contracts and a Flesch-Kinkaid Virginia Codex 38.2 readability check.
- A huge number of publications, including government research, are dedicated to readability formulas science.gov grade level readability
And what about the Russian language?
The Russian language and, accordingly, the texts in Russian differ from the texts in English by syllables, by the length of sentences, by the number of characters, according to which words can be considered complex. In particular, in Russian words are usually longer, but sentences are shorter. In common speech, more complex words and coefficients in the formulas should be different.
When I first became interested in the topic of language clarity, I, first of all, tried to find publications on this topic and any examples of implementations for the Russian language. It turned out that they are absent almost completely. While in Russia there are many strong teams in computational linguistics, in particular, in the analysis of texts, but it is in the field of
understandable language that there is almost a vacuum.
Deciding what to do with it, I decided to go two ways at once. The first way is to find those who are interested in dealing with this topic, and the other is to deal with readability in those areas that I understand.
The search is ours, NP “Information Culture”, this year’s annual contest
Apps4Russia with its theme we took the topic of comprehensibility in general. And the clarity of the Russian language is one of the nominations. The main prize in the nomination is 100 thousand rubles, the second place is 50 thousand. You can win them if you make a tech project in this area. Develop your own formula, make instructions, conduct a study on the level of readability, for example, conditions of use on websites, improve the existing formula, make a browser service for correcting or measuring texts or a special web service. There are many options, nothing more is needed except to think a little over the idea and put it into practice.
But the second direction - this is what led me to that before creating dictionaries of clarity and instructions for rewriting complex languages. And before you talk to the officials about what they say in the creepy office. First of all, it is necessary to make readability assessment formulas for the Russian language.
Having fiddled a bit with modeling coefficients, I rather quickly came up against the fact that American formulas were developed practically with accompanying testing in schools and universities. They were made as scientific studies and scientific articles were published on them. In other words, everything was according to science. I have not yet had the opportunity and resources to conduct offline testing and it took a lot of time to find the right approach.
It consists in choosing the right coefficients by means of texts that were previously identified for which audience they were written for. The most obvious thing here was to take the texts of home reading. All of them have, usually, an exact mark for which class they are intended. Already known hard-to-read official texts that I used as examples were added to them.
To verify this, an assumption was made that there is a relationship between the parameters involved in readability assessment formulas. And, in particular, that the more words in sentences, the more syllables in them.
This thesis was tested and it turned out such graphs
The dependence of the complexity of the text on the average number of syllables
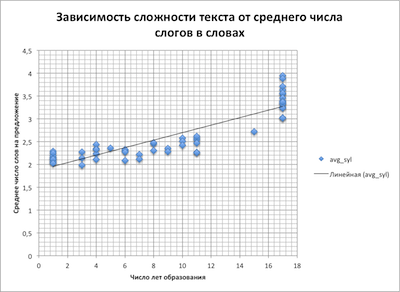
Dependence of text complexity on the average number of words in a sentence

The dependence of the average number of syllables per word on the average number of words in the sentence
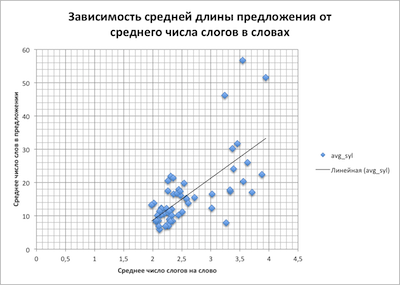
Total turned out 55 texts for which we knew:
- level of education necessary to understand them
- qualitative metrics for each text: average number of syllables per word, average number of words per sentence, average number of letters per word, and so on
Further, the task was to turn over the formulas and solve the system with 3 unknowns and 55 formulas.
The constants in the formula were considered as unknown, and the parameters were taken for each text.
There was only one simple task - the selection of these constants.
Perhaps she had and is and a beautiful mathematical solution, but I personally decided everything in the forehead according to the following scheme:
- for constants, the range of their probable values ​​was set in increments of 0.0001
- for each triple of constants, readability metrics were calculated using the selected formula
- further calculated deviation from the correct value for each text
- deviations for all texts were recalculated and the average deviation of the array was obtained
As a result, of all the variants of the constants, we selected those for which the average deviations are minimal.
All this modeling and calculations took several weeks. But in the end, it was possible to adapt almost all algorithms for the Russian language, with the only proviso that until they pass a full-fledged experimental test, then all the values ​​of the formulas are conditional.
I will give the result for one of the formulas - Automated Readability Index
The values ​​of the constants there are 6.26, 0.2805 and 31.04.
Since Russian is shorter than sentences, the constant is greater for the average number of sentences per word, since words are longer, the constant is less for the average number of letters per word. Plus, a correction factor has been selected that helps to align the text for evaluation of the level of education.
This is what the Python source code looks like for its calculation.
ARI_X_GRADE = 6.26 ARI_Y_GRADE = 0.2805 ARI_Z_GRADE = 31.04 def calc_ARI_index(n_letters, n_words, n_sent): """ Automated Readability Index (ARI) """ if n_words == 0 or n_sent == 0: return 0 n = ARI_X_GRADE * (float(n_letters) / n_words) + ARI_Y_GRADE * (float(n_words) / n_sent) - ARI_Z_GRADE return n
All formulas are implemented as an online service -
ru.readability.io . Actually all adapted formulas were tested there, which I continue to develop and correct. There is an API and the ability to get readability metrics for any text.
And for everyone who wants to want to develop their own formulas on Github, there is all that training sample of texts
https://github.com/infoculture/plain Russian / and metrics calculated from them.
Simple but not too easy
Readability indices are, of course, cool and useful stuff, but they are also very limited. In the western practice of working on language clarity, it is always mentioned that formulas should be used carefully, not relying on them for 100% because they can sometimes give erroneous or insufficiently accurate results. Therefore, despite widespread use, the question arises about their development.
And here I would like to discuss this question here on Habré.
What other approaches can we use to assess the complexity of texts?
Maybe some typical stationery speech turns?
Could there be complex reconciliations in the inside of a sentence?
Surely there is something that will allow you to move on.
* Image under Creative Commons 2.0 Attribution, Sharealike
* original - secure.flickr.com/photos/visualpunch/8746310544